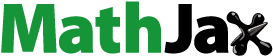
ABSTRACT
Nuclear data-induced uncertainties of infinite neutron multiplication factors (k∞) during fuel depletion are quantified in a single cell and a 3×3 multi-cell including burnable absorbers. Uncertainties of reaction cross sections, fission yields, decay half-lives and decay branching ratios provided in the JENDL libraries are taken into account. Hundred percent uncertainties are assumed to nuclear data to which uncertainty information are not provided in JENDL. Uncertainties propagation calculations are carried out with the adjoint-based procedure, and required sensitivity profiles of k∞ with respect to these nuclear data are efficiently calculated by the depletion perturbation theory. Covariance matrices for fission yields and decay data in a simplified burnup chain are successfully generated by the stochastic-based procedure. k∞ uncertainties of about 0.6% during fuel depletion are obtained, and it is shown that actinoids reaction cross sections are dominant contributors. Nuclide-wise decomposition of the uncertainties and observation of component-wise sensitivity profiles provide physical interpretations. By virtue of the adjoint-based procedure, several parametric surveys are also conducted. Contributions of uncertainties in fission products (FPs) nuclides are quantified, and important nuclides and energy ranges are identified for further evaluation of nuclear data of FP nuclides. Effect of cooling period on k∞ uncertainties is also discussed.
1. Introduction
Reliable and proper management of spent nuclear fuels is an important issue, and there are various aspects of it. One of the most important aspects is criticality safety; systems consisting of spent nuclear fuels should be kept in a sub-critical state in every probable conditions and situations. Spent nuclear fuels generally contain fission product (FP) nuclides, which are neutron absorbers, and less amount of fissile nuclides than fresh fuels, so reactivity of spent nuclear fuels should be much lower than that of fresh fuels. Thus, criticality management of spent nuclear fuels can be attained efficiently if one can properly consider this reactivity degradation. This concept is referred to as the burnup credit, and has been introduced to criticality management in several countries.
In order to predict the reactivity of spent nuclear fuels, one generally solves the eigenvalue equation describing neutron transport by computer codes, and the calculated eigenvalue corresponds to reactivity. Various kinds of macroscopic neutron reaction cross sections are introduced to the neutron transport equation, so one has to know accurate nuclear data (microscopic cross sections) in order to accurately predict reactivity. This dependence of reactivity on nuclear data is generally called as static dependence. On the other hand, nuclides number densities are also important when one considers systems consisting of spent nuclear fuels. Nuclides number densities of spent nuclear fuels are also predicted by computer codes, and nuclear data are used in these calculations. Thus, the calculated nuclides number densities depend on nuclear data, so reactivity of systems consisting of spent nuclear fuels depends indirectly on nuclear data via nuclides number densities. This dependence is called as depletion dependence. These clearly show that nuclear data are one of the most important parameters and quantities in criticality management of spent nuclear fuels.
To properly consider safety margin of design parameters related to criticality safety, uncertainty quantification of predicted reactivity is quite an important subject. Since nuclear data are evaluated from results of direct or indirect measurements and model calculations, these should have a certain degree of uncertainty. This nuclear data uncertainty is one of dominant sources in uncertainty of predicted reactivity. Nuclear data themselves and their uncertainty have been evaluated simultaneously, and both of them are stored in recent evaluated nuclear data files; so uncertainty propagation calculations from nuclear data to reactivity are required for uncertainty quantification of reactivity.
When one only considers static dependence of reactivity to nuclear data, uncertainty propagation calculations can be easily carried out with the adjoint-based procedure, in which sensitivity profiles of reactivity with respect to nuclear data are used. Static components of sensitivity profiles can be easily calculated by the classical perturbation theory. As described above, however, depletion dependence of reactivity on nuclear data should be also taken into account when systems consisting of spent nuclear fuels are considered. This depletion component of sensitivity profiles can be calculated by the depletion perturbation theory (DPT) [Citation1,Citation2], but it requires complicated numerical procedures and is computationally infeasible in calculations of large and complicated systems.
