ABSTRACT
Most biology undergraduates learn about mutations in multiple classrooms throughout their college career. Understanding personalised genome test results, genome editing controversies, and the appearance of new variants of viruses or antibiotic resistant bacteria all require foundational knowledge about mutations. However, the abstract nature of molecular processes surrounding mutations makes them one of the more difficult topics for students to understand and apply. Instructors need valid assessment tools to document student understanding and tailor their instructional methods to address student knowledge gaps. We describe here the development and validation of the Mutations Criterion Referenced Assessment (MuCRA). This formative assessment was developed through an iterative process involving expert feedback and student responses to both open-ended and multiple-choice questions. The final MuCRA is composed of 10 multiple-choice questions aligned with three learning objectives. The item difficulty for each question was between 0.32–0.65, while the discrimination index ranged from 0.31–0.75 and the reliability (KR20) for the MuCRA was 0.69. The congruence analyses demonstrated distractors are capturing student misconceptions in 9/10 questions. These data indicate that the MuCRA can be used to reliably assess student learning and common misconceptions about mutations.
Introduction
Both the United States and the European Union describe science literacy skills as scientific knowledge combined with an understanding of the interactions between science and society (Stern and Kampourakis Citation2017). While knowledge of basic genetics principles is key to genetics literacy, barriers to both teaching and learning genetics include the abstract nature of a gene, students’ lack of statistical reasoning skills necessary to understand transmission genetics, faculty use of discipline-specific terminology and symbols, and students’ lack of understanding of the process of cellular division (Knippels, Waarlo, and Boersma Citation2005). Furthermore, these difficulties are often mirrored in the general public’s understanding (Lanie et al. Citation2004), where misconceptions are reinforced by genetics misinformation used as a plot device in movies and other media (Kampourakis Citation2017; Roberts et al. Citation2018; Muela and Abril Citation2014). One phenomenon that is persistently and pervasively used inaccurately is mutation. Young individuals are introduced to and retain incorrect ‘mutation’ and ‘mutant’ terminology and ideas from comic books, cartoons, and movies, such as the Teenage Mutant Ninja Turtles (Klaehn Citation2015) and the X-Men (Trushell Citation2004). This is particularly troubling given the dark history of eugenics and the use of genetic pseudoscience to justify racism, ableism, and sexism (Hales Citation2020). While popular culture may refer to individuals as mutants, it is stigmatising to those affected by genetic conditions. One way to avoid these problems is to precisely define and apply the concept of mutation as used by biologists.
Inaccuracies related to mutations often lead to misunderstandings regarding truly foundational concepts in biology, including inheritance, evolution, genetic risk assessment, and genetic technologies. Conceptual accuracy is necessary for scientists and citizens to understand and knowledgably use the products of genetic technology. For example, the techniques needed to generate genetically modified organisms (GMO), which are often misunderstood and vilified, are nearly identical to those used in gene therapy and genetic testing, which are frequently praised (Hekmat and Dawson Citation2019). Precision genome editing is now not only feasible, but relatively easy, using CRISPR-based technologies (Ran et al. Citation2013). Mutations in SARS-CoV2, the virus that causes COVID-19, produced the Delta, Omicron, and other variants of concern (Braund Citation2021). For these reasons, understanding the nature, consequences, and applications of mutations is crucial to developing the genetics literacy skills needed to make informed health decisions.
The Genetics Society of America (GSA) has identified mutations as a core concept in their curricular recommendations (Committee, Genetics Society of America Education Citation2016) and most introductory genetics courses include mutations. However, biology educators currently lack tools to reliably assess students’ abilities to define and apply their knowledge concerning mutations. Misconceptions, or alternate conceptions, are the inaccurate ideas and meanings that students associate with science concepts (Bahar, Johnstone, and Hansell Citation1999). These conceptions are frequently linked to past experiences and intuitive thinking rather than empirical data (Coley and Tanner Citation2015; Prokop, Fančovičová, and Krajčovičová Citation2016). For example, a common biology misconception is that different cells in the body have different genes (Coley and Tanner Citation2012a). Identifying which misconceptions students hold can inform teaching practices. Therefore, it is important to create an assessment for educators to accurately gauge mutation conceptual understanding and identify the nature of student misconceptions. With validated and reliable assessment tools, practicing faculty can improve their classroom pedagogy as well as compare teaching and learning across classrooms.
Concept inventories (CIs) are a specific subset of criterion referenced assessments (CRA) that are multiple-choice instruments used to gauge students’ conceptual understanding about a topic (Hestenes, Wells, and Swackhamer Citation1992; Klymkowsky, Garvin-Doxas, and Zeilik Citation2003; Smith, Wood, and Knight Citation2008; Gurel, Eryılmaz, and McDermott Citation2015). A CRA investigates performance relative to specific criteria (McDonald Citation2013). A key feature of concept inventories is the multiple-choice questions with answer options that include common student errors in thinking or misconceptions. These student misconceptions are identified through teaching experience, interviews with students, and open-ended questionnaires in the pilot stage of the instrument. The distractors allow educators to identify and quantify the number and distribution of different misconceptions within a target student population before and after instruction.
Concept inventories in biology
Within the last two decades, biology educators have published multiple instruments for various biological concepts (Klymkowsky, Garvin-Doxas, and Zeilik Citation2003; Stefanski, Gardner, and Seipelt-Thiemann Citation2016; Paustian et al. Citation2017; Smith, Wood, and Knight Citation2008). The Genetics Concept Assessment (GCA) broadly captures student understanding and misconceptions of multiple genetic concepts including transmission, population, and molecular genetics (Smith, Wood, and Knight Citation2008). Since it was published in 2008, the GCA has been cited over 300 times. It has been used to identify common genetics errors (Coley and Tanner Citation2012a; Smith and Knight Citation2012) and study the effectiveness of teaching methods (Andrews et al. Citation2011; Levesque Citation2011). Focused assessments, such as the Lac Operon Concept Inventory (Stefanski, Gardner, and Seipelt-Thiemann Citation2016), are more specific and assess changes in student understanding of a single concept (Ones and Viswesvaran Citation1996). Generalised knowledge and overall skills are better assessed with broad, general assessments, while specific skills and specialised knowledge are better determined by focused, specific instruments (Spector Citation2012; Ones and Viswesvaran Citation1996). Smaller, more focused assessments have the potential for a large benefit because they make timely changes to teaching strategies possible when they are used immediately after a concept is taught. The larger concept inventories, such as the GCA are typically used to assess the entire course, which means the post test is often given during the last week of class, when it is too late to intervene to help students that are struggling. When aligned with specific, measurable, learning objectives, these focused criterion referenced assessments can be used to measure the relative efficacy of different learning tools and methods for a specific concept.
