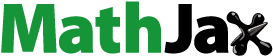
ABSTRACT
This study explored the relationships between voluntary online course enrollment (pre-pandemic), time poverty, and college outcomes. Results indicate that students who enrolled in at least one fully online course were significantly more time poor than other students; these differences were largely explained by age, parental status, and paid work. Yet, despite being more time poor, students who enrolled in online courses were more likely to successfully complete their courses, especially after controlling for time poverty. While students who took at least one online course were less likely to be retained in college and accumulated on average fewer credits, outcomes in online courses did not explain these differences; rather, other factors that make students both more likely to enroll online and to drop out or take fewer credits likely play a role. In particular, time poverty fully mediated the relationship between online enrollment and credit accumulation.
Even before most colleges moved instruction online during the COVID-19 pandemic, online learning had become a fundamental aspect of postsecondary education, with enrollments increasing annually in contrast to declining enrollments in higher education overall (Allen et al., Citation2016). A defining feature of online learning pre-pandemic is that generally students would voluntarily enroll in this modality (Gelles et al., Citation2020). Historically, students who enroll in online courses have had significantly different characteristics than students who only take courses face-to-face (e.g., they are more likely to be employed FT, older, and to have children [e.g., Cavanaugh & Jacquemin, Citation2015; Faidley, Citation2018; Johnson & Mejia, Citation2014). Prior studies have attempted to determine whether online course-taking impacts college outcomes. However, the research has produced mixed results; it is unclear whether enrolling in online courses has a positive, negative or no impact on college outcomes (e.g., Wladis et al., Citation2016; S. Jaggars, Citation2011; Shea & Bidjerano, Citation2014). Finding an answer to this question is even more critical since the pandemic, as enrollment in online courses has been further outpacing higher education enrollment overall (NC-SARA, Citation2021); institutions are grappling with the implications of this, uncertain about whether to maintain increased online offerings or to revert back to prioritizing face-to-face courses.
In this study, we investigated a relatively unexplored factor that may differentiate students who choose to enroll in online courses versus those who do not: time poverty (i.e., the extent to which students have insufficient time for their studies). Research already indicates that students who take online courses do so because they need the flexibility that these courses offer (Jaggars & Bailey, Citation2010; Pontes et al., Citation2010). This suggests that time (or a lack thereof) may be a critical issue. Time poverty may be both a potential mediator of online course enrollment and outcomes, and a potential equity factor distinguishing students who enroll in online courses from those who do not. This study (conducted prior to the COVID-19 pandemic) explored these possibilities.
Conceptual framework: Time poverty
When conceptualizing time as a finite resource (e.g., Giurge et al., Citation2020), time poverty has traditionally been defined as having insufficient time to maintain physical and mental well-being (Vickery, Citation1977). Translating this to the higher education context, Wladis et al. (Citation2018) define time poverty as insufficient time to devote to college work (i.e., lack of available time to maintain academic well-being). Thus we postulate that time poverty, a byproduct of demographic and environmental factors, reduces the quantity and quality of time that students have available for academic study, and that this lack of time simultaneously makes students more likely to enroll in online courses and to be at higher risk of poorer course and college outcomes (see, ).
Figure 1. Conceptual framework: time poverty and online enrollment as they relate to outcomes in higher education.
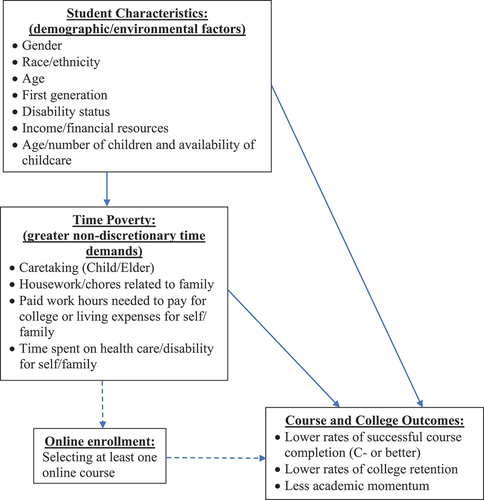
Connections between demographics, environmental variables, online enrollment and course/college outcomes have been supported by prior research (e.g., Johnson & Mejia, Citation2014; McPartlan et al., Citation2021). Research has also established links between both demographic and environmental variables and time poverty, as well as between time poverty and college outcomes (Conway et al., Citation2021; Wladis et al., Citation2018, Citationn.d.a, Citationn.d.b). However, links between online course enrollment and course and college outcomes is mixed (e.g., Authors, 2016; S. Jaggars, Citation2011; Shea & Bidjerano, Citation2014); one reason is that students who choose to enroll online have significantly different characteristics (e.g., work and family responsibilities) than those who do not (e.g., Cavanaugh & Jacquemin, Citation2015; S. S. Jaggars, Citation2014). Thus, time poverty may be a critical missing variable which explains connections between student characteristics, online enrollment, and course/college outcomes. outlines the known relationships (solid arrows) and hypothesized relationships (dotted arrows) investigated in this study.
Many characteristics that have been identified as more likely in students who enroll in online courses (e.g., female, older, family responsibilities, paid work) have also been associated with time poverty. Previous research shows that parents (especially mothers) have higher rates of time poverty than comparable childless peers (e.g., Chatzitheochari & Arber, Citation2012; Kalenkoski et al., Citation2011). Specific to higher education, Wladis et al. (Citation2018) report that student parents were significantly more time poor than comparable childless peers; this directly explained differences in academic momentum. Confirming with national data, Conway et al. (Citation2021) found that having dependents correlated with greater time poverty and lower quality academic time, particularly for mothers. Recent work (Wladis et al., Citationn.d.a) indicates that women, Black and Hispanic college students have significantly less time for their studies on average than men, White and Asian students. These differences correlated with age and were largely explained by the number of children that students had and their access to childcare. Further, Wladis et al. (Citationn.d.b) found that time poverty explained a significant proportion of differential college outcomes by gender and race/ethnicity. McPartlan et al. (Citation2021) report that groups (i.e., women, older students, working students) that choose online learning due to competing responsibilities had outcomes that indicated that their course performance may have suffered because of greater engagement in non-academic activities (e.g., commuting, caring for dependents) and commensurately lower engagement in academic activities. From our conceptualization, this finding suggests that these online students were likely experiencing, and impacted by, time poverty.
Research questions
This study focuses on the following research questions:
To what extent is time poverty more prevalent among students who choose to enroll in online courses versus those who do not?
(2) To what extent do differences in time poverty explain differences in course retention or successful course completion by online versus face-to-face enrollment?
(3) To what extent do differences in time poverty explain or mediate differences in college retention (re-enrollment) and academic momentum (credit accumulation) by online versus face-to-face enrollment?
(4) To what extent do differential outcomes in online versus face-to-face courses explain the relationship between online enrollment and college outcomes?
Literature review
Outcomes in online versus face-to-face courses
Many studies report that there are no positive or negative effects on course outcomes online versus face-to-face as measured by aggregate exams and course grades—supporting the “no significant difference” claim (e.g., Ashby et al., Citation2011; Cavanaugh & Jacquemin, Citation2015). Yet, research suggests that while aggregated data show no significant difference in outcomes between online and face-to-face courses, some studies have suggested that student characteristics moderate learning outcomes (gender, race/ethnicity, age and ability). Men, Latinx and Black students and those with lower GPAs have been found in a few studies to have poorer course outcomes online (lower grades/higher dropout) compared to face-to-face (Figlio et al., Citation2013; Xu & Jaggars, Citation2011b), while older students and women have been found in some studies to withdraw less and have higher grades in courses online compared to face-to-face (Gregory, Citation2016; C. W. Wladis et al., Citation2015; Wagner et al., Citation2011). Some scholars have theorized that taking courses online causes worse course outcomes which in turn lead to worse college outcomes (e.g., Smith, Citation2016; Xu & Jaggars, Citation2011a, Citation2011b).
