Abstract
The pasture growth module AgPasture was integrated into the APSIM (Agricultural Production System Simulator) simulation model, allowing pasture-based systems to be modelled in combination with other land uses at farm scale or within land use change studies. The model's predictions of pasture growth were evaluated against 32 pasture growth datasets from a diverse range of soil types and climatic zones across New Zealand. The pasture herbage accumulation simulated by the model closely matched actual measurements over varying intervals. Both predicted and measured pasture growth rate demonstrated the same seasonal pattern, including mean growth rate and inter-annual variation across measurement years. Predicted and measured annual average net herbage accumulation (NHA) on a dryland pasture was similar over 37 observation years (mean, 6.83 and 7.27 t DM/ha respectively; coefficient of variation, 29% and 27% respectively) and highly correlated (R 2 = 0.838, P < 0.0001; relative root mean squared deviation (RMSD) = 16%). The model's prediction of annual average NHA of all simulated pastures, spanning a wide range of pasture environments, also matched the measurement data well (R 2 = 0.777, P < 0.0001; relative RMSD = 21%). However, discrepancies between simulated and observed values occurred in some seasons and at some sites. Analysis of these discrepancies identified areas where the model could be improved by incorporating more accurate descriptions of the effects of plant development and grazing, soil temperature and the interactive effects of high temperature and soil moisture dynamics.
Introduction
Agricultural system simulation models have been increasingly used to assist with the evaluation of the effects of environmental and management changes on pastoral system performance, particularly the likely economic and environmental outcomes (Moore et al. Citation1997; Clark et al. Citation2008; Johnson et al. Citation2003). Whole pastoral system models enable farm management options to be explored, which, in turn, may assist farmers to adapt to future economic and environmental changes. Predicting variation in pasture growth between different seasons and years, under variable weather/soil conditions and in response to farm management decisions is a fundamental requirement for such models.
There are several deterministic and process-based models that can simulate pasture growth (e.g. Johnson et al. Citation2003; McCall & Bishop-Hurley Citation2003; Barrett et al. Citation2004; Corson et al. Citation2006) and some of these are integrated in dedicated pastoral system models such as the Whole Farm Model (McCall & Bishop-Hurley Citation2003) and SGS/DairyMod/EcoMod (Johnson et al. Citation2003, Citation2008). These models are convenient for modelling pastoral systems, but have limited or no ability to investigate interactions with, or conversion to, other types of land use such as forestry or cropping systems (Jamieson et al. Citation2006). The APSIM (Agriculture Production System Simulator (Keating et al. Citation2003) is an advanced simulator of agricultural systems. It contains a wide range of plant modules of arable crop, pastures and woodlands, and provides the possibility to simulate land use changes. The pasture growth models of AusFarm (Moore et al. Citation1997) and Grasp (Littleboy & McKeon, Citation1997) have been integrated into APSIM and can be used to simulate a range of pasture species, including tropical native grasses. Development and integration of a temperate mixed-species pasture module in APSIM would facilitate simulation of such pasture types, especially the widely distributed ryegrass/clover pastures, and provide more options for modelling diverse and complex agricultural systems. For these reasons, a new pasture module (AgPasture) was developed based on the plant physiological model described by Thornley & Johnson (Citation2000) and as implemented in EcoMod (Johnson et al. Citation2003, Citation2008). The performance of the EcoMod pasture model in simulating pasture growth rates has been extensively evaluated (Cullen et al. Citation2008; Johnson et al. Citation2008; White et al. Citation2008). Cullen et al. (2008) showed that the model simulated the monthly growth patterns and seasonal yields of ryegrass-based pasture at various sites with reasonable accuracy, including the growth rates over 2 years at two sites in New Zealand. White et al. (2008) compared the model simulation outputs for pasture growth and composition with a long-term dataset measured in New Zealand, and confirmed the model's ability to simulate the general behaviour of the observed pasture.
Integration of the AgPasture plant growth model into the APSIM framework required that the model not only respond correctly to changes in biophysical conditions, but also interact correctly with the soil, microclimate and other system components to simulate energy flow, nutrient cycling and water balance of the agro-ecosystem. AgPasture used the same processes of the EcoMod pasture model to calculate plant potential growth, but with many changes and adaptations to maintain APSIM system integrity or to improve model predictions. These changes included the use of different approaches to calculate plant water and nitrogen (N) demand and quantify their deficit effects (details below), and use of a variable instead of constant targeting ratio to partition new growth among plant organs in different seasons. Due to the large number of changes implemented in AgPasture, it is important to know that the model performs well before it can be confidently used to predict pasture growth and production, either stand-alone or in combination with other land uses and at farm scales. This paper gives a brief description of the pasture growth model as an integral module of APSIM, with emphasis on changes/adaptations from the implementation in EcoMod, and examines how accurately the model predicts seasonal and geographical patterns of pasture growth. To achieve this, predictions from the model are compared with long-term measurements of pasture herbage accumulation on diverse soil types and under different climatic conditions across New Zealand.
Method
The pasture model
The pasture growth model AgPasture integrated into APSIM (hereafter, terms in italic are APSIM modules) implemented the same principles for quantifying plant growth in relation to environmental factors as those in EcoMod (Johnson et al. Citation2008). AgPasture is an integrative module of mixed pasture species. While this seems different from other APSIM plant modules, where each plant species is treated as a separate module, all plant growth processes and functions were implemented in AgPasture at the individual species level. These individualised processes included photosynthesis and respiration, biomass accumulation and partitioning among different organs, litter deposition and root senescence. Competition among species for light, water and nutrients was explicitly modelled and pasture properties were aggregated across those of species. As an integral part of APSIM, the AgPasture module transfers energy and mass to other modules within the system, such as deposition of plant roots into the soil fresh organic matter pool and plant litter returns to the soil surface organic matter (SurfaceOM). Also, AgPasture takes up N and water from the soil by interacting with the existing modules SoilN and either SoilWat (Probert et al. Citation1998) or APSwim (Verburg et al. Citation1996) as directed by the simulation setup.
Compared with the implementation in EcoMod, some plant growth processes were changed or adapted to fit with the existing module boundaries in APSIM or to obtain better predictions against existing datasets. These changes included a dynamic partitioning rule for new plant growth between shoots and roots, which allocated less new growth to roots in spring (approximately during the plant reproductive period) and more in autumn, based on experimental evidence (Sheehy et al. Citation1979; Parsons & Robson Citation1981). Water and N balance, as well as their deficit effects on plant growth, were calculated in a similar but different way to that used by Johnson et al. (2008). Briefly, plant water demand was calculated using the Penman–Menteith approach (Micromet). Water available to plants from the soil was calculated using the soil water cascading module (SoilWat) or the soil water infiltration and movement model (APSwim). Soil moisture stress on plant growth (GLFwater) was defined as the ratio of plant extractable water in the soil profile to plant water demand. Plant extractable water was the amount of water that could be taken up by plants during a 1-day time period, calculated according to the volumetric available water content in the root zone and a water extraction coefficient (kl) in soil layers; kl is a plant–soil interface parameter covering the effects of plant root length density (k) and soil diffusivity (l) (Wang & Smith Citation2006). This was different from the approach used in EcoMod, which defined GLFwater as a function of the actual soil water content to the soil water retention parameters and root length density. The effect of water limitation on plant growth was incorporated by multiplying GLFwater by potential plant growth.
