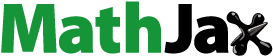
Abstract
Using nationally representative survey data from 34 countries, we assess the extent of intergenerational educational mobility for three-quarters of Africa’s population, over four decades and by gender. We employ both transition probability matrices and regression-based estimates of intergenerational elasticities and correlation coefficients. We find that the educational status of parents is a strong determinant of their children’s educational outcomes, but the strength of this link has diminished in Africa between 1960 and 1999, particularly since the 1980s. We also find that there are considerable differences between countries and by gender. Those in the Southern and Central African region and those with historical links to former British colonies experience relatively higher intergenerational mobility in education. Intergenerational educational mobility is less pronounced for daughters than for sons and mother’s education is generally more strongly associated with children’s educational attainment than fathers’ education.
Keywords:
1. Introduction
Many African countries have undergone a sustained period of reform and expansion of the public sector since the 1990s. The resulting social and economic progress has been reflected, for instance, in primary school enrolment rates in sub-Saharan Africa of 97.3 per cent in 2016, a 20 percentage points increase since 1990; an increase in the proportion of children who completed primary education from 51 to 70 per cent; an increase in adult literacy from 52.2 to 64.4 per cent over the same period, as well as an increase in life expectancy from almost 50 to 65 per cent. The population living in extreme poverty has declined by 13.2 percentage points to 41 per cent between 1990 and 2015. Typical references to the Asian growth miracle have been replaced with references to an African growth miracle (Young, Citation2012). This welcome and unprecedented performance in the continent’s history, however, begs the typical distributional question of whether the positive developments have been equally shared and have led to better outcomes and opportunities for all its citizens. Indeed, recent evidence from the World Bank suggests that income inequalities are widespread while income growth for the bottom 40 per cent of the population in Africa has not been comparable to that in other regions (Bank, Citation2018).
Who one’s parents are is perhaps the circumstance over which people have least choice; that is why the link between the economic conditions of individuals and those of their parents, referred to as intergenerational social mobility, is often considered to be a strong indicator of equality of opportunities. On one, relatively more positive hand, social mobility reflects the opportunity to move up the social ladder: if individuals from a poor background end up in a higher rank on the social ladder, that suggests that opportunities were open to them. On the other, relatively more negative hand, the existence of a system of group elites who transfer their advantageous position to their children reflects a fundamental form of inequality. These two sides of the same coin have generally characterised the political and academic debate on social mobility. For instance, it is typical to hear politicians refer extensively to social mobility and the positive aspects associated with a more socially mobile society. Academic studies mostly emphasise the limited extent of social mobility in the countries that they analyse and, therefore, the need to lessen the strong link between the outcomes of parents and the opportunities open to their children.Footnote1
The analysis of intergenerational mobility in Africa is important: what is its extent? How has it developed over time? Has socio-economic background become more or less important in shaping the opportunities of individuals? In this paper we aim to address these questions, focusing on educational mobility.
The challenge of assessing social mobility in Africa is related to the lack of data that not only report a specific outcome of interest for two linked generations of individuals but also do this at a specific and comparable point in their lives. Few studies exist that provide evidence on the extent of intergenerational mobility in Africa compared to other regions; these focus generally on specific countries such as Tanzania, Ethiopia and South Africa, which have some panel data or other limited surveys containing information on parental background, or which look at specific cities or regions within countries.Footnote2 As a result, the existing evidence does not offer a comprehensive and consistent picture of intergenerational mobility in Africa and its patterns over time.
In this paper, we aim to fill this gap by taking advantage of the increasingly available number of nationally representative cross-sectional surveys that also collect retrospective information of family members. We use innovative approaches to utilise data that report information on educational attainment. We measure the extent of transmission in educational outcomes using nationally representative surveys from 34 African countries, investigating the evolution of mobility since 1960 using birth cohorts.
Our emphasis is twofold. First, we aim to provide an overall assessment of the extent of educational mobility in the African continent and the 34 countries that we have data for, and its development over time. Second, we recognise that the countries that are part of our analysis are very different in many respects, certainly in the policy context that is relevant to a detailed understanding of educational mobility. These aspects of our analysis have two implications. One is that we cannot focus on understanding the causes of social mobility in the 34 different countries and the associated policy implications. The other is that we recognise the heterogeneity by undertaking analyses by regional groupings and historical background as well as by providing a detailed assessment of the gender dimension of intergenerational educational mobility, which is possible in the way we look at the links between mothers’ and fathers’ education at one hand and that of the sons and daughters at the other hand.
We find that intergenerational educational mobility in Africa is low. The educational status of parents remains a strong determinant of childrens’ schooling outcomes. However, there are considerable differences between countries, with countries in the Southern African sub-region having generally higher intergenerational educational mobility than countries in the other sub-regions of Eastern, Central and Western Africa. On a positive note, we also find that educational opportunities have improved for the younger cohorts compared to the older ones. The extent of the link between parents’ and children’s educational outcomes has decreased over time, particularly since the 1980s. We also find important gender differences, the intergenerational link being more persistent for daughters than for sons.
The rest of the paper proceeds as follows: in Section 2 we review the literature, focusing on educational mobility and the African context; Section 3 first describes fully the novel dataset, its coverage and variables and then introduces the two measures of intergenerational mobility we use to carry out the analysis, one based on regression coefficients and one based on transition matrices. We report the analysis and results in Section 4 before drawing conclusions in Section 5.
2. Related literature
There are three aspects that we can focus on to helpfully assess the literature on intergenerational educational mobility: (i) the approach and methodology adopted by a study; (ii) the nature of the data source and its coverage; (iii) the extent of intergenerational mobility estimated. In what follows we summarise the literature through these three lenses, fist more generally and then more specifically for the African context.