With this background and rapid advancement of computers, significant attentions have been paid to the stochastic-based procedure for uncertainty propagation calculations, and a lot of works with it have been carried out in recent years [Citation3–5]. This procedure can treat large and complicated systems, and is free from the first-order approximation generally introduced to the adjoint-based procedure. There are, however, several drawbacks in the stochastic-based procedure, such as inherent statistical uncertainties and relatively long computation time.
In comparison with the stochastic-based procedure, the adjoint-based procedure does not include any statistical uncertainties. Through various kinds of analyses with sensitivity profiles and component decomposition of total uncertainties, physical investigation and consideration on numerical results become possible. Thus, the adjoint-based procedure is still efficient and can be a complementary tool of the stochastic-based procedure.
Our research group has worked on the development of numerical tools for uncertainty quantification of reactor physics parameters which are related to nuclear fuel depletion. On depletion-related reactor physics parameters of light water reactors (LWRs), we have quantified those uncertainties induced by reaction cross sections for single cell problems [Citation6]. We have used a DPT-based numerical module implemented to a reactor physics code system CBZ, which is under development at our research group. This DPT-based module has been extended to multi-cell problems [Citation7], and DPT for fuel depletion calculations with the predictor-corrector method has been established [Citation8]. With this new capability of CBZ, it is possible to perform uncertainty quantification calculations for multi-cell problems including burnable absorbers, which are much more realistic than single cell problems.
In the present paper, we quantify nuclear data-induced uncertainty of infinite neutron multiplication factors (k∞) of an LWR multi-cell including burnable absorbers during depletion with a new DPT-based module of CBZ and the recent version of JENDL nuclear data libraries, and compare the obtained results with those in a single cell. Physical interpretation of numerical results is also attempted by virtue of specific feature of the adjoint-based procedure. Our previous work has considered uncertainties of only reaction cross sections of actinoid nuclides [Citation6], but the present work considers not only uncertainties of reaction cross sections but also those of fission yields and radioactive decay data. JENDL-4.0 does not contain any covariance information on FP nuclides [Citation9], so assumption of 10% uncertainty with full energy correlation is introduced, and effect of FP nuclides on nuclear data-induced uncertainty is quantified. For criticality safety management of spent nuclear fuels, one also should calculate k∞ uncertainties of systems after specific cooling period, so difference between results without any cooling and those with cooling is also discussed.
The present paper is organized as follows. Section 2 briefly describes the theoretical backgrounds of uncertainty quantification with the adjoint-based procedure. In the present study, we employ not an original detail burnup chain, in which all the nuclides given in evaluated nuclear data files are taken into account, but a simplified chain consisting of a limited number of nuclides, so covariance matrices specific for a simplified chain are required. Section 3 describes a procedure to generate covariance matrices of a simplified chain. Numerical conditions and results are presented in Section 4, and all these are summarized in Section 5.
2. Brief description of uncertainty quantification with the adjoint-based procedure
Here, we consider k∞ at burnup period i, k∞, i (i = 1, 2, …, I), and nuclear data σj (j = 1, 2, …, J). Sensitivity coefficient of k∞, i with respect to σj, Sij, is defined as
(1)
(1)
A derivative of k∞, i to σj can be decomposed into
(2)
(2)
where Ni, l is a number density of nuclide l at burnup i. The first term of the right hand side of Equation (Equation2
(2)
(2) ) is referred to as the static term and the second term is referred to as the depletion term in the present paper. Partial derivative of k∞, i to σj or Ni, l can be easily calculated by the classical perturbation theory, and that of Ni, l to σj is calculated by DPT. Interested readers on DPT can refer papers cited in the reference list.
