Abstract
Explainer videos (EV) can enhance learning by increasing motivation and illustrating complex content, yet they may also cause cognitive overload or reduced cognitive activation. Addressing these challenges requires well-designed instructional strategies. Our study hypothesized that cooperative problem-solving before watching an EV improves learning outcomes. We tested this with 58 undergraduates examining the topic of light and shadow. The findings revealed that students who engaged in problem-solving prior to viewing the EV demonstrated superior knowledge transfer compared to those who watched the EV first. This suggests that starting with cooperative problem-solving can effectively prepare learners for EV, fostering deeper understanding and successful application of new knowledge.
Introduction
Video has become an essential alternative for presenting new learning content in education. Teachers use explainer videos (EVs) in their lectures, for example, to implement online teaching formats or to create didactic variety away from purely text-based courses (Handke, Citation2020; Mayer et al., Citation2020; Persike, Citation2020). In addition, learners report using EVs to prepare for exams, learn complicated topics, or as a source of information for homework and essays (Arroyo-Barrigüete et al., Citation2019; Feierabend et al., Citation2021; Rat für kulturelle Bildung e.V., 2019). In particular, short format EVs, in which a single topic excerpt is presented in a compact way to create understanding in the viewer or to trigger a learning process so that primarily new declarative knowledge is generated (Findeisen et al., Citation2019; Handke, Citation2020; tenHove & van der Meij, Citation2015), are particularly attractive.
From a scientific perspective, however, it is evident that solely presenting an EV in an educational setting is nothing more than a passive learning opportunity (Chi & Wylie, Citation2014; Poquet et al., Citation2018; Sailer et al., Citation2021; Wittwer and Renkl, Citation2008) which is likely to lead to insufficient learning outcomes. In addition, research has shown that EVs are associated with some specific learning challenges. For instance, learners may struggle to keep up with the video’s pace, making it challenging to comprehend the content thoroughly. And furthermore, metacognitive misunderstandings (like the illusion of knowledge) can occur and contribute to shallow processing resulting from inadequate cognitive involvement. Therefore, it is evident that EVs require wise instructional scaffolding to become learning opportunities in which learners gain a meaningful understanding of the content.
Based on theoretical considerations, we assume that combining cooperative learning (CL; Chi & Wylie, Citation2014) with the problem-solving-first approach (Loibl et al., Citation2017; Schwartz et al., Citation2011; Sinha & Kapur, Citation2021; Weaver et al., Citation2018) will successfully address the specific difficulties of EV-based learning and lead to enhanced learning success. Our hypothesis is based on the assumption that when learners are engaged cooperatively in a problem-solving task before watching the video, it stimulates cognitive engagement and activates prior knowledge (Chi & Wylie, Citation2014; Kapur & Bielaczyc, Citation2012; Loibl et al., Citation2017; Schwartz et al., Citation2011; Sinha & Kapur, Citation2021). This, in turn, enhances the learner’s ability to comprehend the EV content and increases the likeliness of the learner undertaking learning-related processing while watching the EV. In addition, learning is enhanced by recognizing the importance of the information and identifying gaps in their knowledge (Loibl et al., Citation2017; Sinha & Kapur, Citation2021). Accordingly, this study will examine whether cooperative problem-solving is an optimal preparation for learning (Schwartz & Martin, Citation2004) from EV and will result in the highest learning outcomes. To clarify our research objectives, we first outline the difficulties associated with EVs and then examine the potential benefits of cooperative solving a problem first.
Learning with explainer videos
Although in some way cognitive resources are efficiently used in EVs according to Meyer’s multimedia theory (Mayer & Moreno, Citation1999; Mayer, Citation2005), since they present information coherently over time by utilizing visual-spatial and auditory verbal resources, EVs have some characteristics that can hinder learning more than purely visual material and texts. Critical problems are the transience of information, the illusion of knowledge, the preemption of processes, and cognitive passivity.
Transience of Information: In videos, each piece of information is presented just briefly. At the same time, it is necessary to understand the information that follows. Consequently, viewers must pay sustained attention and actively hold information in working memory to follow subsequent information. This leads to an exceptionally high cognitive load and requires a higher level of cognitive investment when learning from videos (Leahy & Sweller, Citation2016). As a result, it is very easy for learners to become disengaged and not correctly learn the content. When learning with text instead, learners can quickly return to previous sentences and look up the information they overlooked (Caspi et al., Citation2005; Hill & Nelson, Citation2011). Likewise, when teachers give lectures, they usually ask if everyone is following along. If not, teachers can easily repeat information that learners have overheard. In addition, the pace of the lecture can be adjusted, or supplemental images can be used if it is clear from the faces of the learners that they have not been following the lecture.
With videos, learners have fewer strategies for catching up if they fall behind. Of course, it is possible to press stop and rewind, but learners rarely use these options (Mayer & Chandler, Citation2001), and it is also challenging to find the moment when the corresponding word was explained that they have missed. Accordingly, the transience of information is a complex problem associated with insufficient learning outcomes when learning with EV (Leahy & Sweller, Citation2016; Mayer et al., Citation2020; Mayer & Chandler, Citation2001).
Illusion of Knowledge: The phenomenon known as illusion of knowledge occurs when learners mistakenly believe they have understood or learned content despite this not being the case. As a result, cognitive learning processes are reduced, and learning success is compromised. This phenomenon is particularly prevalent with EVs.
Salomon (Citation1984) introduced this concept, demonstrating that TV programs create a false sense of comprehensive understanding. Similarly, Paik and Schraw (Citation2013) found that detailed video animations can have a similar effect, with learners underestimating the complexity and perceiving content as straightforward. Basu Roy and McMahon (Citation2012) study presented similar findings. Although learners did not feel the content was overly simplistic, they assumed that videos made learning more accessible and required less cognitive effort. This also led to a superficial processing with little elaboration. Thus, there is evidence that especially videos frequently create the illusion of knowledge, resulting in a lack of cognitive engagement among learners.
Preempting processes: There are two sides to this aspect. Videos are commonly used to illustrate dynamic relationships that are cumbersome to articulate through text. In this vein, video can aid learners in building an exact mental representation of the content that would otherwise prove challenging to imagine. Nevertheless, forming a mental representation of dynamic relationships also serves as an initial learning experience that promotes deeper comprehension during content acquisition. When describing a process in a text, learners are indirectly stimulated to engage more actively with the content as they attempt to visualize what is being explained. If the teacher takes over this opportunity for active learning with the help of the video, the learner’s opportunity for higher-order processing is hindered (Schnotz & Rasch, Citation2005). This coincides with the concept of the generation effect, which represents one example of desirable difficulties (Bjork & Bjork, Citation2011). A significant amount of research in that field suggests that it is sometimes advantageous to increase the difficulty level to stimulate improved learning. It may benefit learners to have some aspects of the learning process self-generated rather than entirely predetermined, even if it is more complex. Or the other way around, it does not always aid learning to make things with an EV easier. Sometimes, this ease can preempt essential processes that are better handled by the learners themselves.
