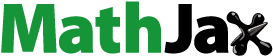
Abstract
Socio-economic differences in mortality are among the most pervasive characteristics of Western societies. While the mortality gradient by income is well established for the period after 1970, knowledge about the origins of this gradient is still rudimentary. We analyse the association between income and cause-specific adult mortality during the period 1905–2014 in an area of southern Sweden, using competing-risk hazard models with individual-level longitudinal data for over 2.2 million person-years and over 35,000 deaths. We find that the present-day income gradient in adult mortality emerged only in the period after the Second World War and did so for the leading causes of death and for men and women largely simultaneously.
Introduction
A clear health and mortality gradient by socio-economic status (SES) is evident both in liberal market societies, such as the United States (US), and in Scandinavian welfare states with mostly public and equal access to healthcare (Mackenbach Citation2019). No matter which indicator of SES is used, higher status is related to better health and longer life (e.g. Cutler et al. Citation2012). Most research has analysed the association between education or social class on the one hand and mortality or self-rated health on the other (see e.g. Cutler and Lleras-Muney Citation2008; Mackenbach et al. Citation2016). The literature on the relationship between income and health/mortality is more limited but generally shows a clear gradient in terms of mortality or life expectancy (Wamala et al. Citation2006; Torssander and Erikson Citation2010; Kondo et al. Citation2014; Chetty et al. Citation2016; Hederos et al. Citation2018). Most of these studies also show a widening of mortality differences over time, although few studies have analysed the period before 1990. Research focusing on cause-specific patterns has suggested that the widening of mortality differentials by income in recent times is especially related to circulatory diseases, alcohol-related diseases, and some forms of cancer (Tarkiainen et al. Citation2012; Hederos et al. Citation2018).
Knowledge of mortality differentials by income and other dimensions of SES further back in time is more limited. While many researchers seem to assume that they were as large, or even larger, in the past (Marmot Citation2004; Elo Citation2009; Deaton Citation2016), recent findings have indicated a rather late emergence of the social class gradient in adult mortality (Bengtsson and Dribe Citation2011; Edvinsson and Lindkvist Citation2011; Edvinsson and Broström Citation2012; Bengtsson et al. Citation2020; Debiasi and Dribe Citation2020). Research on the long-term trends (going back to the period before 1970) in socio-economic differentials in mortality has focused almost exclusively on occupational class, and there are no previous studies considering the long-term association between income and cause-specific mortality. Importantly, different dimensions of SES, such as income, education, and class, are not completely overlapping but to some extent reflect different aspects of social stratification (Erikson and Goldthorpe Citation2010; Blanden Citation2013; Breen et al. Citation2016; Mood Citation2017). Hence, it cannot be expected, without further qualification, that mortality differentials developed identically across the different dimensions, and research on contemporary Sweden has demonstrated similar mortality gradients even when including multiple measures of SES in the same model (Torssander and Erikson Citation2010). Therefore, this study makes an important contribution by complementing previous research on social class differentials with findings about the long-term relationship between income and cause-specific mortality.
In this paper we analyse the relationship between relative income and adult cause-specific mortality during the period 1905–2014 in an area of southern Sweden. The area includes an industrial port town (population of 14,500 in 1900 and 27,500 in 2000) and five nearby rural and semi-rural parishes (combined population of 5,500 in 1900 and 10,000 in 2000 according to our data; see Data and variables section). For this area, we have individual-level longitudinal data on cause-specific mortality, occupation, and individual income from 1905 to 2014. For the entire population of Sweden, such detailed data are not available until 1968. We study the relationship between income quintiles and total and cause-specific adult and old-age mortality for different sub-periods to establish if the present-day pattern has always existed or, if not, when it emerged.
Our findings confirm the existence of an income gradient in adult mortality in contemporary Sweden, for both men and women and for all cause-of-death groups. We also confirm a widening of income differences in mortality since 1970. More importantly, however, we demonstrate that this modern mortality gradient is of quite recent origin and did not emerge until after 1950. When it emerged, the gradient first became visible for infectious diseases and then appeared in circulatory diseases, respiratory diseases, cancers, and external causes.
The rest of the paper is organized as follows. We start by reviewing previous research on the income gradient and the main theoretical pathways linking SES to health and mortality. Next we discuss data and variables, followed by the empirical design. We then present the estimation results, and the final section provides a concluding discussion.
Background
Previous research
While inequalities in health in contemporary societies are well documented and reasonably well understood, the same is not true for historical contexts, which means that we lack knowledge about when and why these differentials emerged. It is often assumed that socio-economic differences in health and mortality were greater in the past, but the empirical evidence to support this assumption is rather scant.
Most studies on socio-economic mortality differentials have been based on occupation (status or social class) or education, whereas there has not been as much focus on income (Bengtsson et al. Citation2020; Debiasi and Dribe Citation2020). Studies that have evaluated several dimensions of SES at the same time have often found that the different dimensions only partly overlap in their associations with mortality (e.g. Geyer et al. Citation2006). Income, for example, has been shown to be associated with health and mortality even when accounting for other socio-economic dimensions, such as class or education (e.g. Smith Citation2004; Torssander and Erikson Citation2010).
Several previous studies of the period after 1970 have demonstrated a clear mortality gradient by income, wealth, or sometimes poverty (Smith Citation1999; Wamala et al. Citation2006; Elo Citation2009; Torssander and Erikson Citation2010; Tarkiainen et al. Citation2012; Kondo et al. Citation2014; Hederos et al. Citation2018). In a study using Swedish register data for 1970–2007, Hederos et al. (Citation2018) showed a clear and consistent association between income and life expectancy at age 35, with income differentials increasing over time more so for men than for women. A register-based study covering the period 1990–2007 found increasing mortality differentials by income for both men and women in Sweden (Kondo et al. Citation2014).
Hederos et al. (Citation2018) also analysed the trends by cause of death and found that mortality from circulatory diseases was especially important for the increasing mortality differentials. Tarkiainen et al. (Citation2012) reported similar findings for Finland in the period 1988–2007, where differences in adult life expectancy by income also widened, possibly due to increasing mortality from alcohol-related illnesses in the lowest income quintile.
A similar mortality gradient by income was found in the US between 1999 and 2014, without any noticeable non-linearities (Chetty et al. Citation2016). As in Sweden and Finland, the mortality inequality increased over time, as improvements in life expectancy were faster for the higher-income groups than the lower-income groups. In fact, at the bottom of the income distribution there was almost no progress at all in life expectancy. Analysing more detailed geographical patterns, they also found that life expectancy among the poorest was correlated with health behaviour but not with access to healthcare, physical environment, labour market factors, or income inequality. Kinge et al. (Citation2019) found similar results for Norway in an analysis of full-count register data for the period 2005–15: as in both Sweden and the US, these differentials increased in Norway over time, mainly due to mortality from cardiovascular disease, cancer, and respiratory disease.
These studies have dealt exclusively with the last 50 years or, in some cases, only the last 10 years, periods for which nationwide register data are available. Our main contribution is to study how these mortality differentials by income developed over a much longer time period, to gain a better understanding of the origins of the mortality gradient and its underlying mechanisms. Previous studies have analysed the long-term development of social class differences in adult all-cause (Bengtsson et al. Citation2020) and cause-specific mortality (Debiasi and Dribe Citation2020). They have pointed to a late emergence of the mortality gradient. It was not until after 1970 that the social class gradient in mortality was fully developed for both men and women. It emerged somewhat earlier for women but in all broader cause-of-death groups at the same time. Previous research has stressed that different dimensions of SES are related to health and mortality through different pathways and that social class, income, and education are independently associated with various health outcomes to a considerable extent (Geyer et al. Citation2006; Torssander and Erikson Citation2010; Cutler et al. Citation2012). Hence, income might be associated with all-cause and cause-specific mortality in different ways from social class.
