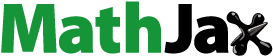
ABSTRACT
This paper aimed to estimate the heritability and genetic correlations for processability (milk coagulation properties, cheese yield, and heat stability), milk production, and milk composition of dairy sheep in a New Zealand flock. Test-day records from 169 ewes were obtained during the 2021–2022 season. Heritability estimates for yield traits (milk, fat, protein, and lactose yield) were moderate and ranged from 0.33 to 0.38. Whereas heritability estimates for processability traits were lower and ranged from 0.17 to 0.27. The genetic correlations indicated that poorer milk coagulation properties were associated with lower mammary health indicated by higher SCS, higher pH, and lower lactose content. Additionally, a higher ratio of casein to calcium i.e. lower availability of soluble calcium was associated with poorer milk coagulation properties. Higher yields of milk, fat, protein, and lactose were associated, but to a smaller degree, with better MCP. It is proposed that genetic improvement of dairy sheep for higher yields of fat and protein, and for lower SCS, could indirectly improve milk processability for cheese-making. However, it is recommended that larger studies involving more dairy sheep flocks are conducted to validate the present results before developing a selection index for this industry.
Introduction
The New Zealand sheep dairy industry is developing and complementing the existing cow dairy industry, with estimates to generate $750 M through exports by 2035 (Scale up Citation2022). Exports currently mainly consist of milk powder and infant formula. This sector is also diversifying the domestic market with alternative products to consumers looking for specific health benefits or experiencing intolerance to milk from other species. Additionally, restaurants and enthusiasts of gourmet food seek sheep milk products, particularly cheeses (Lees and Lees Citation2018). Furthermore, dairy sheep farming has the potential to have a lower environmental impact (Downie-Melrose Citation2014), but this is still under further investigation (McCoard Citation2023). Moreover, the pay for sheep milk is appealing to dairy farmers as it reached between $16 to $17 per kilogram of milk solids in 2021 (Gullery Citation2021).
However, in general, dairy sheep in New Zealand have been considered low producing when compared to dairy sheep in Europe, which has been attributed to a slow rate of genetic progress and suboptimal feed management practices for pasture-based systems (Lees and Lees Citation2018). There has been noted a large gap between low (60–90 litres of milk in a season) and high producing dairy ewes (300–400 litres a year) in New Zealand flocks, evidencing the potential of genetic improvement (MPI & Massey University Citation2020; Gullery Citation2021). The primary genetic makeup of New Zealand dairy sheep comprises East-Friesian breed (Peterson and Prichard Citation2015) alongside some genetic lines of meat breeds such as Coopworth and Border Leicester. Additionally, some flocks have more recently incorporated other breeds such as the Awassi and Lacaune, introduced by the major companies (New Zealand Sheepbreeders Association Citation2024a, Citation2024b, Citation2024c).
Genetic improvement of livestock is one of the ways of increasing animal production efficiently and sustainably, with due consideration for animal health and welfare (Flint and Woolliams Citation2008). However, the significant costs associated with certified milk metres, data collection, pedigree management, and expertise in statistical genetic analyses have restricted advanced breeding programs to large sheep dairy companies. Nonetheless, implementing selection programs in smaller flocks can yield positive economic and social impacts, preserve breeds, and facilitate genetic progress even in small animal populations.
Estimating genetic parameters, such as heritability and genetic correlations, is crucial for developing selection indexes and predicting response to selection (Lopez-Villalobos Citation2012), as selection of one trait can result in undesirable side effects (Flint and Woolliams Citation2008). Therefore, it is important to consider the impact that the selection of dairy sheep on production traits solely would have on milk processability, since sheep milk is mainly used for processing into dairy products, rather than being consumed as fresh milk (Bencini and Pulina Citation1997). The genetic bases of processability traits have yet to be explored in dairy sheep in New Zealand.
Considering this context, the objective of this study was to estimate heritability, genetic and phenotypic correlations of milk processability traits, milk production, and milk composition in dairy sheep from a New Zealand flock. These preliminary estimations of genetic parameters will provide insights for the creation of a selection index to rank ewes and rams in dairy sheep flocks dedicated to cheese production and highlight the importance of advancing genetic research within the sheep dairy industry on a wider scale.
Materials and methods
An animal ethics approval was obtained for this study (MUAEC Protocol 21/45). Data were collected from a commercial flock of 169 ewes in Masterton, New Zealand. Description of the animals and of the farm has been previously reported (Marshall et al. Citation2023). The development of the breed started 27 years ago using East-Friesian genetics, and some Coopworth and Border Leicester genetics.
The farm operates on an extensive seasonal grazing system; the ewes graze clover and lucerne pasture and have limited access to supplementary feed during milking. Mating with rams started in mid-March. Lambs were born between July and November, with median lambing date of 20th August 2021. The farm has an exclusive suckling period, so milking for artisan cheese production starts after the lambs are fully weaned at the discretion of the farmer. In the 2021–2022 season, the average suckling period was 57 days. Milk collection for cheese production happened from the 1st of October until mid-February. Milking frequency was twice-a-day (TAD) during the entire month of October and was shifted to once-a-day (OAD) on the 1st of November, this is a common practice at the farm to align milk production with pasture availability.