Online course-taking and college outcomes
Online course-taking and college retention
Retention is defined here as re-enrollment at the university in the subsequent semester. In large-scale studies, students who took one or more online classes in their first semester were 4–5 percentage points less likely to return for the subsequent semester and students who took higher proportions of online courses were less likely to graduate or transfer to a senior college (Jaggars & Xu, Citation2010; Xu & Jaggars, Citation2011a, Citation2011b). Smith (Citation2016), using multi-institution data and controlling for student self-selection bias, also found an overall negative impact of online enrollment on course and college retention for four-year students.
However, other research that controlled for student characteristics has found opposite trends. Johnson and Mejia (Citation2014), assessing the California community colleges and controlling for demographics and G.P.A., found that while online students did poorly short-term, those who took at least some online courses were more likely to earn their degree or to transfer to a senior college than those who did not. Shea and Bidjerano (Citation2014), using national data and controlling for student characteristics, found that online course-taking helped students reach degree completion. James et al. (Citation2016), using multi-institutional data, found that students taking courses in both modalities had slightly better odds of being retained than students taking either face-to-face or online courses exclusively. For students who are not retained, it is also unclear whether online enrollment is the cause. As suggested in Wladis et al. (Citation2016) and James et al. (Citation2016), there may be characteristics that increase both a student’s likelihood of enrolling online and their odds of college dropout. Given the conflicting results in the literature, research is needed on factors that may correlate both with voluntary online enrollment and college retention.
Online course-taking and academic momentum
Academic momentum is the intensity with which students pursue their academic pathway; students who move through college at faster rates are more likely to complete their degrees than similar students who advance more slowly or have interrupted studies (Attewell et al., Citation2012; Jenkins & Bailey, Citation2017). Using nationally representative data, Attewell and Monaghan (Citation2016) report that students who initially attempted 15 credits (versus 12) were significantly more likely to earn their degree. Similarly, an international comparison of eight U.S. and two Russian universities found that higher academic momentum was associated with lower attrition (Kondratjeva et al., Citation2017). Other studies have shown that students who initially have high academic momentum in their major are more likely to earn their credential (Denley, Citation2016; Jenkins & Cho, Citation2014). High academic momentum has been shown to have particularly large benefits for members of racial/ethnic minority groups (Belfield et al., Citation2016) and can impact the college outcomes of low-income students (Davidson & Blankenship, Citation2017). There is more limited research about the relationship between online course enrollment and academic momentum, but some research suggests that students who choose to enroll in at least one online course may have higher academic momentum or credit ratios in comparison to students who are not enrolled in an online course (Galbraith & Mondal, Citation2018; James et al., Citation2016).
Student characteristics: Students who take courses online vs. those who do not
One of the major difficulties of attempting to assess the impact of online enrollment is that students have traditionally (pre-pandemic) self-selected into online courses.Footnote1 Students who voluntarily enroll in online courses are more likely to have non-traditional characteristics (Pao, Citation2016; Pontes et al., Citation2010), defined as: delayed enrollment (>age 24); no high school diploma; part-time enrollment; financially independent; have dependents; single parent status; or working full-time while enrolled (NCES, Citation2002). Using national data, Shea and Bidjerano (Citation2014) found an overrepresentation of online students in six out of these seven categories. Further, many studies have found that those who take online courses are more likely to be older (>24), to have family responsibilities, and to be employed, in comparison to face-to-face students (Ashby et al., Citation2011; Cavanaugh & Jacquemin, Citation2015; Driscoll et al., Citation2012; Faidley, Citation2018; Jaggars & Xu, Citation2010; Johnson & Mejia, Citation2014; McPartlan et al., Citation2021; Pao, Citation2016; Smith, Citation2016; Wilson & Allen, Citation2011; Xu & Jaggars, Citation2011a, Citation2011b). For students who have commitments such as jobs and family, it is the anywhere/anytime nature of online courses that makes them more desirable (S. S. Jaggars, Citation2014).
There is also strong evidence that a larger proportion of female students self-select into online courses (Ashby et al., Citation2011; Cavanaugh & Jacquemin, Citation2015; Faidley, Citation2018; Pao, Citation2016; Wilson & Allen, Citation2011). Jaggars and Xu (Citation2010), Johnson and Mejia (Citation2014), and Smith (Citation2016) and Xu and Jaggars (Citation2011a, Citation2011b), all using different multi-institution datasets, report that students in online courses are significantly more likely be female, which Shea and Bidjerano (Citation2014) confirmed using national data. McPartlan et al. (Citation2021) found that women were more likely than men to select online courses due to employment conflicts. We also note that student parents are more likely to be women, particularly women of color, and to be single mothers (Institute for Women’s Policy Research, Citation2019), which may in part explain the higher rates of online enrollment among women vs. men. McPartlan et al. (Citation2021) contends that demographic differences (e.g., age or gender) should not be considered as the cause of differential outcomes, rather the relationship between demographics and outcomes is likely mediated by other factors consequential for course performance. As posited in this study, one such mediator may be time poverty.
Method
Sample and data source
This study was conducted at the City University New York (CUNY), the third largest university system in the U. S. (Yale Daily News, Citation2013). More than three-quarters of CUNY’s 275,000 undergraduates identify as nonwhite; 42% are non-native English speakers; 45% are first generation college students; and 40% have household incomes under $20,000. CUNY is not nationally-representative; yet, its diverse student body and large online course portfolio make it a sound choice for exploring the relationship between online course taking, time poverty, and college outcomes, particularly among “non-traditional” college students.
The sample frame contained all students enrolled in any course at CUNY which had at least one fully online and one face-to-face section during a fall or spring term between fall 2015 to spring 2017; these courses came from two- and four-year campuses and were broadly representative of levels/disciplines. Students were invited to take part in an online survey during the term. Institutional records were combined with 41,574 student surveys, representing 34,081 unique students (some students took the survey in multiple terms). The response rate was 18%, more than double those of other official CUNY surveys (CUNY Student Experience Survey, Citation2014). Sample analysis indicates that it is roughly representative of the larger population (see, ). Because of the extremely large sample frame (), almost all minor differences were statistically significant: overall, students who completed the survey were comparable to those who did not, however, women were more likely to complete the survey (75% of respondents vs. 64% of non-respondents). Because there was adequate representation of both men and women and we controlled for gender throughout much of the analysis that follows, we do not consider this to be a major limitation.
Table 1. Summary statistics comparing survey sample to students in sample frame who did not complete the survey.
Researchers have increasingly called for more attention to be paid to survey representativeness, and less to response rates, as a growing body of research has found that response rates alone are poor measures of survey quality, and further, that large surveys with much lower response rates than the one used in this study can be just as representative as surveys with higher response rates. Fosnacht et al. (Citation2017) found that simulated response rates of 15–20% for sample frames of 1,000 students or more generated sample means whose correlation with those of a full survey sample with a larger response rate was 0.98–0.99.
