Abstract
The objective of this study was to identify spatial variability in exposure to outdoor traffic-related air pollution with specific emphasis on comparing exposure estimates for working females and males across a metropolitan area. A spatial exposure simulation model was used to estimate annual average exposure to traffic-related nitrogen dioxide for males and females reporting regular work in census tracts other than that of their residence, in Vancouver, British Columbia. The model produced estimates of annual average exposure in six microenvironments (home indoor, work indoor, other indoor, outdoor, in-vehicle to work, and in-vehicle other) using time-activity patterns and work flow data, for males and females in each of 382 census tracts. This allowed for the identification of spatial variations in exposure estimates for each gender, due to mobility within the study region. Indoor sources of nitrogen dioxide were not included in the simulation. No significant differences in estimated total exposure were found between working females and males in general. Small but observable spatial differences, however, were found between working females and males at the 90th percentile of the exposure distributions associated specifically with the work indoor microenvironment. These were highest in suburban areas (+3 μ g/m3 for females, relative to total exposures in the range of 26 to 37 μ g/m3 annual average hourly nitrogen dioxide). These results identify specific geographic locations in the study area where personal monitoring studies might be warranted and suggest that the inclusion of workplace locations in multivariate modeling could be useful to further understand differences in estimated exposures.
El objetivo de este estudio era identificar la variabilidad espacial en la exposición exterior a la contaminación aérea relacionada con tráfico, con énfasis específico en la comparación de los estimativos de exposición para trabajadores de ambos sexos en un área metropolitana. Se utilizó un modelo de simulación de la exposición espacial para calcular el promedio de exposición anual al dióxido de nitrógeno originado en el tráfico para hombres y mujeres que reportan trabajo regular en tractos censales diferentes a los de su residencia, en Vancouver, Columbia Británica. El modelo generó estimativos de exposición promedia anual en seis microentornos (interior del hogar, lugar de trabajo en el hogar, otros lugares del hogar, al aire libre, en vehículo hacia el trabajo, y en vehículo por otros motivos), usando patrones de tiempo-actividad y datos de flujo del trabajo, para hombres y mujeres, en cada uno de 382 tractos censales. Esto permitió la identificación de variaciones espaciales en los estimativos de exposición para cada género, debidas a la movilidad dentro de la región de estudio. Las fuentes de dióxido de nitrógeno al interior de los hogares no fueron incluidas en la simulación. En general, no se detectaron diferencias significativas en los estimativos de exposición total entre hombres y mujeres. Sin embargo, se encontraron pequeñas aunque observables diferencias espaciales entre trabajadoras y trabajadores en el percentil 90 de las distribuciones de exposición asociadas específicamente con el microentorno de trabajo bajo techo. Las más altas de estas diferencias ocurren en áreas suburbanas (+3 μg/m3 para mujeres, relativo a la exposición total en el ámbito de 26 a 37 μg/m3 de promedio anual por hora de dióxido de nitrógeno). Estos resultados identifican localidades geográficas específicas en el área de estudio, donde estudios de monitoreo personal podrían justificarse, y sugieren que la inclusión de locaciones del lugar de trabajo en modelado multivariado podrían ser útiles para entender mejor las diferencias de exposición calculadas.
Chronic exposure to traffic-related outdoor air pollution has been associated with a range of negative health impacts, including decreased lung function and increased cardiopulmonary mortality in adults (CitationBrunekreef and Holgate 2002; CitationHealth Effects Institute 2004). It is common for epidemiological studies of traffic-related air pollution to use nitrogen dioxide (NO2) as an indicator of exposure to the total mix of pollutants (CitationNyberg et al. 2000; CitationHoek et al. 2002; CitationPedersen et al. 2004; CitationScoggins et al. 2004; CitationSekine et al. 2004). NO2 has been shown to be associated with proximity to roads (CitationRoorda-Knape et al. 1998; CitationJanssen et al. 2001; CitationGilbert et al. 2003; CitationMaruo et al. 2003), traffic volume (CitationKramer et al. 2000; CitationSmargiassi et al. 2005), and some of the constituents of traffic-related air pollution thought to be most harmful, such as black carbon from heavy-duty diesel vehicles (CitationBrunekreef et al. 1997; CitationLe Tertre et al. 2002; CitationKim et al. 2004). Identifying levels and understanding determinants of chronic exposure to traffic-related NO2 in a population therefore is an important step toward managing exposure risk and improving health outcomes.
