Abstract
Working across companies in regional clusters has become a common practice, but there is limited research on the socio-psychological processes that bind cluster actors. Therefore, this study investigates the factors that hold regional clusters and their actors together. We combine research on social identity theory and organizational citizenship behavior with cluster research to advance knowledge of identification processes and citizenship behaviors in regional clusters. The results of a survey of cluster actors in a sensor technology cluster in Germany show that not all antecedents of identification considered important in organizational contexts play a role in cluster actors’ identification with the cluster. Cluster distinctiveness, visible cluster affiliation, and group formation are relevant but cluster prestige and inter- and intra-cluster competition are not. The results also emphasize the importance of cluster identification for cluster actors’ willingness to exhibit citizenship behavior and the importance of these behaviors as predictors of sustained cluster effectiveness.
Introduction
Digital transformation, networked value creation, and modern information and communication technologies (ICT) have changed the business environment, creating considerable challenges for today’s organizations (Hess et al. Citation2016). In these demanding environmental conditions, working across organizations in regional clusters has become a common practice (Provan, Sydow, and Podsakoff Citation2017). Scholars have argued that goal-directed networks, such as regional clusters, are becoming the new dominant organizational form, which is why “Western societies are moving towards a society of networks” (Raab and Kenis Citation2009, 198). Interdependent companies partner and collaborate in regional clusters, which Williams (Citation2002, 105) called “a postmodern form of organization,” to jointly develop new technologies and keep up with the transformations. These clusters provide working conditions that allow institutions and companies to meet new challenges. A regional cluster is “a geographically proximate group of interconnected companies and associated institutions in a particular field, linked by commonalities and complementarities” (Porter Citation2000, 16). Clusters enhance companies’ innovation skills, competitiveness, and growth through positive externalities, such as lower transportation costs, technological and knowledge spillovers, and an infrastructure that attracts specialized suppliers and highly skilled workers (Marshall Citation1920; Mueller and Jungwirth Citation2016; Sölvell Citation2008). To promote these externalities, policy programs at the regional, national, and supranational (e.g., European smart specialization strategies) levels support cluster genesis and development (e.g., Balland et al. Citation2019).
From a governance and coordination perspective, regional clusters correspond to voluntary cooperation agreements between independent organizations that typically do not rely on hierarchy or powers of direction (Hardy, Lawrence, and Grant Citation2005; Lorenzen Citation2001; Kenis and Raab Citation2020; Mueller Citation2021; Sydow et al. Citation2011). Therefore, cluster-facilitating agencies, which manage the clusters (Hurmelinna-Laukkanen, Möller, and Nätti Citation2022), do not have effective incentives or sanctioning mechanisms to stimulate behaviors that benefit the entire cluster. Given the absence of these governance mechanisms, it is important to examine the socio-psychological processes that bind cluster actors together and induce such citizenship behaviors. In this study, we define cluster actors as individuals who represent their organizations (Peteraf and Shanley Citation1997) while acting as boundary spanners (Scott, Hughes, and Kraus Citation2019; Williams Citation2002) between their organizations and the regional cluster.
In a regional cluster, a shared cluster macroculture conveys general norms, values, and practices to the cluster actors and guides their behaviors (Bell, Tracey, and Heide Citation2009). Based on this macroculture, regional clusters can develop a collective identity (Raab and Kenis Citation2009). Only recently, however, have scholars begun to explore the “emotional microfoundations” (Aversa, Furnari, and Jenkins Citation2022, 1340), i.e. the people’s emotional attachment to locations, that contribute to the formation of a collective identity in regional clusters and ultimately lead to the production of the desired macrolevel cluster outcomes. With this study, we aim to address this need for research and gain insights into the socio-psychological processes that promote long-term cohesion at the cluster level.
We combine propositions from social identity theory and research on organizational citizenship behavior (OCB) with cluster research to determine what holds a regional cluster together. We draw on socio-psychological concepts derived from the literature on organizations, such as organizational identification, to investigate whether cluster actors identify with regional clusters. In addition, we examine how such an identification promotes cluster citizenship behavior (CCB) that benefits the entire cluster and enhances sustained cluster effectiveness. The results of our survey of cluster actors from a sensor technology cluster in Germany showed that not all antecedents of identification considered important in the organizational context were important for cluster actors’ identification with a cluster. The results also emphasized the importance of cluster identification for the cluster actors’ willingness to exhibit CCB and the importance of these behaviors as predictors of cluster effectiveness.
With this study, we contribute to research on regional clusters, particularly to research on cluster identification and CCB. First, we add to the ongoing discussion of intentional governance of interorganizational (regional) networks (e.g., Dagnino, Levanti, and Mocciaro Li Destri Citation2016) by showing that in regional clusters without formal governance mechanisms, socio-psychological mechanisms, such as cluster identification and CCB, can serve as informal governance mechanisms. These informal governance mechanisms in turn stimulate behaviors that foster the cluster’s effectiveness. We conceptually and empirically extend social identity theory and examine the relevance of its propositions for entire clusters. Specifically, we investigate the antecedents of cluster identification and distinguish them from the well-known antecedents of organizational identification. Second, this study develops a new concept, CCB, by applying OCB to interorganizational regional clusters. We analyze cluster identification as an antecedent of the novel CCB construct and contribute to the literature on citizenship behavior by opening up avenues for further research on citizenship behavior at the interorganizational level (e.g., Provan, Sydow, and Podsakoff Citation2017; Gerke, Luzzini, and Mena Citation2021). Taken together, these contributions are essential to better understand processes and behaviors at the actor level that in the aggregate are important to the overall sustained effectiveness of a regional cluster.
The rest of this paper proceeds as follows. First, based on an extensive literature review, we develop our hypotheses regarding the factors influencing cluster actors’ identification with the regional cluster and the relationships between cluster identification, CCB, and cluster effectiveness. In the research design section, we present our study setting, data collection process, and measures. Next, in the methods and results section, we explain how we apply structural equation modeling to test our hypotheses with a sample of 102 actors from a German sensor technology cluster. Finally, we conclude the paper by discussing the findings, implications, and limitations of this study and provide suggestions for future research.
Theoretical background and hypothesis development
Our theoretical considerations are guided by the need to apply socio-psychological concepts in an interorganizational context, which requires both a deep understanding and accurate transfer of concepts.
Social identity theory and the antecedents of cluster identification
Social identity theory posits that individuals construe their self-concept according to the social categories they fall into or perceive themselves to belong to (Tajfel Citation1978). Identification is an active process through which individuals come to define themselves in terms of a perceived category or social group. Through such self-definition, people come to see themselves as belonging to an in-group (Mael and Ashforth Citation1992). Organizational identification is a form of social identification and is defined as “the degree to which a member defines himself or herself by the same attributes that he or she believes define the organization” (Dutton, Dukerich, and Harquail Citation1994, 239). Organizational identification means experiencing the organization’s status, success, or failure at a personal level because the organization has become part of one’s self-definition (Mael and Ashforth Citation1992).
The literature has identified four antecedents of organizational identification. First, the distinctiveness of the organization’s values, structures, and practices from those of comparable organizations (out-groups) differentiates the organization from others and provides the organization with a unique identity (Ashforth and Mael Citation1989). Second, the prestige of an organization increases an individual’s organizational identification, as belonging to a prestigious group enhances their self-confidence (Ashforth and Mael Citation1989). Third, identification is likely to be strengthened by the salience of out-groups. The mere awareness that people have of out-groups augments their awareness of their in-group (Ashforth and Mael Citation1989). This mechanism is reinforced by the perception of competition between the focal organization and other comparable organizations, but is weakened by competition within the in-group, as it emphasizes members’ differences rather than their oneness and cohesion (Mael and Ashforth Citation1992). Fourth, a set of group formation factors—interaction, similarity, liking, proximity, shared goals, and common history—can be used to categorize and support the organizational processes of formation and boundary definition (Ashforth and Mael Citation1989).
Building on the literature on group identity and identification in interorganizational settings, such as multi-organizational projects (Beech and Huxham Citation2003), supply chains (Ellis and Ybema Citation2010), strategic groups (Peteraf and Shanley Citation1997), industrial districts (Sammarra and Biggiero Citation2001), and regional clusters (Staber Citation2010), we define cluster identification as cluster actors’ perception of oneness with and belongingness to a regional cluster. The regional cluster represents a social category to which cluster actors perceive themselves as belonging. This study extends the literature on organizational identification by hypothesizing the potential drivers of cluster identification: perceived cluster distinctiveness, perceived cluster prestige, out-group salience, and group formation factors.
