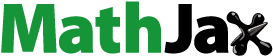
ABSTRACT
In New Zealand, a commonly sown pasture combination is perennial ryegrass with white clover. The hydrothermal time model (HTT) was used to quantify germination under different temperature (T) and water potential (WP) conditions for white clover ‘Nomad’ and perennial ryegrass ‘Stellar AR1’. Seeds were germinated in vitro under a range of T × WP combinations, and HTT models were fitted to germination time course data. Rapid germination of white clover under optimum T and WP was reflected by a much lower value for its HTT constant compared with perennial ryegrass (10.8 vs 80.7 MPa °C d). However, the lower base water potential for perennial ryegrass (−1.29 vs −0.495 MPa) and a higher threshold temperature for thermoinhibition (21.8 vs 18.3°C), meant that predicted perennial ryegrass germination was more resilient in warm dry conditions. HTT models can be used to assist decisions about the timing of perennial ryegrass and white clover sowing.
Introduction
In New Zealand, the most commonly sown pasture combination is perennial ryegrass (Lolium perenne L.) with white clover (Trifolium repens L.). The success of establishment of both species is determined by their relative ability to access resources, and this can be influenced by time of germination since resource availability varies seasonally. However, under field conditions the emergence and early seedling growth of perennial ryegrass are faster than white clover, so management strategies are frequently recommended to assist white clover establishment. These may include reducing perennial ryegrass sowing densities (Black et al. Citation2006a), temporal or spatial separation of the legume from the grass (Hurst et al. Citation2000) and autumn sowing when soil temperatures are greater than 14°C (Moot et al. Citation2000). A complication for farmers without access to irrigation is that in New Zealand, summer dry conditions can extend well into autumn months when soil temperatures begin to decline. Their dilemma becomes whether to sow into dry but warm soil conditions or alternatively wait until rain falls in order to ensure adequate moisture but at a lower soil temperature. Dry soil conditions are known to favour establishment of perennial ryegrass at the expense of white clover (McWilliam et al. Citation1970). However, there are few quantitative studies of how the combination of soil moisture and temperature affects the relative germination of these two species. Under dry autumn conditions, there is a need to understand these combined effects on rapidity of germination and the total percentage of the sown seeds that complete germination (GP) to enable appropriate sowing strategies to be adopted in the establishment phase. If the rapidity of germination is unaffected, but the percentage of sown seeds that complete germination declines, then an increased sowing density may overcome adverse soil conditions at establishment. Alternatively, a decline in germination rapidity of one species relative to the other may require temporal or spatial separation of sown species or else a change in species sown in response to variation in the soil moisture content and temperature.
In this study, the hydrothermal time model (HTT) is used to understand and quantify the relative performance of seed germination rapidity and GP under different in vitro temperature (T) and water potential (WP) conditions for perennial ryegrass and white clover. The expectation is that results can be used to predict the relative outcomes for both species of sowing into seedbeds with different soil moisture and temperature conditions as described by Sharifiamina et al. (Citation2016) for tall fescue.
The HTT model was first proposed by Gummerson (Citation1986) as Equation (1):(1)
(1) where ΘHT (MPa °Cd) is a constant hydrothermal time for a seed population; Ψ (MPa) is ambient water potential; Ψb(g) (MPa) is a base seed water potential for the gth percentile of seed population, where seed percentile is determined by temporal order of germination (g = 1% is the first percentile of the population to germinate, g = 100% is the last percentile to germinate); T (°C) is ambient temperature, Tb (°C) is the base temperature which has a single value for all percentiles of the seed population and tg (d) is the time for the gth percentile of germination.
Based on the hydrothermal time model, a seed will germinate when the accumulated seed hydrothermal time is equal to the hydrothermal time constant ΘHT. Bradford (Citation2002) stated that Tb is constant for any specific seed population, but Ψb(g) varies for each seed percentile g in the population according to a frequency distribution. This frequency distribution is usually the normal distribution although other distributions such as the Weibull distribution can also accurately describe the range of values Ψb(g) in the seed population (Watt et al. Citation2011; Mesgaran et al. Citation2013). The HTT model therefore assumes that all seeds in a population will accumulate the same thermal time ((T–Tb)tg) at any T but each percentile of seed population will accumulate a different hydro-time ([Ψ–Ψb(g)]tg) under any given WP (Bloomberg et al. Citation2009).
To predict the rapidity with which each percentile germinates, Equation (1) can be rearranged as:(2)
(2) Where GR(g) = 1/tg is the germination rate, the inverse of time to germination for the gth percentile.
According to Equations (1) and (2), with increasing temperature GR will continue to increase indefinitely since T–Tb becomes larger, ΘHT is a constant, and so t(g) must become smaller. However, increasing GR only occurs between Tb and To (base and optimal temperatures, respectively). GR reaches a maximum at optimal temperature (To) then declines with further increases in T and tends to zero at a ceiling temperature (Tc). This decline in GR with increasing temperatures above To is sometimes referred to as thermoinhibition (Horowitz and Taylorson Citation1983; Hills and van Staden Citation2003; Watt et al. Citation2011).