Plant growth was limited when soil N supply was less than plant N demand. The extent of limitation was defined by growth limiting factor GLFn: the ratio of plant uptakable N to plant N demand. The concept of critical N concentration (Lemaire & Salette Citation1984) was used in calculating the N demand. It was assumed that each plant species had a maximum (N max), minimum (N min) and critical (N crit) shoot N concentration during its growth and development: N crit is the minimum N concentration that permits maximum plant growth; N max is the maximum N concentration that plants could accumulate; N min is the N concentration below which plants could not survive. The maximum N uptake by new plant growth was calculated using the N max of different plant organs, while the N demand for new plant growth was calculated using N crit. When pasture level GLFn < 1, an explicit partition of soil N among species was performed. The symbiotic N fixation (SNF) of legume species was estimated first based on the formulation of Schwinning & Parsons (Citation1996) and Johnson et al. (Citation2008):
where F max and F min are the fractions of SNF to total N demand when no soil N was available (F max = 0.6) and when soil N was sufficient (F min = 0.2). Then, plant available N in the soil was partitioned among species in proportion to their demands to soil mineral N. The GLFn of each species was separately updated (as the ratio of actual N uptake to N demand) and the predicted potential daily growth would be reduced by a factor of the square root of GLFn. Consequently, plant green leaf area expansion was reduced and the N concentration in plant tissues was diluted. The dilution of N concentration in plant green tissue would in turn reduce plant radiation use efficiency by a N concentration ([N]) factor of:
Options to manage the grazing, mowing and renewal of pasture were added using a set of predefined Manager components. Animal excreta were calculated based on herbage ingestion and digestibility. Excreted N was partitioned to faeces and urine, with faeces returned to soil surface and urine (as urea) into the soil profile.
The pasture production datasets
This analysis used 32 pasture net herbage accumulation (NHA) datasets derived from long-term measurements at 27 geographical locations in New Zealand ( and ). Most of the datasets were compiled from a series of pasture growth rate measurements using a standard technique of movable exclusion cages (Radcliffe Citation1974a) (called the ‘Radcliffe dataset’ in this paper). Two datasets were from two long-term experiments, one at a dry and cool site (Winchmore at 43.825°S, 171.825°E) and one at a warm and humid site (Ruakura at 37.768°S, 175.310°E, ). These two datasets were collected using different measurement methods and schedules to the Radcliffe dataset and had detailed records on weather, soil and pasture management. While all the datasets were used to examine the model's ability to predict pasture growth patterns across various sites, the Winchmore and Ruakura datasets were used to analyse how effectively the model simulated day-to-day, seasonal and inter-annual variation of pasture growth and production.
Figure 1 The 27 pasture sites and the climatic zones in New Zealand. The climatic zones are from NIWA (Citation2011), with permission. The pasture sites are detailed in .
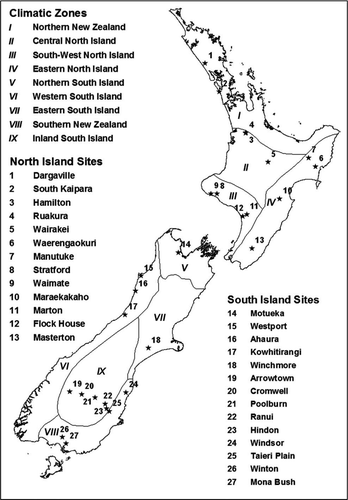
Table 1 Description of experimental pastures.
Winchmore dataset
Pasture growth was measured under different irrigation treatments at the Winchmore Irrigation Research Station (site 18 on ) on an experimental pasture of perennial ryegrass (Lolium perenne) and white clover (Trifolium repens) established in 1958 (Richard & McBride Citation1986). The soil was a Lismore stony silt loam (Pallic orthic brown soil) (NZSB Citation1968) characterised by gravel increasing with depth. Superphosphate was applied annually (250 kg/ha) and lime periodically to maintain adequate phosphorus (P) and sulphur (S) concentrations and soil pH. No N fertiliser was applied. Dryland control (four replicates) and the ‘full’ irrigation treatment (five replicates) were used. The full irrigation treatment comprised applying 100 mm water (border dyke irrigation) whenever the soil volumetric moisture content depleted to 20%, which equated to approximately 50% of plant available water-holding capacity, within the irrigation season of September to April. Both the control and the irrigated treatments were over-drilled with perennial ryegrass and white clover in August 1976. The irrigated pasture treatment was over-drilled once more in September 1980. Paddocks of irrigated and dryland pasture were grazed rotationally by separate flocks of sheep as needed. Pasture NHA was measured using exclusion cages (1 m × 3 m). Pastures were pre-trimmed to 2 cm before placing the cage to exclude grazing animals. Pasture in the cage was cut back to 2 cm and weighed prior to the cage being moved to another pre-trimmed area. Measurement dates varied depending on pasture growth. Six to nine measurements were collected during each season from July to June from 1966 and 2004. White et al. (2008) compared the simulation outputs of the EcoMod pasture model with this pasture dataset. Likewise, this study explores the performance of AgPasture against this dataset.
Ruakura dataset
This pasture growth dataset was measured on grazed dairy pasture at No. 2 Dairy Farm located in Waikato (site 4, Ruakura on ) also using the exclusion cage technique. The pasture contained a mixture of perennial ryegrass and white clover and was on a Horotiu sandy loam soil (Typic orthic allophanic soil) (NZSB Citation1968). Three cages (each 2.06 m2) were placed, one to a paddock, within the three farmlets. Pasture was trimmed to 2 cm using a mower before cage placement, and the cages were harvested every 28 days and placed on a new site within the same paddock. Although measurements were collected over 12 years, only measurements over a 10-year period (1983–1992) were used in this analysis because a different measurement frequency was used in last 2 years of data collection.
Radcliffe dataset
Measurements of pasture growth rate () were predominantly conducted during 1950 to 1980 using a standard exclusion cage technique, with measurements collected every 2 weeks. Measurements were taken for 3–27 years at different sites and have been reported in a series of papers as noted in . Although these pastures were measured only on one site on a particular soil type, measurements over multiple years should reliably show pasture growth seasonal pattern and responses to weather conditions (Radcliffe Citation1974a).
Most of the pastures () were dominated by perennial ryegrass and white clover, with a variety of minor species. The exceptions were site 1 (Dargaville), where paspalum (Paspalum dilatatum) was a major grass along with perennial ryegrass, and sites 5 (Wairakei) (flat) and 6 (Waerengaokuri), where browntop (Agrostis capillaris) and sweet vernal (Anthoxanthum odoratum) were more abundant than perennial ryegrass. All these experimental pastures were fertilised with superphosphate, potassium and lime, but no N was applied. Four pastures were irrigated, including the pasture in Winchmore described above. At site 19 (Arrowtown), two spray irrigations per season were applied to wet soil to at least 30 cm. At 20 (Cromwell) and 21 (Poolburn), irrigations were applied to wet soil to at least 30 cm when soil moisture content dropped to 50% or 25% of plant available water-holding capacity respectively (Radcliffe & Cossen Citation1974). Most pastures were grazed by sheep and beef animals, except those at sites 3 (Hamilton), 4 (Ruakura), 8 (Stratford) and 9 (Waimate), which were grazed by dairy cows.