Masterton has hot and dry summers and wet winters with some frosts. Maximum temperature was 30.2°C, and minimum temperature was −0.2°C during the milk production season. January was the hottest month with the lowest rainfall (13.4 mm) (Meteorological Service NZ Ltd. Citation2022). Pasture quality varied significantly. In October 2021 pasture contained 12.0 MJ of metabolisable energy/kg DM and 26.7% of crude protein (% DM). The level of metabolisable energy and crude protein in the pasture dropped 32% and 47%, respectively in January 2022, whereas the level of non-detergent-fibre increased 40%, resulting in a decrease of 31% in organic dry matter digestibility. More detailed information on pasture nutritional value is provided by Marshall et al. (Citation2023).
Test-day records
A total of 639 test-day records from 169 dairy sheep were gathered from the start of the milking season (04 October 2021) until 31 January 2022, for information on milk production, composition, and processability. At least two milk tests were obtained from each ewe from 35 to 182 days in milk, 78% of the flock had a minimum of four records, 17% had a minimum of three records, and 5% had two records throughout the season.
Milk production
The milk yield from individual ewes was manually recorded as the total volume taken from individual test buckets attached to the cups and to the milk line, and a representative milk sample was taken from the total milk produced by each ewe for compositional and processability analyses. The milk samples were immediately stored in a cooler with ice during collection for transportation within four hours to Palmerston North.
For the statistical analysis of the data from twice-a-day milking, the volume from the afternoon milking and the volume of the following morning milking were added to obtain the test-day milk yield. The corresponding composition traits for that day were the weighted afternoon and morning milk volumes. There was no statistical difference in MCP between afternoon and morning samples, therefore MCPs were averages of afternoon and morning samples. Once-a-day milking happened in the afternoons.
Information on pedigree, litter size, and parity number of ewes were available for this study.
Milk composition
Sodium azide was added to milk samples (to final concentration of 0.025% w/w) on arrival in Palmerston North, prior to aliquoting for the different analyses. An aliquot was analysed by Milk Test NZ Ltd. (Hamilton, NZ) using a Combi FOSS instrument (Foss Analytics). The composition analysis included percentages of fat, protein, casein, lactose, and SCC (cells/mL) converted into somatic cell scores (SCS) using a Log2 transformation. The analyses for percentage of casein and urea (mg/100 mL) were performed using a Fourier-transform Infrared (FTIR) milk-analyser MilkoScan FT6000 (Foss Analytics) calibrated for sheep milk samples. Milk samples were also submitted at a contract laboratory (Massey Nutrition Lab) for analysis of total calcium content (mg/100 mL). The ratio of casein to protein was calculated as casein percentage divided by protein percentage, multiplied by 100. The same rule applied for calculation of ratio of casein to fat, and ratio of casein to calcium content.
Milk processability
Milk coagulation properties
Traditional milk coagulation properties (MCP) were assessed using a Formagraph instrument (Foss Analytics). For each individual milk sample, 10 mL was warmed to 31°C before adding the 200 μL of the rennet solution to achieve 0.0513 IMCU/mL of milk (Pazzola et al. Citation2014). The rennet solution was prepared with 0.24 g of commercial calf rennet powder 1100 IMCU/g (92% chymosin and 8% pepsin) diluted in 100 mL of pure water.
After rennet addition, Formagraph analysis was conducted for 30 minutes at 31°C. Curd firmness (measured in millimetres) was recorded at 0.125-minute intervals (7.5 s). The traditional MCP extracted from the Formagraph indicated the quality of milk for cheesemaking, these included rennet coagulation time (RCT, min), curd firmness at 30 minutes (A30, mm), and time to reach curd firmness of 20 mm (K20, min) (McMahon and Brown Citation1982).
Milk pH was measured at 31°C, using a calibrated pH meter (EcoScan Model pH5) on the same day as milk coagulation properties were obtained.
Individual laboratory cheese yield
Due to practical constraints, a subset of 372 samples and a minimum of two records per ewe, taken during once-a-day milking period, were used for manufacturing of fresh soft curds to measure individual laboratory cheese yield (ILCY), following protocols from Othmane et al. (Citation2002), with temperature adjustment to 31°C, as described in Marshall et al. (Citation2024).
Heat stability
A total of 344 milk samples and a minimum of two records per ewe, taken during once-a-day milking period, were used to measure heat coagulation time (HCT) as described by (Cole and Tarassuk Citation1946). Whole milk in glass tubes were immersed and oscillated in an oil bath set at 140°C. The time at which coagulation particles became visible was recorded.
Statistical analyses
Descriptive statistics (mean, standard deviation, minimum and maximum values) for MCPs, milk production and composition traits, SCS and pH were obtained using the MEAN procedure in SAS version 9.4 software (SAS 2004).
Estimates of (co)variance components for animal additive genetic variance (σ2a), permanent environment variance (σ2c), and residual (σ2e) were obtained by fitting univariate and bivariate repeatability animal models as implemented in the JWAS package (Cheng et al. Citation2018) of Julia version 1.7.3, using Bayesian estimation method and Markov chain Monte Carlo procedures. Marginal posterior distributions of all unknowns were estimated using the Gibbs sampling algorithm. Inference was based on 1000 samples retained from chain lengths of 100,000 samples and burning of 10,000. The following repeatability model was used:
where y is the vector of records for traits 1 and 2; X is the design matrix of fixed effects, b is the vector of fixed effects which included ewe coat-colour as an indicator of variety within the breed (categorical variable with two levels: black or white), litter size (categorical variable with two levels: 1 lamb or 2 lambs and greater), ewe age (categorical variable with four levels: 1, 2, 3, and 4 years and older), milking frequency as a covariate (for records collected at twice- or at once-a-day milking, 1 = once-a-day; 2 = twice-a-day), and stage of lactation also as a covariate (time = days in milk), Z is the design matrix of random animal additive genetic effects (u), W is the design matrix of ewe permanent environment effects (c), and e is the vector of random residual errors. The relationship matrix included 273 individuals, being 23 sires, 113 dams, and 103 founders.