We hypothesize a spatial pattern in the levels of exposure to traffic-related pollution, driven in part by the spatial pattern of pollution and also by individual mobility, as influenced by the relative location of residential neighborhoods with respect to the geographic arrangement of residential, commercial, and industrial areas and the road network. Working people living in the suburbs may commute along major transportation corridors to business centers where pollution levels may be higher. Spatial gradients in traffic-related air pollution exist, not only at the microscale in terms of distance from heavily used roads but also at the mesoscale in terms of the distance between business districts with high traffic volume and residential areas with low traffic volume. Exposures therefore may vary in an associated way and can be influenced by the amount of time spent in locations with elevated pollution. In addition to a spatial pattern in exposure, we hypothesize that these patterns might differ based on gender, given a body of research that suggests that females commute shorter distances in general, and that differences in commute distance are larger than differences in commute time between men and women (CitationBlumen 1994; CitationWyly 1998). This article explores whether spatial differences can be observed between the estimated exposures of working females and males.
There is little information on the spatial variation in exposure to traffic-related NO2 among individuals within an urban area. A number of studies have modeled the determinants of exposure to NO2 using personal monitoring in conjunction with individual time-activity diaries (CitationSpengler et al. 1994; CitationLevy 1998; CitationKousa et al. 2001; CitationLai et al. 2004). In general, spatial variation in exposure among individuals is not addressed. Instead, results are reported for the entire population studied in aggregate. Recently, Global Positioning Systems have been employed to capture the spatial characteristics of individual exposures, but these have been limited to small populations, generally fewer than seventy-five participants (CitationPhillips et al. 2001; CitationNethery et al. 2008). Personal monitoring, although desirable, is not feasible for the study presented in this article, as an inordinately large number of participants would be required to adequately represent spatial variation within the study area at a relatively fine scale.
Exposure simulation studies have been used to identify the probable distribution of exposure levels for large urban populations in multiple environments (i.e., at home, at work, in transit associated with work or nonwork activities, etc.; CitationOtt et al. 1988; CitationMcCurdy 1995; CitationJohnson, Capel, and McCoy 1996; CitationJohnson et al. 1999; CitationBurke, Zufall, and Ozkaynak 2001; CitationHanninen et al. 2003; CitationKruize et al. 2003; CitationBruinen de Bruin et al. 2004). These models use the indirect method of estimating exposure; that is, a time-weighted average of concentrations in the various environments (referred to as microenvironments, or MEs) visited over a specified time period (CitationDuan 1982; CitationOtt 1985; CitationKlepeis 1999). The simulation models use repeated random sampling of distributions representing the time-activity patterns of a population and the pollution concentrations in each ME to produce a single distribution that represents the range of exposures possible (CitationOzkaynak et al. 1999; CitationKruize et al. 2003). Typically, exposure simulation models have been used to produce a single summary distribution of estimated exposures for an entire urban population. Few report results at a finer scale; however, the United States Environmental Protection Agency's (EPA) Hazardous Air Pollutant Exposure Models (HAPEM) is an exception, providing estimated distributions of annual average exposure to various air pollutants for demographically representative populations for each census tract in the United States (CitationU.S. EPA 2006).
The spatial exposure simulation model (SESM) developed and implemented for this study represents a unique version of exposure simulation modeling that allows for the identification of spatial variation in exposure to traffic-related NO2 due specifically to mobility among census tracts. It incorporates highly detailed spatial data, shortest routes between home and work census tracts based on a road network and work flow data, and provides results at the census tract level. The SESM does not attempt to simulate a demographically representative population for each census tract but rather assumes constant demographic characteristics, thereby identifying spatial variability solely due to changing patterns of mobility, pollution, and distributions of residential and commercial properties among census tracts within the study area.