Sensitivity coefficients can be written in a matrix form as
(3)
(3)
If we denote to a covariance matrix of nuclear data as Vσ, which is a J × J square matrix, an uncertainty propagation equation from σ to k∞ can be written as
(4)
(4)
where Vk is a covariance matrix of k∞, i, which is a I × I square matrix, and a superscript T is used for a transpose of matrix. This is the well-known sandwich formula, and this equation is used in the following calculations.
3. Covariance matrices generation for a simplified burnup chain
In the present calculations, we take into account uncertainties of the following nuclear data: reaction cross sections, fission yields, decay half-lives, and decay branching ratios. Those except reaction cross sections depend on used nuclear burnup chain. When all the nuclides given in evaluated nuclear data files are explicitly considered in a burnup chain, covariance data originally provided in evaluated files can be directly used. Generally, over 1000 FP nuclides are provided in evaluated files, so burnup calculations with a burnup chain consisting of all these nuclides require significant computational burden, and simplified burnup chains consisting of several tens or around 100 nuclides are generally preferred. When simplified burnup chains are used, covariance data of fission yields and decay data should be recalculated from original covariance data provided in evaluated nuclear data files.
Attentions should be paid on covariance data of fission yields and decay branching ratios when a simplified burnup chain is used. On fission yields, covariance data of independent fission yields can be applied when an original detail burnup chain is used. On the other hand, when a simplified burnup chain is used, covariance data of partially-cumulative fission yields, which are sum of independent fission yields of nuclides, which are positioned at an upstream chain and neglected in a simplified chain, should be prepared. shows a portion of an original burnup chain related to xenon-131 generation. If tellurium-131 is neglected in a simplified chain, fission yield of iodine-131 in this chain should be sum of independent yields of iodine-131 and tellurium-131. This is an example of partially-cumulative fission yields. On decay branching ratios, it should be emphasized that those uncertainties in original data can become those of decay branching ratios, fission yields, and reaction branching ratios in a simplified chain. As shown in , antimony-131 has two decay channels and each of branching ratios has its uncertainty. If antimony-131 is neglected in a simplified chain, uncertainties of decay branching ratios should be considered as uncertainties of partially-cumulative fission yields of ground and meta-stable states of tellurium-131. These uncertainties of partially-cumulative fission yields induced by antimony-131 decay branching ratios should be correlated among different fissile nuclides, so a unique fully-correlated covariance matrix of partially-cumulative fission yields induced by decay branching ratios should be prepared in uncertainty quantification calculations with a simplified chain.
Uncertainties of reaction branching ratios in a simplified chain induced by uncertainties of decay branching ratios in original data are also noted. shows a portion of original and simplified chains related to gadolinium-152 generation. Meta-stable state of europium-152 has two decay channels and each of branching ratios has its uncertainty. When this nuclide is neglected in a simplified chain, these uncertainties should be considered as uncertainties of (n,γ) reaction branching ratios of europium-151.
Figure 2. Example of reaction branching ratio uncertainty in a simplified chain induced by decay branching ratio uncertainty in an original chain.
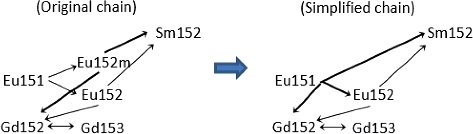
It is possible to analytically obtain covariance matrices of fission yields, decay branching ratios and reaction branching ratios in a simplified chain, but its derivation and implementation to a computer code might be cumbersome. In the present study, the stochastic-based procedure is adopted to these covariance data generation; sets of samples about burnup-related nuclear data are generated stochastically in accordance with their covariance data, simplified chains based on these nuclear data-sets are prepared, and then covariance matrices of the above nuclear data in a simplified chain are calculated.
4. Numerical results
4.1. Numerical procedure
All the calculations are carried out by a reactor physics code system CBZ. Numerical procedure of nuclear fuel depletion calculations with CBZ is briefly described below.
