ABSTRACT
Background
Recent studies have identified monocyte to high-density lipoprotein ratio (MHR) as a simple, practical surrogate of atherosclerosis. Considering atherosclerosis is a major mechanism of coronary heart disease (CHD). The present study aims to evaluate the association between MHR and the prevalence of CHD.
Methods and results
The present cross-sectional work included 6442 participants (mean age: 59.57 years, 60.2% females), all of them were included from rural areas of northern China between October 2019 to April 2020. MHR was acquired as monocytes count divided by high-density lipoprotein concentration. Prevalent CHD researched 3.14%. After adjustment of sex, age, current drinking and smoking, BMI, WC, diabetes, hypertension, LDL-C, TG, eGFR, lipid-lowering therapy and cerebrovascular disease history, each standard deviation increase of MHR cast a 39.5% additional CHD risk. Furthermore, the top quartile of MHR had an additional 89.0% CHD risk than the bottom quartile. Besides, smooth curve fitting revealed a linear pattern of the association. Additionally, the stratified evaluation showed a robust correlation among the subgroups divided by CHD risk factors. Finally, area under the curve demonstrated an advancement when including MHR into common CHD risk factors (0.744 vs 0.761, p < 0.001). Consistently, reclassification analysis indicated the improvement from MHR (all P = 0.003).
Conclusion
Our work suggests the robust and linear relationship between MHR and the prevalent CHD in a general population, providing epidemiological evidence for laboratory studies. More importantly, the findings implicate the efficacy of MHR to be a potential indicator to identify the prevalent CHD.
Introduction
Coronary heart disease (CHD) is a leading cause of disease burden worldwide [Citation1]. In 2010, the prevalence of CHD in America reached 6.4% [Citation2]. In China, previous investigations have also displayed the prevalence of CHD is over 5.0% [Citation3,Citation4]. Due to this grim situation, a method to advance and simplify CHD identification is of great importance to mitigate the healthcare and economic burden.
Atherosclerosis is the core pathophysiological process during the genesis of CHD [Citation5,Citation6]. Development of atheroma results in remodeling of coronary arteries, leading to stiffness and narrowing of the vascular lumen and a limited ability of coronary arteries to supply blood [Citation7,Citation8]. Therefore, when myocardial demand for oxygen rises abruptly, symptoms like angina may occur [Citation5,Citation9]. More seriously, some atherosclerotic plaques are prone to rupture and trigger the thrombotic process [Citation10]. Consequently, the rapidly formed thrombus may obstruct the affected coronary arteries, initiating the onset of acute coronary syndrome [Citation6]. Accordingly, monitoring of atherosclerosis seems to be of prime importance to improve the identification of CHD. However, current imaging techniques used to assess atherosclerosis are complex, expensive, and time-consuming. Hence, a simple, rapid, and cost-effective marker will have greater value in identifying CHD.
Inflammation and dyslipidemia are two interplayed characteristics of atherosclerosis [Citation11,Citation12]. In the atherosclerotic process, monocyte is a major origin of the pro-inflammatory factors [Citation13]. Monocytes migrate into the arterial wall and then transform into macrophages [Citation11]. These macrophages engulf oxidized low-density lipoprotein cholesterol (LDL-C) and derive into lipid-laden foam cells, which is a pivotal element of the atherosclerotic core [Citation14]. Unlike monocytes’ pro-atherosclerotic effect, high-density lipoprotein cholesterol (HDL-C) slows down the atherosclerotic process. Firstly, HDL-C promotes reverse cholesterol transportation to minimize lipid accumulation in arteries [Citation15,Citation16]. Secondly, HDL-C inhibits LDL-C oxidation and prevents the recruitment of monocytes into the arterial wall, thereby neutralizing the pro-oxidant and pro-inflammatory impact of monocytes [Citation17,Citation18]. Lastly, HDL-C suppresses monocyte production, inhibiting the inflammatory process of atherosclerosis [Citation19].
Recently, ‘Monocyte to high-density lipoprotein cholesterol ratio (MHR)’ has been posited for rapid assessment of the atherosclerotic severity through evaluating dyslipidemia and inflammation [Citation20]. Researchers have revealed its value in the prediction of multiple atherosclerotic diseases [Citation21–23]. However, although CHD has a close association with atherosclerosis, the evidence of the correlation between MHR and prevalent CHD in a general and representative population is still limited. Therefore, the present research aimed to explore the association between MHR and the prevalent CHD and exam the potential of MHR to advance the identification of CHD in a general Chinese population.