Simulation settings
Pasture simulations were constructed using the pasture model (AgPasture) with a set of APSIM7.3 modules for simulating weather conditions (Met), plant solar radiation interception and transpiration demand (Micromet), soil water (SoilWat), soil carbon–nitrogen dynamics (SoilN) and soil surface organic matter (SurfaceOM). The management module (Manager) was used to specify irrigation and fertiliser applications as well as grazing management. The detailed parameter settings at each site were as follows.
Winchmore pasture simulation
One simulation was run for each of the dry and irrigated pastures over the period 1966–2004. Weather data measured on site were used. For the irrigated pasture, 100 mm irrigation was applied at the exact dates of irrigation applications during the experimental period. Irrigation efficiency (fraction of irrigated water entering the soil profile) for this border-dyke system was set to 0.75, which was estimated based on the irrigation efficiency used in other APSIM simulations for similar irrigation systems (e.g. 0.7 used by Peake et al. (Citation2008) for flush irrigation of maize). The simulation using this parameter setting predicted the observed pattern of soil moisture dynamics (Li et al.Citation2010). This irrigation setup was different from that in the simulation of White et al. (2008), which automatically applied 100 mm water whenever soil water deficit reached 16 mm for the top 100 mm of soil. Pasture harvests were also simulated on the dates of actual pasture measurements. Pasture residual after harvest was estimated to be 1000 kg DM/ha based on observations (Cossens Citation1990) and previous simulation experience (White et al. Citation2008). The simulated net herbage harvest was used to estimate the herbage ingested by grazing sheep between measurements, and undigested plant materials were calculated and returned as animal excreta. Nitrogen removal from the system due to sheep grazing (removal in animal products and volatilisation from animal excreta) was estimated as 15% of ingested N (Whitehead Citation1995), and the remaining N was returned through faeces (40%) to soil surface or as urine (60%) to the soil profile (mainly in top 5 cm layer but a small portion into the layer below up to 30 cm).
Soil parameters were set up based on measurements taken during the experimental period. Soil organic carbon (SOC) content in the top 10 cm soil layer was set at 4.0% and 3.5% respectively for dry and irrigated pasture at the start of the simulation (Metherell et al. Citation2002; Srinivasan & McDowell Citation2009).
Ruakura pasture simulation
The simulation was run for 10 years (1982–1992) against measurements using observed weather data at the experimental site. Soil profiles were described according to the quantitative description of the soil type (Wilde Citation2003). Pasture harvests were simulated using the exact dates of actual pasture measurements, and pasture residual after each harvest was estimated to be 1000 kg DM/ha. The simulated NHA harvest was used to estimate herbage consumed by grazing cows between two measurements. Undigested plant material was returned as excreta to soil surface. N removal due to cow grazing was estimated as 30%, including 20% in animal products estimated using a Whole Farm Model (Beukes et al. Citation2008 and pers. comm.) and 10% ammonia volatilisation from animal excreta. The remaining N was returned in the faeces (40%) the to soil surface or as urine (60%) into the soil profile (mainly in top 10 cm soil layer but a small portion into the layer of 10–30 cm).
Radcliffe pastures simulation
Simulation settings of pastures at the 25 locations (Radcliffe dataset) were similar to the two pastures described above. On these sites, pasture growth rates were measured, but soil description and weather data were incomplete or unavailable for many sites for the measurement period (). Virtual climate station (VCS) data (Tait et al. Citation2006) were obtained for each pasture site according to its geographical coordinates. The VCS data were available only for the period after 1972. If a pasture was measured before 1972, the VCS data from 1972 for the same number of years as the experimental period were used. The average annual rainfall from the VCS data was compared to that reported from the experiments if available () and, if necessary, the VCS rainfall data were linearly scaled to obtain the same annual average rainfall as recorded during the experiment. Such scaling has previously been shown to be effective (Cichota et al. 2008). Although the model-generated pasture growth at these sites using weather data that differed from that of a measurement period could not simulate day-to-day pasture dynamics for the measurement period, the pasture behaviour derived from these simulations (e.g. mean annual NHA and its inter-annual variability) could be compared with the pasture measurements for evaluating model performance in predicting pasture growth pattern across different regions.
Soil parameters were estimated based on soil type, texture and fertility as described in the literature (see reference citations in ) and by referring to Wilde (2003). Pastures at sites 20 (Cromwell) and 21 (Poolburn) were irrigated to bring soil water content to field capacity when it was depleted to 50% and 25% respectively of plant available water-holding capacity in the top 30 cm (Radcliffe and Cossens 1974) using APSIM's automatic irrigation facility. Two irrigations of 80 mm were assumed to be applied on pasture at site 19 (Arrowtown).
Most pastures were simulated as a ryegrass/clover mixture, except at site 1 (Dargaville), where it was a ryegrass/paspalum/clover mixture. Pasture harvests were simulated on the dates of regular measurements: once every 14 days except at site 16 (Ahaura) and site 17 (Kowhitirangi), which were once every 28 days. Pasture residual after each harvest was also assumed to be 1000 kg DM/ha, unless a higher-than-standard harvest residual was specified where a residual of 1250 kg DM/ha was assumed. Harvested herbage was used to estimate the pasture consumed by grazing animals between measurements. Plant materials not digested by the animals were returned to the soil in faeces and urine as described for the Winchmore and Ruakura pastures.
Data presentation and analysis
For the Winchmore and Ruakura pasture sites, data from simulation and measurements were compared at three levels: (1) NHA at each measurement date (i.e. over each actual measurement period); (2) average pasture growth rate for each calendar month; (3) annual total NHA. To calculate the average daily pasture growth rate for each calendar month, each simulated or measured NHA value was divided by the number of accumulation days to generate an average pasture growth rate (kg DM/ha per day) of the period. The average daily pasture growth rate of a calendar month was then the sum of the growth rate for each day of the month divided by the number of days in the month. Both the mean and variability of the simulated and measured values were compared. Inter-annual variations of pasture growth were also analysed in relation to rainfall patterns to evaluate the model's capability in predicting the effect of water deficit on pasture growth.
For all pasture sites (), average daily pasture growth rates over the measurement interval (mostly 14 days) and its variability between years have been well documented (reference citations in ). Simulated daily pasture growth rates were averaged over measurement intervals of 14 days for each year, and the mean and standard deviation across measurement years were calculated for comparison. Simulated and measured seasonal patterns of pasture growth rates and annual NHA (July to June) across different climatic zones were compared. Root mean squared deviation (RMSD) between simulated and measured values, deviation of the regression between simulation and measurements from the 1:1 line and the coefficient of variation (CoV) of simulated or measured pasture NHA were used to assess accuracy and precision of the model.
Results
Net herbage accumulation for Winchmore and Ruakura sites
The harvested NHA for each measurement was compared with simulated values for all NHA periods (measurement intervals). Simulated and measured NHA matched closely at both the Winchmore and Ruakura pasture sites (). The simulated and measured NHA over all measurement periods were highly correlated, but had a relatively large RMSD. Measured (M) and simulated (S) NHA had the following relationships.