The reasons for increasing GR with increasing T until To are related to thermodynamics, but reasons for decreasing GR from To until Tc are less clear. Alvarado and Bradford (Citation2002) proposed that GR declines above To because the base water potential for the gth percentile (Ψb(g)) (assumed to be constant and independent of germination conditions at suboptimal temperatures) tends upwards to zero with increasing temperature above To. This means that the difference between the ambient WP and Ψb(g) diminishes and the term [Ψ–Ψb(g)] in Equation (1) becomes very small. Since ΘHT is constant, and the term (T–Tb) only increases linearly with increasing T, then tg must become very large, and seeds take longer to germinate. A physiological process which may increase Ψb(g) towards zero with supra-optimal temperatures is increasing endosperm resistance, mediated by abscisic acid (ABA) (Finch-Savage and Leubner-Metzger Citation2006; Tamura et al. Citation2006). It has been shown that ethylene has a role in counteracting this phenomenon in lettuce and chickpea seeds (Nascimento et al. Citation2000).
Alvarado and Bradford (Citation2002) modelled thermoinhibition using an HTT model that describes an upwards adjustment (towards zero) in Ψb(g) at supra-optimal temperatures. The model included an assumption that the maximum rate of thermal time accumulation will be at To, therefore seeds cannot germinate faster at supra-optimal temperatures. If this assumption is incorrect and thermal time does accumulate more rapidly with increasing temperatures above To, this will mitigate the thermoinhibition effect where Ψb(g) tends toward zero. However, as temperatures become much greater than To, the effect of T on Ψb(g) tending to zero outweighs the effect of an increase in the rate at which thermal time is accumulated. At this point, the curve of GR against T begins to decline. This alternative HTT model of thermoinhibition was first advanced by Rowse and Finch-Savage (Citation2003) and will be used in this study for HTT modelling of supra-optimal data as (1) it is reasonable to assume the thermal time accumulation rate continues to increase above To and (2) it is more consistent with observed germination data for many species, than the Alvarado and Bradford (Citation2002) model (Watt and Bloomberg Citation2012).
The Rowse and Finch-Savage model is specified as:(3)
(3) where Td is the temperature at which thermoinhibition (the upwards shift in Ψb(g)) commences, Ψb(g)sup is the adjusted Ψb at supra-optimal temperatures, Ψb(g)d is the Ψb(g) when T = Td and k is a constant. Note that Td will be less than To, in that thermoinhibition can occur at Td even though GR and GP are still increasing, i.e. To has not yet been reached. This will be the case when the increase in thermal time accumulation with increasing T still outweighs the effect of the upwards shift in Ψb(g).
The HTT model provides a complete quantitative description of GR and GP of a seed population under a range of T and WP conditions. A comparison of the HTT model parameters for perennial ryegrass and white clover that includes the hydrothermal time constant, base water potentials and base temperatures at sub- and supra-optimal temperatures, is therefore expected to provide a practical guide for ecological and agronomic management of mixed perennial ryegrass and white clover pastures in the establishment phase, under a wide range of temperature/moisture regimes.
Materials and methods
Perennial ryegrass ‘Stellar AR1’ and white clover ‘Nomad’ seeds (Seed Force Ltd, Christchurch, New Zealand 8441) were screened to remove empty or broken seeds from each population. Then they were lightly dusted with thiram (KiwiCare Corporation Limited, Christchurch, New Zealand 8062) and placed on filter papers (Whatman No.2, Advantech MFS, Inc. NO232) within 500 ml plastic containers with snap-top lids. Containers had been previously sterilised with ethanol 80%. The filter papers had been soaked in polyethylene glycol (PEG) (Sigma-Aldrich New Zealand Ltd., mol. wt. 8000) solutions of specified water potential for 24 h prior to the placement of seeds. PEG solutions were made up to a specific water potential by adding PEG to reverse-osmosis water, using Equation (4) from Hardegree and Emmerich (Citation1990):(4)
(4) Three replicates of 50 seeds were placed on the filter papers and 10 ml of the relevant PEG solutions added to each plastic container so that the filter papers were in contact with the solution. Containers were then sealed and incubated in the dark in all combinations of 5°C, 10°C, 15°C, 20°C, 25°C, 30°C and 35°C; and 0, −0.18, −0.37, −0.63 and −0.95 MPa (0 MPa water potential treatments used reverse osmosis water with no PEG added).
Plastic containers were located within each incubator randomly and rotated systematically every day. Seed germination was monitored every 8 h through the first 4 days, then inspected every 24 h for 25 days. Seeds were considered to have completed germination when a ≥ 2 mm radicle protrusion occurred, at which point they were removed.
Water potentials of the PEG solutions in each container were monitored by a Wescor 5520 vapour pressure osmometer (Wescor Inc., Logan, Utah, USA) and water potentials of germination media were checked twice weekly. Where water potentials of germination media deviated markedly from the specified treatment level, filter papers and solutions were replaced with fresh filter papers and solutions of the desired water potential.
Data analysis
Germination responses
Seed germination data were assessed as time to completed germination for each seed within each treatment combination (WP × T). Data for the three replicates in each treatment combination were analysed as three different observations for the white clover data. For perennial ryegrass, the data were combined into one set of data (i.e. 150 seeds) for preliminary analysis. The raw data identifying individual replicates were subsequently mislaid, and it was only possible to analyse the merged data for each treatment combination as one replicate.