Cognitive Passivity: The last aspect to consider is general and concerns not only inadequate learning with videos but also any learning situation in which new information is given without any additional task. Whether it is a text, video, or lecture, in most cases, situations in which providing information is the primary focus result in learners being passive participants. This implies that learners receive information but do little to process it (Chi & Wylie, Citation2014). However, effective learning can only occur when learners activate their existing knowledge and actively incorporate new information (Mayer, Citation1996). This process is called elaboration (Anderson & Reder, Citation2015) and seldom occurs when information is passively received.
Based on the above, it is evident that effective EV-based learning requires thoughtful instructional design. It must be designed to engage, cognitively activate, and motivate the learner to comprehend the information presented fully. This will facilitate the integration of the content with the learner’s existing knowledge networks and promote positive learning outcomes.
Instructional embedding of explainer videos
We think the most straightforward approach to enhance learning success is through cooperative learning (CL). This assumption is primarily based on the established ICAP model formulated by Chi and Wylie (Citation2014). This model assumes that there are four modes in which learners could engage with learning content. These are interactive, constructive, active, and passive. Chi and Wylie suggest that cognitive activation increases from passive to active to constructive to interactive, thereby increasing learning success. Furthermore, they explain that especially the most activating forms of engagement activities are most likely to be triggered in CL settings. Constructive engagement activities, in which learners are stimulated to actively integrate new knowledge from learning media into their existing prior knowledge network, arise particularly effortlessly when learners explain content to peers (King, Citation1998), for example, while solving complex problems. This is comparable to interactive activities encouraging learners to extend their newly acquired knowledge actively. This cognitive engagement is also elicited easily when learners engage in intensive discussions with peers. Through the exchange of ideas, thoughts, and different perspectives, it very likely initiates the extension of knowledge. Thus, CL is an effective strategy to facilitate higher engagement activity and, with this, a higher cognitive activation and a more comprehensive understanding of the newly acquired learning content (Chi & Wylie, Citation2014). Therefore, embedding EVs in appropriate CL, which creates constructive and interactive learning processes, will solve the critical problem of the lack of cognitive activation while watching EVs and increase cognitive engagement with the EV content. However, merely placing learners together in a group does not inherently constitute CL.
To foster beneficial effects through peer interactions, additional considerations are necessary. In this context, two approaches can be distinguished: one focuses on external guidance and structure to promote favorable interactions (e.g., Fischer et al., Citation2007; Johnson & Johnson, Citation2009; Slavin, Citation1983), which may include methods, scripts, training, or specific incentive systems. Another approach is that particular task situations inherently foster conducive interaction (Cohen, Citation1994; Sears, Citation2006; Sears and Reagin, Citation2013). Meta-analyses indicate that both externally structured CL formats (Johnson & Johnson, Citation2009; Springer et al., Citation1999) and those without (Tenenbaum et al, Citation2020) exhibit comparable positive effects. In light of the aforementioned perspectives, scholars have ongoing discourse concerning the necessary conditions for CL and its definition (Hmelo-Silver & Chinn, Citation2015; Webb & Burnheimer, Citation2023). Nevertheless, various approaches share that a common requirement for CL is the presence of shared and individual learning goals, which can either be facilitated by external structures or inherent task demands. Consequently, we define CL based on this commonality as a form of peer interaction that aims to achieve shared learning goals while striving for individual learning success.
Considering previous research demonstrating the positive effects of CL (Hattie, Citation2023; Johnson & Johnson, Citation2009; King, Citation1995; Kyndt et al., Citation2013; Springer et al., Citation1999; Tenenbaum et al., Citation2020), our primary research interest is not in assessing whether CL surpasses individual learning. Instead, we aim to compare two specific CL formats to determine which is most effective for learning with EVs (Nokes-Malach et al., Citation2015). Given the different approaches to fostering effective CL, each successful in its way, we have decided to identify a task setting in this study that naturally encourages beneficial CL. Rather than relying on external structuring to induce effective CL, we aim to determine a task that inherently facilitates it.
Effective cooperative learning format for videos
Let us imagine we use an EV and have learners subsequently solve cooperatively a complex problem that fits the new subject content – a classic CL scenario. The anticipated exchange during the problem-solving task will likely generate a higher willingness for cognitive engagement while watching the video. Learners understand that paying attention to the information in the video is crucial for success in the remainder of the task. Additionally, in small group settings, learners strive to avoid appearing incompetent in the eyes of their peers due to their inability to participate effectively (Buchs & Butera, Citation2009). In addition, any knowledge gaps can subsequently be filled by building a shared knowledge base. Moreover, joint communication results in significantly enhanced depth of processing. However, the danger of acquiring only superficial and transient knowledge remains. Students might miss crucial information simultaneously, resulting in a dearth of common ground for discussion and reciprocal explanation. Accordingly, this “classic” design might not be sufficient to achieve successful learning results. For this reason, we propose the “problem-solving first” (PF) approach as a promising form of cooperative learning with EVs.
The PF approach refers to learning arrangements in which learners work on a complex task before presenting new learning content (Loibl et al., Citation2017; Schwartz et al., Citation2011; Sinha & Kapur, Citation2021; Weaver et al., Citation2018). In this instructional sequence, it is assumed that prior knowledge is activated, the complexity of the topic is more appropriately assessed, and higher motivation is generated (Loibl et al., Citation2017). These mechanisms, in turn, provide optimal preparation for processing and receiving subsequently presented information, thus enabling elaboration and hence enhancing learning success (Glogger-Frey et al., Citation2017).
Prior Knowledge activation: When working through a complex problem without further instructional guidance, learners must retrieve all of their prior knowledge to get as close as possible to a likely solution. Potentially, this process will be enhanced by working on the problem cooperatively. Due to the extensive activation of prior knowledge, knowledge networks become more malleable. Consequently, incorporating new information is enhanced, and integration into existing knowledge networks is improved (Loibl et al., Citation2017).
Additionally, activating prior knowledge before introducing new learning material may decrease cognitive load during the reception of the new content. This is due to the fact that prior knowledge is often necessary for comprehending new information. When prior knowledge is not activated, more working memory resources are required to retrieve it while simultaneously attending to the EV and processing the new information (Ericsson & Kintsch, Citation1995). This significantly increases situational cognitive load. If the relevant prior knowledge has already been activated, learners could use all resources to process the new information while watching the EV. Accordingly, in PF settings, two crucial cognitive processes – prior knowledge activation and information processing – are time-stretched and occur sequentially, thus reducing cognitive load and facilitating comprehension of complex transient content (Sweller, Citation2011, Citation2020).
Complexity of content: Since solving the problem may be challenging without new knowledge and some students may not reach a solution, learners become aware of the complexity of the learning content and their knowledge gaps. Consequently, they recognize the need for further cognitive effort to find the correct solution (Kerrigan et al., Citation2021). As a result, they are prevented from developing an illusion of knowledge. In addition, cognitive engagement and motivation for the targeted learning material increase by creating a sense of curiosity.