Heritability (h2) and repeatability (t) for traits were calculated using the estimated variance components. The heritability of a trait was calculated as the proportion between the genetic variance and the phenotypic variance. The phenotypic variance σ2p was calculated as the sum of animal genetic variance, permanent environment, and residual (σ2a, σ2c, and σ2e). The repeatability of the trait was calculated as the proportion of permanent variance (the sum of σ2a and σ2c) and the phenotypic variance.
Genetic () and phenotypic (
) correlations are calculated as follows (Falconer and Mackay Citation1996):
where
is the animal (genotypic) covariance between traits 1 and 2;
is the phenotypic covariance between traits 1 and 2;
and
are the additive genetic and phenotypic standard deviations for trait 1, respectively; and
and
are the additive genetic and phenotypic standard deviations for trait 2.
The 95% credibility intervals were computed by setting the 97.5th percentile of the MCMC samples as the upper limit and the 2.5th percentile as the lower limit.
Results
The means, standard deviations, minimum and maximum values for each dependent variable are shown in . The studied flock of dairy sheep produced an average of 660 mL of milk per day per ewe from 35 to 182 days during the 2021–2022 milk production season, which included a transition of milking frequency from twice-a-day to once-a-day. Milk contained an average of 6.12% fat and 5.39% protein.
Table 1. Means, standard deviations (SD), minimum and maximum values for milk production, composition, and milk processability traits for dairy sheep milked in mid and late lactation during the 2021–2022 season.
During the same period, the milk samples of the individual ewes took, on average, 14.46 min to start coagulating after rennet addition, 3.56 min to reach curd firmness of 20 mm after coagulation was initiated, and formed a curd of 49.88 mm of firmness at 30 minutes of analysis. From the total of 639, 36 samples (5.6% of the samples) did not coagulate within 30 minutes of rennet addition, and 43 samples (6.7% of the samples) did not reach curd firmness of 20 mm in the Formagraph. These non-coagulating samples were given penalty values of 30 minutes for RCT, 15 minutes for K20 and 1 mm for A30 for the genetic analyses. Average ILCY produced by the flock was 44.7% and average HCT of was 1.43 minutes.
The estimated variances (additive genetic, permanent environment, and residual), heritabilities, and repeatabilities for each trait are presented in and . Heritability estimates calculated in this study ranged from 0.12 (CSNP:FP) to 0.48 (PP), and repeatability estimates ranged from 0.21 (CSNP:FP) to 0.62 (PP). The 95% confidence intervals were large. The additive genetic variance had a large contribution to the total observed phenotypic variance for some traits, seen by the moderate heritability values obtained for yield traits, PP, LP, and CSNP, which ranged from 0.33 (FY) to 0.48 (PP). Heritability of processability traits were low to moderate and ranged from 0.17 (ILCY) to 0.27 (RCT and pH).
Table 2. Estimates of animal additive genetic (σ2a), ewe permanent environment (σ2c) and residual variances (σ2e), heritability (h2) and repeatability (t), and their corresponding confidence intervals, for milk production and composition of dairy sheep milked in mid and late lactation during the 2021–2022 season.
Table 3. Estimates of animal additive genetic (σ2a), ewe permanent environment (σ2c) and residual variances (σ2e), heritability (h2) and repeatability (t), and their corresponding confidence intervals, for milk processability traits of dairy sheep milked in mid and late lactation.
The genetic and phenotypic correlations between milk production, composition, and processability traits are presented in . Strong absolute correlation values ( > |0.70|) were obtained amongst yield traits, between CSNP and PP, and amongst MCP. Traditional yield traits were weakly correlated with composition traits (−0.11 <
≤ 0.11) and were weak to moderately correlated to MCP (|0.10| <
≤ |0.19|).
Table 4. Genetic correlations (below diagonal) and phenotypic correlations (above diagonal) with confidence interval (in brackets) between milk production, composition, and processability traits, for dairy sheep milked in mid and late lactation.
Moderate correlation values ( > |0.20|) were obtained between RCT and LY, PP, LP, CSNP, CSNP:PP, CSNP:FP, CSNP:Calcium, and SCS. Stronger correlations (
> |0.40|) were obtained between milk pH and processability traits RCT, K20, A30, and HCT. ILCY was strongly correlated to composition traits FP, PP, CSNP, and calcium content (
> |0.40|), and HCT was moderately correlated to MCPs (|0.28| <
≤ |0.40|).
Discussion
The level of milk production for this population of dairy sheep in the studied season has been previously discussed, with lactation curves modelled and total produced yields predicted (Marshall et al. Citation2023). The relatively low milk production in the studied season was suggested to be mainly a consequence of low pasture availability. The average processability characteristics of milk from the individual ewes during the once-a-day milking period have also been discussed in another study, which evaluated the effect of animal factors on processability traits (Marshall et al. Citation2024). Overall, the milk coagulation properties of this flock were within the range reported for dairy sheep, and individual cheese yield was high. However, milk heat stability of individual ewes could not be compared with other flocks due to a lack of studies.