Possible pathways between income and health
While the main focus of this paper is to chart the development of mortality differentials by income over time, we use the previous research summarized here to discuss and theorize on the likely pathways for the associations we observe. Five broader sets of factors linking income with health (and mortality) have often been mentioned: (1) lifestyle (including diet and nutrition); (2) access to healthcare; (3) physical environment; (4) psychosocial stress; and (5) reverse causality.
First, lifestyle factors include smoking, diet, exercise, and alcohol consumption, which have well-documented effects on a number of health outcomes. In modern developed societies, low status is usually associated with higher smoking prevalence, higher alcohol consumption and drug use, greater inactivity, and higher obesity rates (Cavelaars et al. Citation2000; Marmot Citation2004; Elo Citation2009; Adler and Stewart Citation2010; Case and Deaton Citation2020). Previous findings have shown that income does not affect health and mortality to any great extent in adulthood but that education and social class exert an influence on health, mainly through behaviour and lifestyle factors (Cutler et al. Citation2012).
Poor nutrition has sometimes been argued to have been an important cause of premature death in pre-industrial and early-industrial societies (e.g. McKeown Citation1976; Fogel Citation2004), but others have played down the role of nutrition (e.g. Szreter Citation1988). In early-twentieth-century Sweden, nutritional status appears to have been sufficiently high for the calorific needs of even lower-status workers in both rural and urban areas (Lundh Citation2013), following considerable improvements in diet and nutrition between the late nineteenth century and the first decades of the twentieth century (Hirdman Citation1983, ch. 1–2). Hence, we do not expect variation in nutrition by income to have been large enough to produce income differentials in adult mortality, even in the early twentieth century.
Second, access to healthcare could be an explanation behind income differences in health and mortality, especially in contexts lacking universal provision of healthcare at low cost (Adler and Stewart Citation2010) but also in contexts where healthcare is universal or close to universal (van Doorslaer et al. Citation2000). The latter could be related to underutilization of health services by lower-SES groups (Steingrímsdóttir et al. Citation2012) and might also explain why there is no strong evidence that increased provision of healthcare actually reduces the health gradient (Smith Citation1999). Simply providing more universal healthcare is likely not enough to eliminate the health gradient in contemporary societies (Adler and Stewart Citation2010).
Third, environmental factors could be part of the explanation for income differences because income is an important determinant of residential location and exposure to work hazards. Nonetheless, it still remains unclear how much this factor actually contributes to the health gradients observed in the contemporary Western world (Adler and Stewart Citation2010); it may have been more important in the past.
Fourth, Marmot (e.g. Citation2004) has emphasized the role of psychosocial stress for health and mortality differentials. The degree of control an individual has over their life situation is important for psychological well-being, and the lack of such control may lead to stress, which negatively affects health through different physiological mechanisms. In particular, the combination of high stress exposure and a limited ability to cope with it can lead to negative health effects (Adler and Stewart Citation2010). Long-term exposure to stress will take a high toll on bodily functions through frequent use of various physiological coping mechanisms, creating what is often referred to as ‘allostatic load’ (Smith Citation1999; Adler and Stewart Citation2010). Lower income is often associated with a lower degree of control over work and hence higher work-related stress.
Finally, causality is a major issue in the vast literature on socio-economic health differentials. While many have assumed and theorized that economic status has a causal effect on health and mortality, others have pointed out that there is also a causal effect from health to economic status (Smith Citation1999, Citation2004; Deaton Citation2003; Cutler et al. Citation2012; Montez and Friedman Citation2015). Poor health affects investment in education and labour supply, with important long-term effects on income. Deterioration in health status also affects income through sickness absence or reduced work hours. It has been well established in previous research that low nutrition and exposure to disease early in life have long-lasting impacts on health and other outcomes through impaired organ development and cognitive ability (Elo and Preston Citation1992; Barker Citation1998; Bengtsson and Lindström Citation2000; Bengtsson and Broström Citation2009; Case and Paxson Citation2009; Quaranta Citation2014). Hence, parental income could have a direct effect on the health and cognitive development of children, which in turn could influence both their health and earnings in adulthood and thereby explain some of the association between income and health in adulthood (Currie Citation2009; Cutler et al. Citation2012).
Studies using different quasi-experimental designs have shown causal effects of different dimensions of SES on health or mortality (Lindahl Citation2005; Kawachi et al. Citation2010; Lundborg et al. Citation2016). While some studies have found causal effects of income on health using different empirical designs (Lindahl Citation2005; Kawachi et al. Citation2010), another did not find any effects on health and mortality from random wealth shocks following lottery wins (Cesarini et al. Citation2016). We do not assess the direction of causality but focus on the long-term development of the association between income and cause-specific mortality, acknowledging that such associations might reflect causal effects in both directions.
Data and variables
The Scanian Economic–Demographic Database (SEDD)
We used data from the Scanian Economic–Demographic Database (SEDD); these data are linked to nationwide official register data from Statistics Sweden and the National Board of Health and Welfare. SEDD consists of individual-level longitudinal information from five rural and semi-urban parishes and a port town (Landskrona) in southern Sweden, covering the period from approximately 1650 to 1968 (Bengtsson, Dribe, Quaranta et al. Citation2020; Dribe and Quaranta Citation2020). The database is one of very few that follows individuals across multiple generations from pre-industrial times up until the present and contains detailed information on both occupation and income as well as data on different demographic outcomes and causes of death. The parishes included are not a representative sample of Sweden in a statistical sense, but the area is not atypical and reflects conditions shared by most rural and semi-urban areas at the time studied (see Dribe et al. Citation2015). Furthermore, in terms of both mortality development and social class differences in all-cause mortality, previous studies have shown similar results for the area under study and Sweden as a whole (Lazuka Citation2017; Bengtsson and Dribe Citation2022).
Information in SEDD comes from continuous population registers (household-based registers, where information at the individual level is continuously updated) containing information on demographic events, including migration to and from households, for all individuals in the selected parishes. Birth and death registers have been used to adjust for possible under-recording of events in the population registers (Bengtsson, Dribe, Quaranta et al. Citation2020). In our analysis we used a spell-based extraction from the database (Quaranta Citation2015, Citation2016).
From 1968 onwards, individual-level information covering the entire country is available from various administrative registers at Statistics Sweden and the National Board of Health and Welfare. Data from these registers were linked by Statistics Sweden to the historical sample using unique personal identification numbers available from 1947, which allowed an extension of the database along several dimensions. First, individuals who had ever lived in the area prior to 1968 and who were still alive in that year were followed until 2014 or until death or emigration, regardless of their geographic location in Sweden. Additionally, spouses, parents, grandparents, children, and siblings of individuals belonging to the original population in Scania (the southern-most province of Sweden in which the five parishes and Landskrona are located) were added to the database if they were alive and living in Sweden sometime after 1967. All individuals added to the sample population were similarly followed until 2014 or their death or emigration from Sweden.
Periodization
Our period under study began in 1905, the first year for which we had access to individual-level income data as well as other demographic data, for both the five parishes and the port town of Landskrona. Based on social, economic, and epidemiological changes over the last century, we divided the study period into five sub-periods: 1905–21, 1922–49, 1950–67, 1968–89, and 1990–2014. The choice of periodization also accounted for changes in data sources and availability. The long-term economic development of the twentieth century was characterized by a cyclical pattern of growth and stagnation, with profound structural changes in the economy (Schön Citation2010). Mortality declined continuously over the century, with life expectancy at birth increasing from 54.5 for men and 57.0 for women in 1901–10 to 80.0 and 83.8, respectively, in 2015 (SCB Citation2019).
The first period (1905–21) was characterized by a continuation of the industrialization that began in the nineteenth century and constituted the real breakthrough of industrial society (Schön Citation2010). The period also included the First World War, which Sweden did not participate in but was indirectly affected by. It was also the period of the Spanish influenza pandemic, which killed approximately 40,000 people in Sweden (out of a population of approximately 6 million). In 1913, a universal pension system was enacted but with low levels of compensation (Edebalk Citation1996). The period also saw a continuation of the improvements in water and sanitation systems that began in the nineteenth century and which led to dramatic improvements in living conditions, especially in urban areas (Sundin and Willner Citation2007; Helgertz and Önnerfors Citation2019).