First, cluster actors are likely to identify strongly with a cluster if they perceive it as having a unique identity and distinct values, structures, and practices from other regional clusters (e.g., Sammarra and Biggiero Citation2001; Staber Citation2010). Due to the perceived distinctiveness of the in-group (the cluster), cluster actors ascribe prototypical characteristics to other cluster actors with whom they do not necessarily have a personal relationship and expect them to behave in line with the shared cluster identity, which reduces uncertainty (Anand, Joshi, and O'Leary-Kelly Citation2013).
Second, following Mael and Ashforth (Citation1992), the more prestigious the cluster is perceived to be, the more motivated the cluster actors are to identify with it. A cluster’s prestige, high status, and good market reputation are extended to the cluster actors, who benefit from this positive externality (Sammarra and Biggiero Citation2001).
Third, identification is likely to be reinforced by out-group salience, because if cluster actors are more aware of potential out-groups (other regional clusters), they are also more aware of the in-group’s attributes and boundaries (Anand, Joshi, and O'Leary-Kelly Citation2013; Ashforth and Mael Citation1989; Peteraf and Shanley Citation1997). Therefore, identification with the cluster is stronger if the cluster actors’ affiliation with the cluster is visible; for example, through a cluster brand that increases visibility among external stakeholders (Mauroner and Zorn Citation2017), and if competition with other clusters (inter-cluster competition) is strong. In these contexts, the cluster boundaries are clear, and the cluster as an in-group appears homogeneous. In contrast, competition between cluster actors (intra-cluster competition) weakens cluster identification, as cluster actors are more inclined to focus on competing firms rather than on the cluster.
Fourth, group formation factors provide the basis for self-categorization (e.g., Hogg and Turner Citation1985; Turner Citation1984) and are likely to strengthen cluster identification. The perceived similarity between organizational and cluster identity is one of the factors that promote group formation (Anand, Joshi, and O'Leary-Kelly Citation2013; Dutton, Dukerich, and Harquail Citation1994; Sammarra and Biggiero Citation2001). Congruence in organizational and cluster identity reduces complexity, increases mutual understanding, and endorses one’s self-concept (Peteraf and Shanley Citation1997). Interaction between cluster actors is another important group formation factor, as regular interaction fosters mutual observation, comparison, and understanding, which emphasizes commonalities between cluster actors (Peteraf and Shanley Citation1997; Sammarra and Biggiero Citation2001; Staber Citation2010). Similarly, geographical proximity facilitates formal and informal interactions between cluster actors, stimulates benchmarking, learning, and imitation processes, and promotes the alignment of values and behaviors, all of which result in stronger group formation dynamics (Peteraf and Shanley Citation1997; Sammarra and Biggiero Citation2001; Staber Citation2010). Another group formation factor that strengthens group identification is the perceived benefit of affiliation with a group. The perception that group membership, or cluster membership, is valuable for oneself supports group formation (Riketta and Nienaber Citation2007). Last, the longer a person remains in a group, or a regional cluster, the more salient that group membership becomes for self-categorization, especially when comparing several group memberships against one another (Dutton, Dukerich, and Harquail Citation1994; Mael and Ashforth Citation1992).
This discussion leads us to formulate the following hypotheses concerning the antecedents of cluster identification.
Hypothesis 1a:
Perceived cluster distinctiveness is positively related to cluster identification.
Hypothesis 1b:
Perceived cluster prestige is positively related to cluster identification.
Hypothesis 1c:
Visibility of cluster affiliation is positively related to cluster identification.
Hypothesis 1d:
Perceived inter-cluster competition is positively related to cluster identification.
Hypothesis 1e:
Perceived intra-cluster competition is negatively related to cluster identification.
Hypothesis 1f:
Group formation factors (such as similarity, interaction between cluster actors, geographical proximity, perceived benefits of cluster affiliation, and length of cluster affiliation) are positively related to cluster identification.
From OCB to CCB
According to social identity theory, individuals engage in activities that support the organizations they belong to and identify with (Ashforth and Mael Citation1989; Mael and Ashforth Citation1992). Organ (Citation1977) first argued that individual OCB benefits organizations because it supports the social, psychological, and organizational environment in which task performance takes place. OCB is defined as “individual behavior that is discretionary, not directly or explicitly recognized by the formal reward system and that in the aggregate promotes the effective functioning of the organization” (Organ Citation1988, 4). This widely used definition (e.g., Aggarwal and Singh Citation2016; Esper et al. Citation2015) implies that OCB is a voluntary and non-enforceable behavior and is not specified in the individual’s employment contract with the organization.
Supply chain management scholars were the first to study OCB in an interorganizational context, in the form of interorganizational citizenship behaviors (ICB) in dyadic relationships between supply chain partners (e.g., Autry, Skinner, and Lamb Citation2008; Esper et al. Citation2015; Skinner, Autry, and Lamb Citation2009). However, little is known about stimulating and leveraging citizenship behavior in contexts involving more than two organizations, such as networks or regional clusters. This is surprising given that multi-organizational networks are quite common and that it is important to stimulate beneficial behaviors for the entire network when formal governance mechanisms are lacking (Cassanego et al. Citation2019). One exception is the study by Provan, Sydow, and Podsakoff (Citation2017) on the structures and conditions under which individuals with boundary spanning roles show network citizenship behavior (NCB) in a multi-organizational network. The boundary spanners’ NCB can be targeted at individuals outside the focal organization but within the network, other organizations in the network, or the entire network (Provan, Sydow, and Podsakoff Citation2017).
This study focuses on regional clusters, which are voluntary cooperation agreements that do not rely on market- or hierarchy-based control or governance mechanisms (e.g., Hardy, Lawrence, and Grant Citation2005; Kenis and Raab Citation2020; Lorenzen Citation2001; Mueller Citation2021; Sydow et al. Citation2011). Their long-term functioning and effectiveness depend on cluster actors engaging in cluster-conducive behavior. In this context, citizenship behavior serves as an informal governance mechanism, as it is defined as voluntary behavior that cannot be stimulated by incentives or sanctioning mechanisms but is key to the functioning and effectiveness of the entity it refers to. Following scholars who have extended the OCB concept to contexts beyond the organization (e.g., Esper et al. Citation2015; Gerke, Luzzini, and Mena Citation2021; Provan, Sydow, and Podsakoff Citation2017), we define CCB as follows.
Individual, cluster-conducive behavior exhibited by cluster actors (in their role as boundary spanners) that is voluntary and discretionary, not directly or explicitly part of a formal agreement with the cluster, and promotes the effective functioning of the regional cluster as a whole.
Several scholars have discussed the dimensions of the OCB construct. Podsakoff et al. (Citation2000) categorized different OCB-related behaviors into seven dimensions: helping behavior, sportsmanship, organizational loyalty, organizational compliance, individual initiative, civic virtue, and self-development. Based on this study and other studies on ICB and NCB (e.g., Autry, Skinner, and Lamb Citation2008; Skinner, Autry, and Lamb Citation2009), we conceptualize seven dimensions of CCB: helping behavior, tolerance of mistakes, cluster loyalty, cluster compliance, cluster-supporting initiative, cluster participation, and relationship management.
The first dimension, helping behavior, is defined as voluntary behavior “directed at helping a partner firm in solving problems or acquiring needed skills/knowledge” (Autry, Skinner, and Lamb Citation2008, 56). Cluster actors support each other in work-related problems and provide help in areas where they have the relevant expertise. The second dimension is tolerance of mistakes and neglect of duties without retribution (Autry, Skinner, and Lamb Citation2008; Organ Citation1988; Skinner, Autry, and Lamb Citation2009). In an organizational context, this dimension is often referred to as sportsmanship, implying that sportspeople “do not complain when they are inconvenienced by others, but maintain a positive attitude even when things do not go their way” (Podsakoff et al. Citation2000, 517). The third dimension, cluster loyalty, refers to cluster actors’ allegiance to the cluster, expressed by the actors’ willingness to defend the cluster or to speak about it in positive terms (Autry, Skinner, and Lamb Citation2008; Podsakoff et al. Citation2000). The fourth dimension, cluster compliance, refers to cluster actors’ compliance with cluster norms and rules and an orientation toward the policies and processes of other cluster actors even when no one is watching (Autry, Skinner, and Lamb Citation2008; Podsakoff et al. Citation2000). The fifth dimension, cluster-supporting initiative, involves engaging in cluster-related tasks with “higher than normal levels of forethought and effort” (Autry, Skinner, and Lamb Citation2008, 56). The sixth dimension, cluster participation, is inspired by the civic virtue concept of Podsakoff et al. (Citation2000) and defined as active and responsible participation in cluster affairs that affect relationships between cluster actors (Autry, Skinner, and Lamb Citation2008). The seventh dimension, relationship management, refers to cluster actors’ actions to improve the relationships that link cluster actors together. It suggests a collective approach to development rather than individual self-development as proposed by OCB research (Autry, Skinner, and Lamb Citation2008; Podsakoff et al. Citation2000).
