Abstract
Storm-induced Escherichia coli pulses in the Motueka River (2074 km2) and the Sherry River (78.4 km2) are modelled. The model focuses on the catchment outlets, representing key processes, including E. coli transfer to and from the river bed, with account taken of the hysteresis in, and non-linear, non-stationary, response of E. coli concentrations to river stormflows. The model fits the Motueka River observations well, but less well in the Sherry River. A greatly simplified description of headwater and riparian inputs is satisfactory at the larger catchment scale where near-field, in-channel processes dominate the response. Spatial heterogeneity in rainfall-run-off and faecal sources probably contribute to the poorer fit in the smaller catchment. Despite using a relatively small number of driving variables and parameters, the model has the potential to predict real-time E. coli input to Tasman Bay in river plumes causing shellfish and bathing beach contamination.
Introduction
Diffuse pollution from land mobilises three main categories of water pollutant: fine sediment that reduces water clarity and causes sedimentation issues, nutrients (primarily nitrogen and phosphorus) that promote nuisance algae and aquatic plant growths, and faecal microbes that are a risk to human health from contact with contaminated water or consumption of contaminated shellfish (Kay et al. Citation2006). Furthermore, mining and urban land uses may cause diffuse pollution by metals and other toxic materials (e.g. Campbell et al. Citation2004). Considerable research effort, including modelling, has been applied to understanding and quantifying various diffuse pollutants, but faecal microbes are probably least well studied and understood. This is despite the inference (e.g. Kay et al. Citation2007) that a greater overall stream length may be impacted by faecal microbial pollution than by any other pollutant category, including sediment and nutrients.
An important task early in the Motueka Integrated Catchment Management (ICM) programme was the characterisation of water quality over the catchment (Fenemor et al. Citation2011). Young et al. (Citation2005) reported water quality for the Motueka system based on 13 monthly samplings (October 2000–October 2001) of 21 sites at mainly baseflow conditions, augmented by monthly monitoring data since January 1989 at two sites in the National Rivers Water Quality Network (NRWQN). This study showed that water quality of the Motueka system was mostly very good; however, the Sherry River, draining about 3.8% of the total catchment, emerged as a microbially polluted ‘hot spot’, with E. coli concentrations well in excess of guidelines for contact recreation. The faecal pollution in the Sherry River was attributed to intensive pastoral farming, and specifically to regular crossings through the river by dairy herds moving between pasture a nd milking shed. A study in 2001 of the most up-stream dairy crossing on the Sherry confirmed that direct contact of cows with water during crossings was significantly polluting the river (Davies-Colley et al. Citation2004). Since 2007, all four habitual crossings on the Sherry River have been eliminated by construction of bridges, and faecal pollution is now substantially reduced although the river still fails to meet contact recreational guidelines (Ballantine et al. Citation2011).
Although the water quality of the Motueka Catchment is excellent overall, excepting the Sherry and some other pastoral subcatchments (Young et al. Citation2005), the microbial quality can be poor during high flows. McKergow & Davies-Colley (Citation2010) found that E. coli concentrations in the main-stem Motueka River during storm flows were an order of magnitude, or more, higher than at baseflow, and often exceeded contact recreation guidelines. This finding is broadly consistent with earlier reports (Kay & McDonald Citation1980; Jenkins et al. Citation1984) including from dairy land elsewhere in New Zealand (Davies-Colley et al. Citation2008). An ‘obvious’ ramification of these stormflow ‘pulses’ is that microbially contaminated plumes from the Motueka River represent a major hazard to bivalve shellfish and bathing beaches in Tasman Bay. Accordingly, there has been interest in measuring E. coli dynamics in the lower Motueka River and in the river plume in Tasman Bay (Cornelisen et al. Citation2011).
In this paper, the faecal indicator bacteria (E. coli) is simulated in the main stem of the Motueka River and its small tributary the Sherry River, using a model based on that of Wilkinson (2001, 2008) that is broadly similar to that of Hipsey et al. (Citation2008).
A mechanistic model is required to adequately reproduce observed time-series of E. coli storm response pulses, because the relationship between faecal indicator concentrations and flow are non-linear, non-stationary (i.e. they vary from event to event) and exhibit hysteresis (the relationship is different on the hydrograph rise and fall) to varying degrees. Hence, a simple statistical relationship cannot represent this complexity. Mechanistic modelling builds a mathematical structure that represents the dominant processes and mechanisms and can simulate the observed dynamic behaviour in a repeatable manner with a single parameter set. Statistical models do not have ‘state’ variables, and hence cannot track and retain a memory of the system condition, i.e. in this case they cannot follow the accumulation and remobilisation of faecal bacteria in the channel store or on the catchment. A mechanistic model can account for these processes and mechanisms in order to simulate the observed E. coli variations reliably over time (e.g. Wilkinson et al. Citation1995; Wilkinson Citation2001; Collins & Rutherford Citation2004).
It is well known that catchment faecal microbial dynamics are strongly influenced by mobilisation from land and channel stores during storm flows (e.g. McDonald et al. Citation1982; Hunter et al. Citation1992). Although differences in the storm-event transport behaviour of different microorganisms once exposed to environmental pressures have been highlighted (e.g. Jamieson et al. Citation2004; Stott et al. Citation2011), Hipsey et al. (Citation2008) reaffirm that the general processes which influence the fate and distribution of most of these microbes are similar to that presented by Wilkinson (2001, 2008), and in the current study, with the exception that Hipsey et al. (Citation2008), included a separate treatment of sediment-bound and free microorganism transfer to and from the channel store. Other modelling studies have focussed strongly on quantifying faecal delivery from upland pastures, detailing different sources, livestock behaviours and pathways to the stream network (Collins & Rutherford Citation2004). In-stream processes, such as settlement and remobilisation of faecal bacteria are important in riverine fluvial microbial dynamics.
Wilkinson et al. (Citation1995) presented a model that used a distributed channel store to simulate multiple successive faecal coliform peaks in response to an artificial stepped hydrograph event, but later a simpler formulation was found to reproduce the observed pulses adequately (Wilkinson Citation2001). This later re-evaluation identified the role of flow-wave propagation in determining the timing and magnitude of the entrained faecal coliform pulses relative to the average velocity of the moving water (Wilkinson Citation2001; Wilkinson et al. Citation2006). Small faecal pulses coincided with wave-front arrival, and the main peaks arrived later at the mean flow velocity. The flow-waves travelled approximately 1.5 times faster than the mean velocity of the water, and the magnitude of the main pulses was proportional to that of the flow increment in m3/s (Wilkinson Citation2001). This differential transport and arrival has been observed and modelled for faecal indicators and zoonotic disease agents in New Zealand (McBride Citation2011) and is significant for the understanding of the fluvial dynamics of faecal contaminants. As stated by Hipsey et al (Citation2008) a generic model architecture that can be applied in many circumstances and to different contaminant microorganisms is attractive and the model of Wilkinson (2001, 2008) as presented here offers such potential.
The modelling employed data from two separate water quality monitoring campaigns (at very different spatial scales). These included regular storm-flow event sampling with stage-triggered autosamplers, and (pseudo-random) monthly sampling. The goals of the modelling were to improve understanding of faecal pollution sources and dynamics in the Motueka system, and provide a basis for flow-on prediction of contaminated river plumes in Tasman Bay with consequent threats to shellfish and bathing beaches.