Regarding the first aspect, two main concerns of the literature have been on the estimation model adopted and on the definition and study of the transmission channels of intergenerational outcomes. In terms of modelling, and depending on the extent of information available, the literature is characterised by the use of a large range of measures, including estimates of intergenerational elasticity from regressing a child’s years of education on that of a parent.Footnote3 Others use transition probabilities matrices when the survey data collects information only on the level of education attained. Others compare percentile ranks in the educational distribution of parents and children respectively (Kotera & Seshadri, Citation2017). The measures adopted also reflect different approaches used to define intergenerational mobility (Ferreira et al., Citation2012). In terms of the channels of transmission, various studies attempt to isolate the specific causal drivers of parental background on children’s educational outcomes. Since the early 1970s, empirical studies have attempted to unpack the mechanisms through which parental background is transferred on to children’s educational outcomes, pointing out how some of these channels operate even before the birth of the child, including genetic endowments or maternal nutrition and health, while others involve parental socioeconomic status, occupation, parental time and home-learning environment, resources and human capital investment, household composition as well as institutional factors such as compulsory schooling (Baranov, Bhalotra, Biroli, & Maselko, Citation2020; Becker & Tomes, Citation1979; Behrman & Rosenzweig, Citation2002; Belzil & Hansen, Citation2003; Black, Devereux, & Salvanes, Citation2005; Ferreira et al., Citation2012; Holmlund, Lindahl, & Plug, Citation2011; Melhuish et al., Citation2008; Oreopoulos, Page, & Stevens, Citation2006). More recently, Narayan et al. (Citation2018) suggests that parents’ educational attainment level is one of the most important factors explaining the educational outcomes of children.
Moving on to the second aspect, the estimation of intergenerational mobility typically requires representative samples of panel or longitudinal data that track individuals over time. Considering the limited availability of these data in developing countries, some recent studies have employed new approaches, for instance using cross-sectional data to establish synthetic pseudo-panels, relying on the idea that synthetic panels with common characteristics produce data similar to conventional repeated panel data (Bourguignon, Goh, & Kim, Citation2004; Dang & Lanjouw, Citation2013). Another methodological aspect of the literature regards the use of educational attainment information from administrative or census data. For instance, Alesina, Hohmann, Michalopoulos, and Papaioannou (Citation2019) use census data from 26 countries to map intergenerational educational mobility in Africa. In terms of coverage, the literature has tended to focus on regions, in order to undertake comparisons between countries of the same region, or on specific countries. For instance, Hertz et al. (Citation2007) cover five regions of the World, including sub-Saharan Africa, South America, Eastern Europe, Asia and Western countries and Ferreira et al. (Citation2012) cover Latin America. Many studies have a specific country focus (Azam & Bhatt, Citation2015; Behrman & Rosenzweig, Citation2002; Behrman & Wolfe, Citation1987; Emran, Jiang, & Shilpi, Citation2021; Emran & Shilpi, Citation2015b; Emran & Sun, Citation2015a; Fan, Yi, & Zhang, Citation2015; Jalan & Murgai, Citation2008; Leigh, Citation2007; Lillard & Willis, Citation1994; Maitra & Sharma, Citation2009; Nimubona & Vencatachellum, Citation2007; Piraino, Citation2015; Tansel, Citation2015; Thomas, Citation1996).
Finally, the estimates of intergenerational mobility in these many studies vary substantially. Hertz et al. (Citation2007), which is one of the most comprehensive studies, covering 42 countries around the World and reporting mobility patterns over a period of 50 years, find large differences in IGE between countries and regions: 0.80 in Africa, 0.79 in South America, 0.69 in Asia, 0.47 for Eastern Europe and 0.54 for Western Europe and the US. They also find less intergenerational persistence amongst the former communist countries in Eastern Europe and the Nordic countries compared to the non-Nordic high-income countries.
As already mentioned, there are fewer studies of intergenerational educational mobility in Africa. reports the most recent ones, identifying the coverage, in terms of countries and time, the type of data and measure of social mobility employed and, finally, the focus on heterogeneity. These differ in terms of geographical coverage, estimates of mobility and the data used. For instance, Hertz et al. (Citation2007) find IGE to be 0.80 for the four African countries in their sample (Egypt, Ethiopia, Ghana and South Africa), while Azomahou and Yitbarek (Citation2016) find an elasticity of 0.66 using pooled data from nine countries. The more recent study by Narayan et al. (Citation2018) find higher regional educational mobility for cohorts of adults born between 1980 and 1989. Alesina et al. (Citation2019) find that intergenerational mobility is relatively high in areas that experienced colonial investment in transportation networks and missionary activities, in regions close to the coast and around the national capitals and, finally, in regions with higher urbanisation and employment in the services and manufacturing sectors once the countries achieved independence. Regarding the measurement of mobility, Alesina et al. (Citation2019) construct measures of absolute intergenerational mobility that reflect the likelihood that children acquire higher, lower or similar educational attainment than individuals in the same household belonging to the immediately previous generation who cohabitate with them (parents and extended family members, such as aunts and uncles). Narayan et al. (Citation2018) use coefficients from regressions of children’s years of education on the education of their parents and transition matrices that report the probabilities of individual moving up the educational ladder given their parents educational status. Azomahou and Yitbarek (Citation2016, Citation2021) and Hertz et al. (Citation2007) use intergenerational elasticity as well as correlation coefficients.
Table 1. Studies of educational mobility in African countries
Regarding datasets, Alesina et al. (Citation2019) use census data covering more than 14 million individuals across 26 African countries; Hertz et al. (Citation2007) employ the World Bank Living Standards Measurement Surveys (LSMS) and similar household surveys conducted by national statistical agencies; Azomahou and Yitbarek (Citation2016, Citation2021) similarly employ nationally representative household survey data on the parents of adult individuals; Narayan et al. (Citation2018) construct a global database from a comprehensive review of surveys that contain retrospective information on parental educational attainment.
This paper’s contribution is therefore threefold. First, we cover a wider range of countries in Sub-Saharan Africa than has been possible to-date: our representative surveys for 34 countries cover three-quarters of the African population, as opposed to four and 9 countries in, for instance, the ground breaking analyses of (Hertz et al., Citation2007) and Azomahou and Yitbarek (Citation2021), therefore allowing us to provide robust estimates of intergenerational educational mobility for the region as whole and for sub-regions and countries with Sub-Saharan Africa. Secondly, for this sample, we can study the evolution over time, more precisely since the 1960. Thirdly and, importantly, we assess the heterogeneity in terms of gender differences and country’s background. In fact, we distinguish between sons and daughters but also between mothers and fathers and we look at regional groupings and countries’ historical and colonial background. While previous analyses mostly consider mobility in the context of parental educational background, our paper also considers the country’s historical background by ‘mapping’ the extent of intergenerational persistence by historical or colonial background, also in relation to the country’s main official language (i.e. Anglophone, Francophone etc.). This sub-regional classification is important in social mobility estimation, given that the historical contexts, particularly in relation to colonisation, are associated to the educational systems present in different countries. Therefore, mobility analysis by historical background is important to unpack differences in the extent of intergenerational mobility. Consequently, for all this reasons, even in the context of an emerging literature on social mobility in Africa, we believe our analysis contributes to fill the gaps and offer an improved understanding of the extent of the intergenerational links in a Continent that has, to date, received far less attention in analyses of social mobility than other regions of the world.