Due to these mechanisms, PF initiates the basis for a certain depth of processing and leads to increased transfer competence and conceptual knowledge. This has already been demonstrated in numerous experimental studies (e.g., DeCaro & Rittle-Johnson, Citation2012; Fyfe et al., Citation2014; Glogger-Frey et al., Citation2017; Kapur & Bielaczyc, Citation2012; Schwartz et al., Citation2011; Sinha & Kapur, Citation2021). Regarding factual and procedural knowledge, there is a valid debate as incomplete problem-solving efforts may negatively impact attention. Learners may solely concentrate on the content directly related to the problem task and overlook important basic information. For instance, Sears (Citation2006) reported in his study that in the case of PF, higher conceptual knowledge was generated, but lower knowledge related to the explicitly conveyed content, such as factual and procedural knowledge. This finding is consistent with those of Ashman et al. (Citation2020). Nonetheless, the majority of studies investigating PF suggest that factual and procedural knowledge is learned to the same extent as in traditional settings (Loibl et al., Citation2017).
Despite considerable evidence supporting the effectiveness of PF, certain questions persist, as PF does not consistently yield significantly superior outcomes (Loibl et al., Citation2017; Nachtigall et al., Citation2020). One open question is whether PF is a suitable approach for all domains. Nachtigall et al. (Citation2020) argued that PF potentially primarily benefits science learning due to the distinctive structural attributes of domain-specific problems. Science problems typically are well-structured problems (Jonassen, Citation2000), allowing learners to validate their solutions’ accuracy. Conversely, domains like social sciences often feature ill-structured problems with multiple potential solutions (Jonassen, Citation2000). Therefore, the mechanisms of PF—awareness of knowledge gaps and curiosity to find the correct answer—may not necessarily be elicited since learners do not realize their failure.
Another open question is whether learners need to solve the problems within PF settings in groups or individually to derive its benefits. Many studies involve learners solving tasks in small groups, typically pairs (e.g., Kapur & Bielaczyc, Citation2012; Kerrigan et al., Citation2021; Schwartz & Martin, Citation2004). Moreover, Sinha and Kapur (Citation2021) explicitly underscore the essential role of working in groups in PF. However, these studies do not explicitly test the need for CL. An experimental study by Mazziotti et al. (Citation2019), which tested whether CL is needed, found no discernible effect of CL on the learning outcomes of primary school students. However, since PF had no effect overall in this study, whether learning individually or in groups, implications for PF design remain uncertain. In a similar vein, Weaver et al. (Citation2018) also failed to identify a clear advantage for PF in groups. However, the significance of these results is also unclear, as other aspects were altered compared to other PF studies. For instance, the group sizes were larger, and learners in the cooperative condition received additional instructional instructions. These changes could have led to a higher cognitive load, which in turn had a negative effect on learning success.
Conversely, other studies demonstrate that particularly effective interactions between learners reinforce the effects observed within PF conditions (Kapur & Kinzer, Citation2009; Kerrigan et al., Citation2021; Sears, Citation2006). Additionally, Kapur and Kinzer (Citation2009) highlight the importance of minimal task structuring to foster interaction solely driven by the task itself. Accordingly, based on the available empirical evidence, the extent to which CL contributes to the performance of the PF is uncertain.
To facilitate a more comprehensive understanding of the role of CL in PF in the future, we suggest that a slightly altered viewpoint could provide valuable insights. Given our objective of identifying an optimal CL environment for learning from EV, it seems worthwhile to pose the following question: Could PF be providing an instructional setting to foster highly beneficial CL, and consequently, could quality of CL be mediating the efficacy of PF?
Introducing a problem-solving task before presenting information heightens its challenge, encouraging learners to collaborate in evaluating potential solutions (Cohen, Citation1994). In the absence of a pre-established basis of shared knowledge, such as that provided by an EV before CL, learners engage in extensive dialogue to construct shared understanding (Asterhan & Schwarz, Citation2007). This fosters productive interactions, enhancing cognitive activation and activating prior knowledge (Schwartz, Citation1995; Sears, Citation2006). In light of these assumptions, we assume that PF fosters an optimal learning environment that is conducive to highly effective CL, thus facilitating subsequent learning success.
Current study
Altogether, the theoretical considerations suggest that PF, in combination with CL, serves as an effective cognitive preparation to increase learning success when learning with EV. The increased learning success can be partially attributed to more effective CL resulting from the reversed instructional order of PF. Incorporating these instructional design elements with EVs will facilitate deep processing and lead to better learning success. This will manifest in a better transfer of the new knowledge to similar but novel tasks while learners acquire equal factual and procedural knowledge. Furthermore, it is hypothesized that PF improves the quality of CL in terms of group communication and satisfaction, potentially contributing to its effectiveness. In this regard, we propose the following hypothesis:
H1: Learners who perform problem-solving tasks before watching an EV will show better knowledge transfer than those who watch the EV first, while factual and procedural knowledge is not affected.
H2: Learners who perform problem-solving tasks before watching an EV will experience more successful cooperation than learners who watch the EV first. This will be expressed in a higher quality of cooperation and partly explain the beneficial effects of solving the problem task first (mediation hypotheses).
Methods
Participants and design
A field-experimental study was conducted in a lecture for future primary school teachers in physics at the University. The sample consist of N = 58 undergraduates (50 = female, 8 = male; age: M = 21.48, SD = 3.72). These participants were all undergraduates who regularly participated in the lecture.
In order to create a realistic learning environment, the learning module was integrated into the standard physics lecture for these future primary school teachers. This means that a common foundation of prior knowledge was established in the previous lesson (on the topic of light). And in the following lecture, the experimental knowledge was expanded. For their participation, the students received 10 €. Sessions were scheduled throughout the week and randomly assigned to conditions. As a result, 30 individuals were assigned to the Problem-First (PF) condition and 28 to the Video-First (VF) condition. In each session, participants were paired randomly in groups of two.
Procedure
Each session lasted up to 90 min via online videoconference. The session started with a request for informed consent from all participants. Then, students were first asked to complete the pre-session questionnaire (prior knowledge, attitudes, demographic data), which took about 8 min. This was followed by the learning phase, which took the same amount of time (45 min) for all students. During the learning phase, three processes were to take place: (1) watch the video (∼10 min.), (2) capture important aspects of the video (∼10 min.), (3) solve an application task (∼15 min.). Additionally, students should link the content of the EV to the problem-solving task (approximately 5 min). This was incorporated into the problem-solving phase for the VF condition and in the discussion of the EV for the PF condition. Thus, both conditions yield the sequence displayed in .
Figure 1. Schematic overview of the experimental procedure. The upper half shows the condition problem first, while the lower half shows the condition video first.
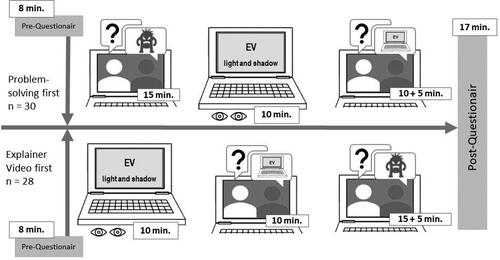
After the learning phase, the students were again asked to complete a questionnaire (quality of CL, technical problems) and the test on learning success, for which the participants needed about 17 min. In the end, there was a closing discussion to clear up any last questions and to give a summary.