Theoretical reflections on the consequences of identification support the assumption that cluster identification has positive effects on CCB. Ashforth and Mael (Citation1989) listed altruism, compliance, loyalty, and pride as potential outcomes of identification—all behaviors that can be called citizenship behavior. Dukerich, Golden, and Shortell (Citation2002) showed that if individuals identify strongly with an organization, cohesion among organizational members becomes stronger and cooperative behavior among individuals becomes more likely. Actors that identify strongly with the organization are “likely to consider those behaviors that benefit the organization as also benefiting themselves” (Dukerich, Golden, and Shortell Citation2002, 511).
Similarly, cluster actors who identify strongly with a regional cluster categorize themselves as belonging to the cluster, which creates an in-group/out-group distinction. In their study of group identification in industrial districts, Sammarra and Biggiero (Citation2001, 74) suggested that identification with a district affects intra-group dynamics and that district firms “become predisposed toward pro-social behaviors as an affirmation of the presumably shared identity with other members.” If the regional cluster is seen as part of the identity of the cluster actors, they will regard the cluster’s goals as their own goals and the cluster’s success as their own success, which will motivate them to behave as good cluster citizens. Hence, we formulate the following hypothesis.
Hypothesis 2:
Cluster identification is positively related to cluster actors’ CCB.
Sustained cluster effectiveness as a consequence of CCB
Studies have examined a variety of outcomes of OCB. As many of the investigated relationships have shown that OCB has positive consequences for organizational performance and effectiveness (e.g., Aggarwal and Singh Citation2016; Podsakoff et al. Citation2000), Organ’s (Citation1988, 4) assumption that OCB “in the aggregate promotes the effective functioning of the organization” seems to be widely supported. However, no study has examined the performance effects of ICB and NCB at the system level; for example, their effects on supply chains or whole networks. Research has focused on the performance implications for actors in dyadic relationships. For example, Sammarra and Biggiero (Citation2001) proposed that citizenship behaviors within industrial districts lead to increased competitiveness between these districts, with competitiveness being defined as the innovative capacity of firms in the district and the high flexibility and diversity of actors within the district.
These insights suggest that in the context of a regional cluster, CCB promotes the effective functioning of the entire cluster and is related to variables that describe the cluster’s sustained effectiveness. In many cases, regional clusters are funded by regional economic policy programs that seek to trigger and strengthen positive cluster externalities (Fromhold-Eisebith and Eisebith Citation2005). As public subsidies for these clusters decrease each year, one of the clusters’ goals is to establish sustainable and enduring structures that allow the continuance and stability of cluster activities even after public funding ends (e.g., Sölvell Citation2008). Therefore, we focus on four outcomes—willingness to pay for the cluster, continuance intentions, knowledge sharing behavior, and collaborative behavior—that are closely related to sustained cluster effectiveness and are influenced by the behavior of cluster actors.
To foster sustained cluster effectiveness, cluster actors should be willing, first and foremost, to dedicate resources to the cluster over the long term. Such resource dedication can be in monetary form, indicating actors’ willingness to pay for the cluster (e.g., membership fees, service fees). In the case of publicly funded clusters, sustained cluster effectiveness depends on the willingness of cluster actors to replace decreasing public subsidies and preserve cluster structures that stimulate inter-firm exchange (Gebhardt and Pohlmann Citation2013). Second, resource dedication can manifest itself in actors’ intentions to continue their involvement in the cluster. These continuance intentions occur especially when the regional cluster is characterized by a relational macroculture that facilitates cluster-conducive behaviors such as cluster loyalty (Autry, Skinner, and Lamb Citation2008; Bell, Tracey, and Heide Citation2009).
Third, cluster actors can influence a cluster’s innovation culture (Sammarra and Biggiero Citation2001). A vibrant innovation culture is considered key to sustained cluster effectiveness, as greater innovation capacity translates into increased competitiveness, job growth, regional image, and so on, and has positive effects at all levels—organizational, cluster, and regional (e.g., Eisingerich, Bell, and Tracey Citation2010; Lindqvist, Ketels, and Sölvell Citation2013). To stimulate innovation within the cluster, cluster actors should be willing to share knowledge, i.e., provide and receive access to new knowledge, and assimilate it (Eisingerich, Bell, and Tracey Citation2010; Provan, Fish, and Sydow Citation2007). These knowledge sharing behaviors are more likely when CCB is high. Fourth, cluster actors need to collaborate to create an agile, vibrant, and thus sustainable innovation culture (e.g., Mueller and Jungwirth Citation2022). Collective cluster benefits require joint action from cluster actors in an interactive and collective process (Bell, Tracey, and Heide Citation2009). Cluster-conducive behaviors such as helping behavior or relationship management can enhance this collaborative behavior (Autry, Skinner, and Lamb Citation2008). Therefore, we propose the following hypotheses.
Hypothesis 3:
CCB is positively related to cluster actors’ willingness to pay for the cluster.
Hypothesis 4:
CCB is positively related to cluster actors’ continuance intentions.
Hypothesis 5:
CCB is positively related to cluster actors’ knowledge sharing behavior.
Hypothesis 6:
CCB is positively related to cluster actors’ collaborative behavior.
summarizes the hypotheses and the expected direction (positive or negative) of the relationships between cluster identification and its influencing factors, CCB, and cluster effectiveness indicators.
Figure 1. Summary of the theoretical model and hypotheses.
Note: Group formation factors and CCB are both modeled as second-order constructs. Group formation factors have the following five dimensions: similarity, interaction, geographical proximity, perceived benefit, and length. CCB has the following seven dimensions: helping behavior; tolerance of mistakes; cluster loyalty; cluster compliance; cluster-supporting initiative; cluster participation; and relationship management.
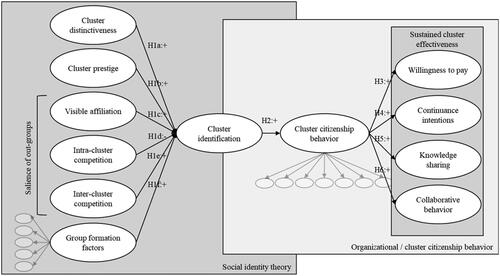
Research design
Research setting
The hypotheses were tested with data collected from a regional sensor technology cluster in the German state of Bavaria. This cluster is part of a state-wide cluster initiative that promotes key technologies in Bavaria. As part of this regional policy initiative, it receives public funding for services and activities to promote the sensor technology industry in Bavaria. The cluster’s efforts in this regard have been noticed at the national level, as evidenced by the Innovative Network accolade and membership in the national go-cluster initiative.
The cluster originated in 2003 with 14 cluster members intending to collaborate over the long term. Since 2006, a professional cluster-facilitating agency, a network administrative organization or third party (Provan and Kenis Citation2008), has been managing the cluster. At the time of data collection, the cluster-facilitating agency employed 14 cluster facilitators. The cluster members are mostly small and medium-sized enterprises, with a few large firms, local universities, and research institutions. The cluster pursues the goal of promoting cluster members’ competitiveness and market opportunities and preserving the region’s market leadership in the field of sensor technology. Therefore, the cluster organizes and coordinates activities (e.g., management workshops, best practice presentations) and services (e.g., training and development, support in partner search) that stimulate collaboration, cohesion, and a shared identity. To finance these activities, cluster members pay annual membership fees that, together with public funding, third-party funds, sponsorship money, and service or conference fees, constitutes the cluster’s annual budget.
The cluster has a long tradition of cooperation with international partners. For example, it was part of the European ICT Entrepreneur project that developed a training package to help ICT students and college graduates enhance their entrepreneurial skills and put their knowledge into practice. In addition, it has been hosting an annual international summer school since 2016 to bring together international actors from the sensor technology industry.
Data collection and sample
We followed a key informant approach, which is perfect when only a limited number of informants (e.g., boundary spanners) are expected to have complete and specialized knowledge of the research questions (Kumar, Stern, and Anderson Citation1993). The surveyed cluster actors were representatives of their organizations and assumed the role of boundary spanners between their organizations and the sensor technology cluster. Therefore, they had in-depth knowledge of both the organization and the cluster. We collected data using a standardized questionnaire.
We were granted access to the cluster member database, which contained the contact details of all 196 cluster actors. We invited the entire population to participate in our survey, which was available in both offline (paper) and online (web) forms. The questionnaire was pretested by 15 academics, cluster facilitators, and cluster actors.
After 10 weeks and two reminder e-mails, 102 cluster actors completed the questionnaire satisfactorily, for a response rate of 52%. In our dataset, 55.4% of the respondents represented small companies (up to 100 employees), 10.9% worked with medium-sized companies (between 100 and 499 employees), and 33.7% represented large companies (500+ employees). The respondents were between 29 and 80 years old (mean = 47). More than four fifths (80.4%) were men and more than 90% had a university degree.