Resonance self-shielding calculations, i.e. effective cross sections calculations, are carried out in a single cell with reflective boundary conditions with the advanced Bondarenko method [Citation10]. A 107-group library based on JENDL-4.0 is used. The resonance self-shielding calculations yield 107-group cross sections which are used in subsequent neutron flux and fuel depletion calculations. Neutron transport equation is solved by a MEC module of CBZ, which is based on the method of characteristics for spatial and angle discretization. Nuclides depletion equation is solved by the matrix exponential method, and the mini-max polynomial approximation method is used to calculate the matrix exponential [Citation11]. A simplified burnup chain consisting of 138 FP nuclides, which are chosen by specific algorithm to reproduce number densities of some important nuclides after depletion [Citation12], is used. Fission yields data and radioactive decay data of FP nuclides are taken from an updated version of JENDL FP yields data file-2011(JENDL/FPY-2011) and FP decay data file-2011(JENDL/FPD-2011) [Citation13], in which nuclear data of over 1300 FP nuclides are evaluated. In calculations of a multi-cell including burnable absorbers, an optimally-weighted predictor-corrector method is adopted to reduce time discretization error [Citation14].
It has been widely recognized that there are strong negative correlations in independent fission yields among different nuclides belonging to a single mass chain, and these correlations have significant influence on uncertainty quantification calculations for nuclide number densities after depletion even though such correlation data are not provided in current evaluated nuclear data files [Citation15]. We apply the following method of estimating covariance among different nuclides in a single mass chain, which has been proposed by Devillers [Citation16]:
(5)
(5)
(6)
(6)
where
are covariance between fission yields of nuclides i and j, Vi is variance of fission yield of nuclide i originally given in JENDL/FPY-2011, VMY is variance of mass yield, and J denotes the number of nuclides in the same mass chain.
Since covariance data are not given for some nuclear data in JENDL/FPD-2011, we assume that these nuclear data have 100% relative uncertainty as a previous work about decay heat uncertainty calculations done by Katakura [Citation13]. Branching ratio uncertainties should have strong negative correlations among different decay paths of a single nuclide because of the normalization condition. This is also taken into consideration.
4.2. Problem specifications
A PWR-simulated UO2 fuel single cell and a 3×3 multi-cell consisting of this single cell with reflective boundary conditions are considered. Geometric configuration of the multi-cell is shown in .
Uranium-235 enrichment of the UO2 fuel pins is 3.4 wt%. Geometric specification and initial nuclide number densities are taken from the reference [Citation17]. At the center position of the multi-cell, a gadolinium-bearing UO2 fuel pin, whose gadolinium concentration is 10 wt% and uranium-235 enrichment is 2.63 wt%, is located. This gadolinium-bearing pin is divided into eight ring-type regions, and nuclides depletion calculations are carried out in each region. On the other normal fuel pins (pin 2 and pin 3), a whole fuel pellet region is treated as one depletion region. The same condition is adopted to a single cell calculation. Neutron flux is normalized by linear power density per one fuel pin of 179 W/cm. Infinite neutron multiplication factors during fuel depletion of a single cell and a 3×3 multi-cell are shown in .