Methods
Site description and data
This study was based on monitoring conducted in the Motueka River catchment in the upper South Island, New Zealand (). The Motueka River drains a catchment of 2180 km2 (2047 km2 upstream of the Woodmans Bend monitoring station, closest to the sea) and flows in a northerly direction for 110 km from the headwaters to Tasman Bay. Mean annual rainfall ranges from <1000 mm/year on the eastern side of the catchment to 3500 mm/year on the western side (Basher Citation2003). Median discharge near the river mouth is about 47 m3/s with a mean annual low flow of around 13 m3/s. Land use is varied and includes native forest in the southern and western headwaters, plantation forest across much of the eastern and central part of the catchment and pastoral farming and horticulture along the valley floors (). The catchment is sparsely populated with a total population of around 12,000, including about 7000 in the town of Motueka near the river mouth (Basher Citation2003).
Figure 1 Map representation of A, the location; B, the river network and main sub-catchments with two major Escherichia coli sampling sites shown at the locations of hydrometric stations; C, land cover; and D, geology of the Motueka catchment.
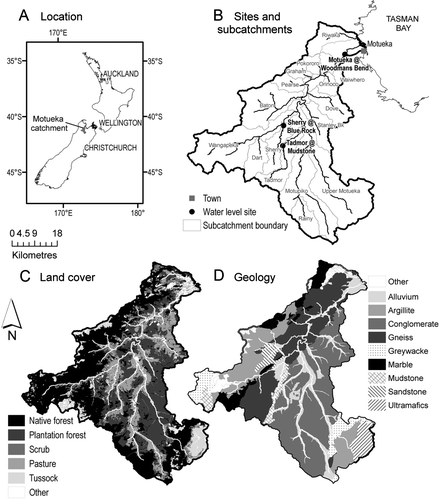
The Sherry River drains a small western subcatchment (79 km2) of the Motueka River where mean annual rainfall is approximately 1300 mm/year (b). The name ‘Sherry’ refers to the orange colour of the river water reflecting the rather high coloured dissolved organic matter (CDOM; indexed by the absorption coefficient at 440 nm, which averages about 2.8/m—high even for inland waters; Kirk Citation1994). Median discharge for the lower reaches at the Blue Rock recorder site is about 1.1 m3/s, whereas mean annual low flow is about 0.22 m3/s. The upper reaches of the Sherry Catchment are dominated by plantation forest with some residual native beech, whereas pastoral farming (dai rying and some sheep-beef farming) is the main land use in the lower valley. Water sampling in the lower Sherry River has demonstrated comparatively high faecal pollution (Davies-Colley et al. Citation2004; Young et al. Citation2005).
Water quality and flow measurements
Motueka River
Water quality samples from the Motueka River were collected at Woodmans Bend (172.96° E, 41.13° S), 8 km upstream of the river mouth and above any tidal influence (b), as reported in McKergow & Davies-Colley (Citation2010). Water levels are measured at Woodmans Bend every 15 min by a bubble gauge (Sutron Accubar; 0–15-m range) and converted to discharge via a stage-discharge rating. Field turbidity was measured every 15 min by an optical backscatter sensor (Greenspan TS100; 0–2000 NTU) fitted with a pump jetting water across the lens to reduce biological fouling. The turbidity records were edited to remove short-term (usually single-value) spikes (e.g. because of items like leaves in the volume sensed by the backscatter detector) and drift related to biofouling. Monthly grab samples for E. coli were collected from May 2003 to October 2009. Escherichia coli were also measured on samples collected during storm events by an autosampler (ISCO 3700) over a 13-month period from June 2003. Sampling was activated when water levels exceeded a seasonally adjusted stage threshold and samples were collected on flow proportional time intervals ranging from 10 min to 22 h. Samples were retrieved usually within 24 h of collection and stored on ice during transport to the NIWA Hamilton water quality laboratory where they were analysed for E. coli and turbidity. Escherichia coli were enumerated mostly within 48 h of sample collection using the IDEXX Colilert Most Probable Number (MPN) method (method 9223B, APHA 2005), and results are expressed as culture forming units (cfu) per 100 ml.
Sherry River
Water quality samples were collected in the Sherry River at the Blue Rock flow gauging station (b), from November 2008 until October 2009. Up until 2008, no flow estimates were available for the Sherry. From 2008 onwards, flow was measured at Blue Rock using a pressure transducer (0–5 m range) and a Waterlog DAA H500XL data logger (DAA HX500 model). For 2003 and 2004, estimates of flow in the Sherry River were based-on a correlation with flow in the adjacent Tadmor catchment (b). A continuous field turbidity meter (Greenspan TS1200, 0–2000 NTU) was deployed at Blue Rock from October 2008 to October 2009, and data was logged every 15 min by the hydrometric logger. Escherichia coli was sampled at approximately monthly intervals from May 2003 to October 2009, mainly at low flow. In October 2008, a year-long storm event sampling campaign, similar to that at Woodmans Bend, commenced at Blue Rock. The autosampler was activated when stage exceeded 800 mm (c. 3800 l/s, 8.5 percentile flow) and samples were collected hourly until flows dropped below the trigger or until the sampler carousel was full with 24 samples. Monthly samples were stored on ice and transported by overnight courier to NIWA's water quality laboratory in Hamilton, whereas event samples were retrieved within 24 h and analysed by Cawthron Institute's water quality laboratory in Nelson. Monthly samples and a selection of storm water samples (3–8 samples per event; chosen to bracket the turbidograph) were analysed for E. coli and other variables including turbidity (Ballantine et al. Citation2011). Escherichia coli were enumerated as for Woodmans Bend.
Autosampler quality assurance
Common concerns when using autosamplers for microbiological surveys are sample holding time (e.g. Pope et al. Citation2003; Stadler et al. Citation2008) and cross-contamination through carry-over from residues in the sampler pipework and mechanisms (e.g. Anderson & Rounds Citation2010; Solo-Gabriele et al. Citation2000). A holding time of 6 h is specified in investigations with a regulatory focus (e.g. Environment Agency Citation2000), for which samples should be held in the dark and chilled, to minimise deterioration from the ‘as sampled’ condition. For the current study, we had to relax these requirements to permit use of auto-samplers (without carousel chilling), which were triggered automatically by events. Pope et al. (Citation2003) found that E. coli concentrations in samples held at less than 10°C were generally consistent with those measured within 8 h. Similarly, Stadler et al. (Citation2008) reported only minor sample deterioration for holding temperatures of around 5°C. So the main issue is the holding time within the instrument shack, after collection, but before auto-samples were retrieved and stored on ice. In the current study there would be negligible sample deterioration with extended holding times in autosampler carousels at winter (air) temperatures of around 6°C, but some deterioration could occur in summer with average air temperatures of around 16°C. Estimates of summer die-off, based-on a range of literature dark die-off parameters, θ and k 0 (Wilkinson Citation2008), suggest that in the worst case for fully 48 h holding before sample retrieval and chilling, up to 48% reduction in E. coli concentration might occur. Three summer events discussed below may have been subject to E. coli underestimation: 9 December 2008 and 12 February 2009 in the Sherry ( below), and 27 February 2004 at Woodmans Bend (Fig. 5 below), although there is no visual suggestion of low-bias vs model predictions.