Figure 2 Simulated and measured NHA for each measurement interval. A, Dryland pasture at Winchmore. B, Irrigated pasture at Winchmore. C, Pasture at Ruakura. For Winchmore pastures, only scenarios from 1991–2000 are shown. The results over the whole simulation period of 1966–2004 were similar. Open circles (○), simulated mean; cross (+), measured mean; grey shaded area, measured range (maximum and minimum); thin cross (×), simulation ‘outliers’ (see ‘Discussion’).
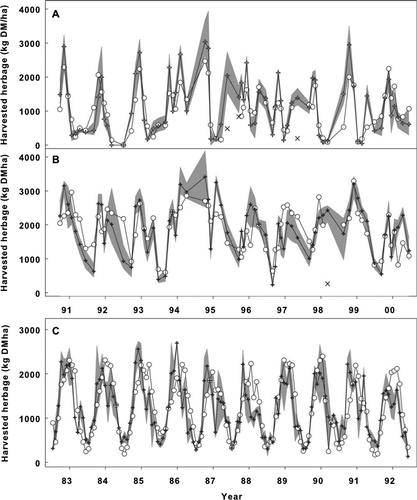
Irrigated pasture at Winchmore:
R 2 = 0.47, n = 281, P < 0.001, relative RMSD = 33% with a measured mean of 1564 kg DM/ha per harvest.
Dryland pasture at Winchmore:
R 2 = 0.60,n = 281,P < 0.0001, relative RMSD = 53% with a measured mean of 923 kg DM/ha per harvest.
The Ruakura pasture:
R 2 = 0.55, n = 130, P < 0.0001, relative RMSD = 34% with a measured mean of 1194 kg DM/ha per harvest.
Seasonal patterns and inter-annual variation of pasture growth rate
Model-predicted monthly means and variability of pasture growth rate matched well with measurements on both irrigated (compare A and 3B) and dryland (Fig. 3C and 3D) pastures in Winchmore. There was a slight difference between simulation and measurement in the inter-annual variability of pasture growth rate for summer irrigation (January and February in Figs. 3A and 3B), with the simulated rate having a slightly larger variability. Both measured and simulated pasture growth rates showed a distinct difference between dry and irrigated pastures. Simulated seasonal patterns of pasture growth rate at the Ruakura sites also matched well with measurements, although the predicted summer growth rate (January and February) was slightly higher than measurements (see Fig. 3E and 3F).
Figure 3 Comparison of measured (left) and simulated (right) monthly average pasture growth rate at Winchmore (over 37 years) and Ruakura (over 10 years). A, Measured for dryland pasture at Winchmore. B, Simulated for dryland pasture at Winchmore. C, Measured for irrigated pasture at Winchmore. D, Simulated for irrigated pasture at Winchmore. E, Measured at Ruakura. F, Simulated at Ruakura. The boxes represent the lower quartile (Q1), median (Q2) and upper quartile (Q3); whiskers represent the 5th and 95th percentiles; open circles (○) represent the mean.
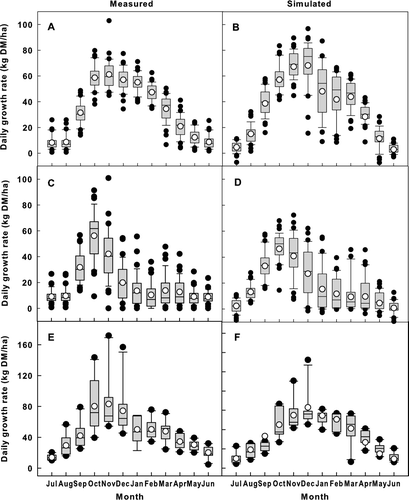
Annual NHA and its variation
Simulated and measured annual NHA were similar for both dry and irrigated pastures in Winchmore and for the pasture in Ruakura. The model nicely predicted the low and largely variable annual NHA of dryland pasture in Winchmore. The simulated and measured annual NHA averaged 6833 and 7277 kg DM/ha respectively over the 37 years (not significantly different, t-test P = 0.40). Annual NHA showed a similar inter-annual variability (CoV = 29% and 27% for simulated and measured annual NHA, respectively) and their variations were highly correlated (R 2 = 0.838, n = 37, P < 0.0001; relative RMSD = 16%). Under irrigation, simulated and measured annual NHA averaged 12,475 and 12,844 kg DM/ha respectively, with a relative RMSD of 17% and a small inter-annual variation (CoV=10% and 11% for simulated and measured annual NHA respectively). There was no statistically significant correlation between simulated and measured annual NHA (P = 0.314; see ).
Figure 4 Simulated versus measured annual NHA at Winchmore (over 37 years) and Ruakura (over 10 years) in New Zealand.
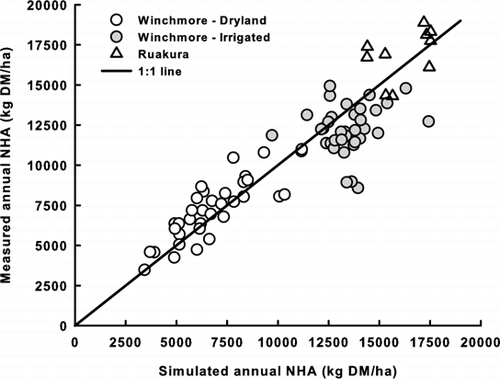
Simulated and measured annual NHA at Ruakura pasture was 16,209 and 16,725 kg DM/ha respectively, with a relative RMSD of 14%. Pasture production varied less across years. Simulated and measured annual NHA had a CoV of 9% and 10% respectively over the 10 years, and were not significantly correlated (P = 0.188).
Geographical pattern of pasture production
Simulated and measured annual NHA of the 32 pastures at 27 sites () averaged 10,429 and 9881 kg DM/ha respectively. That is, the model overestimated annual NHA by 6% across all the pastures. The simulated (S) and measured (M) annual NHA were highly correlated and the regression line M = 0.92S + 282 (R 2 = 0.866, P < 0.0001; relative RMSD = 13%) was not significantly different from a 1:1 line (). The inter-annual variation of annual NHA at these sites over the measurement years () was not analysed in detail because weather data were not synchronised with the observation period at some sites. Overall, simulated annual NHA was about 20% greater than that measured at five pasture sites: three sites in the western South Island region (sites 15, Westport; 16, Ahaura and 17, Kowhitirangi) and two in the eastern and central North Island (sites 5, Wairakei and 6, Waerengaokuri).
Figure 5 Simulated versus measured mean annual NHA of 32 pastures at 27 sites across a wide range of environments in New Zealand. The bars show one standard deviation from the mean annual NHA.