The 1922–49 period saw both rapid economic growth and then deep economic crisis during the Great Depression of the 1930s. Overall, Sweden experienced faster economic growth than average for Europe (Schön Citation2010). This was also a period with rapidly falling income inequality, at least between the top earners and the rest of the population (Roine and Waldenström Citation2008), and it witnessed the introduction of early welfare reforms, such as unemployment insurance, maternity benefits, and childcare (Magnusson Citation2000). As a result of previous improvements in urban and rural infrastructures (e.g. housing, water, and sanitation), disease patterns shifted from infectious diseases to chronic (in particular circulatory) diseases. Other measures were also taken in this period, including the rationing of alcohol consumption, which contributed to keeping alcohol-related diseases and deaths to a minimum (Sundin and Willner Citation2007, p. 159).
The period 1950–67 fell within a longer period of record economic growth in many industrial economies, Sweden included. Between 1950 and 1975, the Swedish economy grew annually by over 3 per cent per capita, while the population grew by only 0.6 per cent (Schön Citation2010). Income inequality continued to decline (Roine and Waldenström Citation2008), and the labour force participation of married women increased rapidly, connected to a large extent to increasing demand for labour from both industry and the growing public sector including childcare, education, and health and welfare (Stanfors Citation2014). This was also a period of high investment in housing and urban infrastructure. Overall, the living standards of the Swedish population increased greatly, and these increases were distributed across income groups through income transfers and provision of public services. As in other Western countries, it was a period of great progress in medical knowledge and technology, which contributed to the continuous increase in life expectancy, albeit with a growing gap between men and women.
During the last two periods (1970 onwards), the welfare state was developed further in terms of income security, education, childcare, and healthcare and covering broad areas of society. After 1973, many industrial economies, including Sweden, suffered from a major structural crisis and struggled with low economic growth and high unemployment. After the industrial crisis, globalization and computerization created new conditions for economic growth but at lower levels than during the 1950s and 1960s (Schön Citation2010). From the 1980s, this development was also connected with new (neoliberal) economic and social policies and rising economic inequality in Sweden, as in other Western countries (Roine and Waldenström Citation2008). Previous labour-related immigration from southern Europe to Sweden was replaced by large-scale immigration of refugees, many of whom came from less developed countries in Africa and Asia (Bengtsson et al. Citation2005). The introduction of new medical drugs and treatments, including large-scale vaccinations in the period after 1950, contributed to a further decline in mortality. However, the withdrawal of alcohol rationing measures in the mid-1950s resulted in a sharp increase in alcohol consumption and alcohol-related deaths (e.g. liver cirrhosis) in the following two decades (Norström Citation1987; Sundin and Willner Citation2007). In the last period, after 1990, the prevalence of tobacco smoking declined significantly, albeit with pronounced socio-economic differences. The lower-status groups lagged behind in this development, producing increasing socio-economic differentials in smoking prevalence (Nordlund Citation2005).
Measuring income
For the entire period 1905–2014, we used information about income from individual tax returns, which were mandated from 1903 onwards (see Helgertz et al. Citation2020). Given that we covered a period of over a century, we needed to account for changes in the tax system. For example, as income-based benefits (unemployment, sickness, parental leave) were introduced, they were included in total income; this was the case mainly for the post-1970 period.
Both men and women had to report their income. Until 1971 Sweden practised joint taxation of married spouses, which affected the reporting of married women’s earnings. Until 1947, married women were not usually included in income and taxation registers, but their earnings were included in their husbands’ tax returns. Between 1948 and 1953, married women’s earnings were included separately from those of their spouses in the registers, and between 1954 and 1971, both income and taxes were reported separately. Nonetheless, until the 1971 tax reform, the taxes to be paid were calculated based on the earnings of both spouses together. To obtain as homogeneous as possible a measure over the entire period, we calculated family income by adding together the incomes of both spouses. In a sensitivity analysis, we also analysed individual income for both men and women for the period after 1950, when such information became available.
There were income thresholds in the tax system, and income below the threshold did not have to be reported. The thresholds remained quite constant over time, and as a consequence, people increasingly had to report an income (see Helgertz et al. Citation2020 for a detailed description of the sources and income measures). In the case of farmers, taxable income was calculated based on the value of their farm, since farmers would otherwise report no or very low income. This was particularly the case at the beginning of the period under study, when famers could to a large extent live on what they produced at the farm. Sometimes, wealthy individuals reported no or very low income, and then a taxable income was calculated by the tax authorities as a fraction of their wealth (1/60 until 1938 and 1/100 between 1939 and 1948, see Roine and Waldenström Citation2008).
In our analyses, we used total income from sources related to labour (including self-employment) and income from capital and real estate. Before 1968, the income and taxation registers included two different total labour-related income measures: the sum of declared income and the income assessed by the taxation board (for state tax) (Helgertz et al. Citation2020). We used the higher of the two, to give the best approximation of actual earnings. Before 1948, the higher income was usually the income assessed by the taxation board for state tax, whereas the difference was negligible after 1948. For 1968 onwards, we relied on income data from national registers maintained by Statistics Sweden. We used the total income from labour, self-employment, various benefits relating to previous labour earnings (pensions, parental leave benefits, unemployment benefits, etc.), and net taxable capital income. For older people, namely those aged 60–89, we used their highest income recorded between ages 50 and 59 to classify them into an income group for the analyses.
We calculated income in constant prices using the consumer price index (SCB Citation2020). We derived quintiles using an approach adopted by Hederos et al. (Citation2018). First, we ran fully interacted regressions of annual income on birth cohort, year, and sex, keeping the residuals for each individual-year observation. Second, we used a three-year average, including the residual from the current year together with the residuals from the preceding two years (e.g. the residual in 1980 was the average of the residuals in 1978, 1979, and 1980). This was carried out to mitigate possible short-term variations in reported earnings. Finally, for each individual in each year, the income measure was assigned to the corresponding quintile. When estimating the mortality differentials by income quintile, we controlled for marital status, which adjusted for the higher income assigned to married couples through the use of family income.
For the studied years before the introduction of contemporary administrative registers in 1968, SEDD includes annual income records from 1905 to 1967 for the five parishes and from 1947 onwards for Landskrona. Prior to 1947, income records for Landskrona were digitized approximately every five years (see for a summary). For years in which Landskrona’s income information was not available, we imputed the data (see supplementary Tables A1–A3) using income values from the previous and next closest years and detailed occupational records accounting for sex and age, by employing a predictive mean matching method (Morris et al. Citation2014; Kleinke Citation2017). See the supplementary material (section 1) for a detailed account of the imputation procedure and consideration of the validity and robustness of the imputed data.
All-cause and cause-specific adult mortality
We studied both all-cause and cause-specific mortality for men and women aged 30–89. Before 1968 causes of death in SEDD were reported as text strings, which have been coded into ICD-10 following Hiltunen and Edvinsson (Citation2018). For the period from 1968, we used information about cause of death from the official cause-of-death register (Dödsorsaksregistret) maintained by the National Board of Health and Welfare. In this register, causes of death are coded following successive versions of the ICD. More specifically, the ICD codes are as follows: ICD-8 between 1968 and 1986; ICD-9 in the period 1987–96; and ICD-10 from 1997 until the end of the observation period.