We compared the means and distributions of the key metrics for early versus late respondents to test whether nonresponse bias affected our study (Armstrong and Overton Citation1977). Additionally, as we offered two modes for completing the survey (postal and web), there could be channel bias. Therefore, we compared the means of the variables in the paper-based and web-based responses. As the differences between the two sets of respondents and the two response modes were not significantly different from zero (p > 0.10) on any of the variables, we are confident that there was neither nonresponse bias nor channel bias in our data.
Measures
Appendix A provides an overview of all of the variables and associated items. Unless otherwise indicated, all of the items used a Likert-type scale ranging from 1 (strongly agree) to 7 (strongly disagree).
We predominantly used validated measures from an (inter-)organizational research context and adapted them linguistically to the cluster context. As the questionnaire was distributed in Germany, we translated the items from English into German, following the translation and back-translation procedures outlined by Brislin (Citation1986).
We measured the antecedents of cluster identification using six latent variables. Perceived cluster distinctiveness and perceived cluster prestige were captured by a four- and three-item scale, respectively, based on Staber (Citation2010; adapted from Jones and Volpe (Citation2011) and Mael and Ashforth (Citation1992)). The concept of out-group salience was operationalized using three scales (e.g., Mael and Ashforth Citation1992). First, as visible affiliation with the cluster heightens self-awareness of the connection with the cluster, we developed three dichotomous items based on Dukerich, Golden, and Shortell (Citation2002) to capture a cluster actor’s visible affiliation with the cluster. Out-group salience was further described by a three-item scale measuring perceived intra-cluster competition (Bengtsson and Sölvell Citation2004; Mael and Ashforth Citation1992). Last, the information on whether cluster actors engaged with other clusters was used to collect bivariate information on perceived inter-cluster competition, another indicator of out-group salience.
As the basis for self-categorization mechanisms, group formation factors, the last antecedent of cluster identification, were modeled as a five-dimensional second-order construct. We measured the first dimension similarity using four items adapted from the perceived fit scale and shared value scale of Cable and DeRue (Citation2002) and Cheng, Yeh, and Tu (Citation2008), respectively. The second dimension interaction was captured by four items that reflect the frequency of interaction between cluster actors (Eisingerich, Bell, and Tracey Citation2010; Staber Citation2010) and one item that indicates the average frequency of contact (Ibarra Citation1995; Rouzies Citation2011). Geographical proximity, the third dimension, was measured by the organization’s distance (time taken for a drive in minutes) from the cluster-facilitating agency, where most of the cluster activities take place. The fourth dimension, the perceived benefit of cluster affiliation, was captured by three items based on Rouzies (Citation2011) and Felzensztein, Brodt, and Gimmon (Citation2014). The last dimension, length of cluster affiliation, was captured by two items.
We captured cluster identification using a six-item scale based on Mael and Ashforth (Citation1992). Following Van Dick et al. (Citation2006), Staber (Citation2010), and Sluss et al. (Citation2012), who used the items in different contexts, such as organizations, clusters, and associations, we adapted the items linguistically.
We operationalized CCB as a multi-dimensional second-order construct (e.g., Organ, Podsakoff, and MacKenzie Citation2006; Podsakoff et al. Citation2014). The items of the CCB dimensions were based on scales from the ICB or NCB literature and were adapted linguistically to the cluster context. Specifically, we used multi-item scales following Skinner, Autry, and Lamb (Citation2009) and Braun (Citation2013) to measure helping behavior, tolerance, cluster loyalty, cluster compliance, cluster-supporting initiative, and relationship management dimensions. Cluster participation was measured by a three-item scale inspired by the civic virtue construct (Podsakoff et al. Citation1990).
Sustained cluster effectiveness was measured by four constructs. The cluster actors’ willingness to pay for the cluster was captured by two items that reveal the organizations’ willingness to give monetary support to the cluster and its activities. We assessed continuance intentions using two items from the continuance commitment scale of Allen and Meyer (Citation1990) that measure a cluster actor’s attachment to and involvement in the cluster. Knowledge sharing behavior among cluster actors was captured using six items based on De Vries, van den Hooff, and de Ridder (Citation2006) and Cheng, Yeh, and Tu (Citation2008). Following Felzensztein, Brodt, and Gimmon (Citation2014), we asked the cluster actors about their organizations’ collaborative behavior. In addition, a dichotomous item captured whether the cluster actor had cooperated with another cluster actor in the previous 3 years.
For control variables, we integrated firm size, firm age, and its current state of business. We also asked the cluster actors to rate their identification with their organization to control for bias caused by overly strong group identification.
Methods and results
Methods
We used SmartPLS software for partial least squares structural equation modeling (PLS-SEM) (Ringle, Wende, and Becker Citation2015) to test the hypothesized relationships. We chose this method because it aligned with our research goal of advancing social identity theory and OCB in the cluster context. Moreover, PLS-SEM accommodates high model complexities with many latent constructs and small sample sizes and yields precise estimates of factor scores even if some data requirements, which must be fulfilled in other estimation methods, are not met (Hair et al. Citation2017).
Measurement model evaluation
The exploratory factor analysis of all items replicated the intended factor structure clearly. Each item loaded on its intended factor and all factors had eigenvalues greater than 1. The resulting measurement model consisted of 12 reflective latent variables. Two of them, group formation factors and CCB, were second-order constructs, specified using a repeated indicators approach (Hair et al. Citation2017) and operationalized by an additional five and seven first-order factors, respectively.
The loading of each item on its intended construct was significant (p < 0.05) and most factor loadings were above the suggested threshold of 0.7. We retained all items with factor loadings below 0.7, as they demonstrated content validity. The values for composite reliability ranged from 0.84 to 0.95, showing that all of the multi-item constructs had satisfactory construct validity (Bagozzi and Yi Citation1988; Hair et al. Citation2017). The average variance extracted values for each construct was above 0.5, which demonstrated convergent validity (Appendix A shows all exact values). The analyses of the cross-loadings and Fornell and Larcker’s criterion (Appendix B) demonstrated discriminant validity. The second-order constructs were assessed indirectly by analyzing the assigned first-order constructs and related items (Hair et al. Citation2017).
Structural model evaluation
shows the results of the PLS-SEM estimation, including path coefficients and t-values. The R2 values ranged from 0.14 to 0.54 for the endogenous constructs cluster identification, CCB, willingness to pay, continuance intentions, knowledge sharing, and collaborative behavior. The positive values of Stone-Geisser’s Q2 ranging from 0.09 to 0.37 demonstrated the predictive relevance of the model. The variance inflation factors were well below 5 for the measurement and structural models, suggesting that there was no multicollinearity in our study. We applied a nonparametric bootstrap procedure with a bootstrap sample of N = 5,000 to estimate standard errors and test the statistical significance of the structural paths.
Regarding the antecedents of cluster identification, the constructs of perceived cluster distinctiveness, visible affiliation with the cluster, and group formation factors were positively and significantly related to cluster identification but cluster prestige and intra- and inter-cluster competition were not. These results supported Hypotheses 1a, 1c, and 1f, but not Hypotheses 1b, 1d, and 1e. Furthermore, the results showed a positive and significant effect of cluster identification on CCB, which supported Hypothesis 2. Last, CCB had a positive and significant effect on cluster actors’ willingness to pay, continuance intentions, knowledge sharing behavior, and collaborative behavior. Therefore, Hypotheses 3–6 were also supported. summarizes our predictions and results.
Table 1. Summary of the predictions and results.
Robustness checks
We performed several robustness checks to ensure the validity and reliability of our results. First, as the data were collected from a one-time survey of cluster actors, there could be common method bias. We tried to eliminate potential sources of common method bias ex ante by formulating our questionnaire items carefully, randomizing them in the survey, and guaranteeing full anonymity to the respondents (Podsakoff et al. Citation2003). We also used an extensive pretest to ensure that the cluster actors were able to answer the questions (Kumar, Stern, and Anderson Citation1993). In addition, we ran Harman’s single-factor test (Harman Citation1976) and found that the variables entered into the analysis did not form a single factor with an eigenvalue greater than 1, and the variance did not come solely from the first factor.
Furthermore, we repeated the measurement of one of our key constructs, cluster identification, at a later point in time (t2) to demonstrate the temporal stability of the self-reports collected at t1 and show the reliability of our instrument (Bagozzi and Yi Citation1988; Podsakoff et al. Citation2003). For this purpose, we administered a short survey to the t1-respondents 11 months after the first survey and asked them to reassess the six cluster identification items. As some of the original respondents had left the organizations or changed positions within the organizations, the second survey pool included only 87 of the 102 t1-respondents. More than half of them (n = 46) completed the survey. Each of the cluster identification items in the t1 sample correlated positively and significantly with the corresponding item in the t2 sample, with test-retest reliability coefficients ranging from 0.52 to 0.65 (p < 0.01). Given the relatively long time between t1 and t2, these coefficients were acceptable and showed that our instrument was reliable. Overall, these results suggested that our findings were not driven predominantly by common method bias, although its effects could not be fully ruled out.