The other concern with auto-samplers is carry-over of water from previous samples, especially with contrasting microbial contamination. Solo-Gabriele et al. (Citation2000) found auto sampler carry-over of E. coli to be insignificant, and Stadler et al. (Citation2008) demonstrated a close correlation with duplicate grab samples, with no evidence of bias from sample carry-over, so we consider this potential source of error can be discounted in our study.
Model description
Wilkinson et al. (Citation1995) presented a model for the remobilisation of river channel store E. coli by artificially generated flood-pulses. This built on work by Kay et al. (Citation1980) and Jenkins et al. (Citation1984). The 1995 model was simplified and extended (Wilkinson Citation2001) to account for ‘real-time’ die-off (as a function of solar irradiance water temperature, turbidity and mean river depth), and diffuse inputs, and was successfully applied to 7 years of 4-weekly sampling data for a drinking water abstraction site on the Welsh River Dee in the UK (Wilkinson Citation2001).
The model at the sub-catchment scale has three layers ( and ):
1. | riparian pasture faecal inputs to the river network; | ||||
2. | river reach flowing water ‘mass balance’, and; | ||||
3. | river reach channel store mass balance. |
Figure 2 Conceptual diagram representing the key Escherichia coli model processes in a river reach with adjacent riparian pasture.
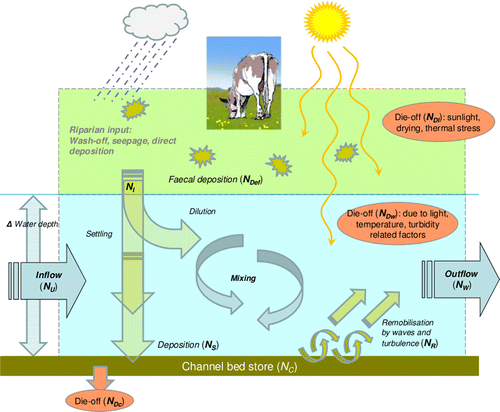
The structure of the system can be presented in a ‘conceptual diagram’ (), representing the general system, including terms from the model Equations (Equation4) and (Equation5) (below). A more mathematical ‘systems’ type diagram may also be useful (). These are just two approaches that can be used to show the arrangement, connection and fluxes into, out of and storage within different compartments of the system. Pictorial diagrams with an overview narrative are becoming increasingly recognised as a valuable tool for the collection and visualisation of information about complex environmental systems, especially for the rapid communication of ideas to varied audiences (e.g. Dennison et al. Citation2007).
Faecal indicator dynamics in rivers are determined by two contrasting sets of processes associated with dry vs wet weather respectively (). During dry weather, transport of faecal matter is minimal; water tends to be shallow, is minimally light-attenuating and water travel velocities are slow, such that die-off dominates the time-variation in faecal indicator numbers. Following rainfall, river flows are elevated and the water is both deep and highly light-attenuating, there is usually cloud cover and travel times are short, and hence die-off related to sunlight is virtually switched off. Consequently, transport mechanisms dominate the dynamic variations in faecal indicators during stormflows.
Figure 4 Schematic representing the transition from wet to dry weather processes associated with faecal indicator dynamics in river catchments.
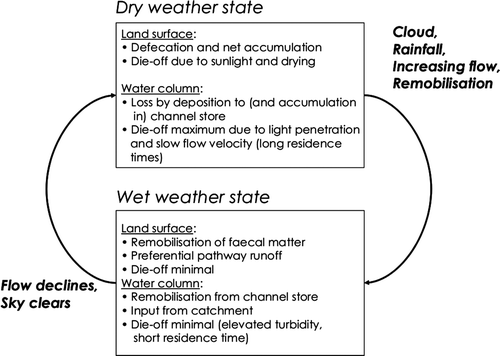
As a narrative description of the role of the three layers in the model, the riparian faecal input layer produces a time-series of input E. coli numbers that varies according to the rate of die-off. It is greater in winter than in summer, and for each sub-catchment, the rate of input is scaled by the area of riparian pasture and the run-off yield, such that wetter sub-catchments deliver more faecal contamination than dryer ones. The riparian input is delivered directly to the river reach, without catchment storage, and then a proportion remains in suspension, whereas the remainder settles and is deposited in the channel store (according to the flow state—during dry-weather flow, the majority of riparian input E. coli settle, whereas during storm flow the majority remain in suspension). This approach for catchment delivery is greatly simplified, and is justified in detail in the discussion. Each river reach has two layers: the flowing water and the channel store. The flowing water provides the mixing and transport environment for E. coli entering from upstream, from riparian input, transfer to and from channel-storage, and loss related to die-off. The dominant dynamic behaviour determining the modelled E. coli concentrations is the remobilisation of E. coli accumulated in the channel store. This is driven by the change in river discharge (flow). The channel store of each river reach provides the ‘reservoir’ of E. coli that accumulates during dry weather and is remobilised by each storm-flow event resulting in the observed peaks of concentration. The model equations are as follows.
Riparian Pasture Input
The faecal loading for each sub-catchment or reach,
River reach ‘mass-balance’
The river reach layers, the flowing water, and the channel store are linked ( and ), and their dominant activity is mainly flow dependent as indicated in Fig. 4. The basic mass-balance equation for the flowing river water is:
Table 1 Summary of model variables and parameters and their units.
The detail of the river reach model components is rather more complicated. The flowing-water layer is based upon a river dispersion (mixing) model (e.g. Wallis et al. Citation1989; Price et al. Citation2000). For a simple one-dimensional model such as that presented here, an important simplifying assumption is that the water is completely mixed across the channel cross-section (Henderson-Sellers et al. Citation1988). In its simplest version (quasi-steady-flow for a conservative solute), this mixing model is formulated as follows:
The full mass-balance equation for the number of E. coli in the flowing water in each river reach (i.e. not including the channel store) is:
Change in water numbers = upstream input−(dispersion + settlement + die-off)×number in reach + number remobilised from channel store + riparian pasture input.
which is given by:
Deposition is used here to refer to the accumulation of microorganisms into the channel store, deposition is only said to have occurred when a particle reaches the river bed and actually stops moving (Graham et al. Citation1990). Deposition is a function of water depth, and the greater the water depth, the lower the probability of a particle being deposited. As Jenkins (Citation1984) demonstrated, remobilisation and deposition are related to bed-substrate factors and by inference to channel habitat patchiness. The hyporheic zone is a key part of the channel store in cobble bed rivers (e.g. Muirhead et al. Citation2004), where microorganisms will be deposited from the water as it is exchanged through the channel substrate. Muirhead et al. (Citation2004) demonstrated that the disturbance of river channel cobbles and gravel by artificial floods resulted in similar remobilisation of faecal indicator microorganisms into the water column compared with natural flood events.