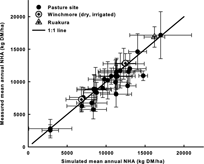
Simulated seasonal patterns of daily pasture growth rates showed a general agreement with measured patterns at all pasture sites ( and ). For example, pastures in the warm northern New Zealand had relative high pasture growth rates (e.g. site 1, Dargaville in ), while pastures in the eastern North Island region had relative low growth rates (sites 5, Wairakei; 6, Waerengaokuri and 10, Maraekakaho). Pasture growth was less variable in the western part of North Island (e.g. site 8, Straford) due to the humid climate. Pasture growth rate in northern South Island was high in spring, but reduced dramatically during summer (site 14, Motueka). Pasture growth was low in the dry eastern (site 18, Winchmore) and the semi-arid inland South Island (site 21, Poolburn) (). Pasture growth rate was also high in southern New Zealand (site 27, Mona Bush) in the moist and sunny climate. The large isolated increases in measured pasture growth rate at site 19 (Arrowtown) were well simulated by applying two spray irrigations each season on this pasture.
Figure 6 Simulated and measured seasonal pattern of pasture growth (mean of the measured years showed in ) at six representative sites in different climatic zones in the North Island of New Zealand. The points on each graph represent the average daily pasture growth rate on each of 26 regular 14-day measurements starting from the 14 June. Thin crosses (×) with thick grey bars represent the measured mean and one standard deviation of the mean rate in different years. Circles (○) and vertical bars represent simulated mean and one standard deviation.
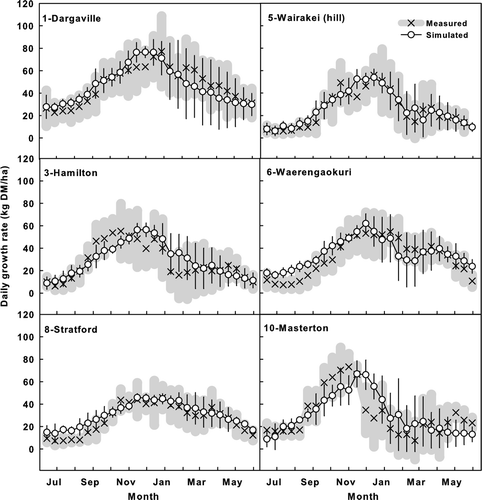
Figure 7 Simulated and measured seasonal pattern of pasture growth rates (mean of the measured years showed in ) in six representative sites in different climatic zones in the South Island of New Zealand. The points on each graph represent the average daily pasture growth rate on each of 26 regular measurements starting from the 14 June. Thin crosses (×) with thick grey bars represent the measured mean and one standard deviation of the mean rate in different years. Circles (○) and vertical bars represent simulated mean and one standard deviation.
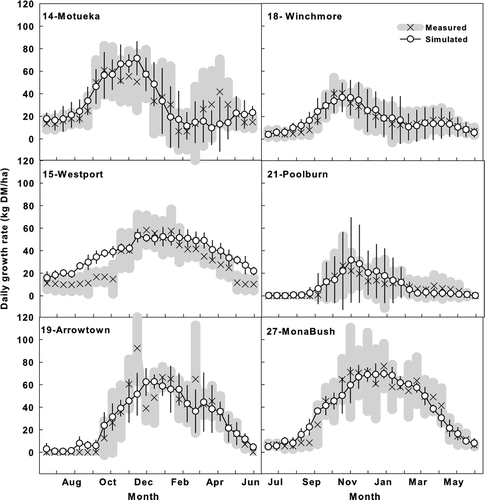
However, the model over-predicted pasture growth rate in the humid western South Island region, especially for the winter period (site 15, Westport) (). Model-simulated inter-annual variation of pasture growth in different seasons was generally smaller than that calculated from herbage measurements. Both simulated and measured growth rates had greater autumn variation (approximately March) than in other seasons in most sites, except those in the semi-arid inland South Island region, as represented by site 21 (Poolburn) ().
Discussion
The pasture model was successful in simulating pasture NHA over actual measurement intervals of different lengths () and predicting average monthly pasture growth rate (), although simulated NHA per harvest showed a relative large RMSD from the measured value. The model also accurately estimated annual NHA across different years at specific sites (), for pastures on diverse soil types and in different climatic zones (). Model-predicted seasonal patterns and inter-annual variability of pasture growth rates also matched well with measurements across New Zealand ( and ).
As expected, and consistent with the work of Richard and Radcliffe (Citation1976), annual NHA for a dryland pasture in a region like Winchmore, where annual rainfall (approximately 700 mm) was less than potential evapo-transpiration (more than 1000 mm), depends on rainfall amount and pattern in time. The correlation between measured annual NHA and annual rainfall (July–June accumulation for both NHA and rainfall) (n = 37, R 2 = 0.306, P = 0.011) was much weaker than that between measured and simulated annual NHA (n = 37, R 2 = 0.838, P < 0.0001; ). This confirmed the importance of rainfall seasonal pattern for pasture growth and that the effect of rainfall pattern was properly incorporated in the model.
Some discrepancies between simulation and measurement were observed. These disparities may arise from the inaccuracy of plant functional processes in the model, but they may also arise from simulation settings that could not capture all the biophysical and management conditions. Also, variability from the difficulties in herbage measurements is well known, especially under animal grazing (Cullen et al. Citation2008). The estimation error arising from measurements should also be taken into account in analysing disparities between simulation and observation.
One of the reasons for the relatively large RMSD between the simulated and measured NHA per harvest was probably associated with the fact that simulated pasture harvest and actual pasture grazing were not synchronised. The actual grazing history at each site immediately prior to setting up the cages for pasture growth measurement could be quite different, while the simulation assumed that the pastures were grazed around harvest dates. Despite the relatively large RMSD, simulated and measured NHA showed a similar pattern and were significantly correlated (); the aggregated monthly pasture growth rate from simulation matched the measurements well.
Inter-annual variability of simulated monthly or fortnightly pasture growth rates was generally smaller than that of measurements ( and ). This difference might partly be attributable to variation in measured NHA due to pasture heterogeneity in sampling areas and differences in grazing management across years. Model prediction was deterministic for the specified simulation settings. For example, it was assumed that animals grazed the pasture and returned urine and excreta on the specified harvest dates in simulations; in reality, pasture herbage harvest dates were not aligned with animal grazing dates. The smaller variability of simulated pasture growth rates might also be attributed to the constant post-grazing residual set in the simulation, while the residual herbage mass might change from season to season and from year to year, even for the same residual height (Cossens, Citation1990). The inaccuracies of simulation could have arisen from the soil profile descriptions based mostly on information from the literature. Some deviation also arose unavoidably from the weather data where the VCS data did not align with the observation period. On these sites, although annual rainfall was adjusted to the recorded amount for the observation period and the simulated mean annual NHA fit in the linear relation with measured annual mean NHA across different sites (), the deviation caused by using these estimated weather data cannot be quantified.
Simulations using a generic ryegrass/clover pasture might also cause some variability when compared with measurements, as pastures at different sites may consist of different plant cultivars and contain different minor component species ().