Materials
Explainer video
An EV of the YouTube channel “musstewissen Physik” (musstewissen Physik [funk], 2017) was used. This EV deals with fundamental physical knowledge about light and shadow. The content of the EV fits entirely with the regular lecture and is of high quality from a didactic perspective, following the criteria of Kulgemeyer and Peters (Citation2016). It lasts 11 min and is moderated by two young men. It uses graphic representations to illustrate, for example, how shadow graphs can be used to identify the positions of light sources.
Problem-solving tasks
For the problem-solving phase, we have created two tasks that are easily solvable using the video’s content, but most students will not succeed in finding the correct solutions without the video. In a pretest, we identified that these two tasks fit the best because the pretest participants had some ideas on solving the tasks, but no student got the correct answer. The first task was to determine the position and characteristics of the light source based on a given image of a cup with some shadows. The second task was to create a monstrous green shadow image of a figure on the screen of a shadow theater.
Learning success
Three subsets of items were created to assess learning success, which assessed three knowledge types (see ). The first subset consisted of three items and assessed procedural knowledge. These items required learners to apply the EV processes to similar new tasks. The second subset consisted of seven items and assessed factual knowledge. The items asked for content that was mentioned verbatim in the EV. The third subset included two open-ended tasks to evaluate learners’ ability to transfer the acquired knowledge. Despite being relatively complex, these tasks were easier to solve if the knowledge from the EV was understood in depth. However, learners had to establish additional connections to provide suitable solutions. presents examples of items. The items were partly multiple-choice or single-choice questions and partly open-ended questions. In order to stay within a reasonable time frame in which students can still concentrate, we used just a few relatively different items. The open-format questions were rated using a rating scheme by two independent raters. One item was excluded due to a lack of interrater agreement. Cohen’s kappa ranged from κ =.707 to κ =.965 for the other items. According to Altman (Citation1990), these values indicate good interrater reliability. Additionally, Cronbach’s α was calculated for each subset. The internal consistency was α =.4 for procedural knowledge, α =.49 for factual knowledge, and α =.24 for transfer knowledge. These low consistencies are not surprising given the short and wide-scale construction. However, as Stadler et al. (Citation2021) suggest, even scales with low consistency can be appropriate for assessing knowledge.
Table 1. Examples of the learning success assessment items.
Additional measurements
We assessed students’ prior knowledge and attitudes toward CL as control variables in the pre-questionnaire. In the post-test, we assessed the quality of CL and whether technical problems had arisen.
We asked students to evaluate their familiarity with the general topic for prior knowledge. We interpret this as a reliable proxy for prior knowledge since a prior knowledge test would interfere with the treatment. Students’ attitudes regarding CL were evaluated using four items constructed for this purpose. The items assessed more general perceptions of CL, including whether students have positive or negative attitudes toward it. These items were used as control variables to ensure that conditions were comparable. The items were: “In group work, I like that I can work together with others.” “I find it easier to learn in group work.” “I like seminars in which a lot of work is done in groups.” “I enjoy learning in groups.” This scale had a Cronbach’s α of .91.
In order to assess the quality of CL in the two conditions, a 7-item self-report scale was employed. The scale encompasses items that assess perceptions of interaction, utility, and satisfaction with CL. The items pertaining to interaction were formulated following the theoretical reasoning of the ICAP model. As previously stated, the benefits of CL lie in the intensive interaction that it facilitates, including the sharing of ideas and the stimulation of new thinking. Consequently, items pertaining to interaction aspects were formulated. The utility items were formulated based on the assumption that effective CL occurs when tasks are challenging and require collaboration (Cohen, Citation1994). Consequently, learners should perceive the learning experience of CL as being helpful. Finally, in instances where CL is found to be beneficial, learners should experience a sense of satisfaction with the collectively developed product and the cooperation. Consequently, items were integrated to assess satisfaction with CL for all items, see . The scale showed a Cronbach’s α of .77.
Table 2. Items of CL quality scale.
While further measures would undoubtedly be advantageous for identifying underlying cognitive mechanisms, we decided against measuring them due to specific challenges in PF experiments. For instance, assessing prior knowledge, curiosity, or awareness of knowledge gaps before the instructional phase (EV) is problematic. Inquiring about these aspects in the VF condition could induce effects similar to those in the PF condition, thereby reducing the contrast between the conditions. Therefore, it is preferable to avoid measuring these mechanisms to prevent biased results when evaluating the potential positive impact of PF on learning outcomes. Additionally, querying these factors through self-reports at the end of the experiment may not yield reliable results, as learners may not accurately recall their initial state of mind at the beginning of the instruction. Therefore, no supplementary measures were assessed during the learning phase.
Power analysis
An a priori power analysis was conducted with GPower (Faul et al., Citation2007) to determine the appropriate sample size for repeated measures (conceptual, factual, and procedural knowledge) MANOVA with a between-subjects factor (condition: PF and VF). The analysis was based on the following parameters: an anticipated effect size of f = 0.25, an alpha error probability of .05, and a desired statistical power level of .90. Based on these parameters, the power analysis indicated that a total sample size of 60 would be required to achieve sufficient power. The power may be lower depending on the ICC (intra-class correlation) of the measurements within the teams. However, since the ICC values cannot be estimated, they are not considered.
Data analysis
The data analysis, conducted using R Studio (R Core Team Citation2023) and Mplus (Muthén & Muthén, Citation2015), involved the R packages readxl, tidyverse, dplyr, tidyselect, descTools, effsize, apaTables, and psych. Considering the hierarchical structure, advanced modeling was carried out using Mplus software, which is mainly needed for specialized structural equation modeling.
Results
As an initial step, the data were processed using RStudio to calculate descriptive data for the two conditions. presents these results. We conducted independent t-tests on the control variables to determine the comparability of the groups. The t-test results showed no significant difference between the groups in terms of attitude toward CL (t(56) = −0.80, p = .426). Thus, these results indicate no significant differences between the groups at the beginning of the experiment regarding attitudes.
Table 3. Means, standard deviations, and correlations with confidence intervals.
Multilevel path analysis
A multilevel analysis was conducted using Mplus version 8.3 to account for potential data dependencies within teams. Individual students were considered as level 1 and the team as level 2. The analysis tested whether the experimental condition (PF vs. VF) affected the three knowledge components. The model also examined whether the quality of cooperation mediates the positive effects of PF. Furthermore, learning outcomes were controlled for individual prior knowledge. The model was estimated using the maximum likelihood estimator with robust standard errors (MLR) and was based on 58 observations across 29 teams ( and ).
Figure 2. The multi-level structural model presented as a path diagram with the three types of knowledge as the dependent variables, condition as an independent variable, cooperation quality as a mediator, and prior knowledge as control variable.