We specifically investigate the spatial pattern of variability in estimates of chronic (annual average hourly) exposure to traffic-related NO2 for working females and males in each of 382 census tracts in the Greater Vancouver Regional District of British Columbia. The SESM as implemented uses six MEs to estimate the distributions of exposure for each group in each census tract in the study area. The MEs included are home indoor (time spent inside at home), work indoor (time spent indoor at work), other indoor (time spent indoors at other locations, primarily shopping and commercial locations near home), outdoor, in-vehicle to work (time spent traveling to work by car or bus, although not differentiated between these two modes), and in-vehicle other (time spent traveling by car or bus to shopping and other commercial locations near home, again not differentiated by mode of travel). A fine-resolution spatial estimate of annual average NO2 is used to develop distributions of pollutant concentrations. ESRI ArcGIS 9.0 and custom C++ programming were used to develop the appropriate pollution concentration distributions and to implement the model.
Study Area
The simulation was conducted for the Greater Vancouver Regional District (GVRD), located in southern British Columbia on the west coast of Canada. As of 2004, the total population in the GVRD was 2,131,340, with 1,286,890 registered vehicles (CitationGVRD 2006a, CitationGVRD 2006c). Given forecasts that suggest that the population of the GVRD will reach 2,437,500 by 2015 (CitationGVRD 2006b), at the current ratio of 0.6 vehicles per capita, there will be an additional 175,600 registered vehicles in the region (for a total of 1,462,500 vehicles) within the next ten years. Current estimates suggest that about 3.5 million trips are made daily in the GVRD; about three quarters of these are by private vehicle (CitationGreater Vancouver Transportation Authority 2004). Statistics on the sixteen municipalities in the study area show that, on average, 57 percent of commuters travel outside of their home municipality to work, although this percentage is much lower near the downtown core and much higher in the inner suburbs (CitationGreater Vancouver Transportation Authority 2004). In addition to private vehicles, truck traffic forms a significant part of daily volume on regional roads, and “truck movements are expected to increase from around 16,000 in the peak hour today, to over 21,000 by 2013—an increase of around 31%” (CitationGreater Vancouver Transportation Authority 2004, 7). This study area presents an opportunity to implement the SESM due to the availability of suitable input data for census tracts in heavily urbanized as well as suburban and rural areas. shows the study area, its population density, and its general features.
Model Specification
The SESM is based on the indirect approach to estimating exposure (CitationDuan 1982; CitationOtt 1985; CitationKlepeis 1999) and includes six MEs, as shown in Equation Equation1:
The SESM was implemented for each of 382 census tracts in the study area, treating females and males differently. This required a set of representative time-activity patterns, as well as census tract-specific work flow patterns and distributions of pollutant.
Time-Activity Patterns
Data from the Canadian Human Activity Pattern Survey study (CHAPS; CitationLeech et al. 1997), conducted in 1994 and 1995, consist of individual time-activity patterns identifying the amount of time spent in different locations (but not the geographic coordinates) for twenty-four hours, as well as the date of survey, age, gender, and the number of hours worked for each respondent. These data were used as a basis for establishing values for the parameters t h , t w , t oi , t o , t vw , and t vo . We selected only time-activity patterns for individuals aged fifteen years or older who reported any time spent at work on a daily basis during weekdays, giving a total of 776 individual time-activity patterns from CHAPS. For each time-activity pattern, time reported as spent in a variety of CHAPS-defined locations was aggregated to develop total time spent in each of the six MEs included in this study; that is, each time-activity pattern consisted of six variables: time spent at home indoor, work indoor, other indoor, outdoor, in-vehicle to work, and in-vehicle other. The 776 individual time-activity patterns were then classified into six groups (the number of individual time-activity patterns in each group is indicated in parentheses): nonworking females summer (n = 161), nonworking females winter (n = 161), nonworking males summer (n = 159), nonworking males winter (n = 117), female workers (n = 84), and male workers (n = 94). Time-activity patterns from responses collected for CHAPS between 21 March and 22 September were classified as summer; all others were classified as winter. The numbers of individual time-activity patterns in each group are comparable to those used in other studies; for example, CitationKruize et al. (2003) used 434, 322, 83, and 100 time-activity pattern records to represent the total population of urban adults in Helsinki, Basel, Prague, and Athens, respectively.