We adopted two levels of detail for cause-specific mortality, grouping ICD codes according to two different classifications. In a first step, we divided ICD codes into non-preventable and preventable mortality. This was done following the Avoidable Mortality in the European Union classification, which provides a list of preventable causes of death; all other diseases not included in this list are considered non-preventable (AMIEHS Citation2011; Ericsson et al. Citation2019). Among the most common non-preventable diseases are malignant neoplasm of prostate, malignant neoplasm of pancreas, and unspecified dementia. Among the most common preventable diseases are acute myocardial infarction, chronic ischemic heart disease, and malignant neoplasm of bronchus and lung. Developments in healthcare, medical treatments, and knowledge about risk factors have certainly influenced the preventability of certain diseases over time. Hence, in a second step, we categorized causes of death following the ICD chapter, ultimately constructing a more objective and detailed measure. This resulted in seven cause-of-death groups: (1) infectious and parasitic diseases; (2) circulatory diseases; (3) respiratory diseases (including lung, larynx, trachea, bronchus, lip, oral cavity, and pharynx cancers); (4) other cancers; (5) external causes; (6) other causes; and (7) missing and ill-defined causes of death (see Debiasi and Dribe Citation2020). Group 6, other causes, includes individuals with an assigned ICD code that does not belong to any of the previous groups. Group 7, missing and ill-defined causes, includes individuals with missing information and, before 1968, individuals with a cause-of-death string reported in the historical register that could not be coded into ICD.
Methods
We used a competing-risk hazard model with causes of death as competing outcomes, such that:
(1)
(1) where
represents an individual’s expected hazard at age t of dying from cause c;
identifies the baseline hazard; and
is a set of covariates with the respective regression coefficient. Each model represents a separate Cox regression for each cause of death, and the estimates reflect the effect of covariates on the mortality hazard from such a cause (Lau et al. Citation2009; Austin et al. Citation2016). Individuals who die from a different cause are right-censored. The hazard ratios indicate the change in the risk of death from the disease of interest due to a change in a given covariate among subjects who are still alive. The assumption of proportional hazards was assessed using a test based on scaled Schoenfeld residuals (using the estat phtest in Stata). We complemented the tests with log–log plots of the survival probability, to evaluate the proportionality. The graphs revealed a non-parallelism, with the lines converging mostly at the high extremes of the age scale (supplementary Figures A1 and A2). To gain a better understanding of how the association under study might change across the time frame (age in this case), we ran a set of models separating the sample into two age groups, namely 30–59 and 60–89 years.
The main analysis was carried out separately by sex and period (1905–21, 1922–49, 1950–67, 1968–89, and 1990–2014), controlling for birth year, marital status, parish of residence, and country of birth (Swedish born or foreign born). Birth year was included as a continuous variable as we did not expect marked non-linearities within the confined time periods analysed. We also ran one set of models adjusting for social class and a number of sensitivity analyses to test the robustness of the findings. For married couples we used the highest class position in the family: usually, but not always, the class position of the husband. We included four separate classes: non-manual workers, manual workers, farmers, and missing. See Debiasi and Dribe (Citation2020) for an in-depth analysis of social class differences in adult mortality in the same area using a more detailed class scheme.
The analytical sample includes more than 2.2 million person-years and over 35,000 deaths. shows the descriptive statistics for men and women in the sample. The changing distributions of social class across periods reflect the social and economic transformation that occurred in Sweden during the twentieth century. There is a clear decline in the share of farmers and unskilled workers over time (from 7.5 to 1.6 per cent and from 22.6 to 3.2 per cent, respectively, for men) and a parallel increase in the share of non-manual occupations, from approximately 20 to over 50 per cent for both men and women. In terms of mortality, it is interesting to note the decreasing proportion of deaths from infectious diseases in parallel to a growing share of deaths from circulatory diseases, respiratory diseases, and cancers.
Table 1 Descriptive statistics: men and women aged 30–89 in selected areas of southern Sweden, by time period
Estimation results
All-cause, non-preventable, and preventable mortality
reports key results for all-cause, non-preventable, and preventable mortality (full sets of estimates are presented in the supplementary material, Table A4). Looking first at men and all-cause mortality, the present-day income gradient in mortality is clearly visible in the coefficients for 1990–2014. The bottom 20 per cent of income earners (the reference category) show the highest mortality, and mortality is then progressively lower for each quintile in a more or less linear fashion. Comparing the poorest and the richest, mortality in the bottom quintile of the income distribution is more than twice as high as the mortality in the top quintile. The pattern is similar in the two preceding periods 1968–89 and 1950–67, but the gradients are not as linear in these periods as in the final period. Mortality differences by income grow over time (from hazard ratio (HR) = 0.624, p < 0.001 for men in the highest income quintile relative to the lowest in the period 1950–67 to HR = 0.408, p < 0.001 in the period 1990–2014). This pattern of growing income differentials in mortality is consistent with previous research for both Sweden and other Western countries, as mentioned earlier. However, for the period 1922–49, there is no clear gradient as seen in the later periods. Instead, the results suggest no mortality differences across income quintiles, due to lack of statistical significance and hazard ratios being close to one. Similarly, there is no evidence for income differences in mortality in the first period.
Table 2 Hazard ratios of all-cause, non-preventable, and preventable mortality by family income: men and women aged 30–89 in selected areas of southern Sweden, by time period
For men’s non-preventable mortality, in the last three periods the pattern is similar to that for all-cause mortality, with decreasing mortality hazards from the bottom to the top of the income distribution but the association being somewhat lower in magnitude. During the first half of the twentieth century, hazard ratios are all close to one (with one exception) and not statistically significant, indicating no mortality inequalities by income quintile. The pattern for preventable mortality also resembles very closely that described for all-cause mortality in terms of the magnitude of the coefficients. In the last three periods, there is a clear income gradient, which is not present in the earlier periods.
The pattern for all-cause mortality for women in the last three periods is similar to the pattern for men. Once again, in the first two periods, we do not find any mortality differences, with hazard ratios close to one across the different quintiles. When looking at non-preventable and preventable mortality, the results are similar to those for men for the entire time frame. The only exception is for preventable mortality in the first period, when we observe a difference in mortality between the two lowest quintiles. In the supplementary material (section 2) we discuss how these hazard ratios translate into survival patterns using estimates of median life expectancy.
Cause-specific mortality
displays hazard ratios for cause-specific mortality for men and women (full sets of estimates are presented in supplementary Table A5). For men, the mortality differences by income showing an advantage for higher income quintiles in the last three periods (1950–2014) are visible across all causes of death; circulatory diseases show a particularly clear mortality gradient in the last two periods. To some extent, this also holds for infectious diseases, although mortality is significantly lower in only the top two quintiles. For other cancers, the results still suggest a higher mortality in the lowest 20 per cent of the income distribution, but there is no clear gradient in the later periods. In the first two periods, there are no statistically significant differences in respiratory diseases, other cancers, external causes, or other causes. For infectious diseases, the mortality gradient is present to a certain extent as far back as the first period, although a statistically significant difference is found only between 1922 and 1949 for individuals in the top 20 per cent of the income distribution. Interestingly, the top quintile display higher mortality in circulatory diseases in the 1922–49 and 1905–21 periods (HR = 1.299, p < 0.01 and HR = 1.638, p < 0.05, respectively).
Table 3 Hazard ratios of cause-specific mortality by family income: men and women aged 30–89 in selected areas of southern Sweden, by time period
For women, there is a clear gradient for circulatory and respiratory diseases in the last three periods. For the remaining causes of death, the results suggest that mortality during the second half of the twentieth century is lower at the top of the income distribution and that the gradient becomes clearer when moving towards the last period. The coefficients tend to become gradually smaller and increase in precision from the bottom to the top quintiles. In the last period, it is only for infectious diseases that there are no significant mortality differences for women, although the point estimates suggest a decreasing risk when moving towards the top of the income distribution. In the first two periods, there are no consistent income differentials in mortality across cause-of-death groups. Differently from men, for circulatory diseases in the first period, the findings indicate that women with incomes above the lowest quintile, particularly those in the centre of the income distribution, experience lower mortality (HR = 0.619, p < 0.05).
Income–mortality associations adjusted for social class
To obtain a better understanding of the relationship between income and mortality, we estimated a set of models in which we adjusted for family social class (see ). We used the occupation of the person with the highest occupational status in the family (in the order non-manual, farmer, manual). For men, all-cause, non-preventable, and preventable mortality results by income remain unchanged. In the last period, there is an independent negative association between social class and mortality in both all-cause and preventable mortality. In the period 1968–89, being in the non-manual group is not associated with a lower hazard for any of the causes of death. Perhaps surprisingly, in the third period (1950–67), the highest social class (non-manual) shows elevated all-cause, non-preventable, and preventable mortality compared with the manual group. Such a positive association is also present in the first two periods but is statistically significant only for preventable causes.