Within the data for each replicate (n = 50 for white clover and n = 150 for perennial ryegrass), seeds were allocated a percentile number (g) according to their sequential rank in terms of time to completed germination. Germination percentage (GP) for each treatment was defined as the final accumulated seed germination (% at the end of each experiment). An assumption of the HTT model is that the maximum GP is 100%, but the recorded maximum GP for perennial ryegrass and white clover under optimal conditions were 93 and 94%, respectively. Therefore, for both species a corrected percentile number was calculated by dividing uncorrected seed percentile by the maximum GP for seed germinated under optimal conditions in this study (see Hay et al. Citation2014). The corrected percentile number was used to estimate the HTT model parameters. Germination responses were examined by plotting germination time courses and responses of GP and GR to T and WP treatments. Germination time courses are graphs showing the time to completed germination for all germination percentiles within the population, from the time the seeds were placed on the germination medium until final accumulated seed germination (GP) is reached. Germination rate (GR) of individual seeds or percentiles in a seed population was defined as the inverse of time taken by that seed or percentile to complete germination.
Prediction of HTT model parameters for sub- and supra-optimal temperatures
While the HTT model is most commonly estimated assuming a normal distribution for Ψb(g), the HTT model can also be fitted using other frequency distributions which allow more flexibility in the form of the distribution (Watt et al. Citation2011; Mesgaran et al. Citation2013). We initially fitted the HTT model using both the normal and 3-parameter Weibull distribution, but found no clear advantage to using the Weibull distribution in terms of relative model quality, assessed using the Akaike information criterion. Therefore all HTT models were estimated using a normal distribution for Ψb(g).
To estimate the HTT model, g was transformed to probit(g) (Gummerson Citation1986):(5)
(5) where probit(g) is the standard normal deviate (z) for a cumulative probability (g) in a normally distributed population with Ψb(50) as the mean value of Ψb and σΨb is one standard deviation of Ψb. The term k(T–Td) I(T>Td) adjusts Ψb(50) upwards at supra-optimal temperatures and takes the value of zero when T ≤ Td
The value of k will indicate how strongly thermoinhibition responds to supra-optimal temperatures. A low k value indicates a weaker thermoinhibition response and a high k-value indicates a strong response – for example, the thermoinhibition response is stronger for true potato seeds (k = 0.12, (Alvarado and Bradford Citation2002)) than for carrot and onion seeds (k = 0.030 and 0.039 respectively (Rowse and Finch-Savage Citation2003)).
Using probit-transformed germination data for all combinations of water potential and temperature treatments, HTT model parameters (ΘHT, Ψb(50), Tb, σΨb, k and Td) were simultaneously estimated using the ‘nls’ function (an iterative least-squares procedure) in R Software (R Development Core Team Citation2018).
Because the non-differentiable term k(T–Td)I(T>Td) affects the nls model convergence, the above model was fitted on a grid of values Td ranging from 15 to 25 with step 0.1. For each proposed value of Td, the Akaike information criterion (AIC) was evaluated, and the model with the lowest value of AIC was selected as the best model.
A feature of germination data obtained from sequential counts of germinated seeds is that they are observed cumulative proportions of the total number of seeds in each replicate. Thus, each percentile is not independent of other seeds in the replicate, since the time to completed germination for each percentile is conditioned by the time to germination of the preceding seeds. A necessary condition for unbiased estimation of parameter errors in a statistical model is that data are independent. Thus, although HTT model parameter values themselves may not be biased, their standard errors will and can be 3–4 times smaller than errors calculated from data where the condition of independence is met (Onofri et al. Citation2014).
Therefore, following Onofri et al. (Citation2014) the standard errors for the HTT model parameters were estimated using the grouped jackknife method (repeated model fitting where one germination dish (replicate) was randomly deleted from the data), which accounted for repeated observations on the same replicate. Note that the gridding procedure is part of fitting the model and was repeated for every model fitting to obtain the standard error and 95% confidence interval for the change point Td.
Results
Observed germination behaviour
Germination time courses and final germination percentage (GP)
Under optimum WP (0 MPa), the maximum GP of perennial ryegrass and white clover were 93% (at T = 15°C) and 94% (at T = 20°C), respectively. These maxima were used to calculate corrected germination percentiles (g) (the ratio of uncorrected seed percentile/maximum GP) for all treatments. Germination time courses, using corrected g, are shown for both perennial ryegrass and white clover in .
Figure 1. Germination time courses of perennial ryegrass (PR) and white clover (WC) seeds for all temperature treatments, at water potentials = 0, −0.37 and −0.63 MPa. The black symbols are observed data, the red lines are the time courses predicted by the HTT model and the shaded areas show the 95% confidence limits on the HTT model predictions. For each germination time course graph, the y-axis (Germination %) encompasses the range 0–100%. HTT models are not plotted for treatments where no germination occurred.
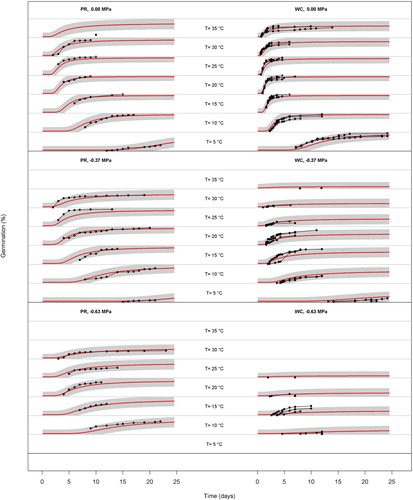
Under optimum moisture (WP = 0 MPa), both species had the lowest final GP at 5°C and 35°C, and germination of white clover was both faster than perennial ryegrass at each constant temperature and commenced sooner. It took no more than three days for white clover seeds to start germination when temperatures were between 10°C and 35°C (). In the same range, germination of perennial ryegrass started between 2–8 days after sowing ().