4.3. Verification of covariance matrices for a simplified burnup chain
In order to verify covariance matrices for a simplified chain generated by the above-described procedure, variance of nuclides number densities after fuel depletion is calculated by a simplified chain and its covariance matrices. Reference values of variance are obtained from sensitivity profiles calculated with an original detail chain and covariance data given in the evaluated nuclear data. A simplified chain consisting of 138 FP nuclides has been proposed so as to reproduce number densities of 33 FP nuclides, which have relatively large impact on reactivity [Citation12], so number densities of these 33 FP nuclides are concerned in this verification calculation. Three sets of covariance matrices of a simplified chain are generated from a 100, 1000, and 10,000 samples. shows root-mean-square (RMS) and maximum relative errors in variance of number densities after 40 GWD/t of burnup in the single cell. Number densities of nuclide in the parenthesis give the maximum error in variance. Variances are separately evaluated as fission yield(FY)-induced one and decay branching ratio (DBR)-induced one. On fission yield-induced variance, RMS and maximum relative errors of simplified chain results become small as the number of samples increases. On the other hand, maximum relative error of decay branching ratio-induced variance is still large (7.6%) even if 10,000 samples are used. Through further investigation on this large error observed in xenon-131 number density, it has been found that uncertainty induced by decay branching ratio of antimony-131 is not reproduced well with a simplified chain. In our procedure of simplified burnup chain construction, unimportant nuclides, which are neglected in a simplified chain, are identified by observing adjoint number densities (or important functions) of these nuclides to target quantity. However, antimony-131 is neglected in the present simplified chain because of similarity in adjoint number density between antimony-131 and tellurium-131, which is different criteria in our important nuclides identification process [Citation12]. We do not yet sufficiently understand the reason why antimony-131 branching ratio-induced variance is not well reproduced. Note that relative standard deviation in xenon-131 number density induced by decay branching ratio is about 0.003%, so impact of this relatively large error of 7.6% is insignificant. The second largest maximum error induced by decay branching ratio is 0.028 in variance of Mo-95 number densities. In our calculations, covariance matrices generated from 10,000 samples are used.
Table 1. Root-mean-square (RMS) and maximum relative errors in variance of number densities after fuel depletion
In order to further verify our procedure of covariance data generation, antimony-131 is added to the FP-138 chain and the same uncertainty propagation calculations are carried out. Results of this FP-139 chain are also shown in . Maximum errors in variance induced by decay branching ratios become small as the number of samples increases. This result also verifies our procedure to generate covariance matrices of a simplified burnup chain.
4.4. Uncertainty quantification of neutron multiplication factors during nuclear fuel depletion
4.4.1. Comparison between a single cell and a multi-cell including burnable absorber
Nuclear data-induced uncertainties of k∞ during fuel depletion of a single cell and a 3×3 multi-cell are shown in . Although slight difference is observed between these two uncertainties around 20 GWD/t of burnup, uncertainties and their dependence on burnup are similar to each other. shows correlation matrix of these uncertainties; this matrix has a block structure and the left-bottom and right-top blocks are sub-matrices about that of a single cell and that of a multi-cell, respectively, and non-diagonal sub-matrices are correlation between these two components.
Figure 5. Nuclear data-induced uncertainty of infinite neutron multiplication factors during fuel depletion.
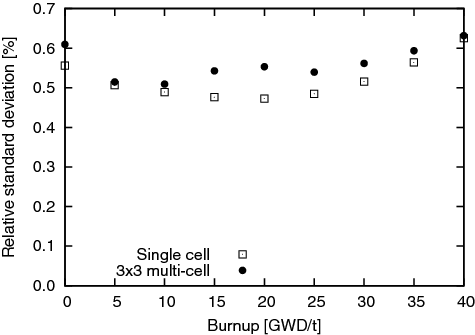
Figure 6. Correlation matrices of nuclear data-induced uncertainty of infinite neutron multiplication factors during fuel depletion.
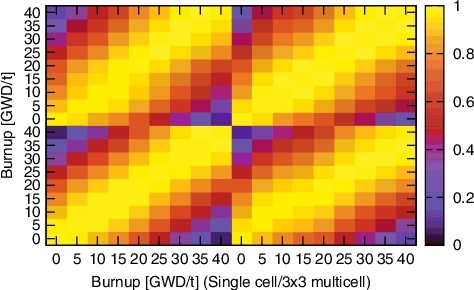
Strong positive correlation between uncertainties of the single cell and the multi-cell at same burnup is observed. Strong correlations among uncertainties at different burnup periods are also observed.