Methods
Study population
This present study originated from a cross-sectional survey performed in rural areas of northern China. The survey started in October 2019 and ended in April 2020. To ensure the samples were representative of the local population, the surveyed followed a geologically stratified, multi-stage, and clustered random sampling method. Randomly selected 17 villages from 2 cities (Linyi and Binzhou) of Shandong province were included. All permanent residents ≥ 40 years old (n = 7972) were recognized as eligible participants. Subjects with cancer, mental disorders or pregnancy were excluded from the present work. Six thousand four hundred and seventy-one (81.2%) subjects finished the survey. In our work, we further excluded 29 subjects because of missed data of co-variables and enrolled 6442 individuals into statistical analyses (). The study protocol was approved by the central ethics committee of the Second Affiliated Hospital of Shandong University of TCM and conformed to the ethical guidelines of the 1975 Declaration of Helsinki. Written consents were provided by all participants. Subjects’ proxies provided written informed consents for them if they were disabled.
Data collection and measurement
A team including cardiologists, neurologists, and specialists trained in preventing and controlling chronic disease was employed to collect the data. During a single clinical visit, a face-to-face interview and a standard questionnaire were performed. Prior to any data collection, all the team members underwent epidemiological training followed by a final exam. Team members were permitted to conduct the questionnaire only after passing the exam. Under the certification of the health commission of Linyi and Binzhou, the clinics in the villages were blueprinted for primary medical care. These clinics were equipped with warm and large rooms which are eligible for the conduction of epidemiological studies. During the process of data collection and cleaning, a double-entry method was conducted for quality control to improve the accuracy and authenticity of the data.
The standardized questionnaire collected demographic information, including sex, age, socioeconomic conditions (annual family income, education) and lifestyle (exercise, drinking and smoking conditions). Moderate to heavy exercise or working intensity (sweating at cool environment) ≥3 days a week was determined as frequent exercise. Concomitant diseases like diabetes mellitus (DM), hypertension, and cerebrovascular diseases history were recorded based on participants’ self-reports.
Measuring of anthropometric parameters was conducted when the subjects wear light clothes without shoes. The standard height was recorded to the nearest 0.1 cm by calibrated stadiometer when participants held in a standing position. In the meantime, a calibrated scale was utilized to record the standard weight to the nearest 0.1 kg.
Standardized electronic sphygmomanometers (HEM-907; Omron, Kyoto, Japan) was employed to measure blood pressure. The measuring process was conducted in a quiet and warm room. Subjects were required to avoid exercise, smoking, and caffeine intake for more than 30 minutes before the measurement. Three consecutive measurements with 2 min interval were recorded for every participant. After that, their mean value was included in the statistical analyses.
MAC 5500 (GE Healthcare; Little Chalfont, Buckinghamshire, UK) was used to conduct 12-lead ECGs (resting, 10 s) for each subject. At least two cardiologists were employed to analyze the ECGs by using callipers and magnifying glasses.
After at least 8 hours of fasting, fasting blood samples were collected in the morning. Blood samples were collected from an antecubital vein and then stored in EDTA vacutainer tubes (Becton, Dickinson and Co., Franklin Lakes, NJ, USA). Serum was then isolated from whole blood through centrifugalizing. Subsequently, samples were stored at −20 Celsius degree. Eventually, the samples were transported to a certified laboratory in the Second Affiliated Hospital of the Shandong University of TCM for analysis. Triglycerides (TG), total cholesterol (TC), LDL-C, HDL-C, and fasting plasma glucose (FPG) were analyzed by a HITACHI auto-analyzer (H7600, HITACHI, Tokyo, Japan). SIEMENS blood auto-analyzer (ADVIA2120i, SIEMENS, Berlin, German) was used to perform the blood routine test. Additionally, we randomly selected 8% of blood samples to be re-tested in the Clinical Laboratory of Shandong health commission to improve the data quality of the laboratory exam.