Variances, heritability, and repeatability
A wide range of heritability values for yield traits has been reported for other populations of dairy sheep across the globe (0.06–0.54) (Mavrogenis and Papachristoforou Citation2000; Barillet Citation2007; Puledda et al. Citation2017). Differences in estimates of heritability and repeatability between studies are mainly due to different breeds and population structures but can also be a consequence of sample size. The overall low repeatability values reported here is due to the lower combined additive genetic and permanent environmental sources of variation in proportion to other temporary environmental influences (Dohm Citation2002).
The moderate estimates of heritability for MY, FY, PY, and LY reported here suggest that there could be a significant response through selective breeding on these traits (Lopez-Villalobos Citation2012). The heritability estimates for MY, FY, PY, and LY were higher than the range reported for other populations of East-Friesian sheep (0.09–0.25) (Horstick et al. Citation2002; Hamann et al. Citation2004; De Vries et al. Citation2005).
The heritability values for FP, PP, LP are usually higher and can be twice the value of the heritability values for FY, PY, LY in dairy cows (Lopez-Villalobos Citation2012; Lembeye et al. Citation2016). However, few studies have reported heritability estimates for both yield and milk composition traits in dairy sheep, and the pattern is not consistent (Barillet Citation2007; Sánchez-Mayor et al. Citation2019; Mucha et al. Citation2022). In the present study, the heritability values for PP and LP were higher than but not twice the heritability values for PY and LY. Whereas the heritability for FP (0.19) was lower than the heritability of FY (0.33). This apparent contradiction could be due to FP being highly affected by the variation of feed (Morand-Fehr et al. Citation2007), and, in this study, the nutritional value of the pasture varied significantly throughout the season.
Overall, heritability estimates for processability traits were lower than heritability values for milk yield and composition traits. Low to moderate heritability estimates for MCP have also been previously reported (Sánchez-Mayor et al. Citation2019). Amongst the MCP traits, RCT had the highest heritability (0.27), and K20 had the lowest (0.23), agreeing with the pattern reported by Bittante et al. (Citation2017). The heritability for RCT estimated here also goes in agreement with the findings gathered from earlier studies on dairy cows (Bittante et al. Citation2012)
Lower heritability estimates for milk pH (0.15–0.20) have been reported by Bittante et al. (Citation2017) and Puledda et al. (Citation2017) for Sarda sheep in Italy, but these studies also reported low heritability for milk composition traits. Higher heritability values of 0.34 and 0.46 for milk pH have been reported for Spanish Churra (Pelayo et al. Citation2021) and Assaf sheep (Sánchez-Mayor et al. Citation2019), respectively.
Genetic and phenotypic correlations
Genetic and phenotypic correlations, in general, followed a similar trend. Differences between phenotypic and genetic correlations could be explained by the relationship between genetic and environmental effects, and certain traits have environmental effects that act in the opposite direction to the genetic effects (Sodini et al. Citation2018).
The high correlation values among milk yield traits were expected (Lopez-Villalobos Citation2012; Scholtens et al. Citation2018). In addition, the high correlations amongst MCP found in the present study agree with findings for dairy cows and ewes (Bittante et al. Citation2012; Pelayo et al. Citation2021).
The weak correlation between MY and FP (−0.07) and PP (
−0.11) reported here could be due to the overall low milk production of the flock in the season. Stronger negative correlations between milk yield and composition traits have been widely reported for dairy sheep (Othmane et al. Citation2002, Barillet Citation2007; Sánchez-Mayor et al. Citation2019).
The yield traits MY, FY, PY, and LY were negatively correlated with RCT and K20 (−0.24 ≤ ≤ −0.11), and positively correlated with A30 (0.10 ≤
≤ 0.15). Although the estimated correlations between milk yield traits and MCP were weak to moderate, and the confidence intervals were wide, the trends suggest that the improvement of these dairy sheep on the traditional milk production traits is likely to result in an indirect improvement of the quality of milk for processing into cheese.
It is also worth noting that the negative correlation observed between traditional yield traits and SCS (−0.28 ≤ ≤ −0.20) and pH (−0.22 ≤
≤ −0.18) could imply that worse milk quality was associated with lower producing animals, which consequently worsened milk rennet coagulation properties. However, the negative correlation between MY and ILCY (
−0.23) indicates that improving milk yield could entail a penalty in cheese quantity, which has also been previously noted (Othmane et al. Citation2002). This is further supported by the strong-moderate positive correlations between ILCY and composition traits FP (
0.58) and PP (
0.43).
Although milk yield traits had contrasting correlations with quality (MCP) and quantity (ILCY) traits relevant to cheese production, the lack of correlation between ILCY and MCP makes it challenging to draw conclusions regarding the relationship between cheese quality and quantity. It is possible that the methodology used for estimating ILCY hindered the assessment of true correlations with MCP. In agreement, others have also estimated poor correlations between ILCY and MCP (Sánchez-Mayor et al. Citation2019; Pelayo et al. Citation2021). Therefore, it is recommended that future research use more sophisticated methods for estimating cheese yield (Cipolat-Gotet et al. Citation2016).