The goal of this research project was to construct a valid and reliable criterion referenced assessment (MuCRA) that: 1) is based in broadly-accepted criteria used by the Genetics Society of America (GSA) and learning objectives identified by experienced educators and 2) accurately measures student understanding of those learning objectives in a wide variety of settings.
Methods
Participants and context
This study was conducted at seven institutions across the United States: Utah Valley University (UVU), Bridgewater State University (BSU), University of Wisconsin at La Crosse (UWL), University of Northwestern at St. Paul (UoN), Iowa State University (ISU), Middle Tennessee State University (MTSU), and University of North Carolina at Asheville (UNC). These institutions include two doctoral universities (R1 and D/PU), three master’s universities (M1, M2, and M3), and two baccalaureate colleges based on their Carnegie classification in Fall 2017. The schools ranged in size from 37,282 to 1,889 students; underrepresented minority student populations range from 11% to 24%, and female student population ranges from 43% to 61%. Data collection occurred across five semesters: Fall 2017, Spring 2018, Fall 2018, Spring 2020 and Summer 2020. Student participants were enrolled in a variety of courses depending on the institution and were registered as either biology majors or non-majors. The Internal Review Board (IRB) from each institution approved this study, with ISU and MTSU being granted primary approval, and collaborating school institutional review board’s (IRB) reviewing the ISU documentation and approving the project described as exempt, giving it their own approval code, or using the ISU approval code (#17-213): (MTSU IRB18-1002, ISU (#17-213); Utah Valley University: (IRB #01995); Bridgewater (Exempt); UW-Lacrosse (Approved ISU IRB 17–213); NW-St. Paul (Exempt); UNC-Asheville (Exempt)).
Table 1. Developing Mutation Learning Objectives based on GSA guidelines
Table 2. Description of validity and reliability statistical measures.
Mutations criterion referenced assessment design overview
The Mutations Criterion Referenced Assessment (MuCRA) was developed using established methodology for concept inventory instrument design (Adams and Wieman Citation2011; D’Avanzo Citation2008; Kalas et al. Citation2013; Paustian et al. Citation2017; Smith, Wood, and Knight Citation2008; Stefanski, Gardner, and Seipelt-Thiemann Citation2016), which included faculty input and student feedback at multiple stages. The MuCRA was created using a four-phase, iterative process involving fourteen steps with feedback from faculty experts at three points, gathering student responses at four points, and data-driven revision of questions and answers at four points (). The four phases were as follows: (I) establishing learning objectives and developing open-ended questions using feedback from multiple genetics faculty, (II) creating multiple choice questions using student words and phrasing where possible, (III) revising and removing multiple choice questions to create the final instrument, and (IV) confirming the discriminant validity of distractors. Collection and analysis of student responses occurred at the end of each phase (: Steps 5, 8, 10, & 13). During steps 9 and 12, we used psychometric methods to evaluate the reliability (KR-20), item difficulty, and internal consistency (point biserial and item discrimination) of each question and the MuCRA as a whole (McDonald Citation2013) (; ).
Table 3. Psychometric Data Table.
Figure 1. Development and testing of the Mutations Criterion Referenced Assessment (MuCRA). The MuCRA was developed in four steps. First (1), the research team developed learning objectives based on their experience, the Genetics Society of America’s curricular guidelines, and feedback from teaching faculty (2). The team then developed open ended questionsto probe student understanding of learning objectives and elicited feedback (3-4) before gathering student data (5). These data were analyzed to identify and encode common errors (6) that were used to develop multiple choice questions (7). We gathered initial and analyzed initial student response data (7-8) and removed 4 questions (9). In phase III, we gathered student and faculty data (10-11) from multiple classrooms and removed 3 questions (12).We confirmed distractors were capturing student thinking by gathering and analyzing student data (13) in phase IV.
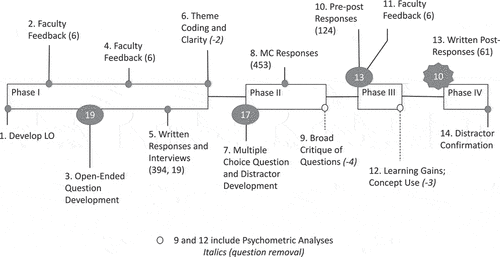
Establishing learning objectives
The research team developed learning objectives based on four major concepts related to mutations ().
These learning objectives were written based on GSA’s Genetics Learning Framework (Committee, Genetics Society of America Education Citation2016) for undergraduate genetics education, Vision and Change for Undergraduate Biology Education Core Competencies (Brewer and Smith Citation2009; Brownell et al. Citation2014), and the expertise of two biology faculty with decades of experience teaching general genetics. The first and most basic learning objective is that students should be able to define mutation (Learning Objective 1; LO1). Predicting the outcome of a DNA change connects genotype to phenotype, and DNA to other cellular components and processes. Students should therefore be able to categorise changes to DNA and predict the effect of these changes on proteins using the universal genetic code table (Learning Objective 2: LO2). Mutations in germline cells behave differently than those in somatic cells. This is a core concept in transmission genetics and this physical basis of mutations and inheritance affects understanding of other topics (e.g. mitosis, meiosis, gene therapy, fertilisation, and transgenic expression). Students should be able to differentiate between somatic and germline mutations and predict the inheritance patterns of each type of mutation (Learning Objective 3: LO3). To demonstrate understanding of the mechanisms by which mutations induce mutations, students should be able to predict the nature of changes to DNA exposed to intercalating agents, base analogues, and radiation (Learning Objective 4: LO4).