Nuclear data-wise uncertainties are also calculated, and it is found that reaction cross sections of actinoids are dominant contributors to total uncertainties, and that other components such as fission yields and decay data are negligible. Uncertainties of k∞ induced by fission yield, half-life, and decay branching ratio uncertainties are shown in . Among them, fission yield-induced uncertainties are the largest, but those are less than 0.03% and much less than reaction cross section-induced uncertainties. These small fission yield-induced uncertainties are consistent with the previous works based on the stochastic-based procedure [Citation15,Citation18].
shows nuclide-wise reaction cross section-induced uncertainties of k∞ during fuel depletion. In this figure, contributions of five dominant nuclides are presented. With burnup, uranium-235-induced uncertainties decrease and plutonium isotopes-induced uncertainties increase because of changes in their inventories. Decreases of uranium-238-induced uncertainties with burnup can be easily understood by observing sensitivity profiles during fuel depletion. shows energy-integrated sensitivities of k∞ to uranium-238 capture cross section during depletion. Static terms of sensitivity are constantly negative, but positive depletion terms increase with burnup. This is due to conversion effect from uranium-238 to plutonium-239 via neutron capture reaction of uranium-238; increase of uranium-238 capture cross section promotes nuclear fuel conversion and makes k∞ large. This is positive contribution to sensitivity, and cancels static contribution out. In k∞ uncertainties in the multi-cell, slight fluctuation is observed around 15 GWD/t, and this can be interpreted as follows; if uranium-238 capture cross section is increased, this makes plutonium-239 inventories large because of promotion of conversion. Plutonium-239 absorbs thermal neutrons strongly, so neutron flux energy spectrum shifts to high energy and thermal neutron captures by gadolinium-155 and -157 are reduced. Thus, these gadolinium isotopes still remain in a fuel, so k∞ becomes small. This is negative contribution to sensitivity and this makes dips in the depletion term around 15 GWD/t in a 3×3 multi-cell as shown in .
4.4.2. Effect of uncertainty of neutron capture cross sections of fission product nuclides
In the preceding calculations, uncertainties of reaction cross sections of FP nuclides have not been considered since no evaluations have been made on covariance data of these nuclides in JENDL-4.0. However, reaction cross sections of FP nuclides should be uncertain and those must be taken into account to obtain more reliable uncertainty of k∞. Here, we assume fully-energy-correlated 10% uncertainty to neutron capture cross sections of all the FP nuclides considered in our simplified chain. It is reported in Ref. [Citation19] that calculation-to-experimental values about material sample worth of several FP such as Mo-95 and Ru-101 range from 0.87 to 1.17, so the present assumption of 10% uncertainty is realistic. In order to distinguish contributions of burnable absorbers from FP nuclides, another case in which 10% uncertainties are assumed to all the FPs except gadolinium-155 and -157 is also prepared. Uncertainties of k∞ induced by capture cross sections of FP nuclides are shown in . Uncertainties of k∞ reach about 0.2% at 40 GWD/t in both cases. Those uncertainties are much smaller than those induced by reaction cross sections of actinoids. In the multi-cell, fluctuations in k∞ uncertainties are observed around 15 GWD/t. shows energy-integrated sensitivities of k∞ to gadolinium-155 and −157 capture cross sections. Like k∞ sensitive to uranium-238 capture cross sections, cancellation between static and depletion terms can be observed. Depletion term is positive because increase in capture cross sections of these gadolinium isotopes enhances depletion of these isotopes. Observed burnup dependence of k∞ uncertainty in the multi-cell can be understood from these sensitivity profiles with burnup.
Figure 11. Energy-integrated sensitivity of k∞ in 3 x 3 multi-cell with respect to gadolinium-155 and −157 capture cross sections.