The channel store is essential to the functioning of the model and represents the aggregate of processes associated with the accumulation of bacteria deposited from the water column and later remobilised by storm flow (Wilkinson et al. Citation2006). Jamieson et al. (Citation2004) showed that critical shear stress for entrainment of cohesive sediments and associated faecal bacteria is directly proportional to flow, but their study did not assess channels of coarser bed material where hyporheic exchange is an important mechanism. Wilkinson (Citation2001) experimented with the use of discharge, flow velocity, water depth and wetted perimeter as analogues for channel remobilisation forcings, but found that discharge produced the best model simulations. Wilkinson (Citation2001) also had additional entrainment terms to account for steep-fronted waves associated with reservoir releases, as well as, for turbulent burst-sweep action, which may maintain erosion at elevated but slowly varying flows (Garcia et al. Citation1996). In the current study with natural gradually varying rainfall-run-off generated flow waves, the model could be simplified to the ‘wave-front disturbance’, ΔQ/Q ave, term only. This was adequate to simulate the remobilisation of faecal bacteria successfully from the channel store. In the formulation applied here, the model effectively lumps together the channel and catchment stores of faecal bacteria. The assumption being that the two stores are supply limited systems (e.g. VanSickle & Beschta Citation1983; Jeje et al. Citation1991; Hunter et al. Citation1992; Wilkinson et al. Citation1995) and act in a similar manner. Both accumulate faecal bacteria during dry weather and these are flushed during storm-events resulting in high concentration pulses. At the larger catchment scale, the integrating effect of the catchment is assumed to be sufficient that localised variations in input are not noticed at the outlet of the system. This may be a function of the size of the defecating units themselves, i.e. the cattle and sheep, relative to the catchment. At larger scales, the movement of the animals from different grazing lands only results in localised effects, such that in a small catchment the movement of stock is more likely to have a direct influence on the adjacent river length.
The mass-balance for the river-reach channel store is:
Change in channel store numbers=riparian input+deposition from water column−(remobilisation+die-off)×channel store number,
Die-off in the model is driven by sunlight and water temperature and influenced by light attenuation through the full depth of the water column. Gameson & Gould (Citation1975) reported that the effect of sunlight on coliform die-off was additive such that the total die-off can be expressed: k tot =f(Temperature) + f(Sunlight).
The equations relating E. coli die-off to sunlight intensity (irradiance) and light attenuation are taken from the literature (e.g. Pommepuy et al. Citation1992; Auer & Niehaus Citation1993). The equation for temperature is derived from common observations in the literature (as reviewed by Wilkinson Citation2001 and Hipsey et al. Citation2008).
Die-off is related to the ‘strength’ of sunlight (solar irradiance, denoted by I, units: W/m2) by a power law
Die-off related to temperature alone is effectively the darkness die-off (in the absence of sunlight) and is much slower than in sunlight. Die-off in natural waters increases exponentially with temperature according to the equation
The mean values of θ and k 0 from various studies as summarised by Wilkinson (Citation2001) are 0.0285 and 0.1067, respectively, and have been used in the st udy presented here (see also the summary of parameter values in Hipsey et al. Citation2008). The two die-off components are combined to give a value of overall die-off per day for average light intensity in the water column,
Model application and calibration
The first model of the Motueka River storm-event pulses (in Wilkinson Citation2008), a single at-a-point model, could reproduce the observed E. coli pulses but the river mixing parameter values were implausible. Hence a semi-distributed approach with linking river reaches and appropriate travel-time delays was applied to simulate the degree of dispersion needed (Wilkinson Citation2008). This approach was further refined by dividing the Motueka River catchment into 19 sub-catchments. These are the basic calculation unit (boxes) for the model. One river reach is defined for each sub-catchment and there are an additional five linking river reaches along the main channel of the Motueka River (Fig. 1b). The linking reaches were defined based on digitised stream lengths. The model was applied at two scales; the whole Motueka River and the Sherry sub-catchment. For the Motueka River application, a 1-h model time step was used. This worked well with the observed E. coli data and seemed appropriate to the size of this river at Woodmans Bend. In the Sherry River sub-catchment, a 15-min time step was used (the smallest sampling data interval for hydrometric monitoring) because the catchment is smaller and more flashy in its response. A backwards difference approximation scheme is used in application of the model, and all parameters and variables are adjusted from time units of days or hours or seconds, as required, to work in units of the time-step.
The E. coli model was calibrated using measured bacteria concentrations (McKergow & Davies-Colley Citation2010; Ballantine et al. Citation2011). The influence of the Sherry on the main Motueka River was then investigated by running the Sherry model with driving variables for 2003–4, with Sherry flow (prior to installation of the Blue Rock hydrometric station) estimated from the neighbouring Tadmor River at Mudstone. The Sherry model output was then input to the Motueka model. The assumption implicit here, was that the faecal storm-event response of the Sherry River had not changed significantly between 2003–4 and 2008–9, which seems reasonable. The 15-min Sherry E. coli simulations were summed to hourly values prior to being input to the Motueka model. The model fit was achieved by manually adjusting the parameters and was assessed using a variety of means: (1) the visual fit of discrete observed vs 15- or 60-min modelled values; (2) the correspondence of the upper and lower percentiles and median concentrations; and (3) the value of the Nash–Sutcliffe efficiency measure ‘E’ (Nash & Sutcliffe Citation1970); E varies between −∞ and 1 (perfect fit). Model E. coli values are expressed in cfu (culture forming units).
Results
Model parameters: Motueka and Sherry Rivers
The Sherry River required a greater rate of E. coli input (adjusted by N def , ) than the main Motueka River model, and a greater remobilisation rate. The other model fit parameters were the same.
Table 2 Model calibration parameters.
Motueka River time-series
Variations in E. coli concentration follow a log-normal distribution and log10 transformation of the data makes visual comparison of the time-series easier (), and the central tendency is best described by the median or geometric mean.
Figure 5 Modelled time-series and observed Escherichia coli concentrations in the Motueka River at Woodmans Bend from May 2003 to December 2004; channel store numbers in the most downstream river reach (top); water concentrations and discharge for events numbered sequentially; and excerpts showing detail of individually numbered events (bottom).
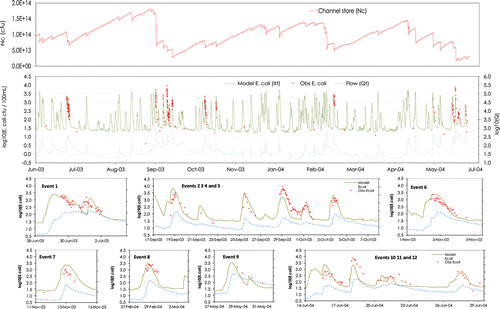
The model appeared to fit both the ‘shape’ and magnitude of observed E. coli concentrations at Woodmans Bend over most events quite well (c), including accounting for complex (non-linear, hysteretic, and otherwise varying) dependence on flow in a way that statistical models cannot. Simulated channel store E. coli numbers show, as expected, a ‘saw tooth’ pattern with an opposite trend from the water concentrations of E. coli, because the bacteria are transferred between channel store and water column, depending on flow conditions (). The channel store builds up gradually between hydrograph events then drops abruptly as the channel store is flushed. The channel store tends to be lower during wet weather when clusters of floods flush E. coli out of storage.
Only 14 dry weather flow E. coli samples were available for the modelled period for the Motueka River at Woodmans Bend. Around half of these values lie below the model line, and the other half are above or close to the line. The observed value from August 2003 is very low (b), and inconsistent with the other low values that occur within a few weeks of a storm-event. It is possible that this particular value may be biased low. The catchment input layer does tend to constrain the range of possible dry-weather flow values and it would be possible to have a greater time variability in N i by increasing the die-off seasonality, and or introducing a seasonality to N Def or reformulating the catchment input layer to incorporate a more realistic treatment of the catchment store. The dry weather samples demonstrate that the E. coli concentrations are low in the Motueka River at Woodmans Bend between storm events; however, further work would be required to identify the inter-storm and diurnal E. coli dynamics in detail.