Furthermore, weighting and multiple imputation were used to adjust for non-response bias at the student and survey question level, respectively. Weights for survey responses were based on the likelihood of responding to the survey by running a logistic regression model with a binary variable indicating whether the student submitted a completed survey as the dependent variable and all independent variables of interest as independent variables.Footnote2 Multivariate multiple imputation by chained equations imputed values for survey questions with missing responses, using all independent variables. Depending on variable type, logit models or predictive mean matching using three nearest neighborsFootnote3 (Schenker & Taylor, Citation1996) was used. A median of 3.7% of data were missing across imputed variables (excluding variables with no missing values). The final imputed dataset contained 15 imputations. All subsequent results reported here used the final imputed and weighted dataset.
Measures
Online courses were defined as all courses for which 80% of instruction was conducted online. In practice, almost all of these courses were 100% online. All other courses are classified as “not online,” but for ease of reading, we call these courses face-to-face. We also note that in this sample, almost all online courses are asynchronous. Thus, this study is comparing students who enrolled in at least one fully online (typically asynchronous) course to those who did not.
Both course and college outcomes were measured in this study. The main course-level outcome of interest is successful course completion, or whether the student completed the course with a C- grade or better, which is the criteria typically used to determine if a student will receive transfer credit or credit in their major for the course.Footnote4
College outcomes measured in this study were college retention (re-enrollment at CUNY in the subsequent regular semester, spring or fall) and academic momentum (number of credits earned in the regular session that term). We chose these measures because they are temporally close to the student’s reported time use (which may vary by term), and because they are also a significant predictor of long-term academic outcomes [e.g., transfer, degree completion] (DesJardins et al., Citation2006).
Time poverty was the primary independent variable in this study; this was operationalized as total reported non-discretionary time, or time spent on “necessary” life activities. Activities classified as “necessary” varies in the literature; we followed a model that classifies non-discretionary time as time spent on paid work, housework (all unpaid work necessary to sustain the household, except childcare), and childcare (Aas, Citation1978; Kalenkoski et al., Citation2011; Wladis et al., Citation2018). Our results describe the linear relationship between non-discretionary time and outcomes; models which included quadratic relationships were also investigated, but linear models fit the data better.
The survey asked students to report the number of hours they spent on different activities during a typical week that semester to record time use. Categories and descriptions in the survey were modeled after those found in the American Time Use Survey (ATUS; U.S. Bureau of Labor Statistics, Citation2020), but streamlined since that survey collects a much wider set of variables. Inputs for questions were restricted to numerical values that prevented students from inputting invalid values (such as negative values or more than 168 hours per week).
Control variables were included in the analyses to account for factors that may correlate strongly with online enrollment, time poverty, and educational outcomes. They included: gender, race/ethnicity, age, G.P.A., median household income of the student’s zip code, first-generation college student status, disability status, and whether the student was a first-time freshman. We present two types of models: base models (including only the independent and dependent variables of interest) and full models (containing other covariates). The base models best describe patterns for groups (e.g., online students) as they currently are, and are therefore particularly relevant to the design of policies and interventions; whereas full models may give insight into how other factors correlate with both online enrollment and academic outcomes, although they may be more difficult to interpret because of complex confounding relationships (see, Foster, Citation2010). Formal mediation analysis also allowed us to explore some of these relationships more formally.
Equations and software packages used for analytical models
All statistical analyses used Stata: the mi command for MI data, the svy for survey-weighted data, logit for logistic regression, regress for linear regression, and the khb package for KHB decomposition.
For logit models (e.g., survey completion, retention as dependent variables), the equation was:
, with logit link
.
For linear regression (non-discretionary time, credits as dependent variables) the equation was:
For both equations, represent the independent variables (e.g., age, ethnicity), and
represents the difference between actual versus predicted probability (e.g., of retention) or between actual versus predicted values (e.g., non-discretionary hours) of the dependent variable for each student.
We also use mediation analysis to explore the extent to which the relationship between two variables (e.g., online enrollment and academic outcomes) can be explained by their joint relationship with a third mediator (M) variable (e.g., time poverty). This uses statistical methods to break up patterns of correlation between the independent variable (IV) (e.g., online enrollment) and the dependent variable (DV) (e.g., college outcomes) into two components: the direct “effect,” measuring the proportion of the relationship between the IV and the DV which cannot be explained by the mediator, and the indirect “effect,” measuring the proportion of the relationship between the IV and the DV which can be explained by the mediator (see Buis, Citation2010, for more details on mediation). We recognize that the language of mediation is often associated with causation, and this is an observational study for which causal inferences are not appropriate. Attempts to reword this study using other terminology seemed too inaccessible to non-methodologists, so we use the language of mediation but put the word “effect” (e.g., direct or indirect “effect”) into quotes. We also remind the reader throughout that these data do not support causal inferences; our goal with this approach is to strike a reasonable balance between rigor of terminology and accessibility.
For mediation analysis, we used the KHB decomposition method (Buis, Citation2010), which is a general decomposition method based on the traditional SEM framework. This model is preferable to others because it generates an indirect effect that is not distorted by the rescaling that occurs when a potential mediator variable that is correlated with the dependent variable is added to a nonlinear model, such as in logistic regression. In the KHB method (in linear regression) if represents the dependent variable,
the independent variable, and
is the potential mediator, then we consider the two equations:
Here represents the direct “effect” of
on
,
represents the total “effect” of
on
, and
is the indirect “effect” of
on
. In the KHB method, residuals of a linear regression of
on
are calculated:
And then is used in place of
in EquationEquation (1)
(1)
(1) that estimates the direct “effect” of
on
:
Because the addition of to the model adds only the component of
that is uncorrelated with , the residuals have the same standard deviation in EquationEquations (1)
(1)
(1) and (Equation4
(4)
(4) ), but the coefficient of
in EquationEquation (1)
(1)
(1) is the direct “effect,” whereas the coefficient of
in EquationEquation (4)
(4)
(4) is the total “effect.” While other methods have also been developed to address the problems of rescaling that occur during mediation analysis with logistic regression, Monte Carlo studies have shown that the KHB method always performs as well or better than these methods in terms of recovering the degree of mediation net of the impact of rescaling (see, Kohler et al., Citation2011).
Results
Time poverty by online enrollment
Students were classified as “no fully online” if none of the courses they enrolled in were fully online and as “some fully online” if they enrolled in at least one fully online course during the study period. The mean non-discretionary time commitments (in hours/week) by students’ course enrollment status is shown in .
Figure 2. Total non-discretionary time (Hours/Week) by course enrollment status (From weighted, imputed linear regression model; Error bars indicate 95% confidence interval).
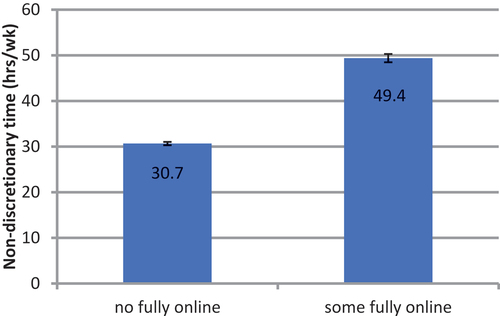
There are both significant and substantial differences in the time that students have available for college when we compared students who enrolled in at least one fully online course versus those who did not. To generate , linear regression was used to model total non-discretionary time (hours/week) as the dependent variable, and enrollment in at least one fully online course as the independent variable (see, ). Students who enrolled in at least one fully online course were significantly more time poor, with 18.7 more hours/week of non-discretionary time commitments on average than those who did not (p < .001). After including control variables, this difference was smaller but still substantial and significant at 11.8 hours/week (p < .001), indicating that controlling for or matching on many standard institutional research variables is likely not sufficient to fully account for differences in time poverty among online-vs.-face-to-face students. This suggests that prior studies that have compared students across mediums without controlling for time poverty have significant limitations, particularly given research which has shown that time poverty is strongly related to college outcomes for some groups such as student parents (Wladis et al., Citation2018). This relationship is explored in more depth later.