3. Data and measurement
The measurement of social mobility is not uncontroversial: one reason for the difficulties in measuring the extent of intergenerational mobility is that we often lack detailed information on parental conditions when individuals are growing up. In addition, the link between the generations, by definition, relates to a long time: typically, more than twenty years will have passed by the time we know the link between the parents’ circumstances while an individual was a child and their circumstances in middle-age. In what follows, we describe how these difficulties can be overcome. We first introduce the dataset and then we describe the two measures we use to document the extent of intergenerational educational mobility in sub-Saharan Africa.
3.1. The data
The analysis covers 34 African countries. shows the list of the countries’ household surveys, which are the backbone of our analysis, the year, the country’s population, the type of information available on educational attainment of the respondents and the total number of households and individuals covered. All these surveys are nationally representative and contain information on the educational attainment of individual household members. The data used for the analysis are therefore from a range of Living Standard Measurement type welfare Surveys (LSMS), including Household Budget Surveys, Income and Expenditure Surveys, Household Income and Expenditure Surveys and LSMS-integrated Survey on Agriculture. These are generally multi-topic welfare surveys, designed by the respective countries national statistical agencies, together with development partners, in order to improve the understanding of the links between various social and economic activities; they contain individual level information on the adult population, including their demographic characteristics and educational attainment variable, which are all harmonised in the Survey-based Harmonised Indicator Programme (SHIP) database.Footnote4 Of the 34 countries selected, 10 have educational attainment information for both co-residents and non residents, which represent about 75 per cent of the estimated total population of about 900 million. By pooling all the surveys, we can estimate intergenerational mobility in Africa. This total sample consists of more than 1.8million individuals and 310,502 households.
Table 2. Household Surveys used in the analysis
The surveys selected contain information on the actual grades of schooling completed.Footnote5 Using the 2011 International Standard Classification of Education (ISCED), we can derive a quasi-continuous variable rather than using the levels of schooling completed, thus avoiding the discontinuities in the schooling distribution that are possible when relying on categorical variables (Azam & Bhatt, Citation2015).Footnote6 This is collected for each family members in the sample. For parents’ educational attainment, we use the average years of schooling of both parents. Most studies use father’s education as a proxy for parent’s education, some because of their interest in father-son pairs only and others due to limited information on the education of mothers. Given that both the father’s and mother’s education are available to us, we use the average years of schooling for both parents as proxy for parents’ educational attainment in the general regression and the individual mother’s and father’s education in the gender specific analysis.
Having complete information on an individual’s relationship to the household head, the data allow for the matching of parent-child pairs. Those aged 17–56 are matched with their parents, who are either co-residents or non-residents.Footnote7 Family members who have moved out, particularly those who have married and established their own families, are regarded as non-residents. The sample, therefore, represents the co-resident and non-resident adult population aged more than 17 years, who are currently not in school or who have completed education. The data also allow matching of the current household head with their parents who are residing with them at the time of the survey, which means that co-resident parents of the household head are part of the sample: the household head would be both a child and a parent.Footnote8 This is done also to reduce downward bias of the results when educational attainment is associated only with parent-child pairs and grandparents are excluded from the household roaster.Footnote9
To show the evolution of intergenerational mobility over time, we derive estimates for each of the four 10-year birth cohorts of the 1960s, the 1970s, the 1980s and the 1990s. It is possible to construct birth-cohorts for the individuals’ reported age in the household roster. A birth cohort refers to a group of individuals who are around the same age and who possibly share a set of common characteristics and experiences. For instance, the 1960s cohort could be identified with the origin and development of colonial independence in Africa, which started in 1957 in Ghana. The 1970s birth-cohort could be identified with the period of military dictatorship and civil wars in Africa. The 1980s birth-cohort could be identified with a decade of economic and social difficulties, widespread famine, particularly in East Africa, and the start of AIDS pandemic. Finally, the 1990s birth-cohort could be identified with the release of Mandela from prison, the peaceful transition and independence in South Africa and the exponential increase in enrolment rates in schooling throughout the continent.Footnote10
Although the pooling of cross-sectional data in estimating educational status across generations is useful and relevant to analyse mobility at a regional level, we also undertake analysis for each specific country. This is important because of the possible underlying differences in educational systems, contexts and policies that might affect mobility outcomes. Although it is impossible, in this paper, to assess the policy context of all the countries, to better understand the heterogeneity we divide the sample into four relevant sub-regions as defined by the African Union, including Central, Eastern, Southern and Western Africa, which also correspond to the regional economic communities with a mandate for building economic integration in Africa. Moreover, we also look at the historical background by dividing the sample according to whether the country was one of the colonies of the British, French or Portuguese empires or whether it was mostly an Arabic speaking country.
shows the sample distribution based on the relationship to the head of household. There are more than 260,000 household heads in the sample, 220,000 children and 6,840 parents of the household head.Footnote11 shows the average years of schooling and average age for the child and the parent by birth cohorts: educational attainment has clearly been increasing; children have, on average, higher educational attainment than their parents in all birth cohorts except for the older ones of 1960–1969, when daughter’s experienced lower years of education. Sons report higher average years of education than daughters until the 1990 while the 1990s saw daughter’s years of education taking over those of sons.
Table 3. Sample distribution: relationship to household head
Table 4. Completed years of schooling and age by birth cohorts at time of survey
3.2. The measurement of intergenerational educational mobility
We employ two approaches (and three measures) to assess the extent to which the educational attainment of individuals in one generation is linked to the educational attainment of their parents: one is based on the regression of children’s educational attainment on that of parents, which results in estimates of intergenerational elasticity and associated correlation coefficients; the other is based on transition probability matrices.