Pasture NHA and soil temperature effects
The model accurately simulated the pasture NHA harvested over actual measurement periods, but a couple of ‘outliers’ were found. For the dryland pasture in Winchmore, a lower NHA was predicted for measurement intervals from 23 March to 25 May and from 25 May to 20 September 1995 (A). The weather and measured NHA over these two intervals did not explain the discrepancy. Rainfall in the first measurement interval (23 March to 25 May) was 95 mm, lower than the average amount over the 37 simulation years (124 mm), but the measured NHA was 2044 kg DM/ha—much greater than the long-term average (820 kg DM/ha). Also, dryland pasture NHA was significantly higher than irrigated over these two measurement intervals (2044> 1769 kg DM/ha for the period from 23 March to 25 May, t-test, P < 0.0001 and 1407>980 kg DM/ha for the period from 25 May to 20 September, t-test, P < 0.0001) (). The observed results might be partly related to the higher soil temperature on the dry pasture than on the irrigated pasture during this period, favouring plant growth, but the small soil temperature difference (averaged 0.25° C for the period, unpublished data) alone can hardly explain the big NHA difference. The large range of measured NHA values might suggest some measurement errors.
Another marked underestimate of dryland pasture NHA occurred on 8 May 1997 (A), which might be attributed to the unusually lower than normal air temperature in April 1997, with daily minimum temperature of 0 °C for several days. It was likely that soil temperature was still much higher in April than in winter, while air temperature was similar to winter. The temperature restriction on plant growth in the model might be much less as it is calculated based on air temperature. Incorporating soil temperature in quantifying plant growth may improve the model's performance.
Interactions among high temperature, soil moisture and plant phenology
For the irrigated pasture in Winchmore, a discrepancy between measured and simulated NHA is evident on 10 March 1998 (B). In the model, the effect of a high-temperature event on plant growth was incorporated as described by Johnson et al. (2008). In this simulation, high temperature started to restrict plant growth when daily maximum temperature reached the onset temperature of 28 °C and to fully restrict plant growth when it reached 35 °C. The predicted lower NHA on this measurement date was clearly a consequence of modelled consecutive high maximum temperature during this herbage accumulation period, while the measured NHA did not show such a severe reduction. To confirm this, high-temperature effects were excluded and the model did give a better NHA prediction for this measurement date (predicted 2680 kg DM/ha versus measured 2430 kg DM/ha), but it also resulted in some overestimates of pasture NHA during the high-temperature period in other years. Both perennial ryegrass and white clover suffer due to high temperature (Mitchell Citation1956; Barrs Citation1976b). The severity of this depends on soil moisture conditions, with a greater effect on dry rather than moist soils for the same high temperature (Archer & Robinson Citation1989). Setting a higher onset temperature for high-temperature effects on irrigated than on dryland pasture gave better simulations for the pasture in Winchmore, as indicated by White et al. (2008). However, it is difficult to determine what temperature should be used for a pasture at a specific site when using the model. A solution could be to build a more general relation between pasture growth and the interactive effects of high temperature and soil moisture into the model.
For the Ruakura pasture, the simulated NHA over most measurement intervals matched the general pattern of measurements (C); a slight dip in measured NHA in the middle of summer in some years was not always predicted by the model. Since the NHA was regularly harvested at this site, its seasonal pattern was the same as that of monthly average of daily pasture growth rate (E and 3F). Pasture growth in spring (October–November) was slightly underestimated, while growth in summer (December–February) was slightly overestimated. In summer, the dip in measured pasture growth might be related to soil moisture deficit and high temperatures (Barrs Citation1976b). However, it may also reflect decreased plant growth in late or post-reproductive stages, since decline in growth rate did not necessarily match with low-rainfall summer. In this pasture, paspalum was also recorded as a minor species. It had more growth in later summer and autumn, which might contribute to the second small peak of measured growth in autumn (C). Predicting plant growth rate could be improved by more accurately representing plant developmental stages and quantifying plant growth differently among those stages (Moore et al. Citation1997; Parsons & Chapman Citation2000; Cullen et al. Citation2008).
Pasture management effects
Simulated and measured annual NHA were highly correlated under dryland (R 2 = 0.838, P < 0.0001), but not irrigated conditions (R 2 = 0.314) at Winchmore, nor the relatively moist conditions of Ruakura (R 2 = 0.188). These results indicate the model was able to simulate soil moisture dynamics and predict water-deficit effects on pasture growth in response to the large inter-annual variations in rainfall patterns under dryland conditions, but it was unable to fully simulate effects of other environmental and management factors that caused the smaller inter-annual variation of pasture growth under moist conditions. These could include variation in other climatic factors, but also variation in pasture management (e.g. pasture age, grazing and fertiliser application schedules) and pasture pest and diseases, which were not modelled.
Over-prediction of annual NHA of the irrigated pasture in Winchmore in 1975–1976, 1976–1977, 1980–1981 and 1985–1986 pasture growth seasons () was difficult to interpret until it was noted, from the archive, that both dry and irrigated pasture were over-drilled with perennial ryegrass and white clover in August 1976 and the irrigated pasture was over-drilled once more in September 1980. These records indicate that the pasture was in a poor condition in 1975–1976 and 1980–1981 seasons, and in recovery in the 1976–1977 and 1981–1982 seasons after over-drilling.
Predicted seasonal pasture growth patterns were improved by partitioning more plant assimilates into above-ground tissues during the reproductive growth stage than in other stages (Parsons & Robson Citation1981). Grazing reduces the development and growth of ryegrass reproductive tillers (Parsons & Chapman Citation2000), which complicates the effects of reproductive growth on herbage accumulation. To increase the accuracy of the model, the effect of grazing on growth and development of reproductive tillers needs to be incorporated, along with more detailed simulation of plant phenology.
Regional divergence of pasture production
The simulated annual NHA was slightly overestimated (6%) and had a RMSD of 13% across all the pasture sites (). This accuracy was adequate in comparison with the prediction of other pasture models (e.g. Clark et al. Citation2008; Roberson Citation2006; Cullen et al. Citation2008), especially considering that there were no detailed measurements of soil profiles and incomplete weather data in some sites. A comparison of simulated versus measured seasonal pattern of pasture growth at each site has been presented ( and ). The number of measured pasture sites varied from 1 to 5 in the climate zones identified in . The overestimate of annual NHA was mostly due to significant over-prediction (>20%) at five sites, three of which were located in humid western South Island regions (e.g. 15, Westport in ). Logically, the overestimate of pasture production in this very humid climatic zone (e.g. mean annual rainfall was 2260 mm in Westport) could be related to modelling of soil water saturation or water logging effects on plant growth. In the model, plant growth was deemed to be reduced by 20% when the soil became saturated. This ‘saturation reduction factor’ was tested by setting it to 100%; that is, assuming a plant would cease growing completely when the soil became saturated. Under this assumption, simulated pasture growth was still markedly higher than the measured values (data not shown). This result suggests that overestimation of pasture NHA was unlikely to be caused by the model's inability in simulating water saturation effects, though underestimation of the water saturation period due to inaccurate specification of soil properties cannot be ruled out. Other factors, such as lower soil pH (Morton & Paterson Citation1982) or nutrient limitation due to high drainage, may also restrict plant growth.
Conclusions
The model AgPasture accurately predicted seasonal patterns and inter-annual variation of pasture growth rate on diverse soil types and under different climatic conditions across New Zealand. The variation of pasture growth in response to irrigation was also predicted. This evaluation provided confidence in the model's ability to simulate pasture herbage accumulation under various management scenarios and under a wide range of pasture environments. It also demonstrated the capability and flexibility of APSIM in setting up simulations, based on actual pasture management on a day-to-day basis. The pasture model was released in APSIM 7.3 (February 2010) and can be used as a stand-alone module in APSIM to simulate pasture growth and production or in combination with other plant modules to simulate the long-term effects of land use changes (e.g. pasture/crop/woodland conversions) in the dynamic landscape of farming systems. Performance of the pasture model could be further improved by incorporating a more accurate description of plant phenology and the effects of grazing and soil temperature, and interactive effects of high-temperature and soil moisture dynamics.