Table 2. Regression analysis of the relationship between online course enrollment and total non-discretionary time.
Exploring some potential explanations for differential rates of time poverty
One possible explanation for why students who voluntarily enroll in online courses may be more time poor (than those who do not) is that they are more likely to work, to have families, and to be older (Ashby et al., Citation2011; Cavanaugh & Jacquemin, Citation2015; Faidley, Citation2018; Johnson & Mejia, Citation2014; Pao, Citation2016; Wilson & Allen, Citation2011). Thus, to better understand the patterns from and , we break up non-discretionary time into three key categories: time spent on housework, paid work, and childcare, and compare differences in these subcategories between online and non-online students. was generated by separate weighted linear regression models which were used to predict the mean time spent separately on each category by enrollment status; we report p-values from those models here but have not included the models themselves because of space constraints.
Figure 3. Mean hours/week spent on childcare, paid work, and housework by enrollment status (From separate, weighted, imputed linear regression models).
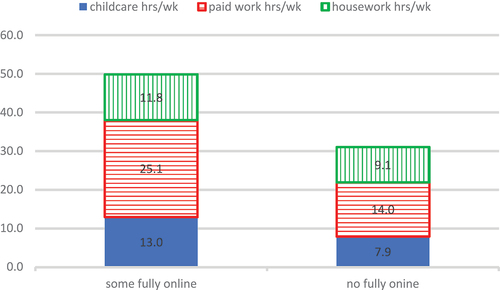
Students who enrolled in at least one online course spent significantly more time on each of these activities than those who did not (p < .001), although the relative magnitude of these differences varied. Work was the biggest difference, with students enrolled in at least one fully online course spending 80% more time compared to those who took no fully online courses during the study period. Childcare was the second biggest difference, with students in at least one fully online course spending 63% more time than those who only enrolled face-to-face. Housework showed the least difference, with students in at least one fully online course spending 39% more time than those who were not enrolled online.
Two major factors contributing to these differences are age and parental status. According to weighted linear regression models, for every additional year of age, a student’s non-discretionary time commitments went up by 1.5 hours/week, and this correlation is highly significant (p < .001).Footnote5 This is likely due to a complex host of factors, including needing to work and family care responsibilities. Using the same weighted linear regression models to predict differences in age by online enrollment, results indicate that students who enrolled in at least one fully online class were on average 4.4 years older than those who did not, which was significant (p < .001).
Likely one of the reasons that older students have more time poverty is that they are more likely to be parents. In this sample, students who enrolled in at least one fully online course had roughly twice as many children as those who did not (p < .001). To briefly test whether number of children mediates the differences in time poverty by online enrollment, the KHB method was used (see, ).
Table 3. Mediation analysis (KHB method) of the extent to which number of children mediates the relationship between voluntary online enrollment and time poverty, linear regression coefficients reported.
In , the total, direct, and indirect “effects” are all significant (p < .001) and controlling for number of children reduced the gap in non-discretionary time by online enrollment by 20.4%, from 18.7 to 14.9 hours/week (in full models, there is a similar pattern, but the number of children explains an even greater proportion of the gap). It makes sense that the number of children does not entirely explain the time poverty gap. For example, students enrolled in online courses are 7.5 percentage points more likely to be women, and even when women and men have the same number of children, women on average spend more time on childcare and housework (Chatzitheochari & Arber, Citation2012; Kalenkoski et al., Citation2011; Wladis et al., Citation2018). However, this does show that the number of children that a student had explains a substantial proportion of the differences in time available for college. There are likely also other explanations that contribute to differences in the time poverty of students who enroll in online courses versus those who do not; for example, students who elect to enroll in online courses are more likely to work full-time (Driscoll et al., Citation2012; Xu & Jaggars, Citation2011a), and often also have other complex life situations such as serious health issues of their own or an immediate family member (Wladis et al., Citation2020).
Course outcomes and time poverty
We next considered the relationship between enrollment in a fully online course, course outcomes, and time poverty (see, ).
Figure 4. Time poverty as a potential mediator between online course enrollment and course outcomes.
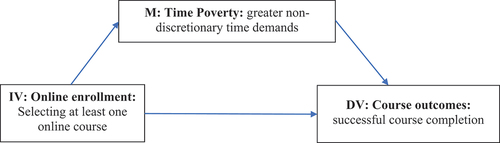
This relationship was explored using KHB mediation models (see, and ). In , the total, indirect, and direct “effects” are all significant, both in base and full models. In both cases, the direct “effect” was positive (i.e., online enrollment was correlated with higher rates of successful course completion; the size of this “effect” was smaller but still significant in full models, suggesting that some of this difference is explained by student characteristics such as GPA, gender, race/ethnicity, or median household income), but in both the base and full models, the indirect “effect” (i.e., the part of this relationship correlated with measures time poverty) was negative. Thus, the significantly higher rates of successful course completion among students enrolled in fully online courses may be lower than they would be if both students in fully online courses and those in courses that are not fully online had the same amount of time poverty, although the difference in these models is not very large.
Figure 5. Positive correlation between online course enrollment and course outcomes, suppression “effect” of time poverty as a mediator.
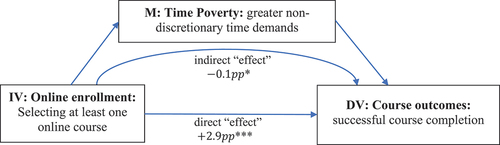
Table 4. Mediation analysis (KHB method) of the extent to which time poverty mediates the relationship between voluntary online enrollment and course outcomes, average partial effects reported.
College outcomes and time poverty
The relationship between fully online course enrollment, college outcomes, and time poverty was then considered (see, ).
Figure 6. Time poverty as a potential mediator between online course enrollment and course outcomes.
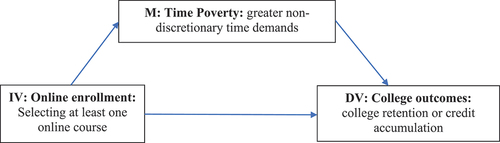
Figure 7. Time poverty as a significant partial mediator of the negative correlation between online course enrollment and college retention.
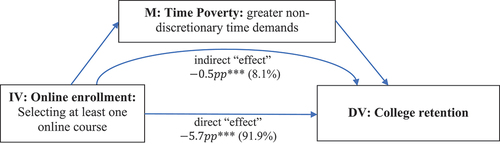
Figure 8. Time poverty as a significant complete mediator of the negative correlation between online course enrollment and academic momentum (Credit accumulation).
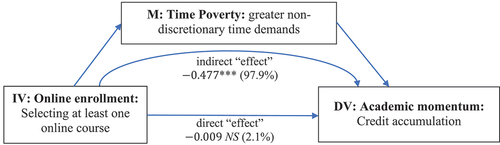
First, college retention (re-enrollment in the subsequent semester within CUNY) was explored: In , using KHB analysis, the total, direct, and indirect “effects” are all highly significant (p < .001).