Starting with the regression approach, the relationship between the educational attainment of a child and that of the parent is estimated by the following equationsFootnote12:
S denotes the educational attainment of child c and parents p, Xi are country fixed effects introduced in equation (2) for the regional estimates.Footnote13 The remaining heterogeneity is left in the residuals .
is the standard measure of the intergenerational regression coefficient (IGRC). The extent of integernerational mobility is measured by
, higher values of
resulting in greater links between the educational attainment of the parents and that of a child and, therefore, lower educational mobility. An alternative method relies on the correlation coefficient
between the parents and a child’s educational outcomes, while adjusting for the differences in educational variance between the two generations, i.e. a measure of standardised persistence, given by:
EquationEquation (3)(3)
(3) estimates the educational mobility conditional on the overall dispersion of educational attainment for each generation: as the correlation
between parent and child’s outcomes increases, the intergenerational mobility measure decreases.Footnote14
Sample selection bias in estimates of IGRC have been extensively documented. In particular, downward bias might result when relying on information from co-resident members only in identifying children’s or parents’ educational attainment (Azam & Bhatt, Citation2015; Emran, Greene, & Shilpi, Citation2018; Emran & Shilpi, Citation2019). Our data covers information for most of the children even when they are not residing with the family at the time of the survey as the respondent, mostly the head of the household, is asked about the educational attainment of all household members, including children that are away for work or at a boarding school. However, some non residents, for instance children who get married and form another household, might not be captured: if those who leave are systematically different from those who stay in the household and are captured by the survey, then the estimates from co-residents are biased. Due to the exclusion of non-resident parents who are not captured at the time of the survey, the younger adults in their 20s could therefore be over-represented in the sample, while the other adults be under-represented. Emran et al. (Citation2018), for instance, show that, in the sample of 13–60 years of age in Bangladesh, the average downward bias in IGRC estimates is 29.7 per cent. We attempt to minimise the effect of downward bias also by making use of the educational attainment of the parents of household heads, who are the co-resident grand parents at the time of the interviews. Moreover, we undertake sensitivity checks to determine the extent of the bias across survey samples and associated with co-residency. In this respect, we compare the estimates for the surveys that have co-resident information only with those that include both co-residents and non resident educational attainment information.Footnote15 Moreover, we also consider just the subset of 10 countries with a non-resident sample and compare estimates on the co-resident only portion of this sample with co-resident and non-resident sample so that there are no other changes in the sample composition.Footnote16
Another source of bias in educational mobility might arise from the so-called ceiling effect: children whose parents have already attained the highest possible educational attainment would not possibly attain a higher educational attainment themselves and, therefore, cannot be shown to be moving up the educational ladder. This is particularly relevant in the case of estimation of educational mobility through transition probability matrices, but less so in the case of IGRCs, where educational attainment is measured in years of schooling. Therefore, the IGRC estimates we present in the following section includes parents with any level of education.
As mentioned above, in addition to IGRC and correlation coefficients, we estimate the extent of intergenerational educational mobility through transition probability matrices, which allow us to estimate mobility by determining the position of the child’s educational attainment conditional on the parent’s position. Appendix B (available in Supplementary Materials) discusses the method and results associated with the use of transition matrices.
4. Analysis and results
We start by reporting the results of the analysis of intergenerational educational mobility from the regression analysis overall and for the different countries and then move on to provide the results of the analysis of heterogeneity by looking at country groupings and historical background before we conclude with the analysis by gender.
4.1. Intergenerational educational mobility overall and by country
shows the estimates for the whole region, based on Equationequation (2)(2)
(2) for the IGRC and Equationequation (3)
(3)
(3) for the correlation coefficient. We also report estimates for the whole period as well as for the four birth cohorts.
Table 5. Relative educational mobility: regression estimates, 1960–1999
The overall regional regression based on the pooled data results in an estimated IGRC of 0.75. This result is comparable to that of Hertz et al. (Citation2007), who find an IGRC of 0.80 for four African countries, including Egypt, Ethiopia, Ghana and South Africa, and higher here than Azomahou and Yitbarek (Citation2016), who find an estimate of 0.66 for nine African countries.Footnote17 These estimates are also consistent with those of Narayan et al. (Citation2018), who report that, across the World, the prospects of relative educational mobility are lowest for children in Africa.
The results also show that parental educational attainment has a significant link to children’s educational attainment for all four birth cohorts and that the strength of this link has diminished over the four decades since 1960. The coefficient was 0.93 for the 1960s cohort and 0.52 for the 1990s cohort. This could be a reflection of the substantial expansion in school and higher education enrolments across Africa: between 1970 and 2013, for instance, the region experienced the fastest growth in tertiary gross enrolment in the world, 4.3 per cent annually compared with 2.8 per cent globally (Darvas, Gao, Shen, & Bawany, Citation2017). also shows the intergenerational correlation: at 0.73 for the whole period, it is higher than the 0.51 reported by Azomahou and Yitbarek (Citation2016) and the 0.36 by Hertz et al. (Citation2007). It is also higher than that for Asia (0.39), Western Europe and United States (0.44) and Eastern Europe (0.46). The comparison between IGRC and correlation coefficients show that the key results on relative educational mobility in the sample are robust. The correlation coefficient has also decreased over time, although the extent of the reduction is different from that reported for the IGRC coefficient. From Equationequations 2(2)
(2) and Equation3
(3)
(3) , we know that the difference between the two measures is represented by the ratio of the standard deviations of schooling of children and parents. Therefore, the extent of the difference between the two measures and, more importantly, the extent of the differences in the way they change over time, depends on the extent to which average years of schooling increases, which we have reported in . Azomahou and Yitbarek (Citation2016) finds a similar result and suggests this is due to the dispersion of the years of schooling between the two generations. Other studies with similar findings for developing countries are those of Azam and Bhatt (Citation2015) and Daude and Robano (Citation2015) for India and Latin America respectively.
graphically shows the evolution of the IGRC and the correlation coefficients over time and also the IGRC by gender for the latest two cohorts.
shows the country-level estimates of IGRC, as per Equationequation (1)(1)
(1) . The 34 countries in our sample experience different levels of educational mobility, ranging from 0.45 in South Africa to 0.96 in Cote d’Ivoire, therefore reflecting substantial variations in the level of persistence in educational attainment between parents and children for the whole period. There is relatively higher mobility in South Africa, Cape Verde, Mauritius and Sudan and relatively lower in Cote d’Ivoire, Benin and Ethiopia.