Sherry River time-series
Escherichia coli in the Sherry River was not as easily modelled as in the main Motueka River (compare and ). In general, the Sherry E. coli concentrations are characterised by fewer observations than in the Motueka at Woodmans Bend (96 as opposed to 252), and there are few observations immediately prior to the events, and there were fewer inter-storm samples (seven as opposed to 14 at Woodmans Bend). Simulations for the steeper hydrograph events tend to fit the observations reasonably well. Peaks driven by broader topped hydrograph events were less well simulated, with the observed peaks generated by broad hydrograph events tending to remain high for longer than those simulated. The cause of this is not clear. Possibly catchment stores persist and ‘feed’ these events; suggesting that at the smaller catchment scale a more detailed description of the catchment store and delivery system is needed. From a closer examination of the flow events, it is apparent that the hydrographs are often composed of more than one peak or rise, which suggests the arrival of water from different parts of the catchment at different times, or even the arrival of groundwater. An investigation of the rainfall time-series might help to clarify this by examining the structure of the triggering rainfall events, but this has not yet been undertaken.
Figure 6 Modelled time-series and observed Escherichia coli concentrations in the Sherry River from November 2008 to October 2009; channel store numbers in the downstream most river reach (top); water concentrations and discharge (middle); and excerpts (below) showing detail of individually numbered events.
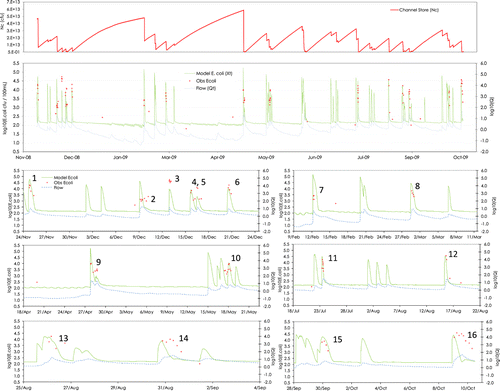
The simulated dry weather flow E. coli concentrations show a lack of variation in concentration over time-scales of days and weeks, but with a noticeable diurnal fluctuation driven by daytime sunlight inactivation (). There were few observations against which to fit the dry weather response, but it might be expected that inter-storm concentrations would exhibit a greater variation because of riparian and direct faecal inputs. In the model, the lack of variation is a consequence of the riparian input layer. Similarly, the diurnal variation would only be observed if the night-time riparian input exceeded the slower darkness rate of die-off, and is an artefact of the simplistic treatment of catchment inputs.
The simulations of the channel store in the Sherry River display a marked ‘saw tooth’ pattern with large variations. The bed store builds up in an exponential pattern between events then is abruptly flushed to low levels by storm wave fronts. The Sherry bed store pattern (a) contrasts with that of the main-stem Motueka (a) in displaying more abrupt drops related to storm events.
Comparison of model fit
The paired observed and modelled values can be compared directly in x–y plots ()—which show good agreement overall for the Motueka River at Woodmans Bend (Nash-Sutcliffe E = 0.54), but more scatter overall in the Sherry at Blue Rock (E = 0.092). The model upper concentration for the Motueka River was around 4500 E. coli/100 ml but several observations exceeded that. The Motueka model generally had symmetric scatter either side of the 1:1 line (a). Modelled concentrations for Events 4 and 11 are underestimated (see also ). The low model values for the Sherry give the impression of bias; however, examination of the time-series plot () shows that this ‘bias’ is generally related to events where the model peaks and drops rapidly, but the observed event pulses remain elevated for longer. This issue is discussed below in the context of broad-topped hydrographs and the treatment of catchment inputs in the model.
Escherichia coli storm dynamics and dispersion characteristics
Several measures were calculated to characterise, and for comparison of, certain aspects of the E. coli event responses in the Motueka and Sherry Rivers (, see also and ). The mixing and dispersion characteristics for a 10-km reach are represented by the mixing time constant T m . The peak duration measures the length of time (h) that the E. coli concentration is elevated above ‘background’ conditions for each pulse. Escherichia coli peak sharpness was calculated as the log of the ratio of peak to baseline concentration divided by peak duration (expressed in days) for each event.
Table 3 Summary statistics for model characteristics for the Motueka (M) and Sherry Rivers (S), S/M and M/S show the ratios of the characteristics for each river.
There were distinct differences between the E. coli storm pulse characteristics in the Sherry and the Motueka Rivers. Modelled dispersion in the Sherry River remains relatively constant and less than in the Motueka River (at Woodmans Bend) over a wide range of flow conditions. Longer mixing time constants apply at low flow and shorter time constants apply at high flow. At Woodmans Bend, the mixing characteristics vary more with flow and time constants are more than twice those in the Sherry at low flow. At high flow the mixing time constant drops (from 2.5) to 1.5 h.
The storm-event pulses in the Sherry were shorter in duration, had higher peaks and were between two and five times sharper than at Woodmans Bend in the Motueka River. The Motueka E. coli peaks were longer than those in the Sherry by a factor of 1.46 (compare and ; ) and varied between 5.0 and 39 h, whereas the shorter Sherry River pulses had durations of between around 3 and 26 h. The median peak sharpness for the Sherry model pulses in 2008/9 was 6.5 (). The sharpest peak had a sharpness of around 50. The peak sharpness values at Woodmans Bend were generally about half those in the Sherry River, indicating peaks of longer duration with sharpness values varying from 2 to around 10. The observed E. coli pulse data for both rivers show that less steep or rounded hydrographs, resulting from extended rainfall events (in contrast to sudden intense downpours), tended to result in longer duration E. coli peaks (e.g. 9 December 2008, 31 August 2009 and 9–10 October 2009).
Discussion
This paper has presented simulations of faecal indicator bacteria (E. coli) concentrations in the Motueka River and its much smaller tributary the Sherry River. The results provide a number of general observations about faecal pollution dynamics in river systems and provide some insight into the differences between the dynamics in catchments of very different scales. Consequently, much of the discussion below approaches different aspects of scale related issues relevant to temporal dynamics and the spatial aspects of faecal source areas.
Scale temporal dynamics in response
Differences in the temporal dynamic nature of the E. coli response at Woodmans Bend and Blue Rock were observed, as measured by peak sharpness and dispersion. These differences are in part a function of scale, but may also relate to the degree of contamination. In terms of scale, in larger catchments issues of timing of arrivals from sub-catchments, the spatial distribution of rainfall, in-stream mixing and the dissipation (attenuation) of flood waves will increase the tendency for responses to be spread-out over time (e.g. Beven Citation1987). Conversely, in smaller catchments, a closer coincidence of pollutant delivery from different parts of the catchment is expected. In the Sherry the relatively small catchment scale and rapid response to hydrological events results in sharp E. coli peaks. The main stem of the Motueka River is much cleaner than the Sherry River, since in the southern and western Motueka River sub-catchments, there are relatively microbially pure waters including drainage from large areas of native forest, plantation forest and scrub-land (c), as well as karst-fed streams that drain areas extending into the Kahurangi National Park (Young et al. Citation2005). In contrast, the Sherry has a high proportion of pastureland with high livestock numbers (about three cows per hectare on dairy farms in the Sherry Valley) causing elevated faecal microbial pollution.