Chronic (i.e., annual average) exposure, rather than single acute exposure, is the focus of this study, so a procedure for estimating annual exposure based on the use of daily time-activity patterns was developed. For example, to simulate exposure for working females residing in a specific census tract, a single time-activity pattern is randomly selected to represent the time spent in each ME on all workdays in a year. On nonworkdays, we assume that female worker patterns are similar to female nonworker patterns, so for summer weekends (fifty-three days between 21 March and 22 September in any given year), a female nonworker summer time-activity pattern is randomly selected, the appropriate distributions sampled for pollution levels, and exposure calculated as per Equation Equation1. This is repeated fifty-three times, and the average is taken to represent the total and partial exposures for summer weekends. A similar procedure is used to calculate the total and partial exposures for winter weekends. Results for workdays and summer and winter weekends are then combined to provide a single time-weighted, seasonally adjusted estimate of total exposure to annual average hourly NO2, as per Equation Equation2:
Importantly, we used the same set of time-activity patterns for every census tract in the study area. This was done to control for the effects of demographic variation on the resulting estimates, thereby revealing variability due only to spatial patterns in mobility and pollution concentrations.
Work Flow Patterns
Work flow data for all females and males aged fifteen years and older reporting employment, based on a 20 percent sample in each census tract on 15 May 2001, were purchased from Statistics Canada for each of the 382 census tracts in the study area. More specifically, for each census tract, 20 percent of the residents were asked to identify the census tract in which they worked, producing a complete list of all other census tracts reported as work locations along with the frequency with which each was reported by the sample population. The work flow data were used to develop separate female and male work pair lists and associated frequencies for each census tract, which were used to guide the selection of values for the parameters t vw and C vw , as described in the subsequent section. We assume that respondents worked at the same location all year, and although this might not be true in all cases, no data are available to refine this assumption. Workers who had a place of employment within the census tract of their residence were excluded from use in the SESM, under the assumption that commuting distance would be negligible and that the ambient pollution levels at home and work would be similar. Approximately 87 percent of 382 census tracts in the study area are less than 5 km2 in size, and on average, 5 percent (SD = 4 percent) of the total population reported working in the same census tract. Data showing a work census tract outside of the study area were not provided by Statistics Canada, but it is likely less than those reporting living and working in the same census tract. Digital boundary files for the 2001 census tracts were acquired via an academic research agreement with DMTI Spatial, an official commercial reseller of Census data.
Pollutant Concentration Distributions
Unique pollution concentration distributions for each ME are required for each census tract in the study area, and this requires a spatial estimate of concentration. The model and the methods used to develop the ME distributions can be applied to any spatial estimate of pollution concentrations; however, for this study a highly detailed estimate is required to capture gradients in pollution concentrations regionally as well as in proximity to roadways.
A spatial estimate of annual average hourly NO2 levels (), developed by researchers at the University of British Columbia using the land use regression (LUR) method with field monitoring conducted in 2003, was used in the SESM and is fully described in CitationHenderson et al. (2007). Briefly, linear stepwise multiple regression is used to develop a model for predicting pollution levels at any given point in a study area based on data collected from temporary monitors and independent variables developed from the land use surrounding each temporary monitor. Typically, independent variables include traffic density or volume, length of roads, percentage of land in commercial or residential use, and so on, within specific distances from the temporary monitor (CitationBriggs et al. 1997; CitationBriggs et al. 2000; CitationBrauer et al. 2005; CitationJerrett et al. 2005; CitationRoss et al. 2006). The LUR model used for this research, noted in Equation Equation3, produces an R 2 of 0.52, which is typical for this method, and was based on NO2 measurements for a two-week period in both the spring and fall of 2003 and associated geographic variables at 116 monitored sites in the GVRD. The model is then used to calculate NO2 for each 5-meter grid cell in the study area.