Table 4 Hazard ratios of all-cause, non-preventable, and preventable mortality by family income and social class: men and women aged 30–89 in selected areas of southern Sweden, by time period
Like for men, the income gradient for women does not change for any of the mortality outcomes (all-cause, non-preventable, and preventable mortality) when adjusted for social class. However, the pattern for social class is different from that of men. For both all-cause and preventable mortality, the estimates suggest that women in the highest social class are already experiencing lower mortality from the late 1960s onwards. Before this period, we do not find any association between social class and mortality. Overall, these results suggest that mortality differentials by income are quite independent from social class.
Table A6 (supplementary material) reports equivalent estimates for cause-specific mortality. Overall, the income–mortality associations (shown in ) are not affected by adjusting for social class. Exceptions exist mainly for men, for whom the advantage of the top income quintile in infectious diseases is not statistically significant in the second period. In addition, the positive association in the earlier periods between social class and mortality noted before seems to be particularly strong for circulatory diseases. The higher circulatory disease mortality in the top income quintile that was found for the first two periods is now only statistically significant in the first period. Instead, the higher circulatory disease mortality in higher social classes holds up until the fourth period, even though the magnitude of the coefficient clearly decreases from the third to the fourth period. The higher mortality in the non-manual group in the third period is also evident for respiratory diseases and other cancers.
Sensitivity analysis
We carried out a set of sensitivity analyses to test the robustness of our results to different specifications. All results are reported in the supplementary material. Tables A7 and A8 show the results for models run separately by age group (30–59 and 60–89 years) for men and women, respectively. The income gradient emerges earlier for younger men than for older men. For all-cause and preventable mortality in the younger age group, the gradient is already pronounced in the period 1922–49. For older men, there are reversed differences (higher mortality for higher-income groups) in all-cause and preventable mortality in the second period, which change in the following period to a negative association and then become more marked with time. Women do not show strong age differences in terms of the emergence of the income gradient in mortality. The analyses divided by age group show similar results to the main analysis.
As a further robustness check, we analysed income differences in mortality using individual income instead of family income. This meant that, for married people, instead of considering the sum of the two spouses’ incomes, we used their own income. The results are reported in Table A9 and show a similar pattern to that for family income. Next, Table A10 reports the results for models restricted to currently married individuals only; it suggests that for men, the income gradient is even stronger in the second period and already showing lower mortality at the centre of the income distribution. Married women instead show, in terms of coefficients, a similar pattern to that described in the main analysis but with less statistical significance.
Moreover, we estimated models where imputed income was not used (Table A11) and models leaving individuals with missing income information as missing (not setting them to ‘1’ as in the main analysis) (Table A12). We also tested if the results changed when assigning an income of 25 per cent of the threshold for income reporting to those below it (instead of 50 per cent; see supplementary material, section 1) or when assigning them an imputed income using the alternative approach described in section 1 of the supplementary material (see Tables A13 and A14, respectively). The results are similar to the main analysis, thus demonstrating that the absence of an income gradient in mortality before 1950 is not related to the way we assigned income below the reporting threshold. Even when assuming that all individuals without a recorded income have a similar income to other individuals of the same age and occupation in the same year, the results (Table A14) are the same.
Additionally, Table A15 shows the pattern for missing and ill-defined causes of death for the first three periods. The pattern is similar to the overall one in that there is no sign of an emergence of a gradient until after 1950, except for men in the first period.
Since in- and out-migration could potentially bias the results, in Tables A16 and A17 we report sensitivity analyses for all-cause mortality of individuals who died outside the area under study. More specifically, in Table A16 we used a sample for the first three periods in which people who had ever lived in the SEDD area and died elsewhere in the country were probabilistically linked using personal details (i.e. names, surnames, birthdate, birthplace) to the Swedish Death Index (a data set of all deaths that occurred in Sweden between 1860 and 2017 [Sveriges Släktforskarförbund Citation2019]). For the post-1968 period, instead we tested the impact of migration by analysing all-cause mortality of everyone who had ever lived in the area under study (as well as their spouses, parents, grandparents, children, and siblings) but had died outside the SEDD area (Table A17). The results are similar to the main findings; this shows that the income–mortality association is not driven by sample selection related to migration.
We also explored whether the pattern changes when using absolute income instead of relative income (Table A18) and if the results change after calculating quintiles by sex, year, and age instead of by sex and year (Table A19). In Table A20, we show how the results change when slightly adjusting the periodization. Table A21 reports results of models further adjusted by household composition, measured by the number of children in the family. Finally, in Table A22 we check whether using actual income in old age (instead of income at age 50–59) changes the results. None of these sensitivity tests highlights a pattern that would contrast with the one in the main analyses.
Conclusion
In this study, we analysed income differences in all-cause and cause-specific adult mortality for men and women for a period spanning 1905–2014. The aim was to establish the timing of the emergence of the income gradient in mortality that has consistently been found in developed countries in the period since 1970.
Our results showed that the present-day income gradient emerged only in the period after the Second World War, for both men and women, and it did so for all cause-of-death groups almost simultaneously. More specifically, for both men and women, we observed an income gradient in all-cause and cause-specific mortality from 1950 onwards. In the first half of the twentieth century, we found no evidence for an income gradient. The only exceptions were that on the one hand, men at the top of the income distribution showed an advantage in mortality from infectious diseases as early as the 1920s; on the other hand, men in this highest quintile experienced higher circulatory disease mortality than those in the lowest quintile during the first half of the twentieth century, particularly in the first two decades. Further detailed analysis showed that the association between income and mortality was independent of social class. Taken together, the results considering both income and social class confirmed that across cause-of-death groups, the income gradient in mortality has been present since the 1950s, and the results showed a pattern by social class in line with previous findings (Debiasi and Dribe Citation2020). Additionally, when splitting the analyses by age group, we found that the income gradient at working ages emerged earlier in time than the gradient at older ages.
Our findings for the two most recent periods are consistent with those from previous research relating to Sweden as a whole, for both all-cause mortality and cause-specific mortality (Hederos et al. Citation2018). Income inequalities steadily increased, particularly in the last period, as did mortality differentials. On the one hand, previous studies have shown a relationship between income inequalities at a macro level and mortality (see Smith Citation1996). One of the explanations for this link could be related to the detrimental health effects of inequalities in relative terms; that is, the psychological adverse effect of relative deprivation from belonging to a lower social stratum in comparison to those higher up the ladder (Wilkinson Citation1994). On the other hand, recent studies have maintained that the association is confounded by other country-level factors (Hu et al. Citation2015). Indeed, a Swedish study found mortality to be negatively associated with absolute income but not significantly related to relative income or income inequality (Gerdtham and Johannesson Citation2004). Interestingly, when extending the time frame of the analyses further back in time in our study, the results showed that the income gradient emerged around the 1950s, a period in which, income inequalities were stable after narrowing down. This could point to the role of absolute income levels (as opposed to changes in macro-level income inequalities) as an alternative explanation for mortality differences. Furthermore, the income gradient emerged earlier for infectious diseases; this could be connected to access to better housing conditions, which would have reduced environmental exposures.
The lack of gradient in the earlier periods could be explained by richer individuals not exploiting the entire array of protective factors. In particular, higher income in the early twentieth century has been linked to higher alcohol consumption and tobacco smoking as well as to a more sedentary lifestyle. Indeed, we observed higher circulatory disease mortality for men in the top income quintile in the first period. These patterns are similar to those found when studying mortality differentials by social class in the first half of the twentieth century, for both the same area and for Sweden as a whole (Dribe and Eriksson Citation2018; Bengtsson et al. Citation2020; Debiasi and Dribe Citation2020).