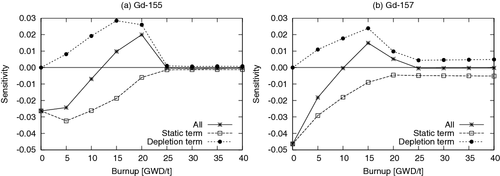
In order to identify FP nuclides that are important in uncertainty quantification of k∞ during fuel depletion, energy-integrated sensitivities of k∞ to capture cross sections during depletion are shown in . Ten FP nuclides, whose sensitivities are large at 40 GWD/t, are chosen here. In addition to energy-integrated sensitivities, energy spectra of sensitivities at 40 GWD/t are also shown in . These information might be important for future evaluation of covariance data of FP nuclides nuclear data.
4.4.3. Effect of cooling time
Reactivity of spent nuclear fuels change with cooling period because of radioactive decay of unstable nuclides. Here, k∞ uncertainties after several different burnup and cooling periods of 5 and 10 years are calculated. shows nuclear data-induced uncertainties of k∞. Note that uncertainties of capture cross sections of FP nuclides are not considered. Changes in k∞ uncertainties due to different cooling period are insignificant when JENDL-4.0 is used. These changes are due to radioactive decay of plutonium-241. shows k∞ uncertainties induced by plutonium-241 and americium-241 nuclear data. Because of decay of plutonium-241 with half-life of 14.29 years, contribution of plutonium-241 decreases and that of americium-241, which is daughter nuclide of plutonium-241, increases as cooling period increases. Gradients of k∞ uncertainties to burnup are steeper in plutonium-241 than in americium-241. This difference can be understood by observing energy-integrated sensitivities of k∞ to capture cross sections of these nuclides, which are shown in . Because most of americium-241 is generated during cooling period, depletion term is small in sensitivity to americium-241 capture cross section.
Figure 16. Energy-integrated sensitivity of k∞ after 5-year cooling with respect to capture cross sections of plutonium-241 and americium-241.
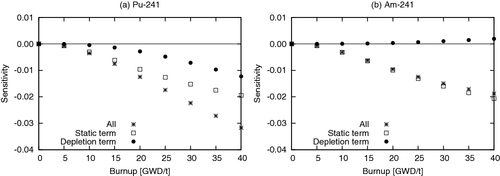
Contributions of capture cross sections of FP nuclides are also considered. shows k∞ uncertainties after specific cooling period induced by FP nuclides nuclear data. Fully-energy-correlated 10% uncertainty is assumed as the preceding section. shows energy-integrated sensitivity of k∞ to capture cross sections of xenon-135, gadolinium-155 and europium-155. When cooling period is considered, xenon-155 contribution becomes small because of its short half-life of 9.14 hours. Also, due to radioactive decay of europium-155 with half-life of 4.753 years, gadolinium-155, which is strong neutron absorber, is produced and this contributes to total uncertainties.
5. Conclusion
We have quantified nuclear data-induced uncertainties of infinite neutron multiplication factors during fuel depletion in a single cell and a 3×3 multi-cell including burnable absorbers. Uncertainties of reaction cross sections of actinoids and FP nuclides, fission yields, decay half-lives, and decay branching ratios have been taken into account. Uncertainties propagation calculations have been carried out with the adjoint-based procedure, and required sensitivity profiles of infinite multiplication factors with respect to these nuclear data have been efficiently calculated by the DPT. Covariance matrices for fission yields and decay data in a simplified chain have been successfully generated by the stochastic-based procedure. Infinite neutron multiplication factors uncertainties of about 0.6% have been obtained during fuel depletion, and it has been shown that actinoids reaction cross sections are dominant contributors. Nuclide-wise decomposition of the uncertainties and observation of component-wise sensitivity profiles have provided physical interpretations.
By virtue of the adjoint-based procedure, several parametric surveys have also been conducted. Contributions of uncertainties in FPs nuclides have been quantified, and important nuclides and energy ranges have been identified for further evaluation of nuclear data of FP nuclides. Effect of cooling period on infinite multiplication factor uncertainties has been also discussed.