A student who enrolled in a fully online course was roughly 6.2 percentage points less likely to re-enroll in college in the subsequent semester and roughly 7.9% of this difference was explained by the greater time poverty of students who enrolled in fully online courses (). Thus, time poverty explains a significant portion of the differences in college retention, but this is only a partial mediation and other factors also contribute to this retention gap (e.g., quality of time; measures of time not included in our measure of non-discretionary time such as eldercare or health care; stressors; etc.).
Second, the relationship between fully online course enrollment, academic momentum (credit accumulation), and time poverty was explored. In and , using KHB analysis, the total and indirect “effects” are highly significant (p < .001), but the direct “effect” is not significant in base models. Thus, in base models, enrolling online itself was not a predictor of earning fewer credits; rather any significant increased time poverty of students who voluntarily enrolled online entirely explained this difference. A student who enrolled in a fully online course earned on average about one half credit less than students who did not enroll in fully online courses, but this difference was 98.1% explained by their higher time poverty (i.e., non-discretionary time commitments). While this may not seem like a large difference, it could impact time-to-degree: for example, at the mean enrollment intensity observed in this study, students who enrolled in only face-to-face courses would be less than one credit shy of finishing in 11 semesters; students who enrolled in at least one online course would need 12 semesters. Time poverty appears to explain almost all of the differences in credit accumulation for students who took fully online courses versus those who did not.
Table 5. Mediation analysis (KHB method) of the extent to which time poverty mediates the relationship between voluntary online enrollment and college outcomes, linear regression coefficients and average partial effects reported.
Table 6. Mediation analysis (KHB Method) of the extent to which successful course completion mediates the relationship between voluntary online enrollment and college outcomes, linear regression coefficients and average partial effects reported.
In the full models, unlike in base models, the direct correlation between online course enrollment with credit accumulation was significant; the reader may wonder whether the outcomes of the courses in which students enrolled online may explain this lower credit accumulation. We note that this is not the only possible explanation. If there are other characteristics that simultaneously correlate with voluntary online course enrollment and lower credit accumulation, but which were not included in our model (e.g., stress, personal health, eldercare, lower time quality for college, etc.), this could also explain this difference. Interpreting more complex models with covariates can be particularly difficult with observational data (Foster, Citation2010). To investigate this further, we next explored whether our data are consistent with a hypothetical mediation model in which poorer outcomes in online courses might explain differences in college outcomes.
Do course outcomes explain differences in college outcomes?
Some scholars have theorized that students enrolled in fully online courses have worse college outcomes because of negative outcomes in fully online courses (Smith, Citation2016; Xu & Jaggars, Citation2011a, Citation2011b; see, ).
Figure 9. Successful course completion as a potential mediator between online course enrollment and college outcomes.
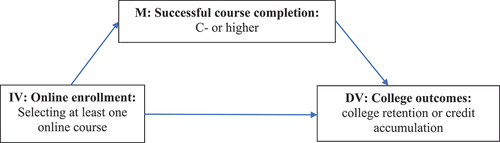
To investigate whether patterns in the data are consistent with this hypothesis, we analyzed the extent to which rates of successful course completion mediate the relationship between fully online course enrollment and college retention, using the KHB method (see ).
Figure 10. A negative correlation between online course enrollment and college retention which is not explained by course outcomes as a mediator (Significant suppression “effect”).
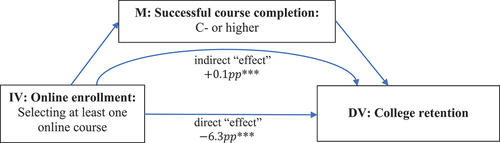
Figure 11. A negative correlation between online course enrollment and academic momentum (credit accumulation) which is not explained by course outcomes as a mediator (Significant suppression “effect”).
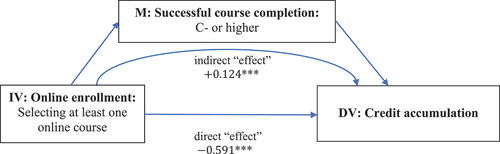
First, we consider college retention: In and the total, direct, and indirect “effects” are all significant in both the base and full models (p < .001); however, the indirect “effect” (of course outcomes on college retention) is positive while the direct “effect” (of online enrollment on college retention) is negative. Thus, while students who enroll in fully online courses were significantly less likely to re-enroll in college in the subsequent semester, this appears not to be the result of their completing online courses less successfully but rather the result of some other factors not included in our models.
Second, we investigated the extent to which rates of successful course completion mediate the relationship between fully online course enrollment and academic momentum: In and the total, direct, and indirect “effects” are all significant in both the base and full models (p < .001); but again, the indirect “effect” (of course outcomes on credit accumulation) is positive while the direct “effect” (of online enrollment on credit accumulation) is negative. Thus, while students who enroll in fully online courses earn on average fewer credits, this appears not to be the result of their completing online courses less successfully; rather, it again appears to be the result of some other factors not included in our models. Perhaps other life stressors or priorities that are not included in actual hours spent on non-discretionary tasks are the reason for these differences. For example, health problems, issues with quality or flexibility of time that are not reflected in quantity of available time, or mental health/stress levels, could all be correlated both with fully online course enrollment and college retention or academic momentum (Eisenberg et al., Citation2009; Suhrcke & De Paz Nieves, Citation2011), yet not necessarily be well-correlated with other variables routinely collected by institutional research.
Limitations
There is a possibility that the time measures in this study were impacted by desirability bias or students’ inaccurate recollections of time use because they were retrospective and self-reported. Hence, future studies seeking to replicate this work may seek to use alternate measures of time use (i.e., other approaches, such as the experience sampling method (e.g., Sonnenberg et al., Citation2012), may result in more accurate time use data). We also note that our measure of time poverty is incomplete. Our measures of non-discretionary time did not include time that students might have spent on eldercare or healthcare, and because of this, findings may actually underestimate the time poverty of some groups, and the relationship between time poverty and course or college outcomes. Future research is currently underway to address this limitation.
We note also that the CUNY student population includes a higher proportion of foreign-born students and ethnic/racial minorities, lower SES students, first-generation students, and students requiring developmental coursework, which may affect the sample’s national representativeness. However, this also makes it a particularly good sample for investigating trends for various traditionally underrepresented and understudied groups in higher education.
New York City has universal pre-kindergarten, which may have reduced the strength of the relationship between parental status, time poverty, and college outcomes for parents with children in this age range. Furthermore, New York City spends more on public benefits than any other U.S. municipality, and New York state provides a higher proportion of on-campus childcare than 47 other U.S. states (Eckerson et al., Citation2016). Thus, we note it is possible that regional policy factors could have influenced the results, and that the time poverty relationships in this study may underestimate national trends.
We also note that there is a complex but important relationship between income and time poverty that we have not investigated in depth here. Income poverty may have both a positive (the more hours one works, the less discretionary time one has) and a negative (the more money one has, the more one can afford childcare or other time-saving help thatincreases one’s discretionary time) relationship with time poverty. While in this study we have used some measures of income as controls in some models, this is insufficient to draw clear conclusions about whether the time poverty of a particular student is voluntary or the result of significant financial need. Some students may be time poor through choice (i.e., opting to enroll part-time because they have other priorities) and other students may be time poor because they lack the resources to pay for childcare or to work fewer hours. Traditional models of student need have often been insufficient in measuring the true financial need of students for college, particularly for more “nontraditional” groups (see Goldrick-Rab, Citation2016). Thus, better measures of financial need are necessary to properly investigate the complex relationship between time and income poverty; we aim to address this in future research.