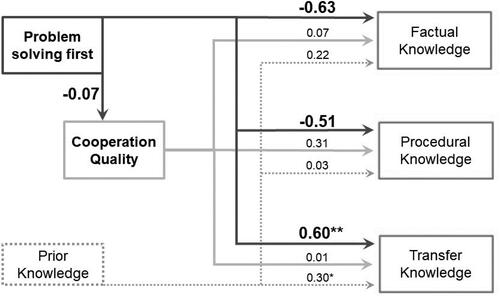
Table 4. Multilevel model for learning outcomes with CL quality as mediator.
First, at the within-level analysis, the impact of prior knowledge on the three measures of learning outcome variables was examined. Prior knowledge exhibited a statistically significant positive effect on the transfer of knowledge (β = 0.30, p = .040), indicating that an increase in prior knowledge was associated with higher values of transfer of knowledge. However, the effect of prior knowledge on procedural knowledge (β = 0.03, p = .813) and factual knowledge (β = 0.22, p = .218) was not statistically significant.
Turning to the between-level analysis, the influence of condition and quality of CL on the learning outcome variables was investigated to answer our research hypotheses. The condition showed a statistically significant positive effect on the transfer of knowledge (β = 0.60, SE = 0.20, p =.003), indicating that teams of the PF condition achieved higher values of transfer of knowledge. However, condition did not significantly affect procedural knowledge (β = −0.51, SE = 0.92, p =.579) or factual knowledge (β = −0.63, SE = 1.05, p =.549). The effect of quality of CL on the dependent variables was not statistically significant. Specifically, quality of CL did not significantly impact the transfer of knowledge (β = 0.01, SE = 0.22, p = .961), procedural knowledge (β = 0.31, SE = 0.82, p =.702), or factual knowledge (β = 0.07, SE = 0.41, p =.861). Furthermore, the condition showed no significant effect on the quality of CL (β = −0.07, SE = 0.19, p =.705).
The chi-square test of model fit yielded a value of 52.58 with 16 degrees of freedom (p < 0.001), indicating a significant deviation between the model and the observed data.
The Comparative Fit Index (CFI) was 0.906, which is slightly below the threshold for an acceptable model fit. Notwithstanding, the Root Mean Square Error of Approximation (RMSEA) was estimated at 0.000, and the Tucker-Lewis Index (TLI) was 1.000, indicating an excellent model fit. Consequently, the model fit may be considered acceptable.
Discussion
This study aimed to identify an instructional design that promotes successful learning with EV. Such a design must predominantly cognitively activate and decrease cognitive load to compensate for the challenges caused by the transience of the presented information, preempting learning processes, and the illusion of knowledge. We hypothesized that CL within the PF approach would generate effects enabling superior elaboration of the EV content. The results indicate that using the PF approach leads indeed to enhanced depth of processing while learning cooperatively with EV. Furthermore, the combination of CL and PF resulted in comparable levels of success in acquiring factual or procedural knowledge compared to an instructional design where problem-solving follows the EV. Accordingly, the study’s objective was achieved, and a successful instructional design was generated. PF appears to be an ideal learning method for preparing learners to receive an EV. It enhances processing depth and elaboration, enabling students to apply their knowledge abstractly in complex new tasks. This is consistent with Schwartz and Martin (Citation2004) notion that PF principles are activities that prepare students for future learning.
From a scientific perspective, these results are interesting, especially for PF-related research. Since in PF settings, usually, the to-be-learned information is presented face-to-face (e.g., DeCaro & Rittle-Johnson, Citation2012; Schwartz et al., Citation2011), which includes the possibility of taking up the learners’ solutions easily (Hartmann et al., Citation2021; Kapur & Bielaczyc, Citation2012; Loibl et al., Citation2017; Sinha & Kapur, Citation2021), it was questionable whether EV would be an appropriate substitute. If typical EVs from the Internet are used, exemplary student solution approaches cannot be integrated, and a flexible adaptation to the students’ initial problem-solving processes is impossible. This may hinder embedding knowledge in existing knowledge networks, potentially limiting the positive effects of PF with EV. However, our study demonstrated typical PF effects even without explicit integration of student solutions. Considering that a linking task was implemented, which replaces the teaching activity of picking up the students’ solutions. Our findings raise doubts regarding the necessity of explicitly incorporating learners’ solutions and ideas, specifically in groups of learners in advanced stages of education. In conclusion, our results suggest that EVs may work as well as a typical face-to-face presentation of the to-be-learned information in PF settings.
Consistent with most literature (Loibl et al., Citation2017), PF did not hinder learning success regarding information close to the EV content. Specifically, in our learning situation, the EV provides some additional information that is not directly related to the problem-solving task, so a strong focus on problem-related information could negatively affect the learning and retrieval of this information. However, since factual knowledge and procedural knowledge scores do not differ even for problem-unrelated information, the clear superiority of the PF setting is evident.
Moreover, our findings have considerable implications for EV learning research. Our results suggest that PF, in combination with CL, is likely to stimulate highly active, generative, and independent learning that engages learners cognitively and fosters successful elaboration of EV content. Nonetheless, the precise mechanisms underlying this superior learning outcome and the efficacy of instructional design across different EV contexts remain unclear.
Regarding our second research hypothesis, the suspected effect of PF on self-reported quality of CL did not emerge in our study. Therefore, it remains unclear what role CL plays. Surprisingly, there was no correlation between the subjective assessment of CL quality and learning success, which contradicts the empirical findings of other PF studies (Kapur & Kinzer, Citation2009; Kerrigan et al., Citation2021). A possible ceiling effect may account for these results because it is evident that all students evaluated group work very positively across measurements. Thus, it can be inferred that CL was highly effective in all groups, highlighting the strategy’s success in creating an environment conducive to CL. Nonetheless, differences cannot be statistically ascribed to CL. As the study was conducted in the spring of 2021, the pandemic situation may have contributed to these exceptionally high positive results. During the initial phase of the pandemic, the university’s teaching formats primarily relied on self-learning methods, while social interactions were minimized. Accordingly, it could have been particularly favorable for students to finally come into contact with other students and be able to share ideas about learning content. As such, external situational factors may have influenced the outcomes of the experimental manipulation. As a result, we could not support the assumption that PF increases the quality of CL and, subsequently, has positive effects on learning success. Discovering the connection between PF and CL is an open question. Therefore, future research should focus on this question and assess CL aspects in detail with additional objective measures.
Finally, our findings are of practical interest to teachers. Our findings can serve as a basis for designing concrete teaching situations with EVs in students’ everyday lives. Teachers just need to search for (or produce) appropriate EVs and create complex tasks. By reversing the order, an effective learning arrangement is created in which students develop a comprehensive understanding. In this scenario, teachers are not required to simultaneously instruct all students in person, thus freeing up resources for individual support. This allows learners to manage their learning time more independently. However, attention should be paid to whether the students being addressed can cooperate effectively and regulate their learning processes. If not, they will maybe require some guidance (Fischer et al., Citation2007).