Perennial ryegrass achieved GP ≥ 40% for all water potential treatments except −0.95 MPa over a wide range of temperatures (). While GP did decline with temperature extremes (5°C and >25°C), it was notably resilient to decreasing WP.
In contrast, white clover GP declined markedly above 25°C, even when WP = 0 MPa and (with the exception of anomalously high GP for 15°C/ −0.63 MPa) declined to <20% when WP was −0.63 or −0.95 MPa, even at optimum temperatures ().
Combined effects of different water potentials and temperatures on germination rate (GR)
Under optimum conditions of T and WP, white clover achieved very rapid germination, but GR declined rapidly for temperatures above and below To, and as water potentials decreased progressively down to −0.95 MPa (A).
Figure 2. HTT model predictions of germination behaviour by perennial ryegrass (PR) and white clover (WC). A, Germination rate (GR) versus temperature when WP = 0, −0.37 and −0.63 MPa. The lines are the GR for 10th, 30th, 50th, 70th and 90th percentiles predicted by the HTT model, shading from light grey (10th percentile) to black (90th percentile). Missing lines for a percentile indicate that germination of that percentile was not predicted to occur at the specified water potential. B, Base water potentials (Ψb(g)) vs temperature. The horizontal lines (where T ≤ Td) are Ψb(g) for 10th, 30th, 50th, 70th and 90th percentiles predicted by the HTT model, shading from light grey (10th percentile) to black (90th percentile). The sloped lines (where T > Td) have a slope of k = 0.067 and 0.025 for perennial ryegrass and white clover, respectively.
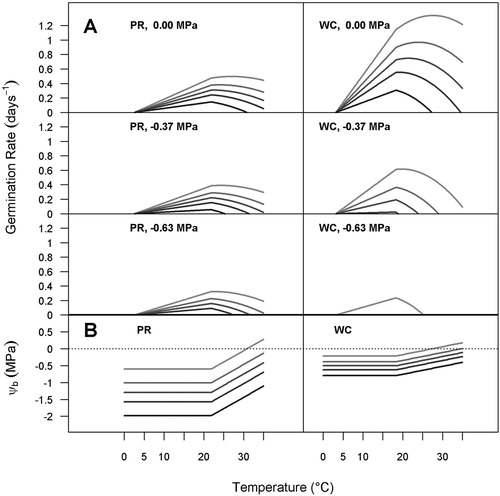
The GR of perennial ryegrass was much lower than white clover under optimal conditions but relatively insensitive to variation in water potential. For example, for 20°C, GR(50) (germination rate of the 50th percentile) of perennial ryegrass decreased by 26% between WP = 0 MPa and −0.37 MPa. At the same temperature white clover GR(50) decreased by 80% between WP = 0 MPa and −0.37 MPa (A).
HTT model of germination
Model parameters
Estimated HTT model parameters are shown in . The estimated HTT constants for perennial ryegrass and white clover were 80.7 and 10.8 MPa °Cd respectively, and white clover compared with perennial ryegrass had a slightly higher Tb (3.22 vs 2.56°C respectively). The much smaller HTT constant for white clover, coupled with a similar Tb, is consistent with the observation that white clover germinated much more rapidly than perennial ryegrass under optimum conditions of WP since it needed to accumulate far less hydrothermal time in order to complete germination. However, perennial ryegrass germination was more resilient to increasing WP at supra-optimal temperatures, which is consistent with it having a greater Td compared with white clover (21.8 vs 18.3°C respectively).
Table 1. Estimated hydrothermal model parameters (standard errors of estimates in brackets) for ‘Stellar AR1’ perennial ryegrass and ‘Nomad’ white clover germinated at five water potentials (0, −0.18, −0.37, −0.63 and −0.95 MPa) at sub- and supra-optimal temperatures. RMSEs are shown for both g and germination rate (GR = 1/ tg).
HTT model predictions
For comparison against actual germination time courses, germination time courses predicted using the HTT models were plotted in , along with 95%confidence intervals for the predicted values. Note that where predicted germination values were close to 0 or 100%, plotted confidence intervals for the predicted values were constrained not to exceed these values.
Predicted GR of 10th, 30th, 50th, 70th and 90th percentiles were plotted against T for WP = 0, −0.37 and −0.63 MPa (A).
Predicted GR values of white clover were higher than perennial ryegrass for all percentiles under optimum conditions (A). For both species, the predicted maximum GR occurred at warmer temperatures for lower percentiles (10th, 30th percentiles) compared with cooler temperatures for the 70th and the 90th percentiles. In other words, thermoinhibition of GR commences at warmer temperatures for the faster, earlier germinating seeds, but commences at cooler temperatures for the slower-germinating seeds. For white clover, the effect of thermoinhibition on GR was increasingly evident with lower WP, whereas the GR for perennial ryegrass declined with temperature less rapidly under dry conditions.