Furthermore, we used cluster documents to externally validate the cluster actors’ willingness to pay. In 95% of the cases, the respondents accurately reported their organization’s willingness to pay, which confirmed the validity of our data.
In addition, following the recommendations of the OCB literature (e.g., Podsakoff et al. Citation2000; Van Dick et al. Citation2006), we validated the self-reported CCB data with the CCB data obtained from external sources. In an organizational context, these external sources are peers or supervisors. For this study, we asked cluster facilitators to rate three of the CCB dimensions (cluster-supporting initiative, cluster compliance, and cluster participation). The results showed that the external ratings and self-reports correlated positively and significantly (cluster-supporting initiative: r = 0.66, p < 0.01, cluster compliance: r = 0.52, p < 0.01, cluster participation: r = 0.74, p < 0.01), making us confident that our CCB construct was valid.
Discussion
This study investigated what holds a regional cluster together. As regional clusters do not have governance mechanisms to stimulate behaviors that benefit the entire cluster, a study of the socio-psychological mechanisms that bind cluster actors together and induce citizenship behaviors could produce useful insights. We posited that cluster actors’ identification with regional clusters is influenced by perceived cluster distinctiveness, perceived cluster prestige, out-group salience (visible cluster affiliation, inter-, and intra-cluster competition), and group formation factors. Furthermore, the theory of citizenship behavior suggests that cluster identification positively influences a cluster actor’s citizenship behavior. In addition, following studies on the outcomes of citizenship behavior (e.g., Aggarwal and Singh Citation2016; Podsakoff et al. Citation2000), we assumed a positive relationship between CCB and the different factors of sustained cluster effectiveness—cluster actors’ willingness to pay for cluster activities and services, continuance intentions, knowledge sharing, and collaborative behavior. These hypotheses were tested using survey data from 102 cluster actors in a sensor technology cluster in Germany.
Our findings confirmed some of our hypotheses. First, regarding the antecedents of cluster identification, the results emphasized the importance of perceived cluster distinctiveness. Cluster actors perceive the cluster, its services and activities, its norms and values, its communication and collaboration structures, and other ascribed characteristics as distinct from that of other regional clusters. We also found that a visible affiliation with the cluster had a positive influence on cluster identification, which is in line with the findings of previous studies highlighting the role of visible cluster branding activities in the formation of a cluster identity (Mauroner and Zorn Citation2017). Visible affiliation reflects the cluster actor’s sense of belonging to the cluster and contributes to the definition of in- and out-groups (e.g., Anand, Joshi, and O'Leary-Kelly Citation2013). The more visible the affiliation, the more the cluster actors are aware of their in-group’s boundaries and the more they identify with the cluster. Similar to other (inter)organizational contexts, group formation factors were found to have a positive and significant impact on cluster identification. Compared with other antecedents proposed by social identity theory and tested in our study, group formation processes had the largest effect (f2 = 0.19) on cluster identification. This finding supports the relevance of associated group formation mechanisms that facilitate and strengthen self-categorization (Hogg and Turner Citation1985; Turner Citation1984).
Surprisingly, however, some of the constructs previously identified as antecedents of identification with a group did not have a significant influence on identification in the cluster context. Contrary to our expectations, perceived prestige was not found to be a significant antecedent of cluster identification. One explanation for this result might be that cluster actors rarely consider the relative prestige, reputation, or status of other clusters due to the industry focus of regional clusters, as most of them operate in different industries. Unlike in organizational contexts (Mael and Ashforth Citation1992), cluster actors do not consider the prestige of the cluster as an integral part of their self-concept and therefore it does not enhance their self-confidence as a member of a specific cluster (in-group). In addition, the founders of public cluster initiatives do not encourage “aggressive” marketing that specifically highlights cluster prestige because they fear the negative spillover effects of competition between clusters that are part of the same initiative. Therefore, the relative prestige of clusters is rarely discussed or perceived by cluster actors.
These reflections also emphasize that competition between regional clusters is rather weak and is not considered relevant in defining the boundaries of the in-group. This explains why the relationship between inter-cluster competition and cluster identification was not significant in our study. Although competition between comparable groups and the increased salience of out-groups make individuals more aware of their in-group in an organizational context (Ashforth and Mael Citation1989; Peteraf and Shanley Citation1997), this mechanism does not seem to hold in the cluster context.
Furthermore, we did not find that intra-cluster competition, another variable related to out-group salience, had a negative effect on cluster identification. One possible reason for this is that the definition of regional clusters (Porter Citation1998, Citation2000) explicitly allows, or even requires, direct competitors to be part of the same cluster. Such intra-cluster competition fosters innovation and learning dynamics within the cluster, resulting in a vibrant innovation culture. Hence, while cluster actors might be in fierce competition with each other, they still see themselves as belonging to the regional cluster (in-group) sharing a collective identity (Aversa, Furnari, and Jenkins Citation2022; Howard-Grenville, Metzger, and Meyer Citation2013). As cluster actors are assumed to be well aware of the competition within the cluster ex ante, intra-cluster competition does not reduce the cohesion within the cluster and does not weaken the actors’ identification with the cluster.
In line with our expectations, we found a strong positive effect (f2 = 0.50) of cluster identification on CCB. If cluster actors identify strongly with a cluster, they are more willing to exhibit voluntary and discretionary behavior, such as helping behavior and tolerance. Such citizenship behavior benefits the cluster as a whole, which is why we coined the term CCB. This result highlights the relevance of studying identification and citizenship behavior in the context of regional clusters and thus directs the attention of future cluster research to these socio-psychological processes.
Last, we also found positive and significant relationships between cluster actors’ citizenship behavior and their willingness to contribute to sustained cluster effectiveness in terms of willingness to pay for cluster activities and services, their continuance intentions, knowledge sharing, and collaborative behavior. The effect sizes (f2) of these relationships ranged from 0.16 to 1.20, supporting the importance of these behaviors for sustained cluster effectiveness. Thus, CCB promotes the effective functioning of regional clusters, as OCB research has suggested (Organ Citation1988). These findings could provide the socio-psychological foundations for an important strand of the cluster literature that focuses on the dynamics of knowledge transfer and the processes of knowledge creation and learning as key advantages of regional clustering (e.g., Eisingerich, Bell, and Tracey Citation2010; Tallman et al. Citation2004). Our results add to this literature by proposing that if cluster actors identify more strongly with the cluster, they are more willing to show behaviors that benefit the cluster as a whole and engage in knowledge co-creation, for example, which facilitates the transfer of tacit knowledge and intangible resources and produces greater innovation.
Implications
Our study has the following implications. First, our findings provide a first answer to the call for research on the microfoundations of the formation of a collective cluster identity (Aversa, Furnari, and Jenkins Citation2022). While we already have knowledge on the consequences of a shared cluster macroculture (Bell, Tracey, and Heide Citation2009) and the emotional microfoundations (Aversa, Furnari, and Jenkins Citation2022) for cluster organization and cluster genesis, respectively, our study highlights the socio-psychological processes that promote long-term cohesion at the cluster level. In particular, we use social identity theory and the literature on organizational citizenship behavior to introduce and define the concepts of cluster identification and CCB. An important finding of our study is that not all antecedents of identification that are important in organizational contexts (Ashforth and Mael Citation1989; Mael and Ashforth Citation1992) are key antecedents of cluster actors’ identification with a cluster. This is mainly due to the peculiarities of the interorganizational cluster context, which by definition promotes competition within the regional cluster (in-group) but neglects competition with other regional clusters (out-group). We hope that these findings will stimulate research to further explore these new concepts.
Second, the literature on cluster (or network) governance (e.g., Cassanego et al. Citation2019; Provan and Kenis Citation2008) could build on our study and investigate cluster actors’ citizenship behavior as an aggregate phenomenon at the cluster level (e.g., using a CCB index) rather than as behaviors of individual actors. Strong CCB at the cluster level could create social pressure on cluster actors to behave as good cluster citizens. Consequently, cluster actors may be guided by the norm of reciprocity and repay other actors’ citizenship behavior, or comply with established CCB routines. From this perspective, CCB (and cluster identification) could serve as informal governance mechanisms for managing a cluster. Therefore, our study moves the ongoing discussion of intentional governance of interorganizational networks (Dagnino, Levanti, and Mocciaro Li Destri Citation2016) forward by showing that in the absence of formal governance mechanisms, socio-psychological processes, such as cluster identification and CCB, can serve as informal governance mechanisms and guide cluster actors’ behavior. So far, our knowledge of informal governance mechanisms is limited to a few studies on reciprocity as an exchange mechanism in networks and a few studies of guanxi, which have highlighted the role of emotional attachment and collective benefits in networks (e.g., Cassanego et al. Citation2019; Yang and Wang Citation2011).