The positive moderate genetic correlations between HCT and RCT (0.40), as well as K20 (
0.28), alongside the negative moderate genetic correlation between HCT and A30 (
0.40), suggests that dairy sheep flocks could be specifically oriented towards either milk production for cheese manufacture or to better milk heat stability for processing into milk powder, infant formula, or UHT beverages. However, there needs to be a way for farmers to capture the added value on milk processability (Cole et al. Citation2023).
There were no genetic correlations between FP and MCP. Whereas the positive moderate correlations found between RCT and PP (0.34) and CSNP (
0.23) indicates that a high content of protein and casein were associated with longer time to reach coagulation after rennet addition. In agreement, Ikonen et al. (Citation2004) and Vacca et al. (Citation2019) also reported lower protein content to be correlated to short RCT in dairy cows and in dairy sheep. The higher rennet stability of high-protein milks may be due to partitioning of more calcium (and other divalent ions) within micelles rather than in the serum (Grimley et al. Citation2009). Lower ionic calcium in the serum would decrease salt-bridging effects and thereby slow coagulation. Indeed, the ratio of casein to calcium (CSNP:Calcium), which is opposite to soluble calcium availability, had a positive correlation with RCT (
0.32) and K20 (
0.25).
Lactose percentage had strong moderate negative genetic correlations with RCT (−0.45) and K20 (
−0.33), and positive correlation with A30 (
0.34), agreeing with others (Vacca et al. Citation2019). Lactose percentage might be indirectly associated with MCP because lactose is an osmotic regulator in the mammary gland (Fox et al. Citation2015; Vacca et al. Citation2019). In case of infections in the mammary gland, LP and the content of milk salts in the soluble phase can be affected (Costa et al. Citation2019; Antanaitis et al. Citation2021). The strong moderate negative correlation between LP and SCS (
−0.47) has also been widely reported (Shuster et al. Citation1991; Albenzio et al. Citation2004; Costa et al. Citation2019), supporting the relationship between LP and mammary gland health. In addition, it has also been proposed that lactose can have a direct effect on casein rennet-induced gelation by strengthening the hydrophobic interactions between the casein micelles (Niki and Motoshima Citation2006).
Furthermore, the moderate positive genetic correlation between SCS and RCT (0.30) and K20 (
0.28), and the negative correlation with A30 (
0.23), indicate that elevated SCS levels were linked to prolonged rennet coagulation times and softer curd. Moreover, higher SCS levels correlated with increased milk pH (
0.37), agreeing with other reports (Ikonen et al. Citation2004; Pazzola et al. Citation2018). Milk of high SCS has been reported to have high plasmin level, which leads to degradation of casein, impairing coagulation (Albenzio et al. Citation2004). Consistent with this, SCS had a negative correlation with the casein to protein ratio (
0.22).
Higher milk pH was associated with longer rennet coagulation times and softer curd, seen by the strong moderate positive genetic correlations with RCT (0.57) and K20 (
0.40), and a negative correlation with A30 (
0.50), agreeing with others (Battacone et al. Citation2005; Puledda et al. Citation2017; Pazzola et al. Citation2018). The same trend has been reported for dairy cows (Ikonen et al. Citation2004; Bonfatti et al. Citation2014). Higher milk pH is known to impair the action of the enzyme chymosin and increase the stability of casein micelles (Pirisi et al. Citation1994; Bencini Citation2002).
Despite having obtained some large confidence intervals due to small sample size, the trends identified in the present study suggest that poorer MCP were associated with lower mammary health indicated by higher SCS, higher pH, and lower LP, which is consistent with previous research (Battacone et al. Citation2005; Pazzola et al. Citation2018; Correddu et al. Citation2022). Additionally, a higher ratio of casein to calcium i.e. lower availability of soluble calcium was associated with poorer MCP. Higher yields of milk, fat, protein, and lactose were associated, but to a smaller degree, with better MCP.
Considering the impracticality of measuring processability traits within recording schemes, the lower heritability values, and that the usual payment systems in dairy industries reward producers for high yields of fat and protein, exemplified by the breeding objective of New Zealand dairy cattle that focuses on maximising the efficient conversion of feed into milk solids (Lopez-Villalobos Citation2012), improving dairy sheep flocks, like the one studied, for higher fat and protein yields is proposed, which could potentially lead to an indirect improvement in processability for cheesemaking. Additionally, a penalty on high somatic cell score is recommended to improve sheep milk quality for processing.
However, it is unheard that manufacturers of sheep milk cheeses in New Zealand encounter issues with non-coagulating milk. Conversely, manufacturers of sheep milk powder may be facing challenges with the heat stability of sheep milk (Pan et al. Citation2023), highlighting a need for deeper investigation into dairy sheep genetics for milk heat stability.
From a technological perspective, exploring genetic correlations between milk processability traits and additional traits such as the profile of protein fractions, fatty acids, and soluble minerals could deepen the understanding of the mechanism behind the milk coagulation process, and further validate findings from experimental studies (Loveday et al. Citation2021; Pan et al. 2023). However, the selection of traits for inclusion in a selection index should consider the feasibility of measurement, economic value, and heritability (Shook Citation1989). Alternatively, processability traits can be measured in a training population, enabling genomic predictions that indicate milk processing quality (Jones and Wilson Citation2022).