Definitions
Estimated glomerular filtration rate was calculated by following the CKD-EPI formula [Citation24]. Body mass index (BMI) was determined as weight (kg) divided by height squared (m*2). MHR was determined as monocytes count/high density-lipoprotein concentration [Citation20]. HTN was defined as mean systolic blood pressure (SBP) ≥ 140 mmHg with or without mean diastolic blood pressure (DBP) ≥ 90 mmHg. Besides, participants reporting usage of anti-hypertensive drugs within 2 weeks were regarded as hypertensive patients [Citation25]. Diabetes mellitus was diagnosed as an FBG ≥ 7.0 mmol/L with or without a previous diagnosis of DM by a physician [Citation26]. Anti-hypertensive medication was recognized as usage of anti-hypertensive drugs within the past 2 weeks. Lipid-lowering medication was determined as continuous usage of lipid-lowering drugs within the past 3 months. Subjects who reported usage of glucocorticoid drugs within the past 2 weeks were included in groups with glucocorticoid medication. According to the global recommendations, two independent neurologists performed the diagnosis of cerebrovascular disease with the confirmation of computed tomography or magnetic resonance imaging [Citation27]. Diagnosis of CHD was based on medical records and ECG results. Copies of all medical records were obtained for each in-hospital and out-hospital cardiovascular diagnoses. Two independent cardiologists reviewed the medication history to confirm the CHD diagnosis (ICD-10 code I 20 for angina pectoris, I 21 for acute myocardial infarction), which is similar to that used in the Framingham Heart Study [Citation28]. In addition, the cardiologists also used the ECG results conducted during the survey to reveal the presence of CHD further. If necessary, they checked the laboratory and imaging results from the hospitals which treated the patients. For a more accurate diagnosis, two members of the study events committee reviewed the diagnosis for the second time.
Statistical analyses
According to the distributions, continuous variables were summarized as median (interquartile) or mean values (standard deviation, SD). Categorical variables were displayed as frequency (percentage). Because of the highly skewed distributions, MHR were transformed by natural log calculation before further analyses. Mann-Whitney test and Student’s t-test were performed to compare continuous variables between subjects with or without CHD if appropriate. Chi-square test was employed to discover differences in categorical variables between subjects with or without CHD. The disparity of ordinal categorical variables between groups was detected by the Rank-Sum test. MHR was divided into quartiles according to the quartile cutoff acquired from SPSS 26 software. The dividing standard is listed below: Quartile 1:0< MHR<0.100, Quartile 2:0.100< MHR<0.131, Quartile 3:0.131< MHR<0.173, Quartile 4:0.173< MHR. The independent correlation between MHR and prevalent CHD was evaluated by multivariate logistic regression analysis. The results were shown as odds ratios (ORs) and 95% confidence intervals (95% CI). Moreover, a generalized additive model (GAM) together with a spline smoothing function was used to explore whether the association between MHR and the prevalence of CHD followed a linear pattern. To determine whether the association was linear, logarithmic likelihood ratio test was used to compare one pairwise and two pairwise logistic regression model. Finally, receiver operating characteristic (ROC) curve, integrated discrimination index (IDI) and category-free net reclassification index (NRI) were used to exam the efficacy of MHR to advance the identification of prevalent CHD. All the statistical analyses were conducted by SPSS 26.0 software (IBP corp), statistical software packages R (http://www.R-project.org, The R Foundation) and EmpowerStats (http://www.empowerstats.com, X&Y Solutions, Inc., Boston, MA). Statistical significance was determined as a two-tailed P value less than 0.05.
Results
summarized the data of the 6442 participants. Among them, 202 (3.14%) subjects were diagnosed with CHD. MHR was significantly higher in the CHD group (median: 0.15, interquartile range: 0.11–0.23) than in the non-CHD group (median: 0.13, interquartile range: 0.10–0.17).
Table 1. Characteristics of subjects divided by CHD
Multivariate logistic regression revealed the correlation between MHR and the prevalent CHD (). With adjustment of sex, age, annual family income, education, exercise, current smoking, and current drinking, each SD increase of MHR cast a 55.6% additional risk of prevalent CHD. After further adjustment of BMI, WC, diabetes, hypertension, LDL-C, TG, eGFR, lipid-lowering therapy and cerebrovascular disease history, the additional risk of prevalent CHD attenuated to 39.5% for each SD increase of MHR. Moreover, after splitting MHR into quartiles, the top quartile also demonstrated an 89.0% increment of the risk of prevalent CHD than the bottom category in the full model.