Acknowledgements
This work was funded by the New Zealand Ministry of Science and Innovation as a part of the programme ‘Rural futures: Building adaptive management capability to deliver sustainable pastoral farm systems (C10X0809)’. The authors thank Brendan Cullen, Craig Beverly, Ian Johnson and Neil Huth for assistance and comments in the early stage of model development and integration, Ray Moss and Mike Sprosen for information on pastures in the two detailed study sites, and Warren King, Ronaldo Vibart and two anonymous referees for comments on an early version of the manuscript.
References
- Archer , K and Robinson , G . 1989 . The role of stolons and seedlings in the persistence and production of white clover (Trifolium repens L. cv. Huia) in temperate pastures on the Northern Tablelands, New South Wales . Australian Journal of Agricultural Research , 40 : 605 – 616 .
- Barratt , BIP and Brush , DW . 1985 . Seasonal pattern of pasture production and the contribution of low fertility tolerant grasses in oversown tussock grassland on the lower Otago Plateau . New Zealand Journal of Experimental Agriculture , 13 : 201 – 207 .
- Barrett , PD , Laidlaw , AS and Mayne , CS . 2004 . An evaluation of selected perennial ryegrass growth models for development and integration into a pasture management decision support systems . Journal of Agricultural Science , 142 : 327 – 334 .
- Barrs , JA . 1976a . Seasonal distribution of pasture production in New Zealand VIII. Dargaville . New Zealand Journal of Experimental Agriculture , 4 : 151 – 156 .
- Barrs , JA . 1976b . Seasonal distribution of pasture production in New Zealand IX. Hamilton . New Zealand Journal of Experimental Agriculture , 4 : 157 – 161 .
- Barrs , JA , Radcliffe , JE and Brunswick , L . 1975 . Seasonal distribution of pasture production in New Zealand VI. Wairakei, pasture and lucerne production . New Zealand Journal of Experimental Agriculture , 3 : 253 – 258 .
- Beukes , PC , Palliser , CC , MacDonald , KA , Lancaster , JAS and Levy , G . 2008 . Evaluation of a whole-farm model for pasture-based dairy systems . Journal of Dairy Science , 91 : 2353 – 2360 .
- Cichota , R , Snow , VO and Tait , AB . 2008 . A functional evaluation of the Virtual Climate Station rainfall data . New Zealand Journal of Agricultural Research , 51 : 317 – 329 .
- Clark , SG , Donnelly , JR and Moore , AD . 2000 . The GrassGro decision support tool: its effectiveness in simulating pasture and animal production and value in determining research priorities . Australian Journal of Experimental Agriculture , 40 : 247 – 256 .
- Corson , MS , Skinner , RH and Rotz , CA . 2006 . Modification of the SPUR rangeland model to simulate species composition and pasture productivity in humid temperate regions . Agricultural Systems , 87 : 169 – 191 .
- Cossens GG 1990 . Pasture and lucerne production in Otago and Southland, 1950–1987 . Invermay Agricultural Centre, Ministry of Agriculture and Fisheries . Technical report no. 21 .
- Cossens , GG and Radcliffe , JE . 1978 . Seasonal distribution of pasture production in New Zealand XIV. The lower Otago Plateau: Hindon . New Zealand Journal of Experimental Agriculture , 6 : 47 – 52 .
- Cossens , GG and Brash , DW . 1981 . Seasonal distribution of pasture production in New Zealand XV. The Higher Otago Plateau: Rock and Pillar Range . New Zealand Journal of Experimental Agriculture , 9 : 73 – 78 .
- Cullen , BR , Eckard , RJ , Callow , MN , Johnson , IR , Chapman , DF , Rawnsley , RP , Garcia , SC , White , T and Snow , VO . 2008 . Simulating pasture growth rates in Australian and New Zealand grazing systems . Australian Journal of Agricultural Research , 59 : 761 – 768 .
- Gray , MH , Korte , CJ and Christieson , WM . 1987 . Seasonal distribution of pasture production in New Zealand XX. Waerengaokuri (Gisborne) . New Zealand Journal of Experimental Agriculture , 15 : 397 – 404 .
- Jamieson PD , Zyskowski RF , Li FY , Francis GS , Snow VO , Lilburne L 2006 . LUCI in the sky with diamonds: modelling the wider impacts of land use change and intensification . In : Turner N , Acuna T Groundbreaking stuff: Proceedings of the 13th Australian Agronomy Conference . Perth, Western Australia, 10–14 September 2006 (doi:www.regional.org.au/au/asa/2006/plenary/technology/4603_jamiesonp.htm) .
- Johnson , IR , Lodge , M and White , RE . 2003 . The sustainable grazing systems pasture model: description, philosophy and adaptation to the SGS national experiment . Australian Journal of Experimental Agriculture , 43 : 711 – 728 .
- Johnson , IR , Chapman , DF , Snow , VO , Eckard , RJ , Parsons , AJ , Lambert , MG and Cullen , BR . 2008 . DairyMod and EcoMod: biophysical pasture simulation models for Australia and New Zealand . Australian Journal of Experimental Agriculture , 48 : 621 – 631 .
- Keating , BA , Carberry , PS , Hammer , GL , Probert , ME , Robertson , MJ , Holzworth , D , Huth , NI , Hargreaves , JNG , Meinke , H , Hochman , Z , McLean , G , Verburg , K , Snow , V , Dimes , JP , Silburn , M , Wang , E , Brown , S , Bristow , KL , Asseng , S , Chapman , S , McCown , RL , Freebairn , DM and Smith , CJ . 2003 . An overview of APSIM, a model designed for farming systems simulation . European Journal of Agronomy , 18 : 267 – 288 .
- Lemaire , G and Salette , J . 1984 . Relation entre dynamique de croissance et dynamique de prelevement d'azote pour un peuplement de graminees fourageres I. Edude de l'effet du milieu . Agronomie , 4 : 423 – 430 .
- Li FY , Snow VO , Holzworth DP , Johnson IR 2010 . Integration of a pasture model in APSIM . In : Dove H , Culvenor RA Food security from sustainable agriculture. Proceedings of 15th Australian Agronomy Conference . Lincoln, New Zealand, 15–18 November 2010 (doi: www.regional.org.au/au/asa/2010/farming-systems/simulation-decision-support/6993_lifyrevis.htm) .
- Littleboy M , McKeon GM 1997 . Subroutine GRASP: grass production model. Documetation of the Marcoola version of subroutine GRASP . Appendix 2 of evaluating the risks of pasture and land degradation in native pasture in Queensland. Final report for the rural industries research and development corporation, DAQ124 .
- McCall , DG and Bishop-Hurley , GJ . 2003 . A pasture growth model for use in a whole-farm dairy production model . Agricultural Systems , 76 : 1183 – 1205 .