Ultimately, establishing selection indexes for dairy sheep is important as it optimises milk production from fewer ewes, improving farmer profitability, whilst significantly reducing the use of natural resources, indirectly contributing to farm sustainability. Research is also needed on the genetic correlations between milk production and sustainability and resilience traits in dairy sheep in New Zealand (Barillet Citation2007; Mucha et al. Citation2022).
It is crucial to preserve the knowledge gained from small farms like the one in this study, as pedigree information for dairy sheep is often difficult to maintain or access. Small farms also contribute to overall genetic diversity and resilience within the dairy sheep farming community in New Zealand and should not be overlooked. Further genome-wide association studies are underway and will allow further understanding of the genetic architecture of milk processability traits. Additionally, genomic selection could increase accuracy and facilitate dairy sheep improvement on traits that are not easily measured.
Conclusion
The results of the present study suggest that genetic improvement of this flock of dairy sheep on the traditional traits of fat yield, protein yield, and lower somatic cell count could also lead to an indirect improvement of milk quality for cheese-making. However, selecting for improved cheese-making aptitude might undesirably worsen milk heat stability, potentially evidencing the need for a separate selection index for dairy sheep flocks destined to milk powder production, which is important for export revenue. It is recommended that larger studies involving more dairy sheep flocks are conducted to validate the present results before developing a selection index for the dairy sheep industry.
Acknowledgements
The authors would like to thank the commercial farm, and the scientific communities from the NZ3M group, Massey University, and AgResearch.
Disclosure statement
No potential conflict of interest was reported by the author(s).
Correction Statement
This article has been corrected with minor changes. These changes do not impact the academic content of the article.
Additional information
Funding
References
- Albenzio M, Caroprese M, Santillo A, Marino R, Taibi L, Sevi A. 2004. Effects of somatic cell count and stage of lactation on the plasmin activity and cheese-making properties of ewe milk. Journal of Dairy Science. 87:533–542. doi:10.3168/jds.S0022-0302(04)73194-X.
- Antanaitis R, Juozaitienė V, Jonike V, Baumgartner W, Paulauskas A. 2021. Milk lactose as a biomarker of subclinical mastitis in dairy cows. Animals. 11(6):1736. doi:10.3390/ani11061736.
- Barillet F. 2007. Genetic improvement for dairy production in sheep and goats. Small Ruminant Research. 70:60–75. doi:10.1016/j.smallrumres.2007.01.004.
- Battacone G, Cannas EA, Mazzette A, Dimauro C, Enne G. 2005. Why does the increase of plasmin worsen the coagulation properties of milk in dairy sheep. Italian Journal of Animal Science. 4:342–344.
- Bencini R. 2002. Factors affecting the clotting properties of sheep milk. Journal of the Science of Food and Agriculture. 82:705–719. doi:10.1002/jsfa.1101.
- Bencini R, Pulina G. 1997. The quality of sheep milk: a review. Australian Journal of Experimental Agriculture. 37:485–504. doi:10.1071/EA96014.
- Bittante G, Cipolat-Gotet C, Pazzola M, Dettori M, Vacca G, Cecchinato A. 2017. Genetic analysis of coagulation properties, curd firming modeling, milk yield, composition, and acidity in Sarda dairy sheep. Journal of Dairy Science. 100:385–394. doi:10.3168/jds.2016-11212.
- Bittante G, Penasa M, Cecchinato A. 2012. Invited review: genetics and modeling of milk coagulation properties. Journal of Dairy Science. 95(12):6843–6870. doi:10.3168/jds.2012-5507.
- Bonfatti V, Tuzzato M, Chiarot G, Carnier P. 2014. Variation in milk coagulation properties does not affect cheese yield and composition of model cheese. International Dairy Journal. 39:139–145.
- Cheng H, Fernando RL, Garrick DJ. 2018. JWAS: Julia implementation of whole-genome analysis software. Proceedings of the World Congress on Genetics Applied to Livestock Production 11:859. Auckland, New Zealand.
- Cipolat-Gotet C, Cecchinato A, Stocco G, Bittante G. 2016. The 9-MilCA method as a rapid, partly automated protocol for simultaneously recording milk coagulation, curd firming, syneresis, cheese yield, and curd nutrients recovery or whey loss. Journal of Dairy Science. 99(2):1065–1082. doi:10.3168/jds.2015-9734.
- Cole JB, Makanjuola BO, Rochus CM, Van Staaveren N, Baes C. 2023. The effects of breeding and selection on lactation in dairy cattle. Animal Frontiers. 13:62–70.
- Cole WC, Tarassuk NP. 1946. Heat Coagulation of Milk. Journal of Dairy Science. 29(7):421–429. doi:10.3168/jds.S0022-0302(46)92498-8.
- Correddu F, Gaspa G, Cesarani A, Macciotta NPP. 2022. Phenotypic and genetic characterization of the occurrence of noncoagulating milk in dairy sheep. Journal of Dairy Science. 105(8):6773–6782. doi:10.3168/jds.2021-21661.
- Costa A, Lopez-Villalobos N, Visentin G, De Marchi M, Cassandro M, Penasa M. 2019. Heritability and repeatability of milk lactose and its relationships with traditional milk traits, somatic cell score and freezing point in Holstein cows. Animal. 13:909–916. doi:10.1017/S1751731118002094.
- De Vries F, Hamann H, Drögemüller C, Ganter M, Distl O. 2005. Analysis of associations between the prion protein genotypes and production traits in East Friesian milk sheep. Journal of Dairy Science. 88:392–398. doi:10.3168/jds.S0022-0302(05)72699-0.