The fact that the older age group displayed a somewhat reversed gradient in all-cause and preventable mortality in the second period could be further evidence of the importance of lifestyle factors. In the early twentieth century, higher-income groups were more exposed to unhealthy lifestyles, and this was more so for the older age group than for the younger. In other words, among richer individuals, those in the younger age group could exploit their knowledge about health risks and apply it in different behaviours at an earlier stage of life, while for older people, it was too late to change bad habits and the consequences of having been exposed to them earlier in their adult life.
Given that the income differences in mortality started to emerge around the time when the welfare state began to expand, we might be tempted to conclude that the welfare system failed in reducing health inequalities. However, it would be inaccurate to draw such a conclusion. Income inequalities in mortality are observed in many countries with wide differences in their health provision systems. As mentioned earlier, our findings seem to indicate that income is as an indicator of status, which is in turn related to lifestyle and psychosocial factors that have become more important explanations over time.
As already stated, establishing a causal relationship between income and mortality was not the aim of this study. However, we can observe that if reverse causality were behind the patterns described here, it would mean that health has had an increasingly larger effect on income over time. This seems unlikely, given the large expansion in welfare policies in terms of sick leave and disability that took place during the time when the income gradient in mortality emerged. A further limitation of our study was that the timing we described was sensitive to the periodization used, so we could provide only an approximate assessment, which is why we described and discussed our results in terms of broad periods rather than specific years. Finally, the data available did not include information about individuals’ wealth and therefore it was not possible to consider changes in the stock of wealth alongside income. Nevertheless, as explained earlier, our exposure (i.e. total income) also included income from capital, which partly captured changes in wealth.
Taken together, our findings highlight the multidimensionality of SES and the fact that different measures cannot be used interchangeably, stressing the importance of considering several indicators. At the same time, they lead to the important conclusion that the income gradient occurred only relatively recently and hence that income has not always been an important determinant of health and mortality. This conclusion is based on a regional sample in Sweden, and even though our findings for the period after 1970 are completely consistent with other research on Sweden and other developed countries, it is impossible to be certain that the findings will be equally valid outside the study area and for earlier periods. Therefore, it will be important for further research to investigate the historical income–mortality relationships in other contexts.
Supplemental Material
Download PDF (2.1 MB)Disclosure statement
No potential conflict of interest was reported by the authors.
Notes
1 Enrico Debiasi, Martin Dribe, and Gabriel Brea Martinez are all based in the Centre for Economic Demography and Department of Economic History, Lund University, Sweden. Please direct all correspondence to Enrico Debiasi, P.O. Box 7080, SE-220 07 Lund, Sweden; or by E-mail: [email protected].
2 Acknowledgements: This work was supported by the LONGPOP project ‘Methodologies and data mining techniques for the analysis of big data based on longitudinal population and epidemiological registers’, funded by the European Union’s Horizon 2020 research and innovation programme under the Marie Skłodowska-Curie Innovative Training Networks [grant agreement No 676060]. Disclaimer: This document reflects only the authors’ view and the Agency [EU] is not responsible for any use that may be made of the information it contains. The study is also part of the research programme ‘Landskrona Population Study’, funded by the Swedish Foundation for Humanities and Social Sciences (RJ). We thank seminar participants at the Swedish Institute for Social Research (Stockholm University) and the University of Minnesota Demography and Aging Seminar Series; the Departments of Economic History and Political Science, Lund University; and especially Tommy Bengtsson, Ingrid van Dijk, and Luciana Quaranta for helpful comments and suggestions.
References
- Adler, Nancy E. and Judith Stewart. 2010. Health disparities across the lifespan: Meaning, methods, and mechanisms, Annals of the New York Academy of Sciences 1186(1): 5–23. https://doi.org/10.1111/j.1749-6632.2009.05337.x
- AMIEHS: Avoidable Mortality in the European Union. 2011. Available: http://amiehs.lshtm.ac.uk/
- Austin, Peter C., Douglas Lee, and Jason Fine. 2016. Introduction to the analysis of survival data in the presence of competing risks, Circulation 133(6): 601–609. https://doi.org/10.1161/CIRCULATIONAHA.115.017719
- Barker, David. 1998. In utero programming of chronic disease, Clinical Science 95(2): 115–128. https://doi.org/10.1042/cs0950115
- Bengtsson, Tommy and Göran Broström. 2009. Do conditions in early life affect old-age mortality directly and indirectly? Evidence from 19th-century rural Sweden, Social Science & Medicine 68(9): 1583–1590. https://doi.org/10.1016/j.socscimed.2009.02.020
- Bengtsson, Tommy and Martin Dribe. 2011. The late emergence of socioeconomic mortality differentials: A micro-level study of adult mortality in southern Sweden 1815–1968, Explorations in Economic History 48(3): 389–400. https://doi.org/10.1016/j.eeh.2011.05.005
- Bengtsson, Tommy and Martin Dribe. 2022. The long road to health and prosperity, southern Sweden, 1765-2015. Research contributions from the Scanian Economic-Demographic Database (SEDD), Historical Life Course Studies 11: 74–96. https://doi.org/10.51964/hlcs10941
- Bengtsson, Tommy, Martin Dribe, and Jonas Helgertz. 2020. When did the health gradient emerge? Social class and adult mortality in southern Sweden, 1813–2015, Demography 57(3): 953–977. https://doi.org/10.1007/s13524-020-00877-5
- Bengtsson, Tommy, Martin Dribe, Luciana Quaranta, and Patrick Svensson. 2020. The Scanian Economic Demographic Database. Version 7.1 (Machine-readable database). Lund: Lund University, Centre for Economic Demography.
- Bengtsson, Tommy and Martin Lindström. 2000. Childhood misery and disease in later life: The effects on mortality in old age of hazards experienced in early life, southern Sweden, 1760-1894, Population Studies 54(3): 263–277. https://doi.org/10.1080/713779096
- Bengtsson, Tommy, Christer Lundh, and Kirk Scott. 2005. From boom to bust. The economic integration of immigrants in post war Sweden, in Klaus F. Zimmermann (ed), European Migration: What Do We Know? Oxford: OUP, pp. 15–58.
- Blanden, Jo. 2013. Cross-country rankings in intergenerational mobility: A comparison of approaches from economics and sociology, Journal of Economic Surveys 27(1): 38–73. https://doi.org/10.1111/j.1467-6419.2011.00690.x
- Breen, Richard, Carina Mood, and Jan Jonsson. 2016. How much scope for a mobility paradox? The relationship between social and income mobility in Sweden, Sociological Science 3: 39–60. https://doi.org/10.15195/v3.a3
- Case Anne and Angus Deaton. 2020. Deaths of Despair and the Future of Capitalism. https://press.princeton.edu/books/hardcover/9780691190785/deaths-of-despair-and-the-future-of-capitalism
- Case, Anne and Christina Paxson. 2009. Early life health and cognitive function in old age, American Economic Review 99(2): 104–109. https://doi.org/10.1257/aer.99.2.104
- Cavelaars, A. E. J. M., A. E. Kunst, J. J. M. Geurts, R. Crialesi, L. Grötvedt, U. Helmert, E. Lahelma, et al. 2000. Educational differences in smoking: International comparison, BMJ : British Medical Journal 320(7242): 1102–1107. https://doi.org/10.1136/bmj.320.7242.1102
- Cesarini, David, Erik Lindqvist, Robert Östling, and Björn Wallace. 2016. Wealth, health, and child development: Evidence from administrative data on Swedish lottery players, The Quarterly Journal of Economics 131(2): 687–738. https://doi.org/10.1093/qje/qjw001
- Chetty, Raj, Michael Stepner, Sarah Abraham, Shelby Lin, Benjamin Scuderi, Nicholas Turner, Augustin Bergeron, et al. 2016. The association between income and life expectancy in the United States, 2001–2014, JAMA 315(16): 1750–1766. https://doi.org/10.1001/jama.2016.4226
- Currie, Janet. 2009. Healthy, wealthy, and wise: Socioeconomic status, poor health in childhood, and human capital development, Journal of Economic Literature 47(1): 87–122. https://doi.org/10.1257/jel.47.1.87
- Cutler, David and Adriana Lleras-Muney. 2008. Education and health: Evaluating theories and evidence, in Robert F. Schoeni, James S. House, George A. Kaplan and Harold Pollack (eds), Making Americans Healthier. Russell Sage Foundation; JSTOR, pp. 29–60. https://www.jstor.org/stable/10.77589781610444873.6
- Cutler, David, Adriana Lleras-Muney, and Tom Vogl. 2012. Socioeconomic status and health: Dimensions and mechanisms, in M. Gleid and P. Smith (eds), The Oxford Handbook of Health Economics. New York: Oxford University Press, pp. 124–163.