These results are in line with those of Hertz et al. (Citation2007) for developing countries, who also conclude that the large differences between countries are informative. This could be a reflection of differences in underlying educational systems across countries as well as evidence of other differences in context and policies that might affect educational attainment more generally. For instance, some countries have free primary education while others do not.
However, cross-country comparisons based on relative measures of mobility such as the IGRC could be misleading if not integrated with measures of the intercept term from the regressions of the educational attainment of the child on that of the parent. More consistent conclusions on the relative mobility in different countries can be obtained by considering their differences in both the long-term variance, determined by the slope coefficient IGRC, and the steady-state means, determined by the intercept. This allows us to be more specific and precise in assessing whether children living in a country with relatively high mobility (South Africa, with IGRC of 0.44) have educational advantage over children living in a country with relatively low educational mobility (Cote D’Ivoire, with IGRC of 0.98). However, continuing with the example of the two countries at the opposite extremes of , the intercept term for South Africa is higher than that for Cote d’Ivoire, implying that the expected educational attainment for children in Cote d’Ivoire is unambiguously lower, conditional on parental education, across the entire range of parent’s education distribution. Given South Africa’s higher intercept term, it may be that children from less educated households fare better in Cote d’Ivoire than in South Africa but then, after a certain level of parental education is reached, the relative advantage shifts in favour of South Africa’s children.Footnote18 reports the value of the intercept terms alongside the IGRC. From considering the intercept terms alongside the IGRC, we can see that the apparently unambiguous ranking presented in needs to be taken with caution, as there are differences not only in the long-term variance but also in the steady state mean.
In order to explore countries’ differences further, reports the estimates for the IGRC and the correlation coefficient at the sub-regional level. It confirms the large spatial differences in educational mobility in Sub-Saharan Africa. The countries in each of the sub-regions, as defined by the African Union, form the same regional economic communities.Footnote19
Table 6. Relative educational mobility: regression estimates, sub-regions
We also explore differences by looking at the historical background, more specifically the colonial set up and the main official language spoken in the country. This classification results in former British colonies (Anglophone), former French colonies (Francophone) and former Portuguese colonies (Portuguese) and Arabic speaking countries.Footnote20 reports the corresponding IGRC and correlation coefficient estimates. Former French and Portuguese colonies are characterised by relatively lower educational mobility than former British colonies and Arabic speaking countries.
Table 7. Relative educational mobility: regression estimates, historical background
4.2. Intergenerational educational mobility by gender
shows the results of the regression for sons and daughters. The intergenerational persistence was higher between sons’ and parents’ years of schooling than between daughters’ and parents’ years of schooling, particularly so in the 1980–1989 cohort, meaning that mobility is more pronounced for daughters than for sons and that it has improved over the two decades. The Table also reports a declining trend in the correlation coefficients over the two decades for both sons and daughters.
Table 8. Relative educational mobility by gender: regression estimates, 1980–1999
We then extend the regression-based analysis of educational mobility by distinguishing between the impact of mothers and that of fathers on both sons and daughters, for the two most recent birth cohorts of 1980–1989 and 1990–1999. The results are shown in and suggest the existence of a significant influence of father’s schooling on the educational outcomes of both sons and daughters. Further comparison shows that there is a higher intergenerational persistence for sons than for daughters. For the 1990–1999 birth cohort, the IGRC for father-son pair is 0.506, while that of father-daughter is 0.407. However, the reduction of intergenerational persistence between the two birth-cohorts has been more pronounced for sons and less for daughters. Just like in the IGRC estimates, the correlation coefficient is higher for sons than for daughters. It decreased by 0.435 for sons and by 0.024 for daughter over the two decades under consideration.
Table 9. Relative educational mobility by gender and mothers and fathers: regression estimates, 1980–1999
The bottom panel of shows the same analysis for the relationship between children and mothers. That relations appear to be stronger between mothers and daughters than between mothers and sons, in both cohorts. This is confirmed by the values of the correlation coefficients reported in the same table.
Appendix B (available in Supplementary Materials) shows the extent of intergenerational educational mobility measured with transition probability matrices. To summarise the gender analysis therein, we find that the strength of the link between the educational attainment of parents and daughters has diminished over time, particularly so for the link between mothers and daughters, which started from a relatively stronger position and which remains, overall, stronger than that between fathers and daughters. A comparison between sons and daughters reveals that the strength of the link between son-father pairs is greater than that between daughter-father pairs in both cohorts. The opposite is true for the strength of the link between son-mother pairs and daughter-mother pairs, the latter being stronger than the former in both cohorts: sons are more likely than daughters to achieve the same educational attainment as that of their fathers, while daughters are more likely than sons to achieve the same educational attainment as that of their mothers; this has not changed over the two decades.
4.3. Sample and co-residency bias
As pointed out earlier, measurement error and a co-resident sample make comparison across groups, cohorts, and countries difficult even when the focus is on relative mobility. For example, Emran et al. (Citation2018) find that the cross-country ranking in educational persistence can be reversed when using co-resident samples compared to the ranking in the full samples. Sample truncation due to co-residency restrictions is likely to affect different genders and cohorts differently. In many developing countries, daughters leave the natal house after marriage, but sons continue to live with the parents after marriage. The extent of sample truncation is thus likely to be more severe for the daughters. Similarly, for cohort-based analysis, it is important to recognise that the co-residency rates are likely to decline for the younger cohorts as the extended family living arrangements become less prevalent and geographic mobility increases, thus making the estimates of relative mobility spuriously lower for the younger cohorts.