Table 2. Multivariate logistic regression of MHR for CHD
The present work further employed a smooth curve fitting to fully elucidate the correlation between MHR and the risk of prevalent CHD (). The results displayed an approximately linear pattern of the correlation, which means the prevalent CHD risk increases proportionally with the increment of MHR. Additionally, the logarithmic likelihood ratio test also suggested the linear trend between MHR and the risk of prevalent CHD (P for non-linear association = 0.133).
Figure 2. Smoothing curve fitting of the correlation between MHR and the CHD risk
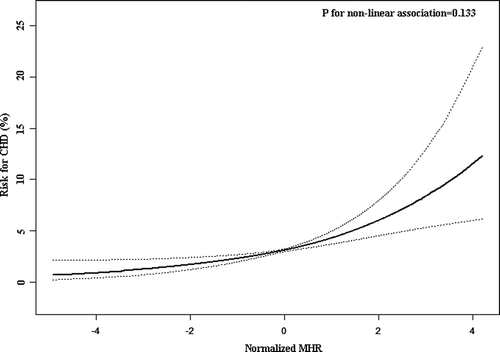
To validate the findings observed in were robust to common CHD risk factors, we conducted subgroup analysis according to subgroups defined by major CHD risk factors, including sex, age, hypertension, BMI, diabetes, and eGFR. All analyses adjusted for all covariates in the full model of , saving the variate that was stratified. displayed no significant difference existed between any subgroups (all P for interaction > 0.05), with ORs ranging from 0.943 to 1.542.
Figure 3. Stratified analyses for the efficacy of MHR on CHD
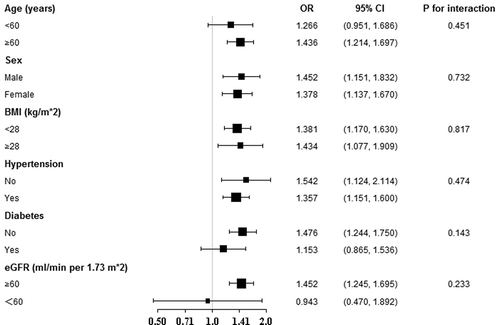
Finally, the present study employed ROC and reclassification analysis to explore the efficacy of MHR in the identification of prevalent CHD (). The AUC of MHR was 0.619 (95% CI: 0.607–0.630, P < 0.001). When introducing MHR into established common CHD risk factors (including sex, age, current smoking, current drinking, BMI, WC, diabetes, hypertension, LDL-C, TG, eGFR, lipid-lowering therapy and cerebrovascular disease history), we observed a significant improvement of AUC (0.744 vs 0.761, P for comparison = 0.028). Furthermore, category-free NRI (0.208, 95% CI: 0.069–0.348, P = 0.003) and IDI (0.007, 95% CI: 0.003–0.012, P = 0.003) in the reclassification analysis also demonstrated a remarkable improvement from MHR.
Table 3. ROC analysis and reclassification analyses for MHR to advance the risk classification of CHD
Discussion
Our work evaluated the correlation between MHR and prevalent CHD based on a general Chinese population. Besides, our results revealed this correlation followed a linear pattern in the whole range of MHR, suggesting the potential of MHR to positively associate with the prevalence of CHD without any threshold or saturation effect. Moreover, our study showed the correlation was robust to several major CHD risk factors, implicating the usefulness of MHR is applicable to multiple groups of specified participants. In addition, our study implicates the efficacy of MHR as a simple, rapid, and cost-effective marker to identify the prevalent CHD.
MHR is a novel index for assessing atherosclerosis by evaluating both dyslipidemia and inflammation [Citation20]. Previous researchers have demonstrated the usefulness of MHR in predicting several atherosclerotic diseases, including metabolic syndrome, ischemic stroke, and intra-stent restenosis [Citation21–23]. Considering that CHD also has a pathophysiological background of atherosclerosis, we postulated that MHR correlated with CHD and could be utilized to indicate the prevalent CHD.