Moreover, our findings on the antecedents of cluster actors’ identification with regional clusters have highlighted the issue of cluster boundaries. Our results for out-group salience suggest that the boundaries between in- and out-groups for clusters are drawn using mechanisms different from those used in other (inter)organizational settings. This finding is in line with the general discussion of the challenges of defining cluster boundaries (e.g., Martin and Sunley Citation2003). Although cluster boundaries seemed to be clearly defined in our sample, the literature on clusters needs to look at how to clearly delineate cluster boundaries (Provan, Fish, and Sydow Citation2007), which becomes even more difficult when cluster actors are not listed in a formal cluster roster. This study emphasized self-categorization (Hogg and Turner Citation1985; Turner Citation1984) as a relevant mechanism for cluster actors’ identification with the cluster as an in-group. This mechanism is supported by signs or symbols that convey the brand and identity of the cluster, as Mauroner and Zorn (Citation2017) suggested. The question of cluster boundaries requires further research on socio-psychological phenomena, such as identification and citizenship behavior.
Our results also have implications for industry practitioners. Cluster actors should be made aware of the positive influence of their citizenship behavior on sustained cluster effectiveness. Cluster actors’ organizations directly benefit from a stable and sustainable cluster through positive cluster externalities, such as knowledge spillovers and, by implication, from the positive effects of their citizenship behavior. For this purpose, as suggested by Skinner, Autry, and Lamb (Citation2009), the concept of CCB could be integrated into training programs for boundary spanning personnel who can leverage these behaviors for their own benefit. Along these lines, further research could shed light on the relevant antecedents of CCB beyond cluster identification. For example, testing the influence of organizational antecedents (e.g., organizational culture) or network-level antecedents (e.g., network legitimacy), which were identified by Provan, Sydow, and Podsakoff (Citation2017), on CCB could help practitioners stimulate behaviors that benefit the cluster as a whole.
Moreover, understanding the mechanisms behind cluster identification, CCB, and cluster effectiveness would help cluster facilitators and policymakers design effective and sustainable public cluster initiatives. For example, cluster facilitators might use our results to develop measures that increase cluster actors’ identification with the cluster. They should regularly highlight the cluster’s unique characteristics (e.g., in cluster newsletters) to make cluster actors aware of the cluster’s distinctiveness, even if they are not familiar with other clusters as a reference group. In addition, they should create signs and symbols that make the cluster actors’ affiliation with the cluster more visible. Specifically, they should develop a cluster brand (Mauroner and Zorn Citation2017) and choose an adequate and visible legal identity (e.g., registered association). Furthermore, cluster facilitators and policymakers could aim to attract as many competitors as possible to a regional cluster, as intra-cluster competition does not seem to weaken cluster actors’ identification with the cluster but has a positive impact on innovation within the cluster. Further research could add to our results by analyzing the role of cluster governance institutions, such as cluster-facilitating agencies (Hurmelinna-Laukkanen, Möller, and Nätti Citation2022), in the process of identity construction and identification. For example, future studies could explore whether certain types of cluster governance institutions (Provan and Kenis Citation2008; Mueller Citation2021) can be catalysts of identification processes.
Limitations and future research directions
This study has the following limitations that offer fruitful avenues for future research. First, we used a static perspective to study cluster identification, which did not include considerations and insights from cluster lifecycle models (Menzel and Fornahl Citation2010). If a dynamic perspective is used and the maturity of clusters is included, then different antecedents of cluster identification might become relevant at different stages of cluster development (e.g., cluster formation vs. cluster transformation) (Eisenbeiss Citation2004). A dynamic approach could also allow for CCB, a consequence of cluster identification in the early stage of cluster development, to become an antecedent of cluster identification in the next development cycle, unfolding a feedback loop as suggested by Ashforth and Mael (Citation1989) and Provan, Sydow, and Podsakoff (Citation2017). Future studies could investigate this dynamic perspective.
Second, the use of self-reported data may have introduced common method bias (Podsakoff et al. Citation2003). We undertook several additional steps to reduce that risk (e.g., repeated measurement of the cluster identification construct and test-retest reliability analysis, external validation of CCB and willingness to pay). These efforts give us confidence that the results of this study were not driven primarily by common method bias. Nevertheless, it would be useful to retest our hypotheses with a panel dataset, for example.
Finally, the results were based on a survey of cluster actors in a single cluster (a sensor technology cluster). This third-party governed cluster is a mature cluster that has been financed by a top-down cluster initiative for more than 15 years, along with membership fees, service fees, and third-party funding. The cluster has already undergone some transformation (e.g., technological focus, internationalization strategy, cross-clustering) and built vertical, horizontal, lateral, institutional, and external relationships. One could argue that our findings are not generalizable due to the specificities of this sensor technology cluster. However, we believe that our results are valuable for other clusters in other regions, as the sensor technology cluster can be considered a typical example of a top-down cluster in terms of governance, financing, structure, and initiatives. This is at least true for clusters within the European Union, as they often face similar challenges, which are rooted in the funding guidelines of the EU regional policy programs (e.g., smart specialization strategies) or in national or regional cluster subsidy programs (Balland et al. Citation2019; Borrás and Tsagdis Citation2008). It would be interesting to investigate whether the results would be different when studying structurally different clusters, such as bottom-up clusters, and if so, to what extent.
Conclusion
Scholars seem to be well informed about regional clusters. They are aware of positive cluster externalities, how organizations benefit from innovativeness and competitiveness, and the relevance of clusters in regional economic policies. However, there is limited knowledge of the actual socio-psychological mechanisms that hold regional clusters together. This study applied the main propositions of social identity theory and research on OCB in the context of a regional cluster, thereby advancing knowledge of identification processes and citizenship behavior in clusters and their antecedents and outcomes. The results showed that not all antecedents of identification that are important in organizational contexts are key antecedents of cluster actors’ identification with a cluster. Cluster distinctiveness, visible cluster affiliation, and group formation factors were relevant but cluster prestige and inter- and intra-cluster competition were not. The results also emphasized the importance of cluster actors’ identification with the cluster in terms of their willingness to exhibit CCB and the importance of these behaviors as predictors of sustained cluster effectiveness.
Acknowledgements
We gratefully acknowledge financial support by the Universität der Bundeswehr München.
Additional information
Notes on contributors
Elisabeth F. Mueller
Elisabeth F. Mueller is a full professor of strategic management at the Universität der Bundeswehr München, Germany. Her research interests lie in the areas of strategic management and entrepreneurship, with a focus on the mechanisms of networked and sustainable value creation of the future.
Stefanie Fuchs
Stefanie Fuchs received her PhD in management from the School of Business, Economics and Information Systems, University of Passau, Germany. Her primary research interests include the socio-psychological foundations of inter-firm networks.
References
- Aggarwal, A., and R. Singh. 2016. “Exploring the Nomological Network of Organizational Citizenship Behavior: A Review of Dimensions, Antecedents and Consequences.” Journal of Organizational Behavior 15 (3): 16–39.
- Allen, N. J., and J. P. Meyer. 1990. “The Measurement and Antecedents of Affective, Continuance and Normative Commitment to the Organization.” Journal of Occupational Psychology 63 (1): 1–18. doi:10.1111/j.2044-8325.1990.tb00506.x.
- Anand, V., M. Joshi, and A. M. O'Leary-Kelly. 2013. “An Organizational Identity Approach to Strategic Groups.” Organization Science 24 (2): 571–90. doi:10.1287/orsc.1120.0752.
- Armstrong, J. S., and T. S. Overton. 1977. “Estimating Nonresponse Bias in Mail Surveys.” Journal of Marketing Research 14 (3): 396–402. doi:10.2307/3150783.
- Ashforth, B. E., and F. Mael. 1989. “Social Identity Theory and the Organization.” Academy of Management Review 14 (1): 20–39. doi:10.2307/258189.
- Autry, C. W., L. R. Skinner, and C. W. Lamb. 2008. “Interorganizational Citizenship Behaviors: An Empirical Study.” Journal of Business Logistics 29 (2): 53–74. doi:10.1002/j.2158-1592.2008.tb00087.x.
- Aversa, P., S. Furnari, and M. Jenkins. 2022. “The Primordial Soup: Exploring the Emotional Microfoundations of Cluster Genesis.” Organization Science 33 (4): 1340–71. doi:10.1287/orsc.2021.1484.
- Bagozzi, R. P., and Y. Yi. 1988. “On the Evaluation of Structural Equation Models.” Journal of the Academy of Marketing Science 16 (1): 74–94. doi:10.1007/BF02723327.