Peaks from local and distant sources
Faecal indicator bacteria such as E. coli tend to accumulate in the channel store with distance downstream, and as a generalisation, channel disturbance becomes more significant, as a source for recontamination of the overlying water, with distance downstream (Hunter et al. Citation1992; Nagels et al. Citation2002; Collins & Rutherford Citation2004; Muirhead et al. Citation2004). Remobilisation of channel stores tends to concentrate bacteria on the hydrograph rise (Wilkinson Citation2008). This is also a consequence of the increasing bed-store with distance downstream. The dispersive mechanism spreads and attenuates peaks. The dispersion and remobilisation mechanisms counteract each other, since remobilisation tends to increase peak sharpness, whereas dispersion (mixing processes) acts to attenuate and spread the remobilised faecal bacteria in the wake of (behind) the flood wave. Observations suggest that bacteria in event pulses are derived from local sources in the river network (McKergow & Davies-Colley Citation2010). Bacteria derived from distant upstream locations have been mixed, and diluted into the tail of the hydrograph, and a proportion settle back into the channel store before reaching the point of interest. The bacteria in the hydrograph rising limb must be locally derived from (channel stores in) the immediate river reaches upstream. The emergence of the use of microbial tracers (e.g. Wyer et al. Citation2009; Cornelisen et al. Citation2011) presents a promising approach for devising experiments to elaborate on the source composition of bacteria in flood-pulses and hence better understand in-stream transport mechanisms.
River network as a contamination shunting system
Assuming that upstream catchment microbial inputs and remobilised bacteria tend to become mixed and spread into the hydrograph tail and settle back out of suspension, a proportion of the bacteria from distant sources will be shunted through the river network with successive storm-events. As die-off in the channel store seems to be slow (with most storage probably in the—comparatively dark—hyporheic zone), the microorganisms comprising a given storm-pulse may be of very mixed ‘age’ composition, some taking weeks to arrive from distant sources, whereas others are from more recent and local sources. The relative contribution of local channel accumulations, and more distant land- and channel-derived inputs, has been under consideration in studies by Muirhead et al. (Citation2004), Nagels et al. (Citation2002) and McBride (Citation2011). For the smaller Sherry River catchment, wash-in may be expected to contribute a greater part of the observed peaks, and is probably more a consequence of the relatively small-scale channel store accumulations. In the Sherry catchment, the channel can only accumulate inputs from local sources, whereas in the larger Motueka catchment, the whole extended catchment area contributes to ‘loading up’ the channel stores, such that the contribution to storm peaks greatly outweighs the catchment wash-in.
Inter-storm microbial levels
During dry weather, the settlement of particle-associated E. coli, clear water, shallow depths, slower flushing and strong sunlight exposure, all combine to favour rapid die-off in the water column—by about a factor of 10 every day. In the Motueka River, dry weather E. coli concentrations are low (around 30 cfu/100 ml), whereas Sherry River values are around a factor of 10 higher. The higher Sherry values probably result from proximal contamination by livestock, particularly cattle, accessing the channel network.
Faecal export rate
In 2008, the export r ate from the (mixed land use) Motueka was 6.6×108 cfu/km2/h (, column iii) and from the Sherry, 2.0×109 cfu/km2/h (, column ix). These export rates are consistent with those published by Kay et al. (Citation2008), who reported values ranging between geometric means of 2.5×108 and 2.5×1010 cfu/km2/h for rough grazing land during low vs high flow, and 8.3×108 and 1.2×1011 for improved pasture. Export rates from catchments that were predominantly woodland had geometric mean export rates between a factor of 10 and 100 less than the pasture catchments (Kay et al. Citation2008). The export rate for the Motueka catchment, which has areas ranging from intensive grazed pasture through to plantation and native forest (Basher Citation2003), is around 2.5 times the geometric mean dry-weather rate for rough grazing in the UK studies, from the Sherry the export rate is around 2.5 times the dry-weather geometric mean for improved pasture.
Table 4 Summaries of observed and modelled E. coli concentrations in the Motueka@Woodmans Bend and Sherry@Blue Rock.
Influence of the Sherry at Woodmans Bend
In order to test the influence of the Sherry sub-catchment on E. coli levels in the Motueka at Woodmans Bend, the Sherry model was run for 2003/4 using driving data for that period and the calibration parameters for 2008. The output faecal time-series was substituted into the model for the Motueka at Woodmans Bend, which was re-run (, column iii and iv). The influence of the Sherry faecal output at Woodmans Bend in the absence of other inputs was tested by switching-off all other sub-catchment bacterial inputs (, column v). The Sherry catchment is more densely stocked with cattle and other livestock than the average of the Motueka catchment, and the export values in suggest that roughly 8% (5.3×107/6.6×108) of the E. coli export at Woodmans Bend was derived from the Sherry, representing only 3.8% of catchment area. So despite the higher concentrations draining the Sherry, the die-off and settlement occurring during travel through the channel network to Woodmans Bend reduce the load by a factor of around 37.
Catchment faecal input
The riparian input layer of the model is a significant simplification of catchment faecal delivery processes and mechanisms (e.g. Collins & Rutherford Citation2004). Wilkinson (Citation2001) used a constant catchment faecal input rate (adjusted only by die-off) and achieved a comparable model efficiency with a more detailed description of catchment input mechanisms.
The constant faecal input rate N Def (cfu/ha/mm/s) is referred to as a defecation rate, but the multiplication by catchment run-off yield means that this name is misleading. The use of run-off yield as a further adjustment to sub-catchment inputs was intended to account for the large gradient in rainfall and hence run-off from south-west to north-east in the Motueka catchment (Basher Citation2003), based-on the assumption that areas with greater rainfall deliver more microorganisms to the river system. In the current formulation, this adjustment is simply a multiplication, which in some sub-catchments effectively increases the defecation rate. A more conceptually appealing approach (without changing the modelling results) might be to apply the yield adjustment as a ‘faecal delivery efficiency’ ranging from 0 to 1, and thus the term defecation rate for N def would still be valid. The adjustment for flow yield takes no account of rainfall temporal variability, this being already accounted for by the river flow, which is the key driving variable. These simplifications of the approaches used by Wilkinson (Citation2001) and Collins & Rutherford (Citation2004) appear to be valid at the larger catchment scale (i.e. the Motueka River), but at smaller scales where river mixing and channel accumulation are less dominant relative to land-based dynamics (such as might be expected in the Sherry River) a more detailed description of the variability with time of catchment inputs may be needed.
Final comment
Beven & Freer (Citation2001) point out that in modelling complex environmental systems there are usually many model structures and sets of calibration parameters that produce acceptable result, and this is true of the work presented here. More than 17 model versions with different formulations were investigated in an attempt to improve some aspect of the model fit or the conceptual representation of the system. The results presented here present just one (the ‘best’ [compromise between model complexity and explanatory power] in our judgement) example. It would be possible to try out alternative structures undertaking many weeks of further work. The datasets used only characterise the end behaviour of two systems, and to make further progress in refining the models and our understanding of these systems, more surveys and data would be needed; notably measurement of channel stores and E. coli distributions, specific assessments of E. coli storm pulses for each major sub-catchment, longitudinal profiles within the Sherry, tracer tests to confirm the travel-times and mixing characteristics of key reaches across a range of typical discharge values. Having said this, to be able successfully to simulate the E. coli pulses passing Woodmans Bend in the Motueka River, with a small number of readily available driving variables, is a useful advance towards prediction of E. coli in coastal waters, and potentially provide warnings for bathers and to the shellfish industry of non-harvest periods.