The results confirmed our hypothesis. With the adjustment of clinical and demographic factors that could influence MHR or the prevalence of CHD, the logistic regression model still revealed a significant correlation between MHR and the prevalent CHD, suggesting MHR could be an efficient marker to indicate the prevalence of CHD. Moreover, the smooth curve fitting demonstrated this correlation followed a linear pattern in the full range of MHR without any threshold or saturation effect. This finding means the prevalence of CHD increases proportionally with the increase of MHR, suggesting the linearity of MHR to estimate the prevalent CHD. In addition, the stratified analysis showed that the positive correlation was robust to multiple major CHD risk factors, indicating our findings were stable and applicable to a wide range of populations.
The findings from ROC analysis showed the efficacy of MHR to identify the presence of CHD. Furthermore, when introducing MHR into several established clinical risk factors of CHD, the results displayed a significant advancement for the CHD identifying ability. These findings suggest the potential of MHR to optimize the identification of prevalent CHD. Moreover, although ROC analysis is the most widely used method to test the identifying ability of a new marker, statisticians have demonstrated its low sensitivity [Citation29]. ROC analysis cannot provide good information about whether introducing a new biomarker to established risk factors can optimize the accuracy to identify disease of individuals [Citation30]. Hence, studies may underestimate the usefulness of some markers. To overcome the disadvantages of ROC analysis, statisticians have put forward IDI and NRI for assessing the efficacy of a new marker to refine the identification of diseases’ presence [Citation31–33]. In the current work, both IDI and category-free NRI displayed a significant advancement when including MHR into established clinical risk factors. The results were consistent with the findings in ROC analysis and implicated the efficacy of MHR in advancing the identification of the presence of CHD. Overall, the results of ROC and reclassification analysis suggest that MHR could improve the identification of people with CHD. When applicating MHR to clinical conditions, clinicians may classify the CHD patients more efficiently and then make more reasonable decisions in the secondary prevention of CHD.
Our results were consistent with findings from similar research. A recent article demonstrated the association between MHR and prevalent CHD among in-hospital patients [Citation34]. The study recruited a total of 1867 in-hospital patients into statistical analysis. MHR was found to be higher in the CHD group than in the non-CHD group. Furthermore, the Spearman correlation analysis showed MHR was positively associated with the SYNTAX score. Finally, the logistic regression analysis displayed that MHR was independently associated with prevalent CHD. The results of the logistic regression analysis from both our work and this previous research supported an independent association between MHR and the prevalent CHD. However, there are still some critical differences between our work and this previous research. First, the two studies derived from different populations. Our work was conducted based on a general population, while the study mentioned above based on in-hospital patients. This difference determined that our results were better used in community clinics while their conclusions were more suitable for large hospitals. Second, our study evaluated whether the association between MHR and prevalent CHD was linear and explored the robustness of the association toward several major CHD risk factors while the aforementioned study did not. Accordingly, the results of our analysis were deeper, more reliable and could provide more information about the association between MHR and prevalent CHD. Some previous studies also discovered the value of MHR in estimating the SYNTAX score among patients with CHD, implicating the association between MHR and coronary atherosclerotic burden in CHD patients [Citation35–37]. These observations were consistent with our findings about the positive association between MHR and prevalent CHD, but since our survey did not collect information about the SYNTAX score, we cannot evaluate the value of MHR in estimating the SYNTAX score in a general population. Through the data of 705 patients who had undergone bare-metal stent implantation and additional control coronary angiography, Yilmaz et al. found the usefulness of MHR in the prediction of bare metal stent restenosis, which is another indicator of coronary atherosclerosis [Citation22]. Additionally, some researchers confirmed the predictive value of MHR for cardiovascular outcomes in CHD patients, providing compelling evidence for the clinical utility of MHR in atherosclerotic diseases [Citation38,Citation39]. More specifically, Ya et al. revealed that MHR is strongly associated with CHD in type 2 diabetes patients through 458 consecutive patients, suggesting the potential of MHR to predict the presence of CHD in these high-risk patients [Citation40]. However, all these previous studies based on relatively small sample size. Therefore, whether MHR correlates with prevalent CHD and whether MHR can refine the identification of the prevalence of CHD in the general population is still unknown. In contrast, our study demonstrated the value of MHR to optimize the identification of the prevalent CHD in a large-scale general population. Hence, our work may provide evidence for the application of MHR to screen CHD in the general population than merely in hospitalized patients.