- Deaton, Angus. 2003. Health, inequality, and economic development, Journal of Economic Literature 46: 113–158.
- Deaton, Angus. 2016. On death and money: History, facts, and explanations, JAMA 315(16): 1703–1705. https://doi.org/10.1001/jama.2016.4072
- Debiasi, Enrico and Martin Dribe. 2020. SES inequalities in cause-specific adult mortality: A study of the long-term trends using longitudinal individual data for Sweden (1813–2014), European Journal of Epidemiology 35(11): 1043–1056. https://doi.org/10.1007/s10654-020-00685-6
- Dribe Martin and Björn Eriksson. 2018. Socioeconomic Status and Adult Life Expectancy in Early 20th-Century Sweden: Evidence from Full-Count Micro Census Data. Lund Papers in Economic Demography 2018:1. Lund University: Centre for Economic Demography. https://www.ed.lu.se/media/ed/papers/working_papers/LPED%202018%201.pdf
- Dribe, Martin, Jonas Helgertz, and Bart van de Putte. 2015. Did social mobility increase during the industrialization process? A micro-level study of a transforming community in southern Sweden 1828–1968, Research in Social Stratification and Mobility 41: 25–39. https://doi.org/10.1016/j.rssm.2015.04.005
- Dribe, Martin and Luciana Quaranta. 2020. The Scanian Economic-Demographic Database (SEDD), Historical Life Course Studies 11(2). https://hdl.handle.net/10622/23526343-2020-0008
- Edebalk Per Gunnar. 1996. Välfärdsstaten träder fram : Svensk socialförsäkring 1884-1955 [The Wefare State Emerges: Social Insurance in Sweden, 1884-1955], in Lund Studies in Social Welfare: Vol. XII. Arkiv. http://lup.lub.lu.se/record/da9ab2bd-4e79-4b5d-968b-6b2dcb642aca
- Edvinsson, Sören and Göran Broström. 2012. Old age, health and social inequality: Exploring the social patterns of mortality in 19th century northern Sweden, Demographic Research 26: 633–660. https://doi.org/10.4054/DemRes.2012.26.23
- Edvinsson, Sören and Marie Lindkvist. 2011. Wealth and health in 19th century Sweden. A study of social differences in adult mortality in the Sundsvall region, Explorations in Economic History 48(3): 376–388. https://doi.org/10.1016/j.eeh.2011.05.007
- Elo, Irma. 2009. Social class differentials in health and mortality: Patterns and explanations in comparative perspective, Annual Review of Sociology 35(1): 553–572. https://doi.org/10.1146/annurev-soc-070308-115929
- Elo, Irma and Samuel Preston. 1992. Effects of early-life conditions on adult mortality: A review, Population Index 58(2): 186–212. https://doi.org/10.2307/3644718
- Ericsson, Malin, Nancy Pedersen, Anna Johansson, Stefan Fors, and Anna K. Dahl Aslan. 2019. Life-course socioeconomic differences and social mobility in preventable and non-preventable mortality: A study of Swedish twins, International Journal of Epidemiology 48(5): 1701–1709. https://doi.org/10.1093/ije/dyz042
- Erikson, Robert and John Goldthorpe. 2010. Has social mobility in Britain decreased? Reconciling divergent findings on income and class mobility, The British Journal of Sociology 61(2): 211–230. https://doi.org/10.1111/j.1468-4446.2010.01310.x
- Fogel, Robert. 2004. The Escape from Hunger and Premature Death, 1700–2100: Europe, America, and the Third World. Cambridge: Cambridge University Press.
- Gerdtham, Ulf-G. and Magnus Johannesson. 2004. Absolute income, relative income, income inequality, and mortality, Journal of Human Resources XXXIX(1): 228–247. https://doi.org/10.3368/jhr.XXXIX.1.228
- Geyer, Siegfried, Örjan Hemström, Richard Peter, and Denny Vågerö. 2006. Education, income, and occupational class cannot be used interchangeably in social epidemiology. Empirical evidence against a common practice, Journal of Epidemiology & Community Health 60(9): 804–810. https://doi.org/10.1136/jech.2005.041319
- Hederos, Karin, Markus Jäntti, Lena Lindahl, and Jenny Torssander. 2018. Trends in life expectancy by income and the role of specific causes of death, Economica 85(339): 606–625. https://doi.org/10.1111/ecca.12224
- Helgertz Jonas, Tommy Bengtsson, and Martin Dribe. 2020. The Scanian Economic Demographic Database’s (SEDD) - Income Data. Lund Papers in Economic Demography 2020:6. Lund University: Centre for Economic Demography. https://www.ed.lu.se/media/ed/papers/working_papers/LPED_2020_6.pdf
- Helgertz, Jonas and Martin Önnerfors. 2019. Public water and sewerage investments and the urban mortality decline in Sweden 1875–1930, The History of the Family 24(2): 307–338. https://doi.org/10.1080/1081602X.2018.1558411
- Hiltunen, Maria and Sören Edvinsson. 2018. Classifying Literate Cause-of-Death Information Originating from Swedish Historical Parish Registers.
- Hirdman, Yvonne. 1983. Magfrågan: mat som mål och medel : Stockholm 1870-1920 [The issue of the stomach. Food as a means and an end. Stockholm 1870-1920]. Stockholm: Rabén and Sjögren.
- Hu, Yannan, Frank van Lenthe, and Johan Mackenbach. 2015. Income inequality, life expectancy and cause-specific mortality in 43 European countries, 1987–2008: A fixed effects study, European Journal of Epidemiology 30(8): 615–625. https://doi.org/10.1007/s10654-015-0066-x
- Kawachi, Ichiro, Nancy Adler, and William Dow. 2010. Money, schooling, and health: Mechanisms and causal evidence, Annals of the New York Academy of Sciences 1186(1): 56–68. https://doi.org/10.1111/j.1749-6632.2009.05340.x
- Kinge, Jonas Minet, Heibø Modalsli, Simon Øverland, Kristian Gjessing Håkon, Christophersen Tollånes, Ann Kristin Knudsen, Vegard Skirbekk, et al. 2019. Association of household income with life expectancy and cause-specific mortality in Norway, 2005-2015, JAMA 321(19): 1916–1925. https://doi.org/10.1001/jama.2019.4329
- Kleinke, Kristian. 2017. Multiple imputation under violated distributional assumptions: A systematic evaluation of the assumed robustness of predictive mean matching, Journal of Educational and Behavioral Statistics 42(4): 371–404. https://doi.org/10.3102/1076998616687084
- Kondo, Naoki, Mikael Rostila, and Yngwe Monica Åberg. 2014. Rising inequality in mortality among working-age men and women in Sweden: A national registry-based repeated cohort study, 1990–2007, Journal of Epidemiology and Community Health 68(12): 1145–1150. https://doi.org/10.1136/jech-2013-203619
- Lau, Bryan, Stephen Cole, and Stephen Gange. 2009. Competing risk regression models for epidemiologic data, American Journal of Epidemiology 170(2): 244–256. https://doi.org/10.1093/aje/kwp107
- Lazuka Volha. 2017. Defeating Disease: Lasting Effects of Public Health and Medical Breakthroughs between 1880 and 1945 on Health and Income in Sweden [Lund University]. https://portal.research.lu.se/portal/en/publications/defeating-disease(1cba7526-042f-43fe-9005-6f6bc8cbf862).html
- Lindahl, Mikael. 2005. Estimating the effect of income on health and mortality using lottery prizes as an exogenous source of variation in income, Journal of Human Resources XL(1): 144–168. https://doi.org/10.3368/jhr.XL.1.144
- Lundborg, Petter, Carl Hampus Lyttkens, and Paul Nystedt. 2016. The effect of schooling on mortality: New evidence from 50,000 Swedish twins, Demography 53(4): 1135–1168. https://doi.org/10.1007/s13524-016-0489-3
- Lundh, Christer. 2013. Was there an urban–rural consumption gap? The standard of living of workers in southern Sweden, 1914–1920, Scandinavian Economic History Review 61(3): 233–258. https://doi.org/10.1080/03585522.2013.794162
- Mackenbach, Johan. 2019. Health Inequalities: Persistence and Change in European Welfare States. Oxford University Press.