- Dohm MR. 2002. Repeatability estimates do not always set an upper limit to heritability. Functional Ecology. 16:273–280. doi:10.1046/j.1365-2435.2002.00621.x.
- Downie-Melrose K. 2014. A Bio-economic feasibility study of sheep dairy systems in Canterbury. Lincoln: Lincoln University.
- Falconer DS, Makay TFC. 1996. Introduction to Quantitative Genetics. Essex: Longman Scientific and Technical.
- Flint APF, Woolliams JA. 2008. Precision animal breeding. Philosophical Transactions of the Royal Society B: Biological Sciences. 363:573–590. doi:10.1098/rstb.2007.2171.
- Fox PF, Uniacke-Lowe T, McSweeney PLH and O’Mahoni JA. 2015. Dairy chemistry and biochemistry. Springer International Publishing, Cham.
- Grimley H, Grandison A, Lewis M. 2009. Changes in milk composition and processing properties during the spring flush period. Dairy Science and Technology. 89(3-4):405–416. doi:10.1051/dst/2009016.
- Gullery L. 2021. Dairy’s other export not sheepish about growth. https://www.stuff.co.nz/business/farming/dairy/126515904/dairys-other-export-not-sheepish-about-growth (accessed on 23 May 2024).
- Hamann H, Horstick A, Wessels A, Distl O. 2004. Estimation of genetic parameters for test day milk production, somatic cell score and litter size at birth in East Friesian ewes. Livestock Production Science. 87:153–160. doi:10.1016/j.livprodsci.2003.09.015.
- Horstick A, Hamann H, Distl O. 2002.. Estimation of genetic parameters for daily milk performance of East Friesian milk sheep by random regression models. Proceedings of the 7th World Congress on Genetics Applied to Livestock Production, Montpellier, France, August, 2002. Session 1. Pp. 1-4.
- Ikonen T, Morri S, Tyrisevä A-M, Ruottinen O, Ojala M. 2004. Genetic and phenotypic correlations between milk coagulation properties, milk production traits, somatic cell count, casein content, and pH of milk. Journal of Dairy Science. 87:458–467. doi:10.3168/jds.S0022-0302(04)73185-9.
- Jones HE, Wilson PB. 2022. Progress and opportunities through use of genomics in animal production. Trends in Genetics. 38:1228–1252. doi:10.1016/j.tig.2022.06.014.
- Lees N, Lees I. 2018. Competitive advantage through responsible innovation in the New Zealand sheep dairy industry. International Food and Agribusiness Management Review. 21:505–524. doi:10.22434/IFAMR2017.0013.
- Lembeye F, López-Villalobos N, Burke J, Davis S. 2016. Estimation of genetic parameters for milk traits in cows milked once-or twice-daily in New Zealand. Livestock Science. 185:142–147. doi:10.1016/j.livsci.2016.01.022.
- Lopez-Villalobos N. 2012. Analysing the genetic basis of milk production traits. Animal Science Reviews. 2012:65–78.
- Loveday SM, Weeks M, Luo D, Cakebread J. 2021. Type A and B bovine milks: Heat stability is driven by different physicochemical parameters. Journal of Dairy Science. 104:11413–11421.
- Marshall AC, Lopez-Villalobos N, Loveday SM, Ellis A, McNabb W. 2023. Modelling lactation curves for dairy sheep in a New Zealand flock. Animals. 13:349. doi:10.3390/ani13030349.
- Marshall AC, Lopez-Villalobos N, Loveday SM, Weeks M, McNabb W. 2024. Animal factors affecting the cheese-making properties and the heat coagulation time of milk from dairy sheep in a New Zealand flock. New Zealand Journal of Agricultural Research. 1–18.
- Mavrogenis A, Papachristoforou C. 2000. Genetic and phenotypic relationships between milk production and body weight in Chios sheep and Damascus goats. Livestock Production Science. 67:81–87. doi:10.1016/S0301-6226(00)00187-1.
- McCoard S, Stevens D, Selbie D, Day L, Young W, Bekhit AED, Samuelsson L. 2023. Supporting the growth of the dairy sheep industry in New Zealand–industry update and review of a programme linking industry and science. New Zealand Journal of Agricultural Research, 1–35. doi:10.1080/00288233.2023.2272594.
- McMahon DJ, Brown RJ. 1982. Evaluation of Formagraph for comparing rennet solutions. Journal of Dairy Science. 65:1639–1642. doi:10.3168/jds.S0022-0302(82)82390-4.
- Meteorological Service of New Zealand Ltd. 2022. Available online: https://www.metservice.com/towns-cities/locations/masterton/past-weather (accessed on 5 September 2022).
- Morand-Fehr P, Fedele V, Decandia M, Le Frileux Y. 2007. Influence of farming and feeding systems on composition and quality of goat and sheep milk. Small Ruminant Research. 68:20–34. doi:10.1016/j.smallrumres.2006.09.019.
- MPI-Ministry of Primary Industries, & Massey University. 2020. New Zealand Sheep dairy survey. https://www.mpi.govt.nz/dmsdocument/43183-Sheep-Dairy-Survey-Report-July-2020 (accessed on 04 May 2024).