It is necessary to address the concomitant diseases and drug treatments when interpreting the association between MHR and prevalent CHD. In the CHD group, the prevalence of HTN, DM and cerebrovascular disease history was significantly higher than that in the non-CHD group. HTN and DM were established CHD risk factor, and cerebrovascular disease history was also found to be associated with CHD [Citation41]. Therefore, the difference in HTN, DM and cerebrovascular disease history could cast an influence on the prevalence of CHD. Furthermore, the rate of lipid-lowering therapy was also significantly different between groups, which may account for all or part of the MHR difference between groups. According to our results, after adjustment of hypertension, diabetes, lipid-lowering, cerebrovascular disease history, and other variables (Model 2 in ), the association between MHR and prevalent CHD was still significant, implicating the association was independent of the influence of HTN, DM, cerebrovascular disease history and lipid-lowering therapy.
Our study still has some limitations that we must mention when interpreting our results. Firstly, because of the cross-sectional design, we can only provide implications for the association between MHR and prevalent CHD, but the causality of this association still needs more longitudinal studies to validate. Secondly, the prevalence of CHD in our current work was relatively lower than those in similar studies. However, according to a previous national survey of cardiovascular diseases in China, the prevalence of CHD in Chinese males and females were 0.74% and 0.51%, respectively [Citation42]. Furthermore, the China cardiovascular diseases report 2018 revealed that the prevalence of CHD maintained in this high level in the past decade [Citation43]. The above national survey demonstrated that the prevalence of CHD in people aged equal to or more than 40 years old ranged from 0.28% (40–49 years old group) to 2.41% (≥70 years old group) [Citation42]. Considering our population derived from northern china, which have a higher prevalence of cardiovascular diseases than southern china, we believe the prevalence of CHD (3.14%) in our population is acceptable. Nevertheless, the prevalence of CHD in cohorts derived from northern China ranged from 4.42% to 5.90% [Citation3,Citation44,Citation45]. There are two major reasons which may account for the relatively low prevalence of CHD in our population. The first reason derives from the definition of CHD. Considering the feasibility of the epidemiological study, our study could not adapt the same diagnostic criteria as studies based on in-hospital patients. Our diagnosis of CHD mainly depended on medical records and ECG results. Therefore, a part of the actual CHD patients would be misrecognized as non-CHD subjects, decreasing the reported prevalence of CHD in our population. However, most of these misrecognized subjects would be in the mild stage of CHD (typically stable angina). Accordingly, the misrecognition of these subjects would not cast a massive influence on the association between MHR and prevalent CHD. Furthermore, our diagnostic criteria are similar to that used in the Framingham Heart Study [Citation28]. The second reason arises from our population itself. Shandong province locates in the eastern area of northern China and close to the sea. Compared with other provinces in northern China, people in Shandong province have a healthier lifestyle (more vegetables, fruits and fishes and less air pollution due to the advantages of Shandong’s geography), which is beneficial for cardiovascular health. However, because our survey did not collect related information, we could not verify this point in the current work. Nevertheless, it is still reasonable that the actual CHD prevalence in Shandong province is lower than that in other provinces in northern China. Based on the above explanation, we believe that the results of our work are still reliable but needed to be verified in other population. Thirdly, our population originated from the rural areas of eastern China. Whether our results are suitable to the population in different geographic and economic conditions also needs further studies to confirm. Lastly, although we have adjusted many possible confounders in our analysis, bias caused by the residual confounding from uncollected risk factors may exist in our results. Hence, studies with a greater sample size and more collected information are required to prove the correlation between MHR and prevalent CHD in the near future.
Declaration of interest
No potential conflict of interest was reported by the author(s).
Peer reviewers on this manuscript have no relevant financial or other relationships to disclose.
Additional information
Funding
References
- Lozano R, Naghavi M, Foreman K, et al. Global and regional mortality from 235 causes of death for 20 age groups in 1990 and 2010: a systematic analysis for the global burden of disease study 2010. Lancet. 2012 Dec 15;380(9859):2095–2128.
- Go AS, Mozaffarian D, Roger VL, et al. Executive summary: heart disease and stroke statistics–2013 update: a report from the American Heart Association. Circulation. 2013 Jan 1;127(1):143–152.
- He L, Tang X, Song Y, et al. Prevalence of cardiovascular disease and risk factors in a rural district of Beijing, China: a population-based survey of 58,308 residents. BMC Public Health. 2012 Jan 16;12:34. .