We distributed these preliminary learning objectives to six faculty from four different institutions (Iowa State University, Middle Tennessee State University, University of California-Irvine/Maastricht University, University of Northwestern at St Paul) with expertise in teaching undergraduate genetics concepts (: Step 2). Faculty provided feedback regarding: 1) whether each learning objective was appropriate to how they taught their classes, 2) if any concepts were missing or unnecessary, and 3) whether the faculty included each concept in their courses. This expert feedback confirmed that the four learning objectives reflected their teaching practices and course goals.
Open-ended question development and review
The team designed 19 open-ended questions to probe student understanding of the four learning objectives and capture student wording and phrasing (: Step 3), 10 of which remain in the final multiple-choice criterion referenced assessment (MuCRA). After we created the initial open-ended questions, six faculty who teach genetics reviewed them to verify that each question aligned with stated learning objectives (: Step 4). Before testing in classrooms, these open-ended questions were revised for clarity based on feedback from this faculty group.
Gathering open-ended student responses
We gathered student written responses to these questions in general biology and genetics courses post-instruction at both ISU and MTSU. Additionally, 19 students who completed the open-ended questionnaire self-selected to participate in interviews (: Step 5). During the interviews, students were given their responses from the open-ended questions and asked to re-answer the questions and explain their reasoning for their answers using a think-aloud method (Padilla and Leighton Citation2017). The research team analysed student written responses (n = 394) and transcribed student interview responses (n = 19) to: 1) identify student phrasing that could be used to make the questions, 2) diagnose problems with question readability, and 3) document common student errors that could be used as distractor answers. We evaluated each question based on student responses (: Step 6). One open-ended question was discarded due to question redundancy (LO1) and another was discarded due to unclear question wording (LO2). Following this step, the questions representing LO1 and LO2 were each reduced from five to four questions. At the end of Phase I, the MuCRA consisted of 17 questions.
Multiple-choice question design
During Phase II of development, we re-formatted the open-ended questions as multiple-choice questions (: Step 7). We constructed a codebook based on the student answers to each question by individually coding 10% of the student responses, and discussing similar mistakes to group into broader code categories. We incorporated student wording, phrasing, and reasoning into both the correct and distractor responses from the open-ended question responses and interview data (Supplement #1). The team constructed the multiple choice question responses (both correct and incorrect) using assessment design best practices so that responses had equivalent lengths, similar phrasing, and the correct answer placed randomly for each question (Haladyna, Downing, and Rodriguez Citation2002).
Initial multiple-choice assessment testing and psychometric analyses
We gathered post-instruction multiple choice response data from 453 students across two universities (ISU and MTSU) at the end of the Fall semester of 2018 (: Step 8). Data were combined and used to calculate preliminary validity and reliability measures ().
Table 4. Congruence Probabilities.
Such as KR20, item difficulty, item discrimination, and point-biserial correlation coefficient using IBM SPSS® (Field Citation2013) (See ‘Assessment Validation” for details of the techniques used in this study). Based on psychometric analyses, four additional questions were removed from the instrument. (: Step 9). One question was removed from LO1 to more evenly distribute the questions across the learning objectives; this question was chosen for removal because it was very similar to another question in LO1. Due to the frequency of incorrect student answers and low point biserial coefficients, two questions were removed from LO3. Finally, one question was removed from LO4 because it required prior knowledge of mitosis and the eukaryotic cell cycle as well as the targeted objective of mutagen action. At the end of Phase II, the MuCRA consisted of 13 questions (: Step 9).
Secondary assessment testing and psychometric analyses
In Phase III, the revised MuCRA was tested in multiple classrooms at various undergraduate levels of experience (i.e. general biology, general genetics, and advanced genetics courses) across seven higher education institutions. The revised MuCRA was given as a pre- (n = 286) and post-test (n = 302) with 124 students answering both pre- and post-tests (: Step 10).
We used post-instruction data to calculate the KR20, item difficulty, item discrimination, and point-biserial correlation coefficient using IBM SPSS®. Several faculty using the Phase III MuCRA reported not teaching mutagen mechanisms in their classes (: Step 11). Unsurprisingly, these mutagen questions showed modest learning gains for this learning objective and the psychometric data indicated these questions had less discriminatory power than the rest of the MuCRA. We removed three more questions, eliminating LO4 entirely (: Step 12). The shortened criterion referenced assessment more accurately reflects the learning objectives taught in general biology and genetics courses. At the end of Phase III, the final MuCRA consisted of 10 questions: three in LO1, four in LO2, and three in LO3 (: Step 12).
Assessment validation
The aim of this project was to design an assessment tool for instructors to measure undergraduate student understanding of mutation concepts. A well-designed assessment tool can accurately assess the efficacy of specific educational activities and can also be used to assess prior knowledge and determine how student reasoning changes as students move from naïve to more expert-like thinking. A criterion referenced assessment should have the ability to accurately measure understanding of described criteria, or learning objectives (i.e. the instrument should be valid in the context given) (Glaser Citation1963) and should be able to consistently evaluate this understanding in different contexts (i.e. the instrument should be reliable). In order to evaluate validity and reliability, we designed and evaluated the mutations criterion referenced assessment (MuCRA) using several common psychometric statistics, including Kuder-Richardson 20 (KR-20), item difficulty, discrimination index (D27), and point-biserial correlation (). We further tested each question and the instrument as a whole by calculating the discrimination index and point-biserial correlation of each question. The discrimination index is a measure of each question’s ability to distinguish between top- and low-performing students. The generally accepted threshold for the discrimination index for assessment questions is 0.30 (Ebel Citation1954). The point-biserial correlation measures the correlation between student responses on one question to their overall test score to determine if each student responses to each question are consistent with the overall performance on the instrument.