- Metherell AK , Stewart DPC , Carey PL , Moss RA 2002 . Long-term irrigation improves soil quality but decreases soil carbon sequestration . In : Stephens P , Callaghan J , Austin A Proceedings of Soil Quality and Sustainable Land Management Conference, Palmerston North, Landcare Research New Zealand Ltd Pp. 55 – 61 .
- Mitchell , KJ . 1956 . Growth of pasture species under controlled environment. I. Growth at various levels of constant temperature . New Zealand Journal of Science and Technology , 38A : 203 – 216 .
- Moore , AD , Donnelly , JR and Freer , M . 1997 . GRAZPLAN: Decision support systems for Australian grazing enterprises. III. Pasture growth and soil moisture sub-models, and the GrassGro DSS . Agricultural Systems , 55 : 535 – 582 .
- Morton , JD and Paterson , DJ . 1982 . Seasonal distribution of pasture production in New Zealand XVII. Kowhitirangi and Ahaura, West Coast, South Island . New Zealand Journal of Experimental Agriculture , 10 : 245 – 252 .
- NIWA 2011 . Overview of New Zealand Climate . http://www.niwa.co.nz/education-and-training/schools/resources/climate/overview (accessed 10 June 2011) .
- NZSB (New Zealand Soil Bureau) 1968 . General survey of the soils of South Island , New Zealand New Zealand Soil Bureau Bulletin 27. Lincoln, New Zealand .
- Parsons AJ , Chapman DF 2000 . The principles of pasture growth and utilization . In : Hopkins A Grass: Its production and utilization . 3rd edition , Oxford , Blackwell Science . Pp. 31 – 89 .
- Parsons , AJ and Robson , MJ . 1981 . Seasonal changes in the physiology of S24 perennial ryegrass (Lolium perenne L.) 3. Partition of assimilates between root and shoot during the transition from vegetative to reproductive growth . Annals of Botany , 48 : 733 – 744 .
- Peake AS Robertson MJ Bidstrup R 2008 Optimising maize plant population and irrigation strategy on the Darling Downs using the APSIM crop simulation model Australian Journal of Experimental Agriculture 48 , 313 – 325
- Piggot , GH , Barrs , JA , Cumberland , GLB and Honore , EN . 1978 . Seasonal distribution of pasture production in New Zealand XIII. South Kaipara, Northland . New Zealand Journal of Experimental Agriculture , 6 : 43 – 46 .
- Probert , ME , Dimes , JP , Keating , BA , Dalal , RC and Strong , WM . 1998 . APSIM's water and nitrogen modules and simulation of the dynamics of water and nitrogen in fallow systems . Agricultural Systems , 56 : 1 – 28 .
- Radcliffe , JE . 1974a . Seasonal distribution of pasture production in New Zealand I. Methods of measurement . New Zealand Journal of Experimental Agriculture , 2 : 337 – 340 .
- Radcliffe , JE . 1974b . Seasonal distribution of pasture production in New Zealand II. Southland Plains . New Zealand Journal of Experimental Agriculture , 2 : 341 – 348 .
- Radcliffe , JE . 1975a . Seasonal distribution of pasture production in New Zealand VII. Masterton (Wairarapa) and Maraekakaho (Hawke's Bay) . New Zealand Journal of Experimental Agriculture , 3 : 259 – 265 .
- Radcliffe , JE . 1975b . Seasonal distribution of pasture production in New Zealand IV. Westport and Motueka . New Zealand Journal of Experimental Agriculture , 3 : 239 – 246 .
- Radcliffe , JE . 1976 . Seasonal distribution of pasture production in New Zealand X. Rangitikei district . New Zealand Journal of Experimental Agriculture , 4 : 163 – 170 .
- Radcliffe , JE and Cossen , GG . 1974 . Seasonal distribution of pasture production in New Zealand III. Central Otago . New Zealand Journal of Experimental Agriculture , 2 : 349 – 358 .
- Radcliffe , JE and Sinclair , DP . 1975 . Seasonal distribution of pasture production in New Zealand V. Gisborne Plains . New Zealand Journal of Experimental Agriculture , 3 : 247 – 251 .
- Richard DS , McBride SD 1986 . Irrigated and non-irrigated pasture production at Winchmore 1960–1985 . Winchmore Irrigation Research Station, Ministry of Agriculture and Fisheries . Technical report no. 21 .
- Richard , DS and Radcliffe , JE . 1976 . Seasonal distribution of pasture production in New Zealand XII. Winchmore, Canterbury Plains dryland and irrigated pastures . New Zealand Journal of Experimental Agriculture , 4 : 329 – 335 .
- Roberson , SM . 2006 . Predicting pasture and sheep production in the Victorian Mallee with the decision support tool GrassGro . Australian Journal of Experimental Agriculture , 46 : 1001 – 1014 .
- Roberts , AH and Thomson , NA . 1984a . Seasonal distribution of pasture production in New Zealand XVIII. South Taranaki . New Zealand Journal of Experimental Agriculture , 12 : 83 – 92 .
- Roberts , AH and Thomson , NA . 1984b . Seasonal distribution of pasture production in New Zealand XIX. Central Taranaki . New Zealand Journal of Experimental Agriculture , 12 : 93 – 101 .
- Round-Turner , NL , Scott , RS and Radcliffe , JE . 1976 . Seasonal distribution of pasture production in New Zealand XI. Otago Downland and Taieri Plain (Invermay) . New Zealand Journal of Experimental Agriculture , 4 : 321 – 328 .
- Schwinning , S and Parsons , AJ . 1996 . Analysis of the coexistence mechanisms for grasses and legumes in grazing systems . Journal of Ecology , 84 : 799 – 813 .
- Sheehy , JE , Cobby , JM and Ryle , GJA . 1979 . The growth of perennial ryegrass: a model . Annals of Botany , 43 : 335 – 354 .
- Srinivasan , MS and McDowell , RW . 2009 . Irrigation and soil physical quality: an investigation at a long-term irrigation site . New Zealand Journal of Agricultural Research , 52 : 113 – 121 .
- Tait , A , Henderson , R , Turner , R and Zheng , XG . 2006 . Thin plate smoothing spline interpolation of daily rainfall for New Zealand using a climatological rainfall surface . International Journal of Climatology , 26 : 2097 – 2115 .
- Thornley JHM , Johnson IR 2000 . Plant and crop modelling: A mathematical approach to plant and crop physiology . Caldwell, NJ , Blackburn Press .
- Verburg , K , Ross , PJ and Bristow , KL . 1996 . SWIMv2.1 User Manual , Clayton, Australia, CSIRO .
- Wang , E and Smith , CJ . 2006 . Modelling the growth and water uptake function of plant root systems: a review . Australian Journal of Agricultural Research , 55 : 501 – 523 .
- White , TA , Johnson , IR and Snow , VO . 2008 . Comparison of outputs of a biological simulation model for pasture growth with measured data under dryland and irrigated conditions in New Zealand . Grass and Forage Science , 63 : 339 – 349 .
- Whitehead , DC . 1995 . Grassland nitrogen , Wallingford, , UK : CAB International .
- Wilde RH 2003 . National soil database of New Zealand . Lincoln : Landcare Research NZ Ltd .