- Yan R, Li W, Yin L, et al. Cardiovascular diseases and risk-factor burden in urban and rural communities in high-, middle-, and low-income regions of China: a large community-based epidemiological study. J Am Heart Assoc. 2017 Feb 6;6(2). DOI:10.1161/JAHA.116.004445.
- Pepine CJ, Nichols WW. The pathophysiology of chronic ischemic heart disease. Clin Cardiol. 2007 Feb;30(2 Suppl 1):I4–9.
- Libby P, Theroux P. Pathophysiology of coronary artery disease. Circulation. 2005 Jun 28;111(25):3481–3488.
- Schoenhagen P, Ziada KM, Vince DG, et al. Arterial remodeling and coronary artery disease: the concept of “dilated” versus “obstructive” coronary atherosclerosis. J Am Coll Cardiol. 2001 Aug;38(2):297–306. .
- Boudoulas KD, Triposciadis F, Geleris P, et al. Coronary atherosclerosis: pathophysiologic basis for diagnosis and management. Prog Cardiovasc Dis. 2016 May-Jun;58(6):676–692. .
- Ardehali A, Ports TA. Myocardial oxygen supply and demand. Chest. 1990 Sep;98(3):699–705.
- Bentzon JF, Otsuka F, Virmani R, et al. Mechanisms of plaque formation and rupture. Circ Res. 2014 Jun 6;114(12):1852–1866.
- Libby P. Inflammation in atherosclerosis. Arterioscler Thromb Vasc Biol. 2012 Sep;32(9):2045–2051.
- Schaftenaar F, Frodermann V, Kuiper J, et al. Atherosclerosis. Curr Opin Lipidol. 2016;27(3):209–215. .
- Swirski FK, Nahrendorf M. Leukocyte behavior in atherosclerosis, myocardial infarction, and heart failure. Science. 2013 Jan 11;339(6116):161–166.
- Simionescu M. Implications of early structural-functional changes in the endothelium for vascular disease. Arterioscler Thromb Vasc Biol. 2007 Feb;27(2):266–274.
- Rosenson RS, Brewer HB Jr., Ansell BJ, et al. Dysfunctional HDL and atherosclerotic cardiovascular disease. Nat Rev Cardiol. 2016 Jan;13(1):48–60. .
- Maiolino G, Rossitto G, Caielli P, et al. The role of oxidized low-density lipoproteins in atherosclerosis: the myths and the facts. Mediators Inflamm. 2013;2013:714653.
- Parthasarathy S, Barnett J, Fong LG. High-density lipoprotein inhibits the oxidative modification of low-density lipoprotein. Biochim Biophys Acta. 1990 May 22;1044(2):275–283.
- Murphy AJ, Woollard KJ, Hoang A, et al. High-density lipoprotein reduces the human monocyte inflammatory response. Arterioscler Thromb Vasc Biol. 2008 Nov;28(11):2071–2077. .
- Yvan-Charvet L, Pagler T, Gautier EL, et al. ATP-binding cassette transporters and HDL suppress hematopoietic stem cell proliferation. Science. 2010 Jun 25;328(5986):1689–1693.
- Kanbay M, Solak Y, Unal H, et al. Monocyte count/HDL cholesterol ratio and cardiovascular events in patients with chronic kidney disease. Int Urol Nephrol. 2014;46(8):1619–1625. .
- Uslu AU, Sekin Y, Tarhan G, et al. Evaluation of monocyte to high-density lipoprotein cholesterol ratio in the presence and severity of metabolic syndrome. Clin Appl Thromb Hemost. 2018 Jul;24(5):828–833. .
- Yilmaz S, Akboga M, Sen F, et al. Usefulness of the monocyte-to-high-density lipoprotein cholesterol ratio to predict bare metal stent restenosis. Biomark Med. 2016;10(9):959–966. .
- Bolayir A, Gokce S, Cigdem B, et al. Monocyte/high-density lipoprotein ratio predicts the mortality in ischemic stroke patients. Neurol Neurochir Pol. 2018;52(2):150–155. .
- Levey A, Stevens L, Schmid C, et al. A new equation to estimate glomerular filtration rate. Ann Intern Med. 2009;150(9):604–612. .
- James PA, Oparil S, Carter BL, et al. 2014 evidence-based guideline for the management of high blood pressure in adults: report from the panel members appointed to the Eighth Joint National Committee (JNC 8). Jama. 2014 Feb 5;311(5):507–520.