Discussion
This study demonstrates for the first time using quantitative data on a large-scale dataset that students who voluntarily enroll online are on average significantly more time poor than those who do not, reinforcing and expanding on trends shown in prior qualitative research (Fox, Citation2017; McPartlan et al., Citation2021; S. S. Jaggars, Citation2014; Wladis et al., Citation2018). The time poverty of students in this study who enrolled in at least one online course was particularly striking: the mean amount of time spent on paid work, childcare, housework, and college work altogether totaled 79.6 hrs/wk, or the equivalent of roughly two full-time jobs.
Data from this study indicate that these differences in time poverty for online-versus-not-online students were largely explained by age and parental status, and also to related measures of time spent on both childcare and paid work. Students who enrolled in at least one online course were significantly older than those who did not, corroborating previous research (Driscoll et al., Citation2012; McPartlan et al., Citation2021; Smith, Citation2016; Wilson & Allen, Citation2011); and age was strongly correlated with time poverty. Additionally, students who enrolled in at least one fully online course had roughly twice as many children; spent 63% more time on childcare; and spent 80% more time working, compared to those who took no fully online courses; the number of children that a student had significantly mediated the relationship between online enrollment and time poverty. This confirms previous research that online learners are more likely to be parents and tend to be employed for more hours than their face-to-face counterparts (e.g., Driscoll et al., Citation2012; Xu & Jaggars, Citation2011a, Citation2011b). However, this study gives precise measures of the costs that online students face in terms of time available for college related to childcare and paid work, by quantifying their non-discretionary time commitments.
Despite being more time poor, students were more likely to successfully complete online courses, especially after controlling for time poverty. This supports Ashby et al. (Citation2011), who report better completion online. However, while course outcomes were positive, students who enrolled in at least one online course had lower academic momentum and were retained in college at lower rates than those who did not. Mediation models revealed that lower rates of retention and credit accumulation among online students could not be explained by outcomes in online courses. This suggests that differences in college outcomes cannot be explained by students completing online courses less successfully; rather other factors (e.g., time poverty, health issues, stressors, quality of time for college) that may make students simultaneously more likely to opt for online courses and also more likely to drop out or accumulate fewer credits likely play a role. Critically, time poverty mediated the relationship between online enrollment and college outcomes in our findings, explaining a significant but partial portion of the difference in retention and all of the significant difference in academic momentum.
Implications for research and practice
The COVID-19 pandemic necessitated a radical shift in higher education, accelerating the systematic adoption of online courses that had slowly been happening in the last decade, and now requiring institutions to re-think how they will offer courses in the “new normal” (Garcia-Morales et al., Citation2021). As institutions debate whether to keep increased online offerings or revert to pre-pandemic levels, a better understanding of who enrolls online and what impacts their success is critical. This study suggests that any such discussion, and future research and interventions, may need to consider student time poverty as a factor. For example, given the time poverty of many online students, and the time costs to attending class face-to-face (e.g., commute, lower flexibility of time; Gherhes et al., Citation2021), there might be unrecognized risks to policies that require or pressure students to enroll in face-to-face sections even when they would prefer to enroll online.
This study also revealed a major limitation in current supports for online students. While interventions to help retain online students often include technological or academic support, we know of no widespread interventions that aim to reduce the unequal time poverty of those who voluntarily enroll online versus those who do not. Yet, results from this study strongly suggest that any intervention aimed to improve the college outcomes of online students would do well to consider ways to measure and address time poverty. For example, supports which aim to reduce students’ paid work hours (e.g., providing more financial aid to cover living expenses), as well as affordable or free childcare for student parents, are promising candidates for future interventions to be tested through causal studies. Also, voluntary enrollment in at least one fully online course is a readily available variable in institutional datasets that could help institutions to identify students who are particularly time poor. Students in online courses are not the only ones who are time poor; however, this is one variable that could help with targeting interventions to provide the resource of time for those students in college who may need it the most.
Pre-pandemic, students who enrolled in at least one online course had 20 hrs/wk less time for college than other students yet spent only 4.9 hrs/wk less on their education. This may have mitigated some of the potential negative impacts of time poverty on course and college outcomes for these students, but the result is that online students had 15 hrs/wk less free time compared to other students, because they dedicated more time to their studies. This increased work may have other hidden negative consequences for time-poor students (e.g., less time for health care, eldercare, sleep, exercise or leisure, which has been associated with higher levels of stress/burnout Mathuews, Citation2018); this raises equity concerns. Future research should focus on measuring some of these potential hidden negative consequences of time poverty.
In higher education, time is often seen as an individual good free from constraint (Bennett & Burke, Citation2017); however, this view may not reflect the lived realities for students who have work and family commitments. Only 20% of student parents in this study said that the childcare available to them allowed them sufficient time for their studies, and just over three quarters of the students in this population who work do so in order to pay for living expenses (CUNY, Citation2018), so increased childcare and work hours among this population may largely be unvoluntary (i.e., many of these students might opt to spend more time on their studies if they could afford to do so). This suggests that there may be critical equity implications for any interventions aimed at improving the time poverty of online students, or other time-poor students in higher education.
Acknowledgments
This research was supported by grants from the National Science Foundation (Grant Nos. 1431649 and 1920599). Opinions reflect those of the authors and do not necessarily reflect those of the granting agency.
Disclosure statement
No potential conflict of interest was reported by the author(s).
Notes
1. During the COVID-19 pandemic, many students were forced online; however, this study focuses on voluntary online enrollment, the standard for decades prior to the pandemic, and likely the model that most colleges return to after the pandemic.
2. Propensity scores for the likelihood of survey completion were computed using logistic regression based on: age, gender, ethnicity, ESL status, college re-enrollment, remedial course placement, G.P.A, credits earned, online course-taking, and college level (community college, baccalaureate, graduate), and weights were normed to reflect the sample frame size.
3. For , Schenker and Taylor (Citation1996) found only small differences between
and
. Increasing the donor pool increases the risk of biased parameter estimates; thus we have chosen
.
4. We also explored patterns for course retention, but they were substantially similar to those for successful course completion, so because of space limitations we do not report them here.
5. We also considered non-linear representations of age, but a linear model fit the data better.
References
- Aas, D. (1978). Households and dwellings: New techniques for recording and analyzing data on time use. In W. Michelson (Ed.), Public policy in temporal perspective. Den Haag: Mouton. 191–205.