While the use of Rasch analysis or other item response theory (IRT) methods to validate assessments has increased in the past decade, these statistical models assume unidimensionality (Hambleton, Zenisky, and Popham Citation2016), meaning the assessment conceptual understanding of a single dimension of information. Since the MuCRA has three separate learning objectives that probe understanding at multiple levels (definition, molecular application, organismal effects), the assumption of unidimensionality is questionable in this context (Huynh Citation2010). To test the assumption that student responses could be reduced to a single dimension of information or conceptual understanding, we ran separate non-parametric multidimensional scaling (nMDS) analyses on the data specifying solution dimensionalities 1 through 9, and examined the stress measurement for each nMDS run. Stress is a measure of the difference between the data and the model, and a stress of zero indicates that the model is over-fit (Kruskal Citation1964). For the pre-test data, stress was zero in solution dimensions 5–10, while for the post-test data the ordination returned non-zero stress values for the first three solution dimensions. These results suggest that the pre-test data contain useful information in up to four dimensions, while the post-test data are informative in up to three. Classical test theory does not rely on the assumption of unidimensionality that is required for Rasch analysis and other IRT models (De Champlain Citation2010; Hambleton and Rogers Citation1989; Hambleton and Jones). We therefore used the well-established tools from classical test theory () and distance-based ordination methods rather than IRT to investigate the relationships among learning objectives, MuCRA questions, and student responses.
We computed a permutational multivariate analysis of variance (PERMANOVA) and non-metric multidimensional scaling (nMDS) using the vegan package (Oksanen Citation2019) in R version 4.0.2 (R Core Team Citation2020) to determine if student responses clustered by learning objective (Napior Citation1972; Jaworska and Chupetlovska-Anastasova Citation2009), and to compare student response patterns for questions given prior to and after instruction. The function vegdist was used to create two Jaccard dissimilarity matrices from binary-coded data (correct/incorrect student response) for pre- and post-instruction (one matrix each, n = 286 and 302 respectively, with 124 students included in both datasets); analyses were conducted on each dissimilarity matrix separately. To test if learning objectives significantly contributed to clustering of student responses, we conducted a PERMANOVA using the adonis function with 9,999 permutations. Because PERMANOVA is sensitive to heterogeneity of variance for unbalanced designs (Anderson Citation2017), heteroscedasticity tests were also performed using the betadisper function and permutest function with 9,999 permutations.
Results from the literature indicate that for ten observations (i.e. ten questions on the MuCRA) an nMDS solution should be calculated using no more than 2 dimensions (Kruskal Citation1964; Shepard Citation1974). We therefore used the metaMDS function to calculate 2-dimensional solutions (specifying k = 2) and plotted results using the ggplot2 (Wickman Citation2016) and ggConvexHull packages (Martin Citation2017).
Distractor confirmation congruence analysis
Students in two general genetics classes (Spring and Summer 2020) were given the final MuCRA and asked to explain the reasoning behind their answer choice. For coding, multiple-choice responses (n = 61) were hidden from the coder and the rationale coded as if it was a short-answer response to an open-ended question using the rationale codebook established during Phase I of the MuCRA construction (: Step 13). These codes were compared with the misconception code each multiple-choice distractor was designed to capture as well. There are four possible relationships between students’ multiple-choice responses and their explanations: (1) students chose the correct multiple-choice response and gave the correct reasoning for their response, coded as congruent-correct, (2) students chose a multiple-choice distractor and their reasoning matched the misconception the distractor was designed to capture, coded as congruent-incorrect, (3) students chose the correct multiple-choice response but their reasoning contained a misconception or error in reasoning, coded as incongruent-correct, and (4) students chose a distractor and their reasoning showed a different misconception than the one used for design of the distractor, coded as incongruent-incorrect. The details of the congruence analysis are found in Supplement #2.
Results & discussion
The MuCRA is both valid and reliable when used in college courses
The MuCRA provides valid and reliable data to measure undergraduate students’ understanding of core learning objectives related to mutations as taught in general genetics, microbiology, and biology classrooms. Student learning gains were not significantly different for males compared to females, first-generation students compared to non-first-generation students, multiple ethnic groups, or grade in school (data not shown). When determining the overall test reliability, the KR20 was found to be 0.64 for the pre-test (n = 285) and 0.69 for the post-test (n = 301), indicating that the assessment is reliable when used in the context of undergraduate biological sciences courses. While the KR-20 values are slightly below the optimal 0.7 threshold, the KR-20 assumes item homogeneity. Since the MuCRA is comprised of three separate learning objectives, this range of content reduces item homogeneity, which in turn reduces the KR-20 value for internal consistency (Cortina Citation1993).
The item difficulty is another psychometric parameter used to assess newly developed instruments. The item difficulty measures the proportion of students answering each question correctly. This means items with a higher item difficulty are actually easier than those with a lower item difficulty. The item difficulty for the pre-testing of the MuCRA questions had a range of 0.30–0.60, with four questions being more difficult (Q1 = 0.30, Q3 = 0.32, Q4 = 0.34, Q5 = 0.32), five questions being in the middle range (Q2 = 0.46, Q6 = 0.55, Q7 = 0.42, Q8 = 0.41, Q10 = 0.41), and one question that was relatively easy (Q9 = 0.60). For the post-test of the MuCRA questions, the item difficulty had a range of 0.32–0.67, with three questions being more difficult (Q1 = 0.32, Q3 = 0.35, Q4 = 0.35), five questions being in the middle range (Q2 = 0.49, Q5 = 0.43, Q7 = 0.53, Q8 = 0.46, Q10 = 0.55), and two questions that were relatively easy (Q6 = 0.67, Q9 = 0.65). The average item difficulty for LO1 (Define Mutation) was 0.36 before and 0.39 after instruction, LO2 (Categorise changes in DNA) moved from 0.40 before to 0.53 after instruction, and for LO3 (Differentiate between somatic and germline) pre- was 0.47 and post-instruction was 0.55. The average item difficulties for the pre- and post-instruction MuCRA administration were 0.41 and 0.48, respectively. A range of difficulty among the questions, as indicated by the range of item difficulty values, is desirable for a criterion referenced assessment because it helps widen the range of scores, and gives instructors information on which concepts most students understand and which more challenging. A moderate average item difficulty across both the pre- and post-tests of the MuCRA and for each learning objective shows that the test is neither too easy nor too hard and can therefore be used at many different course levels.