Acknowledgments
This work is supported by the secretariat of the nuclear regulation authority of Japan.
Disclosure statement
No potential conflict of interest was reported by the authors.
References
- Gandini A. A method of correlation of burnup measurements for physics prediction of fast power-reactor life. Nucl Sci Eng. 1969;38:1–7.
- Williams ML. Development of depletion perturbation theory for coupled neutron/nuclide fields. Nucl Sci Eng. 1979;70:20–36.
- Kawano T, Hanson KM, Frankle S, et al. Evaluation and propagation of the 239Pu fission cross-section uncertainties using a Monte Carlo technique. Nucl Sci Eng. 2006;153:1–7.
- Rochman D, Koning AJ, van der Marck SC. Uncertainties for criticality-safety benchmarks and keff distributions. Ann Nucl Energy 2009;36:810–831.
- Yamamoto A, Kinoshita K, Watanabe T, et al. Uncertainty quantification of LWR core characteristics using random sampling method. Nucl Sci Eng. 2015;181:160–174.
- Chiba G, Tsuji M, Narabayashi T. Uncertainty quantification of neutronic parameters of light water reactor fuel cells with JENDL-4.0 covariance data. J Nucl Sci Technol. 2013;50:751–760.
- Chiba G, Kawamoto Y, Narabayashi T. Development of a fuel depletion sensitivity calculation module for multi-cell problem in a deterministic reactor physics code system CBZ. Ann Nucl Energy. 2016;96:277–286.
- Chiba G. Perturbation theory for nuclear fuel depletion calculations with predictor-corrector method. J Nucl Sci Technol. 2018;55:290–300.
- Shibata K, Iwamoto O, Nakagawa T, et al. JENDL-4.0: a new library for nuclear science and technology. J Nucl Sci Technol. 2011;48:1–30.
- Chiba G, Narabayashi T. Advanced Bondarenko method for resonance self-shielding calculations in deterministic reactor physics code system CBZ. Ann Nucl Energy. 2016;96:277–286.
- Kawamoto Y, Chiba G, Tsuji M, et al. Numerical solution of matrix exponential in burn-up equation using mini-max polynomial approximation. Ann Nucl Energy. 2015;80:219–224.
- Chiba G, Tsuji M, Narabayashi T, et al. Important fission product nuclides identification method for simplified burnup chain construction. J Nucl Sci Technol. 2015;52:953–960.
- Katakura J. JENDL FP decay data file 2011 and fission yields data file 2011. Ibaraki, Japan: Japan Atomic Energy Agency; 2011. JAEA-Data/Code 2011-025.
- Okumura S, Chiba G. Development of nuclear fuel depletion calculation capability for LWR fuel assembly in reactor physics code system CBZ. Proceedings of Reactor Physics Asia 2017; 2017 Aug 24–25; Chengdu, China.
- Cabellos O, Piedra D, Diez CJ. Impact of the fission yield nuclear data uncertainties in the pin-cell burn-up OECD/NEA UAM benchmark. Proceedings of the International Conference on Physics of Reactors; 2014 Sep 28-Oct 3; Kyoto, Japan.
- Devillers C. The importance of fission product nuclear data in reactor design and operation. Petten: IAEA Panel; 1977.
- Katakura J, Kataoka M, Suyama K, et al. [A set of ORIGEN2 cross section libraries based on JENDL-3.3 library: ORLIBJ33]. Japan Atomic Energy Research Institute; 2004. JAERI-Data/Code 2004-015. Japanese.
- Fiorito L, Stankovskiy A, Van den Eynde G, et al. Generation of fission yield covariances to correct discrepancies in the nuclear data libraries. Ann Nucl Energy. 2016;88:12–23.
- Aliberti G, Palmiotti G, Salvatores M, et al. Nuclear data sensitivity, uncertainty and target accuracy assessment for future nuclear systems. Ann Nucl Energy. 2006;33:700–733.