- Allen, I. E., Seaman, J., Poulin, R., & Straut, T. T. (2016). Babson Survey Research Group and Quahog Research Group, LLC. Online report card: Tracking online education in the United States. 1–4. http://onlinelearningsurvey.com/reports/onlinereportcard.pdf
- Ashby, J., Sadera, W. A., & McNary, S. W. (2011). Comparing student success between developmental math courses offered online, blended, and face-to-face. Journal of Interactive Online Learning, 10(3). https://eric.ed.gov/?id=EJ963670
- Attewell, P., Heil, S., & Reisel, L. (2012). What is academic momentum? And does it matter? Educational Evaluation and Policy Analysis, 34(1), 27–44. https://doi.org/10.3102/0162373711421958
- Attewell, P., & Monaghan, D. (2016). How many credits should an undergraduate take? Research in Higher Education, 57(6), 682–713. https://doi.org/10.1007/s11162-015-9401-z
- Belfield, C., Jenkins, P. D., & Lahr, H. E. (2016). Momentum: The academic and economic value of a 15-credit first-semester course load for college students in Tennessee. Community College Research Center. https://ccrc.tc.columbia.edu/publications/momentum-15-credit-course-load.html
- Bennett, A., & Burke, P. J. (2017). Re/conceptualizing time and temporality: An exploration of time in higher education. Discourse: Studies in the Cultural Politics of Education, 39(6), 1–13. https://doi.org/10.1080/01596306.2017.1312285
- Buis, M. L. (2010). Stata tip 87: Interpretation of interactions in nonlinear models. The Stata Journal, 10(2), 305–308. https://doi.org/10.1177/1536867X1001000211
- Cavanaugh, J. K., & Jacquemin, S. J. (2015). A large sample comparison of grade based student learning outcomes in online vs. face-to-face courses. Online Learning, 19(2), n2. https://doi.org/10.24059/olj.v19i2.454
- Chatzitheochari, S., & Arber, S. (2012). Class, gender and time poverty: A time‐use analysis of British workers’ free time resources. The British Journal of Sociology, 63(3), 451–471. https://doi.org/10.1111/j.1468-4446.2012.01419.x
- Conway, K. M., Wladis, C., & Hachey, A. C. (2021). Time poverty and parenthood: Who has time for college? AERA Open, 7, 233285842110116. https://doi.org/10.1177/23328584211011608
- CUNY (2014). The City University of New York 2014 student experience survey. The City University of New York. https://www.cuny.edu/wp-content/uploads/sites/4/page-assets/about/administration/offices/oira/institutional/surveys/SES_2014_Report_Final.pdf
- CUNY. (2018). 2018 Student experience survey: A survey of CUNY undergraduate students [Tableau presentation]. https://public.tableau.com/views/2018StudentExperienceSurvey/CoverPage?:language=en&:display_count=y&:origin=viz_share_link
- Davidson, J. C., & Blankenship, P. (2017). Initial academic momentum and student success: Comparing 4-and 2-year students. Community College Journal of Research and Practice, 41(8), 467–480. https://doi.org/10.1080/10668926.2016.1202158
- Denley, T. (2016). Choice architecture, academic foci and guided pathways. [Community College Research Center. https://postsecondarydata.sheeo.org/wp-content/uploads/2019/04/DenleyFinkJenkins_MetricsforGPReforms_SHEEO-State-Workshops-April-2019_DRAFT_040919.pdf
- DesJardins, S. L., Ahlburg, D. A., & McCall, B. P. (2006). The effects of interrupted enrollment on graduation from college: Racial, income, and ability differences. Economics of Education Review, 25(6), 575–590. https://doi.org/10.1016/j.econedurev.2005.06.002
- Driscoll, A., Jicha, K., Hunt, A. N., Tichavsky, L., & Thompson, G. (2012). Can online courses deliver in-class results? A comparison of student performance and satisfaction in an online versus a face-to-face introductory sociology course. Teaching Sociology, 40(4), 312–331. https://doi.org/10.1177/0092055X12446624
- Eckerson, E., Talbourdet, L., Reichlin, L., Sykes, M., Noll, E., & Gault, B. (2016). Childcare for parents in college: A state-by-state assessment (No IWPR #C445). Institute for Women’s Policy Research. https://iwpr.org/publications/child-care-for-parentsin-college-a-state-by-state-assessment/
- Eisenberg, D., Golberstein, E., & Hunt, J. B. (2009). Mental health and academic success in college. The B.E. Journal of Economic Analysis & Policy, 9(1), Article 40. http://www.bepress.com/bejeap/vol9/iss1/art40
- Faidley, J. (2018). Comparison of learning outcomes from online and face-to-face accounting courses [Electronic Theses and DissertationsPaper 3434]. https://dc.etsu.edu/etd/3434
- Figlio, D., Rush, M., & Yin, L. (2013). Is it live or is it Internet? Experimental estimates of the effects of online instruction on student learning. Journal of Labor Economics, 31(4), 763–784. https://doi.org/10.1086/669930
- Fosnacht, K., Sarraf, S., Howe, E., & Peck, L. K. (2017). How important are high response rates for college surveys? The Review of Higher Education, 40(2), 245–265. https://doi.org/10.1353/rhe.2017.0003
- Foster, E. M. (2010). Medicaid and racial disparities in health: The issue of causality. A commentary on Rose et al. Social Science & Medicine (1982), 70(9), 1271–1276. https://doi.org/10.1016/j.socscimed.2009.12.032
- Fox, H. L. (2017). What motivates community college students to enroll online and why it matters. Insights on equity and outcomes. Office of Community College Research and Leadership, 2017(19). https://files.eric.ed.gov/fulltext/ED574532.pdf
- Galbraith, D., & Mondal, S. (2018). The road to graduation: On-line or classroom. Research in Higher Education Journal, 35. https://files.eric.ed.gov/fulltext/EJ1194413.pdf
- Garcia-Morales, V. J., Garrido-Moreno, A., & Martin-Rojas, R. (2021). The transformation of higher education after the COVID disruption: Emerging challenges in an online learning scenario. Frontiers in Psychology. https://doi.org/10.3389/fpsyg.2021.616059
- Gelles, L. A., Lord, S. M., Hoople, G., Chen, D. A., & Mejia, J. A. (2020). Compassionate flexibility and self-discipline: Student adaptation to emergency remote teaching in an integrated engineering energy course during COVID-19. Education Sciences, 10(11), 304. https://doi.org/10.3390/educsci10110304
- Gherhes, V., Stoian, C. E., Farcasiu, M. A., & Stanici, M. (2021). E-learning vs. face-to-face learning: Analyzing students’ preferences and behaviors. Sustainability, 13(4381), 1–15. https://doi.org/10.3390/su13084381
- Giurge, L. M., Whillans, A. V., & West, C. (2020). Beyond material poverty: Why time poverty matters for individuals, organizations, and nations. Nature Human Behaviour, 4(10), 993–1003. https://doi.org/10.1038/s41562-020-0920-z
- Goldrick-Rab, S. (2016). Paying the price: College costs, financial aid, and the betrayal of the American dream. University of Chicago Press.