The discrimination index was above the accepted threshold of 0.30 for all questions over both the pre- and post-instruction administrations. Prior to instruction the discrimination index for the questions of the MuCRA ranged from 0.31–0.79 with an average of 0.6, while post-instruction values ranged from 0.31–0.75 with an average of 0.5. For the pre-test of the MuCRA, questions had a point-biserial correlation in the range of 0.08 to 0.79, with most questions above the optimal threshold of 0.30 (Nunnally Citation1978). The point biserial correlation for the questions in the post-test were between 0.12 and 0.48, with seven questions above the optimal threshold of 0.30. Three questions (Q 1, 4, 5) that did not meet the 0.3 threshold for point biserial were more difficult than the MuCRA average and each had an item discrimination above the optimal 0.3. This suggests that while the correlation between scoring well on the assessment and answering these questions correctly was not high, these items were still able to discriminate between high and low performing students. We retained these in the final MuCRA as challenge items for high-performing students. The average point biserial correlations for both the pre- and post- test questions were at or above 0.30 (0.30 and 0.35). As a whole, when considering all classical test theory psychometric data, the MuCRA to generates valid and reliable data for each learning objective in a variety of different college classrooms.
Student responses to the MuCRA grouped by learning objective
We next used clustering analysis to determine the relationship of student scores to learning objectives which measured different concepts at multiple difficulty and Bloom’s taxonomic levels (Crowe, Dirks, and Wenderoth Citation2008; Lemons and Lemons Citation2013). The first learning objective is based on understanding the definition of a mutation and requires fundamental skills of remembering, identifying, and understanding (Crowe, Dirks, and Wenderoth Citation2008). The second learning objective (LO2) requires students to apply their knowledge of translation in the context of reading the universal genetic code table. The third learning objective (LO3) contains contextual (story-problem) questions that measure synthesis of mutation and cell division knowledge simultaneously. For this analysis, both dissimilarity matrices (pre- and post-tests) met assumptions for equal variance among learning objective groups (H0: no difference in variance among groups; pre-test: F(2,7) = 0.97, p = 0.426; post-test: F(2,7) = 1.79, p = 0.271). PERMANOVA tests for both pre- and post-instruction indicated that in both cases learning objectives significantly contributed to clustering (H0:adding learning objective to the model does not improve model fit; pre-test: Pseudo F(2,7) = 1.29, p = 0.016; post-test: Pseudo F(2,7) = 1.48, p = 0.0094). The first two axes provided good separation for all three learning objectives (: A, B) and at least two dimensions were needed to represent the three learning objectives. While the grouping for LO1 was more distant in post-instruction measurements, both plots show similar separations between pre- and post-instruction. In the pre-test, we observed clear separation between the three learning objectives, with the second and third appearing closer to each other than either one was to LO1. After instruction, the lower-order (remembering/understanding) learning objective (LO1) was distinct from both higher-order objectives (LO2 & LO3); however, LO3 questions clustered more tightly and also clustered with the questions of the second learning objective. These findings are consistent with a 2013 study that describes questions probing higher-order cognitive skills as multi-faceted (Lemons and Lemons Citation2013). The application questions were of higher complexity on the Bloom’s scale, and responses depended on student experience. This movement to overlap in LO2 and LO3 post-instruction may reflect the acquisition of more advanced higher order skills in students that lacked them prior to instruction.
Figure 2. nMDS plots. Non metric multidimensional scaling plots depicting grouping of MuCRA questions (Q1-Q10) by learning objectives for pre-instruction test data (A) and post-instruction test data (B). Learning objective 1 remains distinct from learning objectives 2 and 3 when the MuCRA is used before and after instruction.
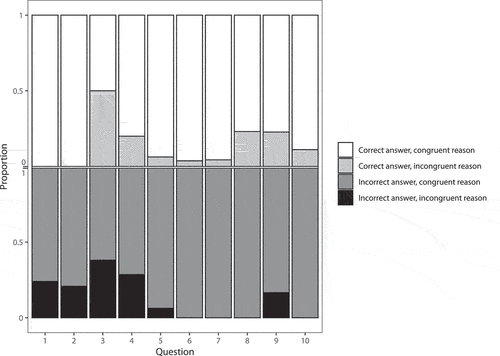
Distractor answers accurately captured their misconceptions
To determine distractor efficacy, we designed a novel confirmatory step that has not been reported for other concept inventories. Distractor congruence provides direct evidence that multiple-choice distractors accurately capture the misconceptions they were designed to address.
Proportions of congruent answers were higher than proportions of incongruent answers for all questions and all answer types (correct or incorrect), except for Question 3 where the proportion of correct congruent responses was exactly 50% (). Students that answered question 3 correctly provided inaccurate reasoning 50% of the time. Question 3 described a change to an intron splice site sequence and several explanations for correct responses focussed on the effects of mutation on the RNA rather than defining the mutation itself. Probabilities of observing the number of congruent answers under the null hypothesis fell between <0.0001 and 0.1723 for each question, with all probabilities <0.05 except for Question 3 (). Overall, congruence analysis confirmed that distractors effectively capture design misconceptions, although our confidence in distractor answers with very few or no responses is lower.
Figure 3 Congruence of student rationale with misconception code. For each question in the MuCRA, the proportion of incorrect answers (bottom half) or correct answers (top half) for which student self reported rationale was incongruent(dark) or congruent (light) with the targeted misconception for incorrect responses or correct reasoning for correct responses.