Conclusions
Small catchments such as the Sherry Catchment tend to react rapidly to storm events, producing short duration (‘sharp’) E. coli peaks. Stormflow E. coli peaks are of longer duration in the much larger Motueka River. In smaller catchments, the degree of mixing and dispersion is lower, the channel store remobilisation rate is greater, and the contribution of land-based sources is likely to be greater (since the relative magnitude of the channel store is less).
Under stormflow conditions, the action of river mixing and dispersion means that event-derived microorganisms (either washed-in or remobilised from the channel store) from distant parts of the catchment contribute minimally to the E. coli peak observed at the catchment outlet. Steep E. coli peaks, arriving ahead of hydrograph peaks, at the Motueka outlet, reflect the resuspension of microorganisms from (nearby) channel stores (McKergow & Davies-Colley Citation2010).
A river system acts as a shunting mechanism for faecal indicator bacteria, which may be displaced by several successive storm-events before eventually reaching the catchment outlet (unless die-off has occurred).
The simplified treatment of catchment delivery processes, loads and the accumulation and flushing of land-based faecal reservoirs, used in this model is suitable for larger catchments, but at the smaller scale of the Sherry Catchment, a more conceptually complete treatment seems desirable. In addition, the specific spatial and temporal patterns of rainfall and erosion may need to be accounted for to characterise smaller catchments adequately where faecal source patchiness may have a greater influence on the observed in-stream dynamics.
Stormflow pulses of poor microbial quality in river plumes have major implications for downstream bathing and shellfish harvesting waters. Simulation of faecal contamination using models such as that presented here have a potentially valuable application to protection of bathing and bivalve shellfish raising waters that are episodically contaminated by plumes from even fairly ‘clean’ rivers such as the Motueka.
Acknowledgements
The able assistance of Rob Merrilees (NIWA-Nelson) on hydrological data and storm sampling for E. coli analysis, and John Nagels (NIWA-Hamilton) for data collation, is gratefully acknowledged.
References
- Anderson CW , Rounds SA 2010 . Use of continuous monitors and autosamplers to predict unmeasured water-quality constituents in tributaries of the Tualatin River , Oregon . US Geological Survey Scientific Investigations Report 2010–5008 .
- Auer , MT and Niehaus , SL . 1993 . Modeling faecal coliform bacteria—1. Field and laboratory determination of loss kinetics . Water Research , 27 : 693 – 701 .
- Ballantine D , Davies-Colley RJ , Merrilees R 2011 . Water quality and pollution loads from the Sherry River . NIWA Client Report No. HAM2010 .
- Basher LR 2003 . The Motueka and Riwaka catchments . Technical report, LCR Lincoln. Landcare Research , Tasman District Council and Cawthron Institute .
- Beven KJ 1987 . Towards a new paradigm in hydrology. Water for the future: hydrology in perspective ( Proceedings of the Rome Symposium, April 1987). IAHS Publ. no. 16 .
- Beven , KJ and Freer , J . 2001 . Equifinality, data assimilation, and uncertainty estimation in mechanistic modelling of complex environmental systems . Journal of Hydrology , 249 : 11 – 29 .
- Biskie HA , Sherer BM , Moore JA , Miner JR , Buckhouse JM 1988 . Fate of manure point loading into a rangeland stream . ASAE Paper 88-2081 . ASAE, St. Joseph, Michigan .
- Campbell N , D'Arcy B , Frost A , Novotny V , Sansom A 2004 . Diffuse pollution: an introduction to the problems and solutions . London , IWA Publishing .
- Collins , R and Rutherford , K . 2004 . Modelling bacterial water quality in streams draining pastoral land . Water Research , 38 : 3700 – 712 .
- Cornelisen CD , Gillespie P , Kirs M , Young RG , Forrest R , Barter P , Knight B , Harwood VJ 2011 . Motueka River plume facilitates transport of ruminant faecal contaminants into shellfish growing waters, Tasman Bay, New Zealand . New Zealand Journal of Marine and Freshwater Research 45 : 477 – 495 .
- Davies-Colley , RJ , Nagels , JW and Lydiard , E . 2008 . Faecal bacterial dynamics and yields from an intensively dairy-farmed catchment . Water Science and Technology , 57 : 1519 – 1523 .
- Davies-Colley , RJ , Nagels , JW , Smith , R , Young , R and Phillips , C . 2004 . Water quality impacts of a dairy cow herd crossing a stream . New Zealand Journal of Marine and Freshwater Research , 38 : 569 – 576 .
- Davies-Colley , RJ and Nagels , JW . 2008 . Predicting light penetration into river waters . Journal of Geophysical Research , 113 : G03028
- Dennison , WC , Lookingbill , TR , Carruthers , TJB , Hawkey , JM and Carter , SL . 2007 . An eye-opening approach to developing and communicating integrated environmental assessments . Frontiers in Ecology and the Environment , 5 : 307 – 314 .
- Environment Agency 2000 . The microbiology of recreational and environmental waters: methods for the enumeration of waters and associated material . standing committee of analysts . Bristol , The Environment Agency .
- Fenemor A , Phillips C , Allen W , Young RG , Harmsworth G , Bowden W , Basher L , Gillespie P , Kilvington M , Davies-Colley R , Dymond J , Cole A , Lauder G , Davie T , Smith R , Markham S , Deans N , Stuart B , Atkinson M , Collins A. Integrated catchment management—interweaving social process and science knowledge . New Zealand Journal of Marine and Freshwater Research 45 : 307 – 311 .
- Gameson ALH , Gould DJ 1975 . Effects of solar radiation on the mortality of some terrestrial bacteria in sea water . In : Gameson ALH . Proceedings of the International Symposium on Discharge of Sewage from Sea Outfalls . London , Pergamon Press .
- Garcia , M , Nino , Y and Lopez , F . 1996 . “ Laboratory observations of particle entrainment into suspension by turbulent bursting ” . In Coherent flow structures in open channels , Edited by: Ashworth , PJ , Bennet , SJ , Best , JL and McLelland , SJ . New York : John Wiley .
- Graham , AA . 1990 . Siltation of stone-surface periphyton in rivers by clay-sized particles from low concentrations in suspension . Hydrobiologia , 199 : 107 – 115 .
- Henderson-Sellers B , Young PC , Ribiero da Costa J 1988 . Water quality models: rivers and reservoirs . In: Proceedings of the International Symposium on Water Quality Modelling of Agricultural Non-point Sources, Part 1 . Logan, UT , Utah State University , ARS-81
- Hipsey , MR , Antenucci , JP and Brookes , JD . 2008 . A generic, process-based model of microbial pollution in aquatic systems . Water Resources Research , 44 : W07408
- Hunter , C , McDonald , AT and Beven , KJ . 1992 . Input of faecal coliform bacteria to an upland stream channel in the Yorkshire Dales . Water Resources Research , 2.8 : 1869 – 1876 .
- Jamieson , R , Gordon , R , Joy , D and Lee , H . 2004 . Assessing microbial pollution of rural surface waters—a review of current watershed scale modeling approaches . Agricultural Water Management , 70 : 1 – 17 .