- Mucha S, Tortereau F, Doeschl-Wilson A, Rupp R, Conington J. 2022. Animal board invited review: meta-analysis of genetic parameters for resilience and efficiency traits in goats and sheep. Animal. 16:100456. doi:10.1016/j.animal.2022.100456.
- New Zealand Sheepbreeders Association. 2024a. Dairymeade breed information. https://nzsheep.co.nz/dairymeade-nz/ (accessed on 23 May 2024).
- New Zealand Sheepbreeders Association. 2024b. Southern cross breed information. https://nzsheep.co.nz/southern-cross/ (accessed on 23 May 2024).
- New Zealand Sheepbreeders Association. 2024c. Zealandia breed information. https://nzsheep.co.nz/zealandia/ (accessed on 23 May 2024).
- Niki R, Motoshima H. 2006. Influence of lactose on viscoelastic properties of acid-induced casein micelle gels: the secondary roll of lactose in yoghurt production. Milchwissenschaft. 61:411–415.
- Othmane MH, Carriedo JA, de la Fuente Crespo LF, San Primitivo F. 2002. An individual laboratory cheese-making method for selection in dairy ewes. Small Ruminant Research. 45(1):67–73. doi:10.1016/S0921-4488(02)00079-2.
- Othmane MH, De La Fuente LF, Carriedo JA, San Primitivo F. 2002. Heritability and genetic correlations of test day milk yield and composition, individual laboratory cheese yield, and somatic cell count for dairy ewes. Journal of Dairy Science. 85:2692–2698.
- Pan Z, Ye A, Dave A, Fraser K, Singh H. 2023. pH-dependent sedimentation and protein interactions in ultra-high-temperature-treated sheep skim milk. Journal of Dairy Science. 106:1626–1637.
- Pazzola M, Cipolat-Gotet C, Bittante G, Cecchinato A, Dettori ML, Vacca GM. 2018. Phenotypic and genetic relationships between indicators of the mammary gland health status and milk composition, coagulation, and curd firming in dairy sheep. Journal of Dairy Science. 101:3164–3175. doi:10.3168/jds.2017-13975.
- Pazzola M, Dettori ML, Cipolat-Gotet C, Cecchinato A, Bittante G, Vacca GM. 2014. Phenotypic factors affecting coagulation properties of milk from Sarda ewes. Journal of Dairy Science. 97(11):7247–7257. doi:10.3168/jds.2014-8138.
- Pelayo R, Gutiérrez-Gil B, Garzón A, Esteban-Blanco C, Marina H, Arranz J. 2021. Estimation of genetic parameters for cheese-making traits in Spanish Churra sheep. Journal of Dairy Science. 104:3250–3260. doi:10.3168/jds.2020-19387.
- Peterson S, Prichard C. 2015. The sheep dairy industry in New Zealand: A review. New Zealand Society of Animal Production. Pp. 119-126.
- Pirisi A, Murgia A, Scintu MF. 1994. Estimate of Pecorino romano and Pecorino sardo cheese yield from the protein and fat contents in sheep milk [Latium, Sardinia]. Scienza e Tecnica Lattiero-Casearia (Italy). 45:476–483.
- Puledda A, Gaspa G, Manca MG, Serdino J, Urgeghe PP, Dimauro C, Negrini R, Macciotta NPP. 2017. Estimates of heritability and genetic correlations for milk coagulation properties and individual laboratory cheese yield in Sarda ewes. Animal. 11:920–928. doi:10.1017/S1751731116002147.
- Sánchez-Mayor M, Pong-Wong R, Gutiérrez-Gil B, Garzón A, de la Fuente L, Arranz J. 2019. Phenotypic and genetic parameter estimates of cheese-making traits and their relationships with milk production, composition and functional traits in Spanish Assaf sheep. Livestock Science. 228:76–83. doi:10.1016/j.livsci.2019.08.004.
- SAS. 2004. Statistical Analysis System, Version 9.4; SAS Institute Inc.: Cary, NC, USA.
- Scale up. 2022. Government supports innovative dairy sheep sector to scale up. https://www.beehive.govt.nz/release/government-supports-innovative-dairy-sheep-sector-scale (accessed on 23 May 2024).
- Scholtens MR, Peterson S, Gunson K, Gunson A, Lopez-Villalobos N. 2018. Genetic evaluation of dairy sheep in a New Zealand flock. New Zealand Journal of Agricultural Research. 61:81–91. doi:10.1080/00288233.2017.1393757.
- Shook GE. 1989. Selection for disease resistance. Journal of Dairy Science. 72:1349–1362. doi:10.3168/jds.S0022-0302(89)79242-0.
- Shuster D, Harmon R, Jackson J, Hemken R. 1991. Suppression of milk production during endotoxin-induced mastitis. Journal of Dairy Science. 74:3763–3774. doi:10.3168/jds.S0022-0302(91)78568-8.
- Sodini SM, Kemper KE, Wray NR, Trzaskowski M. 2018. Comparison of genotypic and phenotypic correlations: Cheverud’s conjecture in humans. Genetics. 209:941–948. doi:10.1534/genetics.117.300630.
- Vacca G, Cipolat-Gotet C, Paschino P, Casu S, Usai M, Bittante G, Pazzola M. 2019. Variation of milk technological properties in sheep milk: Relationships among composition, coagulation and cheese-making traits. International Dairy Journal. 97:5–14. doi:10.1016/j.idairyj.2019.05.002.