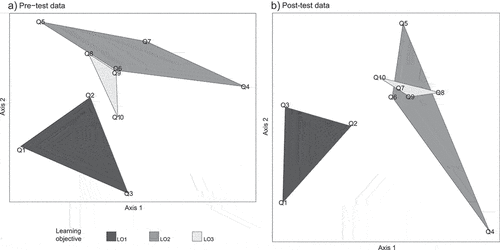
Conclusions
Implementation of concept inventory
While concept inventories are available for general biology (Knight Citation2010), genetics (Smith, Wood, and Knight Citation2008), and cell biology (Couch, Wood, and Knight Citation2015), these are too broad for specific concepts (Ones and Viswesvaran Citation1996). Instruments with a narrower scope, such as the MuCRA, are valuable because they are focused and capture more detail. They are used to measure student learning gains, evaluate a teaching practice, and determine the baseline knowledge of the students in a given class about a specific (targeted) aspect of genetics. The lac operon concept inventory (Stefanski, Gardner, and Seipelt-Thiemann Citation2016) has been used to assess several new teaching techniques used to teach students about gene regulation in prokaryotes. These include use of models ((Gordy et al. Citation2020), virtual reality (Lui, McEwen, and Mullally Citation2020), and computational modelling (Dauer et al. Citation2019).
The MuCRA has utility as a criterion referenced test designed to measure and identify student misconceptions about mutations. In addition, it supports innovation and evidence-based teaching by providing valid and reliable data useful in optimising learning. For example, faculty implementing a new case study to visualise molecular changes related to mutations can use the MuCRA before and after instruction to assess its effectiveness. The data gathered would be specific to mutations and of immediate use. Careful analysis of the pre-instruction data would help faculty identify both prior knowledge and common misconceptions. Faculty can also determine which concepts showed strong learning gains and where any mastery gaps persist after instruction. These data can then be used to inform course and curricular design for future terms. The MuCRA is available to instructors upon request and can be used in either a written (pdf) or digital (LASSO) format.
Lastly, we developed a final and direct measure of criterion referenced assessment validity: congruence analysis. This is a direct, rather than indirect, measure of how well distractors accurately capture the misconceptions for which they were designed and is an important measure for a robust criterion referenced assessment.
Supplemental Material
Download Zip (24.5 KB)Acknowledgments
The authors would like to thank the faculty and universities participating in MuCRA testing.
Disclosure statement
No potential conflict of interest was reported by the author(s).
Supplementary material
Supplemental data for this article can be accessed online at https://doi.org/10.1080/00219266.2022.2100451
Additional information
Funding
References
- Adams, W., and C. Wieman. 2011. “Development and Validation of Instruments to Measure Learning of Expert-Like Thinking.” International Journal of Science Education 33 (9): 1289–1312. doi:10.1080/09500693.2010.512369.
- Anderson, M. J. 2017. “Permutational Multivariate Analysis of Variance (PERMANOVA).” Wiley StatsRef: Statistics Reference Online 1–15. doi:10.1002/9781118445112.stat07841.
- Andrews, T. M., M. J. Leonard, C. A. Colgrove, and S. T. Kalinowski. 2011. “Active Learning Not Associated with Student Learning in a Random Sample of College Biology Courses.” CBE—Life Sciences Education 10 (4): 394–405. doi:10.1187/cbe.11-07-0061.
- Bahar, M., A. H. Johnstone, and M. H. Hansell. 1999. “Revisiting Learning Difficulties in Biology.” Journal of Biological Education 33 (2): 84–86. doi:10.1080/00219266.1999.9655648.
- Braund, M. 2021. “Critical STEM Literacy and the COVID-19 Pandemic.” Canadian Journal of Science, Mathematics and Technology Education 1–18. doi:10.1007/s42330-021-00150-w.
- Brewer, C. A., and D. Smith. 2009. Vision and Change in Undergraduate Biology Education. Washington DC: American Association for the Advancement of Science. https://visionandchange.org/wp-content/uploads/2010/03/VC_report.pdf
- Brownell, S. E., S. Freeman, M. P. Wenderoth, and A. J. Crowe. 2014. “BioCore Guide: A Tool for Interpreting the Core Concepts of Vision and Change for Biology Majors.” CBE-Life Sciences Education 13 (2): 200–211. doi:10.1187/cbe.13-12-0233.
- Coley, J. D., and K. D. Tanner. 2012a. “Common Origins of Diverse Misconceptions: Cognitive Principles and the Development of Biology Thinking.” CBE-Life Sciences Education 11 (3): 209–215. doi:10.1187/cbe.12-06-0074.
- Coley, J. D., and K. Tanner. 2015. “Relations between Intuitive Biological Thinking and Biological Misconceptions in Biology Majors and Nonmajors.” CBE-Life Sciences Education 14 (1): ar8. doi:10.1187/cbe.14-06-0094.
- Committee, Genetics Society of America Education. 2016. “Genetics Learning Framework.” Genetics Society of America, Accessed 15 October 2016. http://www.genetics-gsa.org/education/GSAPREP_CoreConcepts_CoreCompetencies.shtml
- Cortina, J. M. 1993. “What Is Coefficient Alpha? An Examination of Theory and Applications.” Journal of Applied Psychology 78 (1): 98. doi:10.1037/0021-9010.78.1.98.
- Couch, B. A., W. B. Wood, and J. K. Knight. 2015. “The Molecular Biology Capstone Assessment: A Concept Assessment for upper-division Molecular Biology Students.” CBE-Life Sciences Education 14 (1): ar10. doi:10.1187/cbe.14-04-0071.
- Crocker, L., and J. Algina. 1986. “Introduction to Classical and Modern Test Theory: ERIC”.
- Crowe, A., C. Dirks, and M. P. Wenderoth. 2008. “Biology in Bloom: Implementing Bloom’s Taxonomy to Enhance Student Learning in Biology.” CBE—Life Sciences Education 7 (4): 368–381. doi:10.1187/cbe.08-05-0024.