- Gregory, C. B. (2016). Community college student success in online versus equivalent face-to-facecourses [Electronic Theses and Dissertations]. Paper 3007. https://dc.etsu.edu/etd/3007
- Institute for Women’s Policy Research. (2019). Parents in college by the numbers: Today’s student parent population. https://iwpr.org/iwpr-issues/student-parent-success-initiative/parents-in-college-by-the-numbers/
- Jaggars, S. (2011). Online learning: Does it help low-income and underprepared students? (Assessment of evidence series). Community College Research Center. Teachers College, Columbia University. https://doi.org/10.7916/D82R40WD
- Jaggars, S. S. (2014). Choosing between online and face-to-face courses: Community college student voices. American Journal of Distance Education, 28(1), 27–38. https://doi.org/10.1080/08923647.2014.867697
- Jaggars, S., & Bailey, T. R. (2010). Effectiveness of fully online courses for college students: Response to a department of education meta-analysis. Community College Research Center. Teachers College, Columbia University. https://doi.org/10.7916/D85M63SM
- Jaggars, S., & Xu, D. (2010). Online learning in the Virginia community college system. Community College Research Center. Teachers College, Columbia University. https://doi.org/10.7916/D80V89VM
- James, S., Swan, K., & Daston, C. (2016). Retention, progression and the taking of online courses. Online Learning, 20(2), 75–96. https://olj.onlinelearningconsortium.org/index.php/olj/article/view/780/204
- Jenkins, P. D., & Bailey, T. R. (2017). Early momentum metrics: Why they matter for college improvement. Community College Research Center, Teachers College. https://files.eric.ed.gov/fulltext/ED572783.pdf
- Jenkins, D., & Cho, S. ‐. W. (2014). Get with the program … and finish it: Building guided pathways to accelerate student completion. New Directions for Community Colleges, 2013(164), 27–35. https://doi.org/10.1002/cc.20078
- Johnson, H. P., & Mejia, M. C. (2014). Online learning and student outcomes in California’s community colleges. Public Policy Institute. http://citeseerx.ist.psu.edu/viewdoc/download?doi=10.1.1.432.6575&rep=rep1&type=pdf
- Kalenkoski, C. M., Hamrick, K. S., & Andrews, M. (2011). Time poverty thresholds and rates for the US population. Social Indicators Research, 104(1), 129–155. https://doi.org/10.1007/s11205-010-9732-2
- Kohler, U., Karlson, K. B., & Holm, A. (2011). Comparing coefficients of nested nonlinear probability models. The Stata Journal, 11(3), 420–438. https://doi.org/10.1177/1536867X1101100306
- Kondratjeva, O., Gorbunova, E. V., & Hawley, J. D. (2017). Academic momentum and undergraduate student attrition: Comparative analysis in US and Russian universities. Comparative Education Review, 61(3), 607–633. http://dx.doi.org/10.1086/692608
- Mathuews, K. B. (2018). The working time-poor: Time poverty implication for working students’ involvement. [Doctoral dissertation, Ohio University]. OhioLINK Electronic Theses and Dissertations Center. http://rave.ohiolink.edu/etdc/view?acc_num=ohiou1540829773983031
- McPartlan, P., Rutherford, T., Rodriguez, F., Shaffer, J. F., & Holdton, A. (2021). Modality motivation: Selection effects and motivational difference in student who choose to take courses online. The Internet & Higher Education, 49(2021), 100793, 1–14. https://doi.org/10.1016/j.iheduc.2021.100793
- NCES. (2002). The condition of education 2002. U.S. Department of Education, National Center for Education Statistics. https://nces.ed.gov/pubs2002/2002025.pdf
- NC-SARA. (2021). NC-SARA ANNUAL DATA REPORT. Technical Report for Fall 2020 Exclusively Distance Education Enrollment & 2020 Out-of-State Learning Placements. https://nc-sara.org/sites/default/files/files/2021-10/NC-SARA_2020_Data_Report_PUBLISH_19Oct21.pdf
- Pao, T. C. (2016). Nontraditional student risk factors and gender as predictors for enrollment in college distance education. Chapman University Digital Commons. [Doctoral dissertation]. https://doi.org/10.36837/chapman.000009
- Pew Research Center . (2013). Closing the digital divide: Latinos and technology adoption. Pew Hispanic Center. https://assets.pewresearch.org/wp-content/uploads/sites/7/2013/03/Latinos_Social_Media_and_Mobile_Tech_03-2013_final.pdf
- Pontes, M. C., Pontes, N. M., Hasit, C., Lewis, P. A., & Siefring, K. T. (2010). Variables related to undergraduate students preference for distance education classes. Online Journal of Distance Learning Administration, 13(2). https://www.westga.edu/~distance/ojdla/summer132/pontes_pontes132.html
- Schenker, N., & Taylor, J. M. (1996). Partially parametric techniques for multiple imputation. Computational Statistics & Data Analysis, 22(4), 425–446. https://doi.org/10.1016/0167-9473(95)00057-7
- Shea, P., & Bidjerano, T. (2014). Does online learning impede degree completion? A national study of community college students. Computers & Education, 75, 103–111. https://doi.org/10.1016/j.compedu.2014.02.009
- Smith, N. D. (2016). Examining the effects of college courses on student outcomes using a joint nearest neighbor matching procedure on a state-wide university system. North Carolina State University. https://aefpweb.org/sites/default/files/webform/41/NicholeDSmith_ExaminingOnline.pdf
- Sonnenberg, B., Riediger, M., Wrzus, C., & Wagner, G. G. (2012). Measuring time use in surveys–concordance of survey and experience sampling measures. Social Science Research, 41(5), 1037–1052 https://doi.org/10.1016/j.ssresearch.2012.03.013.
- Suhrcke, M., & De Paz Nieves, C. (2011). The impact of health and health behaviours on educational outcomes in high-income countries: A review of the evidence. WHO Regional Office for Europe. https://www.euro.who.int/__data/assets/pdf_file/0004/134671/e94805.pdf
- U.S. Bureau of Labor Statistics. (2020). American time use survey [ATUS]. https://www.bls.gov/news.release/atus.toc.htm
- Vickery, C. (1977). The time-poor: A new look at poverty. Journal of Human Resources, 12(1), 27–48. https://doi.org/10.2307/145597
- Wagner, S. C., Garippo, S. J., & Lovaas, P. (2011). A longitudinal comparison of online versus traditional instruction. MERLOT Journal of Online Learning and Teaching, 7(1), 30–42. https://jolt.merlot.org/vol7no1/wagner_0311.pdf
- Wilson, D., & Allen, D. (2011). Success rates of online versus traditional college students. Research in Higher Education Journal, 14, 1–9. https://www.aabri.com/manuscripts/11761.pdf
- Wladis, C. W., Conway, K. M., & Hachey, A. C. (2015). The online STEM Classroom—Who succeeds? An exploration of the impact of ethnicity, gender, and non-traditional student characteristics in the community college context. Community College Review, 43(2), 142–164. https://doi.org/10.1177/0091552115571729
- Wladis, C., Conway, K. M., & Hachey, A. C. (2016). Assessing readiness for online education – Research models for identifying students at risk. Online Learning Journal, 20(3). https://olj.onlinelearningconsortium.org/index.php/olj/article/view/980
- Wladis, C., Conway, K. M., & Hachey, A. C. (2016). Assessing readiness for online education: Research models for identifying students at risk. Online Learning Journal, 20(3).
- Wladis, C., Hachey, A. C., & Conway, K. (2018). No time for college? An investigation of time poverty and parenthood. The Journal of Higher Education, 89(6), 807–831. https://doi.org/10.1080/00221546.2018.1442983
- Wladis, C., Hachey, A. C., & Conway, K. (2020). In S. K. Softic, D. Andone, & A. Szucs (Eds.). External stressors and time poverty among online students: An exploratory study. Proceedings of the EDEN 2020 Annual Conference. Timisoara, Romania: European Distance and E-Learning Network (EDEN), 172–183.
- Wladis, C., Hachey, A. C., & Conway, K. M. (n.d.a). It’s about time: The inequitable distribution of time as a resource for college, by gender and race/ethnicity.
- Wladis, C., Hachey, A. C., & Conway, K. M. (n.d.b). It’s about time, Part II: Does time poverty contribute to inequitable college outcomes by gender and race/ethnicity?
- Xu, D., & Jaggars, S. S. (2011a). The effectiveness of distance education across virginia’s community colleges: Evidence from introductory college-level math and English courses. Educational Evaluation and Policy Analysis, 33(3), 360–377. https://doi.org/10.3102/0162373711413814
- Xu, D., & Jaggars, S. S. (2011b). Online and hybrid course enrollment and performance in Washington state community and technical colleges. CCRC Working Paper No. 31, Community College Research Center, Columbia University. https://doi.org/10.7916/D8862QJ6
- Yale Daily News. (2013). Insider’s guide to colleges (39th ed.). St. Martin’s Griffin.