Additionally, the practical relevance does not only apply to school settings. Especially in higher education, like the lecture setting in this study, where teachers in training learn scientific concepts that they will teach their students, an appropriate depth of processing is crucial. It is not enough for the content to be absorbed superficially. Teachers in training must grasp this content in depth to make accurate didactical decisions. Therefore, this instructional design could increase the quality of teachers’ education and, overall, higher education.
Furthermore, since educational settings need flexible digital and face-to-face lecture strategies, the experimental learning arrangement fits effortlessly. Our experiment’s entire learning process took place online and digitally, without complicated digital media. Since the initial problem-solving is primarily designed to optimize the video content’s processing, only a digital means of communication and a digital device are required to play the video. Accordingly, most teachers and educators should have no problem implementing this arrangement digitally, and it can be assumed that if it is implemented face-to-face, it will lead to equal effects.
Limitations
The first concern regarding the present study is the small sample size. This sample size allowed us to detect only medium to large effects with sufficient power. Therefore, replication with a larger number of learners is needed to confirm evidence of our non-significant findings.
The second concern relates to cooperative learning (CL). No objective measurements were used, such as audio or video recordings with a rating schema. Due to the COVID-19 pandemic, conducting the experiment in a controlled environment where we could make our own recordings was not feasible. Technically, students could only record themselves and send in these recordings. Previous studies showed this led to significant data loss, as groups spent excessive time on technical issues rather than the task, focusing on organizational matters instead. Therefore, we decided against recording to allow learners to concentrate fully on the task and engage in intensive discussions. As a result, we relied solely on self-assessment measures for CL. These measures may be biased due to social desirability (DeMaio, Citation1984) and a lack of self-assessment competence (Jurkowski et al., Citation2022).
Nevertheless, self-assessment was used for economic reasons. Given that the participants were undergraduates, we assumed they could reasonably assess the adequacy of CL. However, it is advisable to investigate the effects with objective measures in more complex studies.
Conclusions
Considering the abovementioned limitations, the present study showed examples of effective CL arrangements with an EV. Since digital media are a permanent part of everyday life, they need to be implemented in lectures to guide learners on dealing with these information resources. Even if EVs seem promising, learning can fail when they lead to cognitive overload or a lack of cognitive activation. Therefore, the implementation is not trivial. Our results indicate that solving complex problems cooperatively before watching an EV enhances elaboration and, consequently, learning success. These findings could guide educators in implementing EVs methodically and effectively in diverse (digital) learning settings. For future research, examining the cognitive mechanisms and whether these findings could be transferred to other domains would be interesting. Finally, exploring the relationship between CL and PF in learning arrangements remains challenging.
Authors’ contributions
The first Author conceived the study, conducted the research, and drafted most of the manuscript. The second author acted as a supervisor, providing substantial input in shaping the content and contributing to the revision and editing of the manuscript.
Acknowledgments
This project is part of the “Qualitätsoffensive Lehrerbildung,” a joint initiative of the Federal Government and the Länder, aiming to improve teacher training quality. The authors are responsible for the content of this publication. The investigation followed all established legal requirements. The instructors of the relevant courses and their students were informed about the study and gave their consent for its implementation. Ethical approval was not required since no personally identifiable data was collected.
Disclosure statement
The authors report there are no competing interests to declare.
Data availability statement
The data supporting this study’s findings are available on request from the corresponding author.
Additional information
Funding
References
- Altman, D. G. (1990). Practical statistics for medical research. CRC Press.
- Anderson, J. R., & Reder, L. M. (2015). An elaborative processing explanation of depth of processing. In L. S. Cermak & F. I. M. Craik (Eds.), Psychology library editions: Memory: Volume 5. Levels of processing in human memory (pp. 385–403). Psychology Press.
- Arroyo-Barrigüete, J. L., López-Sánchez, J. I., Minguela-Rata, B., & Rodríguez-Duarte, A. (2019). Use patterns of educational videos: A quantitative study among university students. WPOM-Working Papers on Operations Management, 10(2), 1–19. https://doi.org/10.4995/wpom.v10i2.12625
- Ashman, G., Kalyuga, S., & Sweller, J. (2020). Problem-solving or explicit instruction: Which should go first when element interactivity is high? Educational Psychology Review, 32(1), 229–247. https://doi.org/10.1007/s10648-019-09500-5
- Asterhan, C. S., & Schwarz, B. B. (2007). The effects of monological and dialogical argumentation on concept learning in evolutionary theory. Journal of Educational Psychology, 99(3), 626–639. https://doi.org/10.1037/0022-0663.99.3.626
- Basu Roy, R., & McMahon, G. T. (2012). Video-based cases disrupt deep critical thinking in problem-based learning. Medical Education, 46(4), 426–435. https://doi.org/10.1111/j.1365-2923.2011.04197.x
- Bjork, E. L., & Bjork, R. A. (2011). Making things hard on yourself, but in a good way: Creating desirable difficulties to enhance learning. Psychology and the Real World: Essays Illustrating Fundamental Contributions to Society, 2, 59–68.
- Buchs, C., & Butera, F. (2009). Is a partner’s competence threatening during dyadic cooperative work? It depends on resource interdependence. European Journal of Psychology of Education, 24(2), 145–154. https://doi.org/10.1007/BF03173007
- Caspi, A., Gorsky, P., & Privman, M. (2005). Viewing comprehension: Students? Learning preferences and strategies when studying from video. Instructional Science, 33(1), 31–47. https://doi.org/10.1007/s11251-004-2576-x
- Chi, M. T. H., & Wylie, R. (2014). The ICAP framework: Linking cognitive engagement to active learning outcomes. Educational Psychologist, 49(4), 219–243. https://doi.org/10.1080/00461520.2014.965823
- Cohen, E. G. (1994). Restructuring the classroom: Conditions for productive small groups. Review of Educational Research, 64(1), 1–35. https://doi.org/10.3102/00346543064001001
- DeCaro, M. S., & Rittle-Johnson, B. (2012). Exploring mathematics problems prepares children to learn from instruction. Journal of Experimental Child Psychology, 113(4), 552–568. https://doi.org/10.1016/j.jecp.2012.06.009
- DeMaio, T. J. (1984). Social desirability and survey. Surveying Subjective Phenomena, 2, 257.
- Ericsson, K. A., & Kintsch, W. (1995). Long-term working memory. Psychological Review, 102(2), 211–245. https://doi.org/10.1037/0033-295x.102.2.211
- Faul, F., Erdfelder, E., Lang, A.-G., & Buchner, A. (2007). G*Power 3: A flexible statistical power analysis program for the social, behavioral, and biomedical sciences. Behavior Research Methods, 39(2), 175–191. https://doi.org/10.3758/BF03193146
- Feierabend, S., Rathgeb, T., Kheredmand, H., & Glöckler, S. (2021). JIM-Studie 2021: Basisuntersuchung zum Medienumgang 12-bis 19-Jähriger [JIM Study 2021: Basic study on media use among 12- to 19-year-olds]. https://www.mpfs.de/fileadmin/files/Studien/JIM/2021/JIM-Studie_2021_barrierefrei.pdf
- Findeisen, S., Horn, S., & Seifried, J. (2019). Lernen durch videos – Empirische Befunde zur Gestaltung von Erklärvideos [Learning through videos – Empirical findings on the design of explainer videos]. MedienPädagogik: Zeitschrift Für Theorie Und Praxis Der Medienbildung, 19(1), 16–36. https://doi.org/10.21240/mpaed/00/2019.10.01.X
- Fischer, F., Kollar, I., Mandl, H., & Haake, J. M. (Eds.) (2007). Scripting computer-supported collaborative learning: Cognitive, computational and educational perspectives (Vol. 6). Springer Science & Business Media.