The most important feature of the spatial estimate derived via land use regression is that gradients in NO2 with distance from roadways are captured, as are higher concentrations of NO2 in commercially developed areas, and the regional gradient from the more polluted urban core to the less polluted rural areas is clearly represented. It is this level of detail in the spatial allocation of NO2 levels that allows the SESM, as employed in this research, to differentiate among census tracts based on their location in the study area and given the range of MEs included in the model. A limitation of the LUR method is the potential underestimation of NO2 levels directly on the roadways, as field samplers are located near roadways and not in the actual traffic lanes. In addition, it is important to note that the NO2 estimate used for this study represents annual average hourly NO2, which is useful for investigating chronic exposures but does not support the simulation of acute exposure estimates; that is, for specific times within a twenty-four-hour period.
Outdoor Microenvironment
For the outdoor ME, a regularly spaced grid of sample points is used to extract NO2 values from the LUR estimate within 5 km of the centroid of the census tract. These values create a distribution of possible outdoor pollutant concentrations in the vicinity of the census tract. For a given census tract, a randomly selected value from the associated set of values was used for the parameter C o .
Home Indoor, Work Indoor, and Other Indoor Microenvironments
Additional geographic detail is incorporated in the SESM through the use of spatial property assessment data. These data were used to identify the geographic location of every property in the study area for which taxes are assessed, as well as the primary use of the building on the property. For a specific census tract, the distribution of NO2 levels for the home indoor ME is comprised of values extracted from the NO2 surface for each residential building location (assumed to be the centroid of each property parcel) in the census tract. For the work indoor ME, the pollution distribution is developed the same way, extracting NO2 levels at commercial building locations within the census tract. Pollution distributions for the other indoor ME are based on sampling the pollution surface at commercial locations within 5 km of the centroid of the census tract.
Because outdoor air pollution does not always fully penetrate into indoor areas, indoor/outdoor (I/O) ratios are applied to adjust the pollution level distributions for the indoor MEs used in the SESM. In the absence of empirical data on long-term I/O ratios for NO2 for different building types, fixed I/O ratios are used in the SESM, an approach similar to that used in the U.S. EPA HAPEM model.Footnote 1 For the SESM, I/O ratios were subjectively assigned based on a review of available I/O monitoring studies (CitationLiao, Bacon-shone, and Kim 1991; CitationEkberg 1995; CitationMonn et al. 1998; CitationLee, Chan, and Chiu 1999; CitationChao and Law 2000; CitationPartti-Pellinen et al. 2000; CitationGuo, Lee, and Chan 2004; CitationLai et al. 2004; CitationBlondeau et al. 2005) and the primary building use documented in the spatial property assessment data. Residential buildings were assigned an I/O ratio of 0.70; large office buildings 0.35; manufacturing, industrial, and civic buildings 0.50; and small stores, services, and restaurants 0.70. During the development of the SESM, results were found to be insensitive to the use of different I/O ratios. For example, results were not significantly different under a scenario designed to maximize differences between commuters and noncommuters, where all commercial buildings were set to 0.35 and all residential buildings to 0.75, when compared to results using the more moderate scenario used in the application of the SESM described here.
For a given census tract, random selections from these adjusted pollution values provide the inputs for parameters C h , C w , and C oi .
In-Vehicle Other Microenvironment
For the in-vehicle other ME, a digital road data set was acquired for the study area from DMTI Spatial Inc. under an academic research agreement. The road network was converted to regularly spaced points along the roads, which were used as sampling points to extract NO2 values along roadways within 5 km of the census tract centroid; these values are then averaged to provide a single transit other value for the census tract, rather than a distribution of values. A distance of 5 km was chosen to represent the average distance people travel from home on non-work-related trips based on a review of studies reporting a typical range between 2 and 8 km (CitationJanelle, Goodchild, and Klinkenberg 1988; CitationHandy 1996; CitationCrane and Crepeau 1998). As well, the SESM results were found to be insensitive to variations in the distance chosen between 2.5 and 7.5 km (CitationSetton 2007). The I/O ratio was assumed to be 1.0; that is, pollution concentrations inside vehicles are equivalent to concentrations on roadways. For a given census tract, the single average value was used for the parameter C vo .