There were distinct differences between the two species for both the predicted seed base water potentials (Ψb(g)) and their response to supra-optimal temperatures (B). The Ψb(g) for white clover are much closer to zero; therefore white clover accumulates much less ‘hydro- time’ ((Ψ–Ψb(g)) tg) under any specific WP. This does not matter under moist conditions, as the HTT constant for white clover is much lower than for perennial ryegrass (10.8 vs 80.7). Thus, white clover needs far less hydrothermal time accumulation to germinate, and under moist conditions it will rapidly accumulate the necessary amount. However, B shows that when soil WP becomes drier at warm temperatures, the higher seed percentiles for white clover are likely to have Ψb(g) that is close to or even greater than ambient WP, and thus cannot accumulate hydrothermal time.
For perennial ryegrass, Ψb(g) increased more rapidly with increasing temperature above Td, than for white clover. The estimated slopes (k) of Ψb(g) vs T–Td at supra-optimal temperatures (k) were 0.067 and 0.025 for perennial ryegrass and white clover, respectively (, B). However, while the upwards shift in Ψb(g) is therefore more influenced by increasing temperature in perennial ryegrass, it occurs at a higher threshold temperature (21.8vs 18.3°C). And because the Ψb(g) of perennial ryegrass are so much lower than for white clover, perennial ryegrass still accumulates more ‘hydro-time’ and therefore germinates more rapidly even at 30 and 35°C.
HTT model goodness of fit
Almost all observed germination time courses were within the 95% confidence intervals for HTT predictions, although the time courses for the 15°C /−0.63 MPa treatments for white clover were anomalously high (). and the HTT model performed less well at supra-optimal temperatures. For example, the HTT predicted good germination of perennial ryegrass at 35°C/ 0 MPa, but thermoinhibition led to no more than 14% actual germination (). The HTT model also predicted some perennial ryegrass germination at 35°C under drier conditions (A), but no germination actually occurred for WP≤ −0.37 MPa at this temperature.
A comparison of predicted vs actual cumulative germination (g) and GR is shown for both species for suboptimal and supra-optimal temperatures (). This shows small but systematic bias in prediction for both variables. Two factors are likely to have contributed to this bias:
For cumulative germination counts in trays, all counted seeds will have completed germination prior to the time of observation (and therefore observed g for any t will always be an underestimate). This error is proportionally greater when t is small, i.e. when the lowest percentiles are germinating at faster GRs. The HTT model is estimated over the whole range of g, and therefore tends to (1) overestimate g when observed g is small since these observations are proportionally the most downwards biased, and (2) underestimate g when observed g is large since these are less proportionally downwards biased.
The HTT model was less accurate for low seed percentiles at supra-optimal temperatures, especially for white clover. For white clover, the actual GRs for low percentiles at temperatures slightly higher than Td (20 or 25°C) were extremely rapid, and the HTT model markedly underpredicted GR. This poor performance of the HTT supra-optimal model for white clover contributed to its marked underprediction of GR for the fastest-germinating white clover seeds. Underprediction of GR for the fastest germinating perennial ryegrass seeds was not as marked (B).
Figure 3. HTT model predictions against observations for perennial ryegrass (PR) and white clover (WC). A, Cumulative germination proportion (g). B, Germination rate (GR). The solid line is the correlation between predicted and observed data, the dashed line is the line of equality (y = x). Axis scales for GR differ between PR and white clover WC.
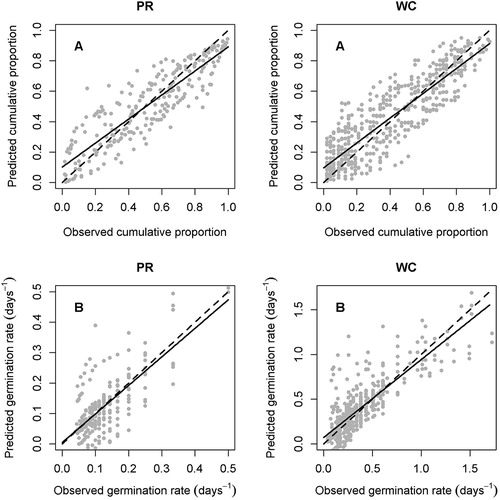
Discussion and conclusions
Observed germination behaviour and the HTT model
The HTT model provided reasonably accurate descriptions of in vitro germination behaviour of both perennial ryegrass and white clover. Results were consistent with previous studies that used the HTT model for predicting seed germination behaviours according to the interaction between their physiological responses and soil T and WP (Bradford Citation1995; Rowse and Finch-Savage Citation2003). In addition, the probit-based model has been confirmed as helpful to an understanding of seed germination processes (Hay et al. Citation2014).
Particularly for white clover but also for perennial ryegrass, the HTT model did not accurately model GR of fast-germinating lower percentiles. The reason for this is not clear. One possible explanation is that the thermoinhibition model used here did not account for time of exposure to supra-optimal temperatures, instead assuming that for all g, Ψb(g) adjusts upwards by an equal amount (k(T–Td)) for any specific supra-optimal temperature. However it is possible that the fastest-germinating seeds were not fully thermoinhibited, since they were exposed to supra-optimal temperatures for only a short time (see Bloomberg et al. Citation2009) and therefore germinated more rapidly than predicted by the HTT model. Watt and Bloomberg (Citation2011) used a 3-parameter Weibull distribution to model Ψb(g), and this had the advantage that the values for the lowest percentiles could be ‘anchored’ using the location parameter for the distribution so they did not move towards zero at supra-optimal temperatures. They therefore accumulated ‘hydrotime’ at the same rate as for suboptimal temperatures, but their accumulation rate for thermal time continued to increase linearly as a function of T–Tb, and this resulted in more accurate predictions of the very fast GRs for the lowest seed percentiles at supra-optimal temperatures. Although overall using the Weibull distribution for Ψb(g) did not result in better HTT models for white clover compared with the normal distribution, it is worth investigating its usefulness in describing the very rapid germination of the lowest percentiles of white clover at supraoptimal temperatures.