- D’Avanzo, C. 2008. “Biology Concept Inventories: Overview, Status, and Next Steps.” BioScience 58 (11): 1079–1085. doi:10.1641/B581111.
- Dauer, J. T., H. E. Bergan-Roller, G. P. King, M. Kjose, N. J. Galt, and T. Helikar. 2019. “Changes in Students’ Mental Models from Computational Modeling of Gene Regulatory Networks.” International Journal of STEM Education 6 (1): 1–12. doi:10.1186/s40594-019-0193-0.
- De Champlain, A. F. 2010. “A Primer on Classical Test Theory and Item Response Theory for Assessments in Medical Education.” Medical Education 44 (1): 109–117. doi:10.1111/j.1365-2923.2009.03425.x.
- Ebel, R. L. 1954. “Procedures for the Analysis of Classroom Tests.” Educational and Psychological Measurement 14 (2): 352–364. doi:10.1177/001316445401400215.
- Ebel, R. L., and D. A. Frisbie. 1972 Reliablity of Test Scores Essentials of Educational Measurement 1st (Englewood, NJ USA: Prentice-Hall).
- Field, A. 2013. Discovering Statistics Using IBM SPSS Statistics: Sage 4th (Los Angelos, CA: SAGE Publications Ltd), 915–978. http://hdl.handle.net/123456789/12816
- Glaser, R. 1963. “Instructional Technology and the Measurement of Learing Outcomes: Some Questions.” American Psychologist 18 (8): 519. doi:10.1037/h0049294.
- Gordy, C. L., C. I. Sandefur, T. Lacara, F. R. Harris, and M. V. Ramirez. 2020. “Building the Lac Operon: A guided-inquiry Activity Using 3D-printed Models.” Journal of Microbiology & Biology Education 21 (1): 60. doi:10.1128/jmbe.v21i1.2091.
- Gurel, D. K., A. Eryılmaz, and L. C. McDermott. 2015. “A Review and Comparison of Diagnostic Instruments to Identify Students’ Misconceptions in Science.” Eurasia Journal of Mathematics, Science & Technology Education 11 (5). doi:10.12973/eurasia.2015.1369a.
- Haladyna, T. M., S. M. Downing, and M. C. Rodriguez. 2002. “A Review of multiple-choice item-writing Guidelines for Classroom Assessment.” Applied Measurement in Education 15 (3): 309–333. doi:10.1207/S15324818AME1503_5.
- Hales, K. 2020. “Signaling Inclusivity in Undergraduate Biology Courses through Deliberate Framing of Genetics Topics Relevant to Gender Identity, Disability, and Race.” CBE—Life Sciences Education 19 (2): es2. doi:10.1187/cbe.19-08-0156.
- Hambleton, R. K., and H. J. Rogers. 1989. “Solving criterion-referenced Measurement Problems with Item Response Models.” International Journal of Educational Research 13 (2): 145–160. doi:10.1016/0883-0355(89)90003-7.
- Hambleton, R. K., A. L. Zenisky, and W. J. Popham. 2016. “Criterion-referenced Testing: Advances over 40 Years.” Educational Measurement: From Foundations to Future. 23–37.
- Hekmat, S., and L. N. Dawson. 2019. “Students’ Knowledge and Attitudes Towards GMOs and Nanotechnology.” Nutrition and Food Science 49 (4): 628–638. doi:10.1108/NFS-07-2018-0193.
- Hestenes, D., M. Wells, and G. Swackhamer. 1992. “Force Concept Inventory.” The Physics Teacher 30 (3): 141–158. doi:10.1119/1.2343497.
- Huynh, H. 2010. “Psychometric Aspects of Item Mapping for criterion-referenced Interpretation and Bookmark Standard Setting.”Journal of Applied Measurement 11(1): 91–98, PMID: 20351450.
- Jari Oksanen, F., G. Blanchet, M. Friendly, R. Kindt, P. Legendre, D. McGlinn, P. R. Minchin, et al. (2020). vegan: Community Ecology Package. R package version 2.5-7. https://CRAN.R-project.org/package=vegan
- Jaworska, N., and A. Chupetlovska-Anastasova. 2009. “A Review of Multidimensional Scaling (MDS) and Its Utility in Various Psychological Domains.” Tutorials in Quantitative Methods for Psychology 5 (1): 1–10. doi:10.20982/tqmp.05.1.p001.
- Kalas, P., A. O’Neill, C. Pollock, and G. Birol. 2013. “Development of a Meiosis Concept Inventory.” CBE-Life Sciences Education 12 (4): 655–664. doi:10.1187/cbe.12-10-0174.
- Kampourakis, K. 2017. “Public Understanding of Genetic Testing and Obstacles to Genetics Literacy” . In Molecular Diagnostics. Third. 469–477, Elsevier, doi:10.1016/B978-0-12-802971-8.00027-4.
- Klaehn, J. 2015. “Synergy and Synthesis: An Interview with Comic Book Creator Benjamin Marra.” Journal of Graphic Novels and Comics 6 (3): 284–292. doi:10.1080/21504857.2014.943413.
- Klymkowsky, M., K. Garvin-Doxas, and M. Zeilik. 2003. “Bioliteracy and Teaching Efficacy: What Biologists Can Learn from Physicists.” Cell Biology Education 2 (3): 155–161. doi:10.1187/cbe.03-03-0014.
- Knight, J. 2010. “Biology Concept Assessment Tools: Design and Use.” Microbiology Australia 31 (1): 5–8. doi:10.1071/MA10005.
- Knippels, M.-C. P. J., A. J. Waarlo, and K. T. Boersma. 2005. “Design Criteria for Learning and Teaching Genetics.” Journal of Biological Education 39 (3): 108–112. doi:10.1080/00219266.2005.9655976.