- Mackenbach, Johan, Ivana Kulhánová, Barbara Artnik, Bopp Matthias, Borrell Carme, Clemens Tom, Costa Giuseppe, et al. 2016. Changes in mortality inequalities over two decades: Register based study of European countries, BMJ 353. https://doi.org/10.1136/bmj.i1732
- Magnusson, Lars. 2000. An Economic History of Sweden. London: Routledge.
- Marmot, Michael. 2004. Status Syndrome: How Your Social Standing Directly Affects Your Health. London: A & C Black.
- McKeown, Thomas. 1976. The Modern Rise of Population. London: Edward Arnold.
- Montez, Jennifer Karas and Esther Friedman. 2015. Educational attainment and adult health: Under what conditions is the association causal?, Social Science & Medicine 127: 1–7. https://doi.org/10.1016/j.socscimed.2014.12.029
- Mood, Carina. 2017. More than money: Social class, income, and the intergenerational persistence of advantage, Sociological Science 4: 263–287. https://doi.org/10.15195/v4.a12
- Morris, Tim, Ian White, and Patrick Royston. 2014. Tuning multiple imputation by predictive mean matching and local residual draws, BMC Medical Research Methodology 14(1): 75. https://doi.org/10.1186/1471-2288-14-75
- Nordlund, A., 2005. Tobaksrökning och hälsa i Sverige under 1900-talet [Tobacco smoking and health in Sweden during the 20th century], in Jan Sundin et al. (eds), Svenska folkets hälsa i historiskt perspektiv. Stockholm: Statens folkhälsoinstitut. https://www.bibliotekfh.se/work/svenska-folkets-hälsa-i-historiskt-perspektiv?id=0a387cc8-d2a4-f815-f58a-ccc4258e33bdandcatalogId=ef88079b-d2c5-4b13-a326-6e631b2a2483andworkId=4bc63bc8-91fd-498d-80c1-a6c3013f9f88
- Norström, Thor. 1987. The abolition of the Swedish alcohol rationing system: Effects on consumption distribution and cirrhosis mortality, Addiction 82(6): 633–641. https://doi.org/10.1111/j.1360-0443.1987.tb01525.x
- Quaranta, Luciana. 2014. Early life effects across the life course: The impact of individually defined exogenous measures of disease exposure on mortality by sex in 19th- and 20th-century southern Sweden, Social Science & Medicine 119 (Online May 15, 2014): 266–273. https://doi.org/10.1016/j.socscimed.2014.04.007
- Quaranta, Luciana. 2015. Using the intermediate data structure (IDS) to construct files for statistical analysis, Historical Life Course Studies 2: 86–107. https://doi.org/10.51964/hlcs9360
- Quaranta, Luciana. 2016. STATA programs for using the intermediate data structure (IDS) to construct files for statistical analysis, Historical Life Course Studies 3: 1–19. https://doi.org/10.51964/hlcs9350
- Roine, Jesper and Daniel Waldenström. 2008. The evolution of top incomes in an egalitarian society: Sweden, 1903–2004, Journal of Public Economics 92(1): 366–387. https://doi.org/10.1016/j.jpubeco.2007.06.012
- SCB. 2019. Life Expectancy 1751–2019. https://www.scb.se/en/finding-statistics/statistics-by-subject-area/population/population-composition/population-statistics/pong/tables-and-graphs/yearly-statistics–the-whole-country/life-expectancy/
- SCB. 2020. CPI Sweden, Historical Numbers 1830-. https://www.scb.se/en/finding-statistics/statistics-by-subject-area/prices-and-consumption/consumer-price-index/consumer-price-index-cpi/pong/tables-and-graphs/consumer-price-index-cpi/cpi-historical-numbers-1830/
- Schön, Lennart. 2010. Sweden’s Road to Modernity: An Economic History. 1st ed. Stockholm: SNS förlag.
- Smith, George Davey. 1996. Income inequality and mortality: Why are they related?, BMJ 312(7037): 987–988. https://doi.org/10.1136/bmj.312.7037.987
- Smith, James. 1999. Healthy bodies and thick wallets: The dual relation between health and economic status, The Journal of Economic Perspectives : A Journal of the American Economic Association 13(2): 144–166.
- Smith, James. 2004. Unraveling the SES: Health connection, Population and Development Review 30: 108–132. JSTOR.
- Stanfors, Maria. 2014. Women in a changing economy: The misleading tale of participation rates in a historical perspective, The History of the Family 19(4): 513–536. https://doi.org/10.1080/1081602X.2014.909737
- Steingrímsdóttir, Ólöf Anna, Øyvind Næss, Moe Joakim Oliu, Else-Karin Grøholt, Thelle Dag Steinar, Bjørn Heine Strand, and Bævre Kåre. 2012. Trends in life expectancy by education in Norway 1961–2009, European Journal of Epidemiology 27(3): 163–171. https://doi.org/10.1007/s10654-012-9663-0
- Sundin, Jan and Sam Willner. 2007. Social Change and Health in Sweden : 250 Years of Politics and Practice. Swedish National Institute of Public Health. http://urn.kb.se/resolve?urn=urn:nbn:se:liu:diva-11323
- Sveriges Släktforskarförbund. 2019. Sveriges dödbok version 7, 1860-2017 [The Swedish Death Index, version 7, 1860-2017]. https://www.rotterbokhandeln.se/product.html/sveriges-dodbok-7?category_id=10
- Szreter, Simon. 1988. The importance of social intervention in Britain's mortality decline c.1850–1914: A re-interpretation of the role of public health, Social History of Medicine 1(1): 1–38. https://doi.org/10.1093/shm/1.1.1
- Tarkiainen, Lasse, Pekka Martikainen, Mikko Laaksonen, and Tapani Valkonen. 2012. Trends in life expectancy by income from 1988 to 2007: Decomposition by age and cause of death, Journal of Epidemiology and Community Health 66(7): 573–578. https://doi.org/10.1136/jech.2010.123182
- Torssander, Jenny and Robert Erikson. 2010. Stratification and mortality—A comparison of education, class, status, and income, European Sociological Review 26(4): 465–474. https://doi.org/10.1093/esr/jcp034
- van Doorslaer, Eddy, Adam Wagstaff, Hattem van der Burg, Christiansen Terkel, De Graeve Diana, Duchesne Inge, Gerdtham Ulf-G, et al. 2000. Equity in the delivery of health care in Europe and the US, Journal of Health Economics 19(5): 553–583. https://doi.org/10.1016/S0167-6296(00)00050-3
- Wamala, Sarah, Tony Blakely, and June Atkinson. 2006. Trends in absolute socioeconomic inequalities in mortality in Sweden and New Zealand. A 20-year gender perspective, BMC Public Health 6: 164. https://doi.org/10.1186/1471-2458-6-164
- Wilkinson, Richard. 1994. Unfair Shares: The Effects of Widening Income Differences on the Welfare of the Young. London: Barnardo’s.