In-Vehicle to Work Microenvironment
The digital road data set was converted into a geographic information system (GIS) network using ArcGIS 9.0, thereby allowing for the identification of the shortest route (assuming travel by motor vehicle) between two points while observing appropriate travel restrictions such as one-way streets and speed limits. For every census tract centroid, the shortest route based on time to the centroid of every work census tract listed in the work pair file was identified and saved as a unique GIS file using a specific identifier (i.e., originCT10_destinationCT_205) with attributes including total distance and total time associated with the route. In total, 34,782 GIS files were created, representing the shortest routes between each home census tract and all associated work census tracts. These routes represent a best case scenario, as we did not adjust for additional time that might be spent in this ME due to potential congestion during typical commute times. For each unique route, a single length-weighted average pollution level was calculated based on the LUR estimate.
In the calculation of Equation Equation1 for a given census tract, a time-activity pattern was randomly chosen, as was a work pair from that census tract's work pair frequency list. The time associated with the shortest route was used for time spent in the in-vehicle to work ME (t vw ), rather than the amount of time noted in the time-activity pattern. An adjustment was made to the amount of time spent in the home indoor ME to offset the substitution of the shortest route time. The single pollution value associated with the shortest route was used for the parameter C vw .
Estimating Annual Average Exposures
The calculation described in Equation Equation2 was repeated 10,000 times for each gender in each census tract, producing a single gender- and census tract-specific distribution for total exposure, as well as six distributions for partial exposures associated with each ME, with 95 percent confidence of estimating exposure at any percentile to within ± 1 percentile (CitationCullen and Frey 1998). These distributions can be compared statistically within census tracts and between census tracts, as well as visually through graphs and maps. For example, the median (50th percentile values) of the total or partial exposure estimate distributions in each census tract can be mapped to identify spatial patterns. The 10th percentile and 90th percentile levels can be similarly mapped and compared to identify the lower and upper ranges of the exposure estimates. The differences between the 10th and 90th percentiles can be calculated and mapped to show where variability within census tracts is highest (i.e., where the range between the 10th and 90th percentile values is largest).
Results
Overall, estimated total exposures, expressed as annual average hourly NO2 (μ g/m3), ranged from approximately 12 to 35 μ g/m3 between the 10th and 90th percentiles for both working females and males. The spatial pattern of estimated total exposure levels in each census tract is similar to the pattern of the underlying estimated NO2 levels, with higher exposures in the downtown core and suburban nodes and lower exposures in more rural areas. Boxplots () show the minimum and maximum (the lowest and highest horizontal line) and the 25th percentile, median, and 75th percentile level (bottom, middle, and top of box) of the simulated exposure estimates at the 10th, 50th, and 90th percentile, as observed across the 382 census tracts in the study area.
Figure 3 Box plots of the 10th, 50th, and 90th percentile estimates of the total exposure distributions in 382 census tracts for working females and males.
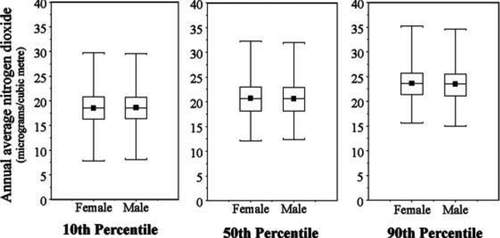
The means of the 50th percentile values for males and females in all census tracts were compared using the standard t test (i.e., the t test was applied to a distribution of 50th percentile male values with n = 382 and a similar distribution of 50th percentile female values, again with n = 382). No statistically significant differences were found between means of the distributions of total exposure at the 50th percentile, nor were any significant differences found between the means of the distributions of 90th percentile or 10th percentile values.