- Kruskal, J. B. 1964. “Multidimensional Scaling by Optimizing Goodness of Fit to a Nonmetric Hypothesis.” Psychometrika 29 (1): 1–27. doi:10.1007/BF02289565.
- Lanie, A. D., T. E. Jayaratne, J. P. Sheldon, S. L. R. Kardia, E. S. Anderson, M. Feldbaum, and E. M. Petty. 2004. “Exploring the Public Understanding of Basic Genetic Concepts.” Journal of Genetic Counseling 13 (4): 305–320. doi:10.1023/B:JOGC.0000035524.66944.6d.
- Lemons, P., and J. D. Lemons. 2013. “Questions for Assessing higher-order Cognitive Skills: It’s Not Just Bloom’s.” CBE—Life Sciences Education 12 (1): 47–58. doi:10.1187/cbe.12-03-0024.
- Levesque, A. A. 2011. “Using Clickers to Facilitate Development of problem-solving Skills.” CBE—Life Sciences Education 10 (4): 406–417. doi:10.1187/cbe.11-03-0024.
- Lui, M., R. McEwen, and M. Mullally. 2020. “Immersive Virtual Reality for Supporting Complex Scientific Knowledge: Augmenting Our Understanding with Physiological Monitoring.” British Journal of Educational Technology 51 (6): 2181–2199. doi:10.1111/bjet.13022.
- Martin, C. A. 2017. “ggConvexHull: Add a Convex Hull Geom to ggplot2.”
- McCowan, R. J., and S. C. McCowan. 1999. “Item Analysis for Criterion-Referenced Tests.” Online Submission. ERIC Number: ED501716.
- McDonald, R. P. 2013. Test Theory: A Unified Treatment. Psychology Press. doi:10.4324/9781410601087.
- Muela, F. J., and A. M. Abril. 2014. “Genetics and Cinema: Personal Misconceptions that Constitute Obstacles to Learning.” International Journal of Science Education, Part B 4 (3): 260–280. doi:10.1080/21548455.2013.817026.
- Napior, D. 1972. “Nonmetric Multidimensional Techniques for Summated Ratings.” Multidimensional Scaling. 1: 157–178.
- Nunnally, J. 1978. “Psychometric Methods.” In In. New York: McGraw-Hill.
- Ones, D. S., and C. Viswesvaran. 1996. “Bandwidth–fidelity Dilemma in Personality Measurement for Personnel Selection.” Journal of Organizational Behavior 17 (6): 609–626.
- Padilla, J.-L., and J. P. Leighton. 2017. “Cognitive Interviewing and Think Aloud Methods.” In Understanding and Investigating Response Processes in Validation Research, 211–228. Springer. doi:10.1007/978-3-319-56129-5_12.
- Paustian, T. D., A. G. Briggs, R. E. Brennan, N. Boury, J. Buchner, S. Harris, R. E. A. Horak, L. E. Hughes, D. S. Katz-Amburn, and M. J. Massimelli. 2017. Development, Validation and Application of the Microbiology Concept Inventory. doi:10.1128/jmbe.v18i3.1320.
- Prokop, P., J. Fančovičová, and A. Krajčovičová. 2016. “Alternative Conceptions about micro-organisms are Influenced by Experiences with Disease in Children.” Journal of Biological Education 50 (1): 61–72. doi:10.1080/00219266.2014.1002521.
- R Core Team. 2020. R Foundation for Statistical Computing, Vienna, Austria. https://www.r-project.org
- Ran, F. A., P. D. Hsu, J. Wright, V. Agarwala, D. A. Scott, and F. Zhang. 2013. “Genome Engineering Using the CRISPR-Cas9 System.” Nature Protocols 8 (11): 2281–2308. doi:10.1038/nprot.2013.143.
- Roberts, J., L. Archer, J. DeWitt, and A. Middleton. 2018. “Popular Culture and Genetics; Friend, Foe or Something More Complex?” European Journal of Medical Genetics 62 (5): 368–375. doi:10.1016/j.ejmg.2018.12.005.
- Shepard, R. N. 1974. “Representation of Structure in Similarity Data: Problems and Prospects.” Psychometrika 39 (4): 373–421. doi:10.1007/BF02291665.
- Smith, M., W. Wood, and J. Knight. 2008. “The Genetics Concept Assessment: A New Concept Inventory for Gauging Student Understanding of Genetics.” CBE Life Sci Educ 7 (4): 422–430. doi:10.1187/cbe.08-08-0045.
- Smith, M. K., and J. K. Knight. 2012. “Using the Genetics Concept Assessment to Document Persistent Conceptual Difficulties in Undergraduate Genetics Courses.” Genetics 191 (1): 21–32. doi:10.1534/genetics.111.137810.
- Spector, P. E. 2012. “Introduction: General versus Specific Measures and the Special Case of Core Self‐evaluations.” Journal of Organizational Behavior 33 (2): 151–152. doi:10.1002/job.762.
- Stefanski, K. M., G. E. Gardner, and R. L. Seipelt-Thiemann. 2016. “Development of a Lac Operon Concept Inventory (LOCI).” CBE-Life Sciences Education 15 (2): ar24. doi:10.1187/cbe.15-07-0162.
- Stern, F., and K. Kampourakis. 2017. “Teaching for Genetics Literacy in the post-genomic Era.” Studies in Science Education 53 (2): 193–225. doi:10.1080/03057267.2017.1392731.
- Streiner, D. L. 2003. “Starting at the Beginning: An Introduction to Coefficient Alpha and Internal Consistency.” Journal of Personality Assessment 80 (1): 99–103. doi:10.1207/S15327752JPA8001_18.
- Trushell, J. M. 2004. “American Dreams of Mutants: The X-Men-” Pulp” Fiction, Science Fiction, and Superheroes.” Journal of Popular Culture 38 (1): 149. doi:10.1111/j.0022-3840.2004.00104.x.
- Wickman, H. 2016. “ggplot2: Elegant Graphics for Data Analysis.” In In, New York: Springer-Verlag. doi:10.1080/15366367.2019.1565254.