This study revealed differences in germination responses of the two species to WP and T treatments, and these differences have implications for their germination under field conditions. Previous studies showed that seeds of both species germinated under moist conditions after accumulating a similar amount of thermal time (Moot et al. Citation2000; Larsen and Bibby Citation2005; Black et al. Citation2006a; Lonati et al. Citation2009; Monks et al. Citation2009). However, important differences in germination behaviour between the two species appear to be driven by differences in their responses to WP, interacting with thermal time accumulation, so that they must accumulate markedly different hydrothermal time before they complete germination.
Differences in seed germination among cultivars
This study used germination data for white clover ‘Nomad’ and perennial ryegrass ‘Stellar AR1’, therefore results and conclusions from this study may not be applicable to other cultivars of these species. For ‘Nomad’ white clover and ‘Stellar AR1’perennial ryegrass, GRs at 0 MPa and over a range of temperatures were similar to previous results for ‘Ladino’ and ‘Demand’ white clover and ‘Nui’, ‘Commando’ and ‘Victorian’ perennial ryegrass (McWilliam et al. Citation1970; Moot et al. Citation2000; Black et al. Citation2006b). Thus, results show some consistency within species given these studies used different cultivars to those in our study. Reported Tb/To for white clover ‘Demand’ (−0.6°C to 3.5°C/27°C), ryegrass ‘Commando’ (3.2°C/ 27°C) and ryegrass ‘Nui’ (2.6°C/27°C) were generally similar to those found for white clover ‘Nomad’ and perennial ryegrass ‘Stellar AR1’ in this study – although the To = 27°C reported by Black et al. (Citation2006b) for white clover ‘Demand’ seems high compared with the To of approximately 20°C for white clover ‘Nomad’ found in this study. This discrepancy may be due to the different definitions of To. In our study, To was defined as the temperature treatment where GP was highest and achieved in the shortest time. Black et al. (Citation2006b) defined To as the temperature where the maximum GR of the 75th percentile occurred, and commented that for ryegrass ‘Nui’ and white clover ‘Demand’, the ‘observed data suggest little change in maximum germination rate between 22°C and 27°C, which implies that a single maximum rate across this optimum range may be more appropriate.’ Using this definition of To based on the maximum GR for a specific seed percentile, the temperature where GR of the 70th percentile was at a maximum for perennial ryegrass ‘Stellar AR1’ was 25°C (), which is reasonably similar to the 27°C reported by Black et al. (Citation2006b) for ryegrass ‘Nui’. However, the temperature where GR of the 70th percentile was at a maximum for white clover ‘Nomad’ was only 20°C, much lower than the 27°C reported by Black et al. (Citation2006b) for white clover ‘Demand’.
From the above discussion, an important conclusion is that differences in study methodology may lead to differences in reported germination behaviour between species and cultivars that are not due to their inherent germination characteristics. One advantage of the HTT model is that its parameters describe germination behaviour of both the whole seed population and of individual percentiles within the population, which is desirable since germination responses to T and WP may vary widely and non-linearly among different seed percentiles within a single population. The HTT model also provides a consistent methodology for estimation of cardinal temperatures for germination as well as responses to soil WP.
Responses of GR to T and WP
Two important assumptions of the Rowse and Finch-Savage (Citation2003) model are that seeds can accumulate thermal time as a linear function of (T–Tb). However, above Td the resulting increase in seed GR is progressively inhibited by ‘thermoinhibition’, where seed base water potential (Ψb(g)) increases towards zero with increasing supra-optimal temperatures. This has the consequence of progressively reducing GR and delaying germination over the range Td to Tc since seeds accumulate hydrothermal time more slowly (Watt et al. Citation2011). Because thermoinhibition is mediated by changes in Ψb(g), it is therefore more strongly expressed under low WP conditions.
For both perennial ryegrass and white clover, trends in GR versus temperature were in agreement with the HTT model proposed by Rowse and Finch-Savage (Citation2003). For both species GR increased linearly with temperature from Tb upwards (A) until they reached a broad optimum range. They then began to decrease towards zero at ceiling temperature Tc (although for moister WP treatments, ceiling temperatures were not reached by all percentiles at the maximum temperature treatment of 35°C). The optimum temperature for GR did not have a single unique value but was lower for higher percentiles of both species (A). These variations between higher and lower percentiles in responses of GR to temperature have been shown to occur in germinating seeds of many species (Watt and Bloomberg Citation2012).