- Balland, P.-A., R. Boschma, J. Crespo, and D. L. Rigby. 2019. “Smart Specialization Policy in the European Union: Relatedness, Knowledge Complexity and Regional Diversification.” Regional Studies 53 (9): 1252–68. doi:10.1080/00343404.2018.1437900.
- Beech, N., and C. Huxham. 2003. “Cycles of Identity Formation in Interorganizational Collaborations.” International Studies of Management & Organization 33 (3): 28–52. doi:10.1080/00208825.2003.11043686.
- Bell, S. J., P. Tracey, and J. B. Heide. 2009. “The Organization of Regional Clusters.” Academy of Management Review 34 (4): 623–42. doi:10.5465/AMR.2009.44882930.
- Bengtsson, M., and Ö. Sölvell. 2004. “Climate of Competition, Clusters and Innovative Performance.” Scandinavian Journal of Management 20 (3): 225–44. doi:10.1016/j.scaman.2004.06.003.
- Borrás, S., and D. Tsagdis. 2008. Cluster Policies in Europe: Firms, Institutions, and Governance. Cheltenham, England: Edward Elgar.
- Braun, T. 2013. Kooperatives Verhalten in interorganisationalen Projekten. Eine konzeptionelle und empirische Weiterentwicklung des OCB-Ansatzes. Cologne: Kölner Wissenschaftsverlag.
- Brislin, R. W. 1986. “The Wording and Translation of Research Instruments.” In Field Methods in Cross-Cultural Research, edited by W. J. Lonner, 137–64. 1st ed. Beverly Hills: Sage Publ.
- Cable, D. M., and D. S. DeRue. 2002. “The Convergent and Discriminant Validity of Subjective Fit Perceptions.” Journal of Applied Psychology 87 (5): 875–84. doi:10.1037/0021-9010.87.5.875.
- Cassanego, P. V., Jr., J. M. Gama Boaventura, A. C. Azevedo, and R. Telles. 2019. “Governance in Business Clusters: Proposal for an Application of an Analytical Model.” Entrepreneurship & Regional Development 31 (9-10): 984–1010. doi:10.1080/08985626.2019.1652351.
- Cheng, J.-H., C.-H. Yeh, and C.-W. Tu. 2008. “Trust and Knowledge Sharing in Green Supply Chains.” Supply Chain Management: An International Journal 13 (4): 283–95. doi:10.1108/13598540810882170.
- Dagnino, G. B., G. Levanti, and A. Mocciaro Li Destri. 2016. “Structural Dynamics and Intentional Governance in Strategic Interorganizational Network Evolution: A Multilevel Approach.” Organization Studies 37 (3): 349–73. doi:10.1177/0170840615625706.
- De Vries, R. E., B. van den Hooff, and J. A. de Ridder. 2006. “Explaining Knowledge Sharing. The Role of Team Communication Styles, Job Satisfaction, and Performance Beliefs.” Communication Research 33 (2): 115–35. doi:10.1177/0093650205285366.
- Dukerich, J., B. R. Golden, and S. M. Shortell. 2002. “Beauty is in the Eye of the Beholder: The Impact of Organizational Identification, Identity, and Image on the Cooperative Behaviors of Physicians.” Administrative Science Quarterly 47 (3): 507–33. doi:10.2307/3094849.
- Dutton, J. E., J. Dukerich, and C. V. Harquail. 1994. “Organizational Images and Member Identification.” Administrative Science Quarterly 39 (2): 239–63. doi:10.2307/2393235.
- Eisenbeiss, K. K. 2004. “A Dynamic Perspective on Social Identification: Predictors and Consequences of Identification during Group Formation.” Dissertation, Friedrich Schiller University Jena.
- Eisingerich, A. B., S. J. Bell, and P. Tracey. 2010. “How Can Clusters Sustain Performance? The Role of Network Strength, Network Openness, and Environmental Uncertainty.” Research Policy 39 (2): 239–53. doi:10.1016/j.respol.2009.12.007.
- Ellis, N., and S. Ybema. 2010. “Marketing Identities: Shifting Circles of Identification in Inter-Organizational Relationships.” Organization Studies 31 (3): 279–305. doi:10.1177/0170840609357397.
- Esper, T. L., R. V. Bradley, R. Thomas, and L. M. Thornton. 2015. “Supply Chain Citizenship: Investigating the Antecedents of Customer Interorganizational Citizenship Behaviors.” Journal of Business Logistics 36 (4): 306–20. doi:10.1111/jbl.12108.
- Felzensztein, C., S. E. Brodt, and E. Gimmon. 2014. “Do Strategic Marketing and Social Capital Really Matter in Regional Clusters? Lessons from an Emerging Economy of Latin America.” Journal of Business Research 67 (4): 498–507. doi:10.1016/j.jbusres.2013.11.006.
- Fromhold-Eisebith, M., and G. Eisebith. 2005. “How to Institutionalize Innovate Clusters? Comparing Explicit Top-down and Implicit Bottom-up Approaches.” Research Policy 34 (8): 1250–68. doi:10.1016/j.respol.2005.02.008.
- Gebhardt, C., and M. C. Pohlmann. 2013. “Managing the Organisation 2.0: Entrepreneurial Spirit and General Management Competences as Early Indicators for Cluster Success and Sustainable Regional Development. Findings from the German Entrepreneurial Regions Program.” Journal of High Technology Management Research 24 (2): 153–60. doi:10.1016/j.hitech.2013.09.007.
- Gerke, A., D. Luzzini, and C. Mena. 2021. “Innovation Configurations in Sport Clusters: The Role of Interorganizational Citizenship and Social Capital.” Journal of Business Research 133:409–19. doi:10.1016/j.jbusres.2021.04.064.
- Hair, J. F., G. T. M. Hult, C. Ringle, and M. Sarstedt. 2017. A Primer on Partial Least Squares Structural Equation Modeling (PLS-SEM). 2nd ed. Thousand Oaks, CA: Sage Publications.
- Hardy, C., T. B. Lawrence, and D. Grant. 2005. “Discourse and Collaboration: The Role of Conversations and Collective Identity.” Academy of Management Review 30 (1): 58–77. doi:10.5465/amr.2005.15281426.
- Harman, H. H. 1976. Modern Factor Analysis. 3rd ed. Chicago, IL: University of Chicago Press.
- Hess, T., C. Matt, A. Benlian, and F. Wiesböck. 2016. “Options for Formulating a Digital Transformation Strategy.” MIS Quarterly Executive 15 (2): 123–39.
- Hogg, M. A., and J. C. Turner. 1985. “Interpersonal Attraction, Social Identification and Psychological Group Formation.” European Journal of Social Psychology 15 (1): 51–66. doi:10.1002/ejsp.2420150105.
- Howard-Grenville, J., M. L. Metzger, and A. D. Meyer. 2013. “Rekindling the Flame: Processes of Identity Resurrection.” Academy of Management Journal 56 (1): 113–36. doi:10.5465/amj.2010.0778.
- Hurmelinna-Laukkanen, P., K. Möller, and S. Nätti. 2022. “Orchestrating Innovation Networks: Alignment and Orchestration Profile Approach.” Journal of Business Research 140:170–88. doi:10.1016/j.jbusres.2021.11.084.
- Ibarra, H. 1995. “Race, Opportunity, and Diversity of Social Circles in Managerial Networks.” Academy of Management Journal 38 (3): 673–703. doi:10.5465/256742.
- Jones, C., and E. H. Volpe. 2011. “Organizational Identification: Extending Our Understanding of Social Identities through Social Networks.” Journal of Organizational Behavior 32 (3): 413–34. doi:10.1002/job.694.
- Kenis, P., and J. Raab. 2020. “Back to the Future: Using Organization Design Theory for Effective Organizational Networks.” Perspectives on Public Management and Governance 3 (2): 109–23. doi:10.1093/ppmgov/gvaa005.
- Kumar, N., L. Stern, and J. Anderson. 1993. “Conducting Interorganizational Research Using Key Informants.” Academy of Management Journal 36 (6): 1633–51. doi:10.5465/256824.
- Lindqvist, G., C. Ketels, and Ö. Sölvell. 2013. The Cluster Initiative Greenbook 2.0. Stockholm: Ivory Tower Publishing.
- Lorenzen, M. 2001. “Ties, Trust, and Trade: Elements of a Theory of Coordination in Industrial Clusters.” International Studies of Management & Organization 31 (4): 14–34. doi:10.1080/00208825.2001.11656825.
- Mael, F., and B. E. Ashforth. 1992. “Alumni and Their Alma Mater: A Partial Test of the Reformulated Model of Organizational Identification.” Journal of Organizational Behavior 13 (2): 103–23. doi:10.1002/job.4030130202.
- Marshall, A. 1920. Principles of Economics. 8th ed. London: Macmillan.