The range of estimated exposures within specific MEs provides an indication of the importance of each with respect to overall exposure and is generally associated with the amount of time spent in each ME, given the time-weighted nature of the indirect exposure calculation used in this study (). Notably, exposures in the in-vehicle to work ME are low. Although it is recognized that traffic-related pollutant levels in vehicles or while in congested traffic can be very high in relation to levels away from these environments (CitationAkland et al. 1985; CitationJo and Park 1999; CitationAdams et al. 2001; CitationChau et al. 2002; CitationGeorgoulis et al. 2002; CitationRiediker et al. 2003), when weighted in relation to the amount of time spent in this ME compared to other MEs, the contribution to total exposure in this study is low.
Table 1 Range of estimated exposures and percentage of day spent in each microenvironment, females and males
Within-census tract differences between the distributions of exposure estimates for females and males in each ME are generally small. In both the home indoor and work indoor MEs, differences range from less than 1 to 3 μ g/m3; in the outdoor ME, differences range from less than 1 to 1.5 μ g/m3; in all other MEs, differences are less than 1 μ g/m3. Again, differences in the amount of time spent in each ME are reflected, with females having consistently higher exposures in the home indoor ME and lower exposures in the other indoor and outdoor MEs.
Mapping the within-census tract differences in the distributions for the home indoor and work indoor MEs provides useful insights into the spatial pattern of the differences. Spatial patterns associated with other MEs are not presented, given the small magnitude of the differences in the results. For home indoor, reveals that the highest differences at the 50th percentile are located in the areas associated with the highest NO2 levels; that is, the downtown core and some of the suburban nodes. This spatial pattern is the same for the 10th and 90th percentiles (not shown). On average, working females spend slightly more time at home (), which could contribute to higher total exposures (i.e., up to + 3 μ g/m3) where pollution levels are higher (i.e., urban core areas) than working males in the same areas.
Figure 4 Map of female minus male NO2 exposure estimate differences at the 50th percentile for the home indoor microenvironment.
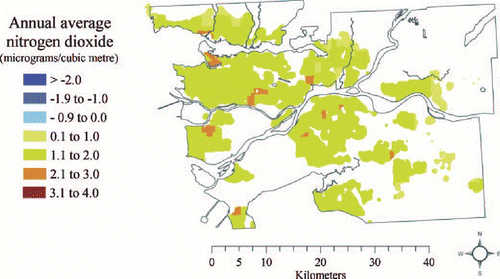
For work indoor, shows the spatial pattern of differences at the 90th percentile. Differences are highest (between 2 and 3 μ g/m3) predominantly in suburban areas. Several possible reasons could explain the spatial pattern seen in . One is that suburban working males may be working in census tracts with larger proportions of office buildings with lower I/O ratios than suburban working females, thereby reducing their exposure due to a protective effect of being indoors in buildings where less traffic-related NO2 infiltrates. In the study area, these buildings are more common in the downtown core, so if suburban males are more often working in the downtown core than suburban females, this might contribute to the observed difference. A second explanation is that suburban working females are working in census tracts in the study area where pollution levels are higher more often than suburban working males, and therefore they have higher exposures. A third possibility is that female and male workers from the same home census tract are going to the same work census tracts, but the females are systematically going to buildings with higher I/O ratios (i.e., small stores, services, and restaurants). No data are available that identify the building-specific coordinates for working females and males, so it is not possible to explore this scenario further; however, comparing the home–work pairs and frequencies for females and males in those suburban census tracts exhibiting high differences suggests that the second explanation is more likely. and show proportional work flows for a selected suburban census tract where the difference between working females and males at the 90th percentile of partial exposure for the work indoor ME is 3 μ g/m3 of annual average hourly NO2. In , the circles identify the census tracts where females from the selected home census tract reported working; the size of the circle is related to how many respondents reported working in the census tract. Overall, for the selected home census tract, a high proportion of females worked in census tracts located in the developed commercial areas in the downtown core and suburban nodes where estimated NO2 levels are among the highest in the study area. The work flow patterns are markedly different for the working males from the same census tract, as shown in . In this case, the largest proportions of males worked in census tracts that are not in the downtown core or suburban commercial centers. This pattern tends to hold true for each of the census tracts where differences are at least 2 μ g/m3, although not always as clearly as in the example in and .