The HTT model predicted that Ψb(g) of both perennial ryegrass and white clover both began to shift upward above Td (B). However, the k value for perennial ryegrass was three times greater than for white clover, implying more rapid thermoinhibition of perennial ryegrass. This seems inconsistent with and , where white clover GP and GR declined more severely under dry and warm conditions than did perennial ryegrass GP. An explanation is that the estimated seed base water potential of perennial ryegrass was much lower than for white clover (Ψb(50) = −1.29 MPa and −0.495 MPa, respectively ()). This lower (more negative) base water potential of perennial ryegrass, and its higher temperature threshold for commencement of thermoinhibition were both consistent with the resilient germination of this species in dry soils compared with white clover. This means that (1) even under optimal temperature conditions, white clover germination will be more strongly inhibited by lower WP, and (2) under supra-optimal temperatures, even a relatively small amount of thermoinhibition (increase in Ψb(g)) for white clover will result in a markedly reduced rate of hydrothermal time accumulation.
Implications for pasture establishment
The evidence of temperature-mediated declines in GR and GP of white clover at relatively low T and high WP mean that white clover germination is more likely to be inhibited by dry soils under warm conditions, possibly an adaptation to the ‘false breaks’ in dry summer conditions that may occur in white clover’s natural range. This is consistent with the idea of thermoinhibition having a role in ‘hedging’ the risk of suicidal germination of seed populations caused by rainfall during a false break (Watt and Bloomberg Citation2012).
In contrast, thermoinhibition of perennial ryegrass occurred at higher T, suggesting that it is a species with a weaker adaptive response to the risk of germination under dry warm conditions. This may be an advantage for an agricultural species where rapid germination under a range of conditions is desirable, and germination failure is not ‘fatal’ since a failed sowing can be resown. It also means that in the event of rainfall after sowing, the weak thermoinhibition allows rapid germination of perennial ryegrass and consequently rapid establishment of canopy cover with fewer opportunities for weeds to establish. These attributes are consistent with its reputation as an aggressive competitor at establishment (Black et al. Citation2006a).
This study has shown that time to completed germination of perennial ryegrass and white clover, and subsequent development of mixed white clover/perennial ryegrass pastures, depend on hydrothermal time requirements. According to Moot et al. (Citation2000) and this research, GR under optimum WP and T was faster for white clover than perennial ryegrass, as reflected in a much lower hydrothermal time requirement for germination (ΘHT = 10.8 MPa °C d for white clover vs 80.7 MPa °C d for perennial ryegrass). This faster germination of white clover could compensate for the rapid growth rate of perennial ryegrass seedlings reported by Brougham (Citation1953) if a mixed pasture is the objective. However, if there is a lack of autumn moisture, the impact on white clover in the pasture mixture is greater because perennial ryegrass germination is more tolerant of warm, dry conditions than white clover. In practice, these conditions can be expected to result in perennial ryegrass dominant pasture with minimal white clover cover. Under these circumstances, it may therefore be necessary to sow white clover earlier and delay sowing of perennial ryegrass to allow development of a mixed pasture cover (Praat et al. Citation1996; Moot et al. Citation2000).
One advantage of HTT models is that they can be used to predict seed germination under specified conditions of soil temperature and moisture (Finch-Savage and Leubner-Metzger Citation2006; Garcia et al. Citation2013). Once these HTT models are validated in the field, they could be used for predictive purposes. Given a set of climate and soil moisture/temperature scenarios for autumn sowing, it may be possible to predict the timing and completeness of germination of perennial ryegrass and white clover. This would allow farmers to predict the likely relative germination of the two species, and make real-time decisions about sowing densities and sowing dates of both species, or even whether to sow at all if germination is likely to be low.
Acknowledgements
Seeds used in this study were provided by Seed Force Ltd. The authors acknowledge the financial support of Farshid Ghaderifar (Gorgan University of Agricultural Sciences and Natural Resources) and Roland Stead, and the technical assistance of Stephen Stilwell (Lincoln University).
Disclosure statement
No potential conflict of interest was reported by the authors.
Additional information
Funding
References
- Alvarado V, Bradford KJ. 2002. A hydrothermal time model explains the cardinal temperatures for seed germination. Plant, Cell and Environment. 25:1061–1069. doi: 10.1046/j.1365-3040.2002.00894.x
- Black AD, Moot DJ, Lucas RJ. 2006a. Spring and autumn establishment of Caucasian and white clovers with different sowing rates of perennial ryegrass. Grass and Forage Science. 61:430–441. doi: 10.1111/j.1365-2494.2006.00552.x
- Black AD, Moot DJ, Lucas RJ. 2006b. Development and growth characteristics of Caucasian and white clover seedlings, compared with perennial ryegrass. Grass and Forage Science. 61:442–453. doi: 10.1111/j.1365-2494.2006.00553.x
- Bloomberg M, Sedcole JR, Mason EG, Buchan G. 2009. Hydrothermal time germination models for radiata pine (Pinus radiata D. Don). Seed Science Research. 19:171–182. doi: 10.1017/S0960258509990031
- Bradford KJ. 1995. Water relations in seed germination. In: Kigel J, Galili G, editors. Seed development and germination. New York: Marcel Dekker; p. 351–396.
- Bradford KJ. 2002. Applications of hydrothermal time to quantifying and modeling seed germination and dormancy. Weed Science. 50:248–260. doi: 10.1614/0043-1745(2002)050[0248:AOHTTQ]2.0.CO;2
- Brougham RW. 1953. Seeding rates of short rotation ryegrass. Proceedings of the New Zealand Grassland Association. 14:172–181.