- Fyfe, E. R., Decaro, M. S., & Rittle-Johnson, B. (2014). An alternative time for telling: When conceptual instruction prior to problem solving improves mathematical knowledge. British Journal of Educational Psychology, 84(Pt 3), 502–519. https://doi.org/10.1111/bjep.12035
- Glogger-Frey, I., Gaus, K., & Renkl, A. (2017). Learning from direct instruction: Best prepared by several self-regulated or guided invention activities? Learning and Instruction, 51, 26–35. https://doi.org/10.1016/j.learninstruc.2016.11.002
- Handke, J. (2020). Handbuch Hochschullehre Digital: Leitfaden für eine moderne und mediengerechte Lehre (3. Auflage) [Handbook university teaching digital: Guide for modern and media-compatible teaching (3rd ed.). Tectum Wissenschaftsverlag. https://doi.org/10.5771/9783828875302
- Hartmann, C., Van Gog, T., & Rummel, N. (2021). Preparatory effects of problem solving versus studying examples prior to instruction. Instructional Science, 49(1), 1–21. https://doi.org/10.1007/s11251-020-09528-z
- Hattie, J. (2023). Visible learning: The sequel. A synthesis of over 2,100 meta-analyses relating to achievement. Routledge.
- Hill, J. L., & Nelson, A. (2011). New technology, new pedagogy? Employing video podcasts in learning and teaching about exotic ecosystems. Environmental Education Research, 17(3), 393–408. https://doi.org/10.1080/13504622.2010.545873
- Hmelo-Silver, C. E., & Chinn, C. A. (2015). Collaborative learning. In Handbook of educational psychology (pp. 363–377). Routledge.
- Isohätälä, J., Näykki, P., Järvelä, S., & Baker, M. J. (2018). Striking a balance: Socio-emotional processes during argumentation in collaborative learning interaction. Learning, Culture and Social Interaction, 16, 1–19. https://doi.org/10.1016/j.lcsi.2017.09.003
- Johnson, D. W., & Johnson, R. T. (2009). An educational psychology success story: Social interdependence theory and cooperative learning. Educational Researcher, 38(5), 365–379. https://doi.org/10.3102/0013189X09339057
- Jonassen, D. H. (2000). Toward a design theory of problem solving. Educational Technology Research and Development, 48(4), 63–85. https://doi.org/10.1007/BF02300500
- Jurkowski, S., Mundelsee, L., Jüngst, C., & Hänze, M. (2022). Measuring knowledge co-construction: A comparison of high-inference observation, low-inference coding and self-report of transactive communication. Zeitschrift Für Erziehungswissenschaft, 25(6), 1505–1527. https://doi.org/10.1007/s11618-022-01124-w
- Kapur, M., & Bielaczyc, K. (2012). Designing for productive failure. Journal of the Learning Sciences, 21(1), 45–83. https://doi.org/10.1080/10508406.2011.591717
- Kapur, M., & Kinzer, C. K. (2009). Productive failure in CSCL groups. International Journal of Computer-Supported Collaborative Learning, 4(1), 21–46. https://doi.org/10.1007/s11412-008-9059-z
- Kerrigan, J., Weber, K., & Chinn, C. (2021). Effective collaboration in the productive failure process. Journal of Mathematical Behavior, 63, 100892. https://doi.org/10.1016/j.jmathb.2021.100892
- King, A. (1995). Inquiring minds really do want to know: Using questioning to teach critical thinking. Teaching of Psychology, 22(1), 13–17. https://doi.org/10.1207/s15328023top2201_5
- King, A. (1998). Transactive peer tutoring: Distributing cognition and metacognition. Educational Psychology Review, 10(1), 57–74. https://doi.org/10.1023/A:1022858115001
- Kulgemeyer, C., & Peters, C. H. (2016). Exploring the explaining quality of physics online explanatory videos. European Journal of Physics, 37(6), 065705. https://doi.org/10.1088/0143-0807/37/6/06570
- Kyndt, E., Raes, E., Lismont, B., Timmers, F., Cascallar, E., & Dochy, F. (2013). A meta-analysis of the effects of face-to-face cooperative learning. Do recent studies falsify or verify earlier findings? Educational Research Review, 10, 133–149. https://doi.org/10.1016/j.edurev.2013.02.002
- Leahy, W., & Sweller, J. (2016). Cognitive load theory and the effects of transient information on the modality effect. Instructional Science, 44(1), 107–123. https://doi.org/10.1007/s11251-015-9362-9
- Loibl, K., Roll, I., & Rummel, N. (2017). Towards a theory of when and how problem solving followed by instruction supports learning. Educational Psychology Review, 29(4), 693–715. https://doi.org/10.1007/s10648-016-9379-x
- Mayer, R. E. (1996). Learning strategies for making sense out of expository text: The SOI model for guiding three cognitive processes in knowledge construction. Educational Psychology Review, 8(4), 357–371. https://doi.org/10.1007/BF01463939
- Mayer, R. E. (2005). Cognitive theory of multimedia learning. Cambridge Handbook of Multimedia Learning, 41, 31–48.