First, in order to address possible bias across survey samples, we compare IGRC and correlation coefficients for three samples: one made of 24 out of the 34 countries, which contains information on educational attainment only for co-residents (first column in ); one made of the remaining 10 countries, which contains information on both co-residents and no-residents (second column) and the third made of the pooled sample comprising all 34 countries (third column).Footnote21
Table 10. Differences in estimates across data samples
The results reveal that the IGRC estimates are biased downward, by about 21 and 33 per cent, when using the co-resident sample only compared to the other two approaches. Similarly, the estimates for the inter generational correlation are also biased downward by about 24 and 31 per cent respectively. These estimates are comparable to those of Emran et al. (Citation2018), who report downward bias in the intergenerational elasticity estimates of almost 30 per cent. Moreover, it is mostly children who still live in the household who are captured while those who have left are excluded. shows that the bias is relatively more pronounced for sons than daughters, consistent with them being more likely than daughters to move away from the family to attend boarding school or university, or to work away from home for long periods of time and, therefore, not being available at the time of the interview.
Figure 4. IGRC and correlation coefficient: daughters and sons. (a). IGRC estimates. (b) Correlation coefficients estimates
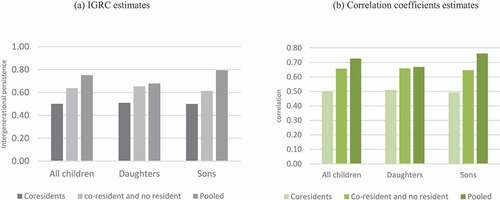
Moving on to investigate co-residency bias, we unpack the estimates provided in the second column of by estimating the coefficients for two samples from the same surveys: the full sample containing information of both resident and non-resident members and a sub-sample containing information only of co-resident family members identified at the time of the survey. The percentage difference in the estimates between the two reveals the co-residency bias, associated with relying only on a sample of co-resident members only. shows that the intergenerational persistence in the co-resident sample is biased downward by about 11 per cent, when compared to the full sample, while the intergenerational correlation is biased downward by about 7 per cent. The lower estimates could be due to the fact that the years of education are truncated as a result of relying mostly on information from relatively younger cohorts of respondents to the survey. In addition, there is a selection bias problem arising from information that is mostly from children who still live in the household and excludes those who have left (Emran et al., Citation2018; Emran & Shilpi, Citation2019). In their global study, Narayan et al. (Citation2018) find that co-residency bias is not significant across samples based on different member residency status.
Table 11. Co-residency bias
5. Concluding discussion
Using nationally representative survey data, we have assessed the extent of intergenerational educational mobility for 34 countries and three-quarters of Africa’s population.
We have found that the educational status of parents is a strong determinant of their childrens’ educational outcomes: intergenerational educational mobility is low in Africa compared to the rest of the world. The results for the overall sample reports is higher than those reported in most regions, indicating that additional years of parental schooling, on average, increases children’s years schooling by 0.75. This is comparable with estimates by Hertz et al. (Citation2007) but higher than estimate of by Azomahou and Yitbarek (Citation2016) for nine African countries (Comoros, Ghana, Guinea, Madagascar, Malawi, Nigeria, Rwanda, Tanzania, and Uganda).Footnote22 It is also comparable to those of other developing countries in Latin America and Asia, also reported by Hertz et al. (Citation2007). The results are also consistent with those of Narayan et al. (Citation2018) who report that across the world’s six regions of Middle East and North Africa, East Asia and Pacific, Latin America and Caribbean, East Asia and Sub-Saharan Africa, the prospects of relative mobility are lowest among children in Africa.
However, there are considerable differences between countries: South Africa, Cape Verde and Mauritius experience, for instance, relatively higher educational mobility than Cote d’Ivoire, Benin and Ethiopia. More generally, at the sub-regional level, countries in Southern Africa and, to a lesser extent in Central Africa, experience higher mobility than countries in the Eastern and Western Africa sub regions. We also explored the role of historical background in potentially explaining the differences between countries, finding that the former French and Portuguese colonies experience lower educational mobility than the former British colonies and Arabic speaking countries. This is consistent with other research, which, even though more limited in scope, found that former British colonies have higher levels of intergenerational mobility compared to French colonies (Bossuroy and Cogneau (Citation2013). More recently, Alesina et al. (Citation2019) found that proximity to infrastructure established during colonial times, such as railroads and roads, are significant factors positively related to the extent of intergenerational mobility in Africa.
We also found important gender differences. Our results suggest that the intergenerational link is overall more persistent for daughters than for sons. When we disentangled the paternal from the maternal link, we found that the link between mother-daughter pairs is stronger than that between mother-son pairs, while the opposite is true in the case of father-son and father-daughter pairs, under both the 1980s and the 1990s, the two most recent decades we have considered for the gender analysis. The IGRC estimates suggest that the association between mothers’ educational attainment and that of children (whether sons or daughters) is stronger than that between fathers’ educational attainment and that of children. Using four birth cohorts, from 1960 up to 1999, we found that there has been a significant improvement over time with a lessening of the intergenerational links in educational outcomes. The values for the two measures of educational mobility used in the study, the intergenerational elasticity and the intergenerational correlation coefficient, decreased for each cohort compared to the preceding ones, but particularly so for the 1980s and 1990s cohorts. The sources of this extremely encouraging progress over the period are not clear, considering that we do not aim here to assess the impact of any particular policy. However, we could speculate that it could be a reflection of the substantial improvements in school and higher education enrolment experienced in many African countries, particularly since the 1980s. This is certainly a good platform to build on to ensure increasing investment in education and more equitable and efficient use of the existing educational resources, aiming to target them to relatively poorer areas.
We think this empirical analysis provides important insights on the extent of intergenerational educational mobility in Africa. However, it is important to outline the limitations. First, although the survey data we have used are nationally representative and contain relevant information, the analysis is still based on cross-sectional data rather than the ideal longitudinal data used in estimates of mobility in various developed countries. Furthermore, we do not attempt any causal analysis nor assessment of determining factors: the aim of this paper is to fill in a more basic, nonetheless extremely important, gap in the evidence on intergenerational educational mobility in Africa, by providing estimates of both the IGRC and the correlation coefficients, as well as transition matrices when possible, for a substantially larger sample of countries and population in Africa. Indeed, further research could rely on different measures of intergenerational mobility to those used here, including rank-rank methods that allow an analysis of differences across the distributions. Given the nature of the dataset, further research could also look at multigenerational mobility by aiming to assess the link between the educational outcomes of three generations.
Supplemental Material
Download PDF (77.9 KB)Acknowledgements
We are very grateful for the comments from anonymous referees. Data available from the authors upon request.
Disclosure statement
No potential conflict of interest was reported by the author(s).