- Martin, R., and P. Sunley. 2003. “Deconstructing Clusters: Chaotic Concept or Policy Panacea?” Journal of Economic Geography 3 (1): 5–35. doi:10.1093/jeg/3.1.5.
- Mauroner, O., and J. Zorn. 2017. “Cluster Branding – A Case Study on Regional Cluster Initiatives, Cluster Management, and Cluster Brands.” International Journal of Innovation and Regional Development 7 (4): 290–312. doi:10.1504/IJIRD.2017.086234.
- Menzel, M.-P., and D. Fornahl. 2010. “Cluster Life Cycles – Dimensions and Rationales of Cluster Evolution.” Industrial and Corporate Change 19 (1): 205–38. doi:10.1093/icc/dtp036.
- Mueller, E. F. 2021. “Towards a Theory of Network Facilitation: A Microfoundations Perspective on the Antecedents, Practices and Outcomes of Network Facilitation.” British Journal of Management 32 (1): 80–96. doi:10.1111/1467-8551.12470.
- Mueller, E. F., and C. Jungwirth. 2016. “What Drives the Effectiveness of Industrial Clusters? Exploring the Impact of Contextual, Structural and Functioning Determinants.” Entrepreneurship & Regional Development 28 (5-6): 424–47. doi:10.1080/08985626.2016.1186748.
- Mueller, E. F., and C. Jungwirth. 2022. “Are Cooperative Firms More Agile? A Contingency Perspective on Small and Medium-Sized Enterprises in Agglomerations and Peripheral Areas.” Small Business Economics 58 (1): 281–302. doi:10.1007/s11187-020-00410-3.
- Organ, D. W. 1977. “A Reappraisal and Reinterpretation of the Satisfaction-Causes-Performance Hypothesis.” Academy of Management Review 2 (1): 46–53. doi:10.2307/257604.
- Organ, D. W. 1988. Organizational Citizenship Behavior: The Good Soldier. Lexington, MA: Lexington Books.
- Organ, D. W., P. M. Podsakoff, and S. B. MacKenzie. 2006. Organizational Citizenship Behavior: Its Nature, Antecedents and Consequences. Thousand Oaks, CA: Sage Publications.
- Peteraf, M., and M. Shanley. 1997. “Getting to Know You: A Theory of Strategic Group Identity.” Strategic Management Journal 18 (S1): 165–86. doi:10.1002/(SICI)1097-0266(199707)18:1+<165::AID-SMJ914>3.0.CO;2-#.
- Podsakoff, P. M., S. B. MacKenzie, J. Y. Lee, and N. P. Podsakoff. 2003. “Common Method Biases in Behavioral Research: A Critical Review of the Literature and Recommended Remedies.” Journal of Applied Psychology 88 (5): 879–903. doi:10.1037/0021-9010.88.5.879.
- Podsakoff, P. M., S. B. MacKenzie, R. H. Moorman, and R. Fetter. 1990. “Transformational Leader Behaviors and Their Effects on Followers’ Trust in Leader, Satisfaction, and Organizational Citizenship Behaviors.” Leadership Quarterly 1 (2): 107–42. doi:10.1016/1048-9843(90)90009-7.
- Podsakoff, P. M., S. B. MacKenzie, J. B. Paine, and D. G. Bachrach. 2000. “Organizational Citizenship Behaviors: A Critical Review of the Theoretical and Empirical Literature and Suggestions for Future Research.” Journal of Management 26 (3): 513–63. doi:10.1177/014920630002600307.
- Podsakoff, N. P., P. M. Podsakoff, S. B. MacKenzie, T. D. Maynes, and T. M. Spoelma. 2014. “Consequences of Unit-Level Organizational Citizenship Behaviors: A Review and Recommendations for Future Research.” Journal of Organizational Behavior 35 (S1): S87–S119. doi:10.1002/job.1911.
- Porter, M. E. 1998. “Clusters and the New Economics of Competition.” Harvard Business Review 76 (6): 77–90.
- Porter, M. E. 2000. “Location, Competition, and Economic Development: Local Clusters in a Global Economy.” Economic Development Quarterly 14 (1): 15–34. doi:10.1177/089124240001400105.
- Provan, K. G., A. Fish, and J. Sydow. 2007. “Interorganizational Networks at the Network Level: A Review of the Empirical Literature on Whole Networks.” Journal of Management 33 (3): 479–516. doi:10.1177/0149206307302554.
- Provan, K. G., and P. Kenis. 2008. “Modes of Network Governance: Structure, Management, and Effectiveness.” Journal of Public Administration Research and Theory 18 (2): 229–52. doi:10.1093/jopart/mum015.
- Provan, K. G., J. Sydow, and N. P. Podsakoff. 2017. “Network Citizenship Behavior: Toward a Behavioral Perspective on Multi-Organizational Networks.” In Culture and Behavioral Strategy, edited by T. K. Das, 155–87. Charlotte, NC: IAP.
- Raab, J., and P. Kenis. 2009. “Heading Toward a Society of Networks.” Journal of Management Inquiry 18 (3): 198–210. doi:10.1177/1056492609337493.
- Riketta, M., and S. Nienaber. 2007. “Multiple Identities and Work Motivation: The Role of Perceived Compatibility between Nested Organizational Units.” British Journal of Management 18 (S1): S61–S77. doi:10.1111/j.1467-8551.2007.00526.x.
- Ringle, C., S. Wende, and J.-M. Becker. 2015. SmartPLS 3. Boenningstedt: SmartPLS GmbH. http://www.smartpls.com.
- Rouzies, A. 2011. “Antecedents of Employees’ Identification with a Merger. A Three-Stage Empirical Study.” International Studies of Management & Organization 41 (3): 25–41. doi:10.2753/IMO0020-8825410302.
- Sammarra, A., and L. Biggiero. 2001. “Identity and Identification in Industrial Districts.” Journal of Management and Governance 5 (1): 61–82. doi:10.1023/A:1017937506664.
- Scott, S., M. Hughes, and S. Kraus. 2019. “Developing Relationships in Innovation Clusters.” Entrepreneurship & Regional Development 31 (1–2): 22–45. doi:10.1080/08985626.2018.1537145.
- Skinner, L. R., C. W. Autry, and C. W. Lamb. 2009. “Some Measures of Interorganizational Citizenship Behaviors: Scale Development and Validation.” International Journal of Logistics Management 20 (2): 228–42. doi:10.1108/09574090910981314.
- Sluss, D. M., R. E. Ployhart, M. G. Cobb, and B. E. Ashforth. 2012. “Generalizing Newcomer’s Relational and Collective Identifications: Processes and Prototypicality.” Academy of Management Journal 55 (4): 949–75. doi:10.5465/amj.2010.0420.
- Sölvell, Ö. 2008. Clusters: Balancing Evolutionary and Constructive Forces. Stockholm: Ivory Tower Publishing.
- Staber, U. 2010. “Imitation without Interaction: How Firms Identify with Clusters.” Organization Studies 31 (2): 153–74. doi:10.1177/0170840609357369.
- Sydow, J., F. Lerch, C. Huxham, and P. Hibbert. 2011. “A Silent Cry for Leadership: Organizing for Leading (in) Clusters.” The Leadership Quarterly 22 (2): 328–43. doi:10.1016/j.leaqua.2011.02.008.
- Tajfel, H. 1978. “Social Categorization, Social Identity and Social Comparison.” In Differentiation between Social Groups. Studies in the Social Psychology of Intergroup Relations, edited by H. Tajfel, 61–76. London: Academic Press.
- Tallman, S., M. Jenkins, N. Henry, and S. Pinch. 2004. “Knowledge, Clusters and Competitive Advantage.” Academy of Management Review 29 (2): 258–71. doi:10.2307/20159032.
- Turner, J. C. 1984. “Social Identification and Psychological Group Formation.” In The Social Dimension: European Developments in Social Psychology, edited by H. Tajfel, 15–40. 2nd ed. Cambridge: Cambridge University Press.
- Van Dick, R., M. W. Grojean, O. Christ, and J. Wieseke. 2006. “Identity and the Extra Mile: Relationships between Organizational Identification and Organizational Citizenship Behaviour.” British Journal of Management 17 (4): 283–301. doi:10.1111/j.1467-8551.2006.00520.x.
- Williams, P. 2002. “The Competent Boundary Spanner.” Public Administration 80 (1): 103–24. doi:10.1111/1467-9299.00296.
- Yang, Z., and C. L. Wang. 2011. “Guanxi as a Governance Mechanism in Business Markets: Its Characteristics, Relevant Theories, and Future Research Directions.” Industrial Marketing Management 40 (4): 492–5. doi:10.1016/j.indmarman.2010.12.004.
Appendix A.
Measurement items, constructs, validity and reliability
Appendix B. Discriminant validity analysis: Fornell and larcker’s criterion