Abstract
A new class of predictor–corrector integration methods intended for molecular dynamics simulation is presented. The methods are derived from the original Gear methods by analysing the numerical solution of the harmonic oscillator. The corrector coefficients are chosen to improve the time-reversibility while keeping maximum stability. Tests performed on systems with forces not dependent on velocities (classical two-body problem and three simple atomistic systems) show good energy conservation and reduced deviations of measured quantities in comparison with the Verlet method. As regards the systems with velocity-dependent right-hand sides, a moderate improvement is found for the Nosé–Hoover thermostat but no improvement for fixed bonds constrained by the general constraint dynamics based on Lagrange multipliers.
GRAPHICAL ABSTRACT
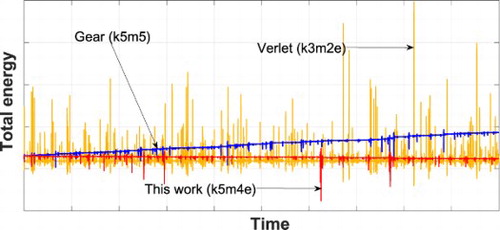
Disclosure statement
No potential conflict of interest was reported by the authors.