Supplementary Materials
Supplementary Materials are available for this article which can be accessed via the online version of this journal available at https://doi.org/10.1080/00220388.2021.2008366.
Notes
1. The relatively more positive political jargon is explicit in references to ‘realisation of own potential’ or to ‘successive generations getting more and better jobs’ than previous generations. In the Uk, the major political parties refer directly to social mobility in their political manifestos (Payne, Citation2012).
2. We review the evidence in Section 2 below.
3. In this section, we refer to intergenerational elasticity as either IGE or IGRC. The former, often associated with measurement of income mobility, is the slope parameter of a double-log specification of the relationship between the income of parents and that of children; the latter, associated with studies of educational mobility, is the slope paramater of a level-level specification of the relationship between the education of parents and that of children, often motivated by the fact that a large proportion of parents have zero years of schooling. Our analysis, from section 3 onward, uses IGRC. See Emran and Shilpi (Citation2019) for a detailed discussion. We thank an anonymous referee for this important clarification.
4. The analysis uses SHIP, a database which harmonises a set of key variables from nationally representative surveys in the World Bank African region. This ensures standardisation and comparability of the educational attainment variables (i.e. levels and years of education completed). A full description is available in the World Bank Microdata catalogue and the Datilibweb Stata module to access microdata.
5. For example, the completed years of education is 0 for pre-school, 1 for grade 1, 2 for grade 2 and so on. In harmonising the education variable, the SHIP database assumes that children less than 5 years old have not attended primary school and may have only attended pre-school programmes depending on specific country definitions. Although few exceptions may exists when 4-5 year-olds are in primary school, almost all have not completed grade 1 and are therefore recorded as missing.
6. ISCED provides a framework for organising educational programmmes and related qualifications by educational levels and duration, see http://uis.unesco.org/en/isced-mappingsat UNESCO.
7. Individuals are selected if they are aged 17+ and have completed education, if they are not enrolled at school at the time of the survey and have been out of school over the previous 12 months. This is in line with previous research including Narayan et al. (Citation2018) and Azomahou and Yitbarek (Citation2016). Generally, students are not out of school for more than 12 months.
8. See Appendix A (available in Supplementary Materials) for details on data and definitions in relation to the household and the matching of parent-child pairs.
9. The issue of co-residence bias is addressed in the following section 3.2.
10. Estimates of intergenerational mobility for older cohorts are arguably less precise when compared to estimates for younger cohorts. Older respondents, interviewed in 2018 for example, may not provide representative samples, especially if survival rates vary with an individual’s level of education.
11. Appendix A provides details on the sample selection.
12. For the theoretical underpinnings of the specifications, see Becker and Tomes (Citation1979) and Solon (Citation2014).
13. Country fixed-effects are only applied to the pooled data set in order to estimate regional elasticity. EquationEquation (1)(1)
(1) is used for country estimates.
14. ρ, therefore, provides a measure of rank mobility between the two generations (Blanden, Gregg, and Macmillan (Citation2013).
15. This sensitivity analysis makes use of country surveys from Kenya (2015), Liberia (2016), the Gambia (2015), Ghana (2016) and Nigeria (2016).
16. See Section 4.3. for details.
17. Including Comoros, Ghana, Guinea, Madagascar, Malawi, Nigeria, Rwanda, Tanzania and Uganda.
18. This is due to the expectation functions intersecting each other at that ‘certain’ level of parental education, which is unlikely in our case, given that the substantial difference in the intercept terms between South Africa and Cote d’Ivoire are likely to result in one country’s expectation function
always above the other. From the results in , the case of intersecting functions might be more likely, for instance, in the case of South Africa and Botswana.
19. Western African countries include: Mauritania, Senegal, Guinea, Liberia, Cote d’Ivoire, Ghana, Togo, Benin, Burkina Faso, Nigeria and Niger. Central Africa includes Cameroon, Rwanda and Central African Republic. Eastern Africa sub-region includes Sudan, South Sudan, Ethiopia, Uganda, Kenya, Tanzania and Madagascar. South African sub-region include Angola, Zambia, Mozambique, Botswana, Swaziland and South Africa.
20. Anglophone countries are: Liberia, Ghana, Nigeria, South Sudan, Ethiopia, Uganda, Kenya, Tanzania, Zambia, Botswana, Swaziland and South Africa. Francophone countries include Senegal, Guinea, Cote d’Ivoire, Togo, Benin, Burkina Faso, Niger, Cameroon, CAF, Rwanda and Madagascar. Portuguese countries include Angola and Mozambique and the Arabic countries include Mauritania and Sudan.
21. See for detailed information.
22. Azomahou and Yitbarek (Citation2016) for Malawi reports slightly different estimate from those in our findings, despite employing the same survey and data and a similar methodological approach. However, the difference emerges from the different samples employed, which were determined so as to be comparable with other countries in the same paper. In their sample selection, they consider five years birth cohorts between 1942–1991 and individuals aged between 20 and 69. In our analysis, we select the sample of individuals aged 17 years and above and born between 1960 and 1999. Moreover, in our paper if, in the household roster, the grandfather or grandmother information is available, we consider the household head as a ‘child’, either a son or a daughter.
References
- Alesina, A., Hohmann, S., Michalopoulos, S., & Papaioannou, E. 2019. Intergenerational mobility in Africa (NBER Working Papers 25534). National Bureau of Economic Research, Inc.
- Azam, M., & Bhatt, V. (2015). Like father, like son: Intergenerational educational mobility in India. Demography, 52(6), 1929–1959.
- Azomahou, T. T., & Yitbarek, E. A. (2016). Intergenerational education mobility in Africa: Has progress been inclusive? Washington Dc: The World Bank.
- Azomahou, T. T., & Yitbarek, E. (2021). Intergenerational mobility in education: Is Africa different? Contemporary Economic Policy, 39(3), 503–523.
- Bank, T. W. (2018). Poverty and shared prosperity 2018: Piecing together the poverty puzzle. Washington DC: The World Bank.
- Baranov, V., Bhalotra, S., Biroli, P., & Maselko, J. (2020). Maternal depression, women’s empowerment, and parental investment: Evidence from a randomized controlled trial. American Economic Review, 110(3), 824–859.