- Jeje , LK , Ogunkoya , O and Oluwatimilehin , JM . 1991 . Variation in suspended sediment concentration during storm discharges in three small streams in the upper Osun Basin, Central Western Nigeria . Hydrological Processes , 5 : 361 – 369 .
- Jenkins A 1984 . The effect of fluvial processes on the dynamics of sanitary bacteria in upland streams . Unpublished PhD thesis, University of Leeds .
- Jenkins , A , Kirkby , MJ , McDonald , AT , Naden , P and Kay , D . 1984 . A process based model of faecal bacterial levels in upland catchments . Water Science and Technology , 16 : 453 – 462 .
- Kay D , McDonald AT 1980 . Towards an understanding of the effects of catchment management on water quality . Technical Paper , Lancaster IBG .
- Kay , D , McDonald , A , Stapleton , C , Wyer , M and Fewtrell , L . 2006 . Europe: a challenging new framework for water quality . Proceedings of ICE Civil Engineering , 159 : 58 – 64 .
- Kay , D , Edwards , AC , Ferrier , B , Francis , C , Kay , C , Rushby , L , Watkins , J , McDonald , AT , Wyer , MD , Crowther , J and Wilkinson , J . 2007 . Catchment microbial dynamics: the emergence of a research agenda . Progress in Physical Geography , 31 : 59 – 76 .
- Kay , D , Crowther , J , Stapleton , CM , Wyer , MD , Anthony , S , Bradford , M , Edwards , A , Fewtrell , L , Francis , C , Hopkins , M , Kay , C , McDonald , AT , Watkins , J and Wilkinson , J . 2008 . Faecal indicator organism concentrations and catchment export coefficients in the UK . Water Research , 42 : 2649 – 2661 .
- Kirk , JTO . 1994 . Light and photosynthesis in aquatic ecosystems , New York : Cambridge University Press .
- McBride , GB . 2011 . Explaining differential sources of zoonotic pathogens in intensively-farmed catchments using kinematic waves . Water Science and Technology , 63 : 695 – 703 .
- McDonald , AT , Kay , D and Jenkins , A . 1982 . Generation of faecal and total coliform surges by stream flow manipulation in the absence of normal hydrometeorological stimuli . Applied Environmental Microbiology , 44 : 292 – 300 .
- McKergow , LA and Davies-Colley , RJ . 2010 . Stormflow dynamics and loads of Escherichia coli in a large mixed land use catchment . Hydrological Processes , 24 : 276 – 289 .
- Morowitz , HJ . 1950 . Absorption effects in volume irradiation of micro-organisms . Science , 111 : 229 – 230 .
- Muirhead , RW , Davies-Colley , RJ , Donnison , AM and Nagels , JW . 2004 . Faecal bacteria yields in artificial flood events: quantifying in-stream stores . Water Research , 38 : 1215 – 1224 .
- Nagels , JW , Davies-Colley , RJ , Donnison , AM and Muirhead , RW . 2002 . Faecal contamination over flood events in a pastoral agricultural stream in New Zealand . Water Science and Technology , 45 : 45 – 52 .
- Nash , JE and Sutcliffe , JV . 1970 . River flow forecasting through conceptual models, Part I—A discussion of principles . Journal of Hydrology , 10 : 282 – 290 .
- Pommepuy , M , Guillaud , JF , Dupray , E , Derrien , A , Le Guyader , F and Cormier , M . 1992 . Enteric bacteria survival factors . Water Science and Technology , 25 : 93 – 103 .
- Pope , ML , Bussen , M , Feige , MA , Shadix , L , Gonder , S , Rodgers , C , Chambers , Y , Pulz , J , Miller , K , Connell , K and Standridge , J . 2003 . Assessment of the effects of holding time and temperature on E. coli densities in surface water samples . Applied Environmental Microbiology , 69 : 6201 – 6207 .
- Price , LE , Goodwill , P , Young , PC and Rowan , JS . 2000 . A data-based mechanistic modelling (DBM) approach to understanding dynamic sediment transmission through Wyresdale Park Reservoir, Lancashire, UK . Hydrological Processes , 14 : 63 – 78 .
- Qin , D , Bliss , JP , Barnes , D and FitzGerald , PA . 1991 . Bacterial (total coliform) die-off in maturation ponds . Water Science and Technology , 23 : 1525 – 1534 .
- Reynolds , CS , Carling , PA and Beven , KJ . 1991 . Flow in river channels: new insights into hydraulic retention . Archives of Hydrobiology , 121 : 171 – 179 .
- Richardson , K and Carling , PA . 2006 . The hydraulics of a straight bedrock channel: insights from solute dispersion studies . Geomorphology , 82 : 98 – 125 .
- Sarikaya , HZ , Asce , AM , Saatci , AM and Abdulfattah , AF . 1987 . Effect of pond depth on bacterial die-off . Journal of Environmental Engineering , 113 : 1350 – 1362 .
- Solo-Gabriele , HM , Wolfert , MA , Desmarais , TR and Palmer , CJ . 2000 . Sources of Escherichia coli in a coastal subtropical environment . Applied Environmental Microbiology , 66 : 230 – 237 .
- Stadler , H , Skritek , P , Sommer , R , Mach , RL , Zerobin , W and Farnleitner , AH . 2008 . Microbiological monitoring and automated event sampling at Karst Springs using LEO-satellites . Water Science & Technology , 58 : 899 – 909 .
- Stott , R , Davies-Colley , R , Nagels , J , Donnison , A , Ross , C and Muirhead , R . 2011 . Differential behaviour of Escherichia coli and Campylobacter spp. in a stream draining dairy pasture . Journal of Water and Health , 09 : 59 – 69 .
- VanSickle , J and Beschta , RL . 1983 . Supply limited models of sediment transport in streams . Water Resources Research , 19 : 768 – 778 .
- Wallis , SG , Crowther , JM , Curran , JC , Milne , DP and Findlay , JS . 1989 . “ Consideration of one-dimensional transport model of the Upper Clyde Estuary ” . In Advances in water modelling and measurement , Edited by: Palmer , HA . Cranfield : BHRA .
- Wilkinson J 2001 . Modelling faecal coliform dynamics in streams and rivers . Unpublished PhD thesis , University of Wales , Aberystwyth .
- Wilkinson J 2008 . Faecal indicator organism modelling (FIO): application to Motueka River . Prepared for Integrated Catchment Management project. Cawthron Report No. 1454 .
- Wilkinson , J , Jenkins , A , Wyer , M and Kay , D . 1995 . Modelling faecal coliform dynamics in streams and rivers . Water Research , 29 : 847 – 855 .
- Wilkinson , J , Kay , D , Wyer , M and Jenkins , A . 2006 . Processes driving the episodic flux of faecal indicator organisms in streams impacting on recreational and shellfish harvesting waters . Water Research , 40 : 153 – 161 .
- Wyer M , Kay D , Stapleton C , Watkins J , Davies C , Moore H 2009 . An investigation into bathing water quality at Amroth, Pembrokeshire . CREH Report to Welsh Environment Agency .
- Young , RG , Quarterman , AJ , Eyles , RF , Smith , RA and Bowden , WB . 2005 . Water quality and thermal patterns of the Motueka River: influences of land cover, geology and position in the catchment . New Zealand Journal of Marine and Freshwater Research , 39 : 803 – 825 .