- Mayer, R. E., & Chandler, P. (2001). When learning is just a click away: Does simple user interaction foster deeper understanding of multimedia messages? Journal of Educational Psychology, 93(2), 390–397. https://doi.org/10.1037/0022-0663.93.2.390
- Mayer, R. E., Fiorella, L., & Stull, A. (2020). Five ways to increase the effectiveness of instructional video. Educational Technology Research and Development, 68(3), 837–852. https://doi.org/10.1007/s11423-020-09749-6
- Mayer, R. E., & Moreno, R. (1999). A cognitive theory of multimedia learning: Implications for design principles. Journal of Educational Psychology, 91(2), 358–368. https://esoluk.co.uk/calling/pdf/chi.pdf https://doi.org/10.1037/0022-0663.91.2.358
- Mazziotti, C., Rummel, N., Deiglmayr, A., & Loibl, K. (2019). Probing boundary conditions of productive failure and analyzing the role of young students’ collaboration. NPJ Science of Learning, 4(1), 2. https://doi.org/10.1038/s41539-019-0041-5
- Musstewissen Physik. (2017). Licht und Schatten I Optik I musstewissen Physik [Video]. YouTube. https://www.youtube.com/watch?v=ljEOxXbff2c
- Muthén, L. K., Muthén, B. O. (2015). Mplus (Version 8.3) [Computer software]. Muthén & Muthén. https://www.statmodel.com/index.shtml
- Nachtigall, V., Serova, K., & Rummel, N. (2020). When failure fails to be productive: Probing the effectiveness of productive failure for learning beyond STEM domains. Instructional Science, 48(6), 651–697. https://doi.org/10.1007/s11251-020-09525-2
- Nokes-Malach, T. J., Richey, J. E., & Gadgil, S. (2015). When is it better to learn together? Insights from research on collaborative learning. Educational Psychology Review, 27(4), 645–656. https://doi.org/10.1007/s10648-015-9312-8
- Paik, E. S., & Schraw, G. (2013). Learning with animation and illusions of understanding. Journal of Educational Psychology, 105(2), 278–289. https://doi.org/10.1037/a0030281
- Persike, M. (2020). Videos in der Lehre: Wirkungen und Nebenwirkungen [Videos in teaching: Effects and side effects]. In H. Niegemann & A. Weinberger (Eds.), Handbuch Bildungstechnologie (pp. 271–301). Springer. https://doi.org/10.1007/978-3-662-54368-9_23
- Poquet, O., Lim, L., Mirriahi, N., & Dawson, S. (2018). Video and learning: A systematic review (2007–2017) [Paper presentation]. Proceedings of the 8th International Conference on Learning Analytics and Knowledge (pp. 151–160). https://doi.org/10.1145/3170358.3170376
- R Core Team. (2023). R (4.3.1): A language and environment for statistical computing. R Foundation for Statistical Computing. https://www.R-project.org
- Rat für kulturelle Bildung e.V. (2019). Jugend/YouTube/Kulturelle Bildung. Horizont 2019: Studie: Eine repräsentative Umfrage unter 12-bis 19-jährigen zur Nutzung kultureller Bildungsangebote an digitalen Kultur-Orten [Youth/YouTube/Cultural Education. Horizon 2019: Study: A representative survey among 12- to 19-year-olds on the use of cultural education offerings at digital cultural venues]https://www.flipsnack.com/ratkulturellebildung/jugend-youtube-kulturelle-bildung-2019/full-view.html
- Sailer, M., Murböck, J., & Fischer, F. (2021). Digital learning in schools: What does it take beyond digital technology? Teaching and Teacher Education, 103, 103346. https://doi.org/10.1016/j.tate.2021.103346
- Salomon, G. (1984). Television is “easy” and print is “tough”: The differential investment of mental effort in learning as a function of perceptions and attributions. Journal of Educational Psychology, 76(4), 647–658. https://doi.org/10.1037/0022-0663.76.4.647
- Schnotz, W., & Rasch, T. (2005). Enabling, facilitating, and inhibiting effects of animations in multimedia learning: Why reduction of cognitive load can have negative results on learning. Educational Technology Research and Development, 53(3), 47–58. https://eric.ed.gov/?id=ej732685 https://doi.org/10.1007/BF02504797
- Schwartz, D. L. (1995). The Emergence of Abstract Representations in Dyad Problem Solving. Journal of the Learning Sciences, 4(3), 321–354. https://doi.org/10.1207/s15327809jls0403_3
- Schwartz, D. L., Chase, C. C., Oppezzo, M. A., & Chin, D. B. (2011). Practicing versus inventing with contrasting cases: The effects of telling first on learning and transfer. Journal of Educational Psychology, 103(4), 759–775. https://doi.org/10.1037/a0025140
- Schwartz, D. L., & Martin, T. (2004). Inventing to prepare for future learning: The hidden efficiency of encouraging original student production in statistics instruction. Cognition and Instruction, 22(2), 129–184. https://doi.org/10.1207/s1532690xci2202_1
- Sears, D. A., & Reagin, J. M. (2013). Individual versus collaborative problem solving: divergent outcomes depending on task complexity. Instructional Science, 41(6), 1153–1172. https://doi.org/10.1007/s11251-013-9271-8
- Sears, D. A. (2006). Effects of innovation versus efficiency tasks on collaboration and learning (Publication No. 3219375) [Doctoral dissertation]. Stanford University. ProQuest Information and Learning Company. https://www.proquest.com/dissertations-theses/effects-innovation-versus-efficiency-tasks-on/docview/304976770/se-2?accountid=14627
- Sinha, T., & Kapur, M. (2021). When problem solving followed by instruction works: Evidence for productive failure. Review of Educational Research, 91(5), 761–798. https://doi.org/10.3102/00346543211019105
- Slavin, R. E. (1983). When does cooperative learning increase student achievement? Psychological Bulletin, 94(3), 429–445. https://doi.org/10.1037/0033-2909.94.3.429
- Springer, L., Stanne, M. E., & Donovan, S. S. (1999). Effects of small-group learning on undergraduates in science, mathematics, engineering, and technology: A meta-analysis. Review of Educational Research, 69(1), 21–51. https://doi.org/10.3102/00346543069001021
- Stadler, M., Sailer, M., & Fischer, F. (2021). Knowledge as a formative construct: A good alpha is not always better. New Ideas in Psychology, 60, 100832. https://doi.org/10.1016/j.newideapsych.2020.100832
- Sweller, J. (2011). Cognitive load theory. In Psychology of learning and motivation (Vol. 55, pp. 37–76). Elsevier. https://doi.org/10.1016/B978-0-12-387691-1.00002-8
- Sweller, J. (2020). Cognitive load theory and educational technology. Educational Technology Research and Development, 68(1), 1–16. https://doi.org/10.1007/s11423-019-09701-3
- Tenenbaum, H. R., Winstone, N. E., Leman, P. J., & Avery, R. E. (2020). How effective is peer interaction in facilitating learning? A meta-analysis. Journal of Educational Psychology, 112(7), 1303–1319. https://doi.org/10.1037/edu0000436
- tenHove, P., & van der Meij, H. (2015). Like it or not: What characterizes YouTube’s more popular instructional videos? Technical Communication, 62(1), 48–62. http://www.ingentaconnect.com/content/stc/tc/2015/00000062/00000001/art00005
- Weaver, J. P., Chastain, R. J., DeCaro, D. A., & DeCaro, M. S. (2018). Reverse the routine: Problem-solving before instruction improves conceptual knowledge in undergraduate physics. Contemporary Educational Psychology, 52, 36–47. https://doi.org/10.1016/j.cedpsych.2017.12.003
- Webb, N. M., & Burnheimer, E. (2023). Cooperative and collaborative learning. In R. J. Tierney, F. Rizvi, & K. Ercikan (Eds.), International encyclopedia of education (4th ed., pp. 593–599). Elsevier. https://doi.org/10.1016/B978-0-12-818630-5.14070-9
- Wittwer, J., & Renkl, A. (2008). Why Instructional Explanations Often Do Not Work: A Framework for Understanding the Effectiveness of Instructional Explanations. Educational Psychologist, 43(1), 49–64. https://doi.org/10.1080/00461520701756420