- Becker, G. S., & Tomes, N. (1979). An equilibrium theory of the distribution of income and intergenerational mobility. Journal of Political Economy, 87(6), 1153–1189.
- Behrman, J. R., & Rosenzweig, M. R. (2002). Does increasing women’s schooling raise the schooling of the next generation? American Economic Review, 92(1), 323–334.
- Behrman, J. R., & Wolfe, B. L. (1987). Investments in schooling in two generations in pre-revolutionary Nicaragua: The roles of family background and school supply. Journal of Development Economics, 27(1–2), 395–419.
- Belzil, C., & Hansen, J. (2003). Structural estimates of the intergenerational education correlation. Journal of Applied Econometrics, 18(6), 679–696.
- Black, S. E., Devereux, P. J., & Salvanes, K. G. (2005). The more the merrier? The effect of family size and birth order on children’s education. The Quarterly Journal of Economics, 120(2), 669–700.
- Blanden, J., Gregg, P., & Macmillan, L. (2013). Intergenerational persistence in income and social class: The effect of within-group inequality. Journal of the Royal Statistical Society: Series A (Statistics in Society), 176(2), 541–563.
- Bossuroy, T., & Cogneau, D. (2013). Social mobility in five African countries. Review of Income and Wealth, 59, 84–110.
- Bourguignon, F., Goh, C.-C., & Kim, D. I. (2004). Estimating individual vulnerability to poverty with pseudo-panel data. Washington DC: The World Bank.
- Dang, H.-A., & Lanjouw, P. (2013). Measuring poverty dynamics with synthetic panels based on cross-sections. Washington DC: The World Bank.
- Darvas, P., Gao, S., Shen, Y., & Bawany, B. (2017). Sharing higher education’s promise beyond the Few in Sub-Saharan Africa. Washington DC: The World Bank.
- Daude, C., & Robano, V. (2015). On intergenerational (im) mobility in Latin America. Latin American Economic Review, 24(1). doi:https://doi.org/10.1007/s40503-015-0030-x.
- Emran, M. S., Jiang, H., & Shilpi, F. (2021). Is gender destiny? Gender bias and intergenerational educational mobility in India. GLO Discussion paper, 807. Global Labour Organization (GLO), Essen.
- Emran, M. S., & Shilpi, F. (2015b). Gender, geography, and generations: Intergenerational educational mobility in post-reform India. World Development, 72, 362–380.
- Emran, M. S., & Shilpi, F. 2019. Economic approach to intergenerational mobility: Measures, methods, and challenges in developing countries (Working Paper 2019/98). UNU–WIDER.
- Emran, S., Greene, W. H., & Shilpi, F. (2018). When measure matters: Coresident sample selection bias in estimating intergenerational mobility in developing countries. Journal of Human Resources, 53(3), 589–607.
- Emran, S., & Sun, Y. (2015a). Magical transition? Intergenerational educational and occupational mobility in rural China: 1988–2002. Washington DC: The World Bank.
- Fan, Y., Yi, J., & Zhang, J. 2015. The great gatsby curve in China: Cross-sectional inequality and intergenerational mobility. Technical report, Working Paper, CUHK, Hongkong.
- Ferreira, F. H., Messina, J., Rigolini, J., López-Calva, L.-F., Lugo, M. A., & Vakis, R. (2012). Economic mobility and the rise of the Latin American middle class. Washington DC: The World Bank.
- Hertz, T., Jayasundera, T., Piraino, P., Selcuk, S., Smith, N., & Verashchagina, A. (2007). The inheritance of educational inequality: International comparisons and fifty-year trends. The BE Journal of Economic Analysis & Policy, 7, 2.
- Holmlund, H., Lindahl, M., & Plug, E. (2011). The causal effect of parents’ schooling on children’s schooling: A comparison of estimation methods. Journal of Economic Literature, 49(3), 615–651.
- Jalan, J., & Murgai, R. (2008). Intergenerational mobility in education in India. Paper presented in the 5th annual conference on economic growth and development at the ISI, Delhi. India Statistical Institute (ISI), Delhi.
- Kotera, T., & Seshadri, A. (2017). Educational policy and intergenerational mobility. Review of Economic Dynamics, 25, 187–207.
- Leigh, A. (2007). Intergenerational mobility in Australia. The BE Journal of Economic Analysis & Policy, 7(2), 1–28.
- Lillard, L. A., & Willis, R. J. (1994). Intergenerational educational mobility: Effects of family and state in Malaysia. Journal of Human Resources, 29(4), 1126–1166.
- Maitra, P., & Sharma, A. 2009. Parents and children: Education across generations in India. In Paper presented in the 5th annual conference on economic growth and development, ISI Delhi. India Statistical Institute (ISI), Delhi.
- Melhuish, E. C., Phan, M. B., Sylva, K., Sammons, P., Siraj-Blatchford, I., & Taggart, B. (2008). Effects of the home learning environment and preschool center experience upon literacy and numeracy development in early primary school. Journal of Social Issues, 64(1), 95–114.
- Narayan, A., Van der Weide, R., Cojocaru, A., Lakner, C., Redaelli, S., Mahler, D. G., … Thewissen, S. (2018). Fair progress?: Economic mobility across generations around the world. Washington DC: The World Bank.
- Nimubona, A.-D., & Vencatachellum, D. (2007). Intergenerational education mobility of black and white South Africans. Journal of Population Economics, 20(1), 149–182.
- Oreopoulos, P., Page, M. E., & Stevens, A. H. (2006). The intergenerational effects of compulsory schooling. Journal of Labor Economics, 24(4), 729–760.
- Payne, G. (2012). A new social mobility? the political redefinition of a sociological problem. Contemporary Social Science, 7(1), 55–71.
- Piraino, P. (2015). Intergenerational earnings mobility and equality of opportunity in South Africa. World Development, 67, 396–405.
- Solon, G. (2014). Theoretical models of inequality transmission across multiple generations. Research in Social Stratification and Mobility, 35, 13–18.
- Tansel, A. 2015. Intergenerational educational mobility in Turkey. Available at SSRN 2705453.
- Thomas, D. (1996). Education across generations in South Africa. American Economic Review, 86(2), 330–334.
- Young, A. (2012). The African growth miracle. Journal of Political Economy, 120(4), 696–739.