- Report of the expert committee on the diagnosis and classification of diabetes mellitus. Diabetes Care. 1997 Jul;20(7):1183–1197. .
- Hatano S. Experience from a multicentre stroke register: a preliminary report. Bull World Health Organ. 1976;54(5):541–553.
- Wilson PW, D’Agostino RB, Levy D, et al. Prediction of coronary heart disease using risk factor categories. Circulation. 1998 May 12;97(18):1837–1847.
- Cook NR. Use and Misuse of the Receiver Operating Characteristic Curve in Risk Prediction. Circulation. 2007;115(7):928–935.
- Pickering JW, Endre ZH. New metrics for assessing diagnostic potential of candidate biomarkers. Clin J Am Soc Nephrol. 2012 Aug;7(8):1355–1364.
- Pencina M, D’Agostino R, Steyerberg E. Extensions of net reclassification improvement calculations to measure usefulness of new biomarkers. Stat Med. 2011;30(1):11–21.
- Pencina MJ, D’ Agostino RB, D’ Agostino RB, et al. Evaluating the added predictive ability of a new marker: from area under the ROC curve to reclassification and beyond. Stat Med. 2008;27(2):157–172. .
- Grunkemeier GL, Jin R. Net reclassification index: measuring the incremental value of adding a new risk factor to an existing risk model. Ann Thorac Surg. 2015 Feb;99(2):388–392.
- Li Y, Li S, Ma Y, et al. Relationship between non-high-density lipoprotein cholesterol/apolipoprotein A-I and monocyte/high-density lipoprotein cholesterol ratio and coronary heart disease. Coron Artery Dis. 2020 Nov;31(7):623–627. .
- Akboga MK, Balci KG, Maden O, et al. Usefulness of monocyte to HDL-cholesterol ratio to predict high SYNTAX score in patients with stable coronary artery disease. Biomark Med. 2016;10(4):375–383. .
- Kundi H, Gok M, Kiziltunc E, et al. Relation between monocyte to high-density lipoprotein cholesterol ratio with presence and severity of isolated coronary artery ectasia. Am J Cardiol. 2015;116(11):1685–1689. .
- Cagdas M, Karakoyun S, Yesin M, et al. The association between monocyte HDL-C ratio and SYNTAX score and SYNTAX score II in STEMI patients treated with primary PCI. Acta Cardiol Sin. 2018 Jan;34(1):23–30.
- Zhang Y, Li S, Guo YL, et al. Is monocyte to HDL ratio superior to monocyte count in predicting the cardiovascular outcomes: evidence from a large cohort of Chinese patients undergoing coronary angiography. Ann Med. 2016 Aug;48(5):305–312. .
- Cetin MS, Ozcan Cetin EH, Kalender E, et al. Monocyte to HDL cholesterol ratio predicts coronary artery disease severity and future major cardiovascular adverse events in acute coronary syndrome. Heart Lung Circ. 2016 Nov;25(11):1077–1086. .
- Ya G, Qiu Z, Tianrong P. Relation of monocyte/high-density lipoprotein cholesterol ratio with coronary artery disease in type 2 diabetes mellitus. Clin Lab. 2018;64(6):901–906.
- Malakar AK, Choudhury D, Halder B, et al. A review on coronary artery disease, its risk factors, and therapeutics. J Cell Physiol. 2019 Aug;234(10):16812–16823. .
- Yang ZJ, Liu J, Ge JP, et al. Prevalence of cardiovascular disease risk factor in the Chinese population: the 2007-2008 China national diabetes and metabolic disorders study. Eur Heart J. 2012 Jan;33(2):213–220. .
- Ma LY, Chen WW, Gao RL, et al. China cardiovascular diseases report 2018: an updated summary. J Geriatr Cardiol. 2020 Jan;17(1):1–8.
- Wang Y, Li Y, Liu X, et al. Prevalence and influencing factors of coronary heart disease and stroke in Chinese rural adults: the henan rural cohort study. Front Public Health. 2019;7:411.
- Chen LZ, Ding RJ, Shi QT, et al. Risk factors and prevalence of cardiovascular disease of rural residents in Xianghe of Hebei province. Zhonghua Xin Xue Guan Bing Za Zhi. 2013 Oct;41(10):882–885.