- Finch-Savage WE, Leubner-Metzger G. 2006. Seed dormancy and the control of germination. New Phytologist. 171:501–523. doi: 10.1111/j.1469-8137.2006.01787.x
- Garcia AL, Recasens J, Forcella F, Torra J, Royo-Esnal A. 2013. Hydrothermal emergence model for ripgut brome (Bromus diandrus). Weed Science. 61:146–153. doi: 10.1614/WS-D-12-00023.1
- Gummerson RJ. 1986. The effect of constant temperatures and osmotic potentials on the germination of sugar beet. Journal of Experimental Botany. 37:729–741. doi: 10.1093/jxb/37.6.729
- Hardegree SP, Emmerich WE. 1990. Effect of polyethylene glycol exclusion on the water potential of solution-saturated filter paper. Plant Physiology. 92:462–466. doi: 10.1104/pp.92.2.462
- Hay FR, Mead A, Bloomberg M. 2014. Modelling seed germination in response to continuous variables: use and limitations of probit analysis and alternative approaches. Seed Science Research. 24:165–186. doi: 10.1017/S096025851400021X
- Hills PN, van Staden J. 2003. Thermoinhibition of seed germination. South African Journal of Botany. 69:455–461. doi: 10.1016/S0254-6299(15)30281-7
- Horowitz M, Taylorson RB. 1983. Effect of high temperatures on imbibition, germination, and thermal death of velvetleaf (Abutilon theophrasti) seeds. Canadian Journal of Botany. 61(9):2269–2276. doi: 10.1139/b83-248
- Hurst R, Black AD, Lucas RJ, Moot DJ. 2000. Sowing strategies for slow-establishing pasture species on a North Otago dairy farm. Conference Contribution–Paper in Published Proceedings. http://hdl.handle.net/10182/4566.
- Larsen SU, Bibby BM. 2005. Differences in thermal time requirement for germination of three turfgrass species. Crop Science. 45:2030–2037. doi: 10.2135/cropsci2004.0731
- Lonati M, Moot DJ, Aceto P, Cavallero A. 2009. Thermal time requirements for germination, emergence and seedling development of adventive legume and grass species. New Zealand Journal of Agricultural Research. 52:17–29. doi: 10.1080/00288230909510485
- McWilliam JR, Clements RJ, Dowling PM. 1970. Some factors influencing the germination and early seedling development of pasture plants. Australian Journal of Agricultural Research. 21:19–32. doi: 10.1071/AR9700019
- Mesgaran MB, Mashhadi HR, Alizadeh H, Hunt J, Young KR, Cousens RD. 2013. Importance of distribution function selection for hydrothermal time models of seed germination. Weed Research. 53:89–101. doi: 10.1111/wre.12008
- Monks D, Sadatasilan K, Moot D. 2009. Cardinal temperatures and thermal time requirements for germination of annual and perennial temperate pasture species. Agronomy New Zealand. 39:95–109.
- Moot DJ, Scott WR, Roy AM, Nicholls AC. 2000. Base temperature and thermal time requirements for germination and emergence of temperate pasture species. New Zealand Journal of Agricultural Research. 43:15–25. doi: 10.1080/00288233.2000.9513404
- Nascimento WM, Cantliffe DJ, Huber DJ. 2000. Thermotolerance in lettuce seeds: association with ethylene and endo-beta-mannanase. Journal of the American Society for Horticultural Science. 125:518–524. doi: 10.21273/JASHS.125.4.518
- Onofri A, Mesgaran MB, Neve P, Cousens RD. 2014. Experimental design and parameter estimation for threshold models in seed germination. Weed Research. 54(5):425–435. doi: 10.1111/wre.12095
- Praat JP, Ritchie WR, Baker CJ, Hodgson J. 1996. Target populations for direct-drilled ryegrass and tall fescue. Proceedings of the New Zealand Grassland Association. 55:133–137.
- R Development Core Team. 2018. R: a language and environment for statistical computing. Vienna: R Foundation for Statistical Computing. http://www.R-project.org/.
- Rowse HR, Finch-Savage WE. 2003. Hydrothermal threshold models can describe the germination response of carrot (Daucus carota) and onion (Allium cepa) seed populations across both sub- and supra-optimal temperatures. New Phytologist. 158:101–108. doi: 10.1046/j.1469-8137.2003.00707.x
- Sharifiamina S, Moot DJ, Bloomberg M. 2016. Calculating hydrothermal time to quantify seed germination of tall fescue. Journal of New Zealand Grasslands. 78:163–168. doi: 10.33584/jnzg.2016.78.499
- Tamura N, Yoshida T, Tanaka A, Sasaki R, Bando A, Toh S, Lepiniec L, Kawakami N. 2006. Isolation and characterization of high temperature-resistant germination mutants of Arabidopsis thaliana. Plant and Cell Physiology. 47:1081–1094. doi: 10.1093/pcp/pcj078
- Watt MS, Bloomberg M. 2012. Key features of the seed germination response to high temperatures. New Phytologist. 196:332–336. doi: 10.1111/j.1469-8137.2012.04280.x
- Watt MS, Bloomberg M, Finch-Savage WE. 2011. Development of a hydrothermal time model that accurately characterises how thermoinhibition regulates seed germination. Plant, Cell and Environment. 34:870–876. doi: 10.1111/j.1365-3040.2011.02292.x