Discussion
No statistically significant difference in the means of the distributions of total exposure estimates to NO2 was observed between working females and males. Although not directly comparable, personal monitoring studies provide similar evidence. Personal monitoring of working-age adults conducted for the EXPOLIS study in Europe found no significant difference between genders, with the mean hourly NO2 exposures measured over twenty-four hours being 25.5 and 24.6 μ g/m3 for males and females, respectively, when there were no significant indoor sources present, such as gas stoves (CitationRotko et al. 2001). Similarly, in a multicity study on NO2 exposure based on personal monitoring of 568 working and nonworking adults, gender was found not to be significantly correlated with total NO2 exposure over forty-eight hours (CitationLevy 1998). Conversely, in a sample of working and nonworking participants in Wisconsin, some with gas stoves, gender (female) was found to be a significant predictor of total NO2 exposure (measured over a one-week period) in the summer, along with being a full-time worker; working with or near gas furnaces, boilers, ovens, or flames; and commute distance (CitationQuackenboss et al. 1986). In the winter, gender was not a factor; only working with or near welding or cutting torches and individual age were found to be significant predictors of total NO2 exposure. The magnitude of differences in exposures between working females and males was not reported in CitationQuackenboss et al. (1986), making it difficult to compare to the SESM results.
In the simulation results presented here, differences between working females and males were seen at the 90th percentile of partial exposure distributions associated with the work indoor ME. The largest differences were in the suburban portions of the study area. This result appears to be due to systematic differences in where males and females go to work within the study area in relation to the urban core and suburban nodes. This observation is consistent with a number of studies on gender-based differences in commuting distance. CitationBlumen (1994) presents a comparison of studies from the United States, Canada, France, Australia, and Israel, showing that differences in commute distance between female and male commuters are more pronounced in suburban areas. More recently, CitationShearmur (2006) analyzed the characteristics of the female and male commuter populations associated with six employment nodes in Montreal, including the central business district. Shearmur found that, on average, women worked closer to home than did men by about 1.05 km, and no particular gender association in the differences was seen between each employment node. Instead, Shearmur did find that females were more often employed in the central business district and other employment nodes, whereas males more often worked at various locations throughout the region rather than preferentially in the central business district or employment nodes. These findings correspond to the patterns seen in and . Why this occurs is not illuminated by the SESM results, but various theoretical approaches that inform research on what influences commuting patterns in general include a spatial mismatch between housing and employment opportunities (CitationKain 1968; CitationMcLafferty and Preston 1996); rational economic decisions made by workers to optimize the trade-offs between wages, housing costs, and transport costs (CitationRouwendal and Nijkamp 2004; CitationShearmur 2006); and gender-specific roles in terms of household duties and economic sectors (CitationHanson and Pratt 1995).
Due to the specification of the SESM as applied here, results are subject to the following caveats. First, results apply to workers commuting by motor vehicle, not working in the census tract of their residence, and who have a fixed indoor place of employment all year. Therefore, the SESM does not reflect exposures for people who drive for work (e.g., bus, taxi, or truck drivers), who regularly work outside or at different locations within a region (e.g., real estate sales, home care workers, construction workers), or who use other modes of transportation such as cycling or walking. Results also do not reflect real measured personal exposures but instead reflect possible exposures based on the input data. The exposure estimates reported here are specific to ambient traffic-related air pollution as indicated by NO2 and do not include any indoor sources of NO2 such as gas stoves or work-related equipment. It is also assumed that there are no significant sources of ambient NO2 in the study area other than traffic. Finally, SESM results are sensitive to the use of different methods for estimating the spatial allocation of pollution levels (CitationSetton 2007). Here, the most spatially detailed estimate of NO2 levels available for the study area was employed. Less detailed estimates, such as might be produced via spatial interpolation from fixed-site air quality monitors, might produce results that differ from those reported here. Similarly, patterns might differ for other pollutants, such as ozone.
Other considerations include the lack of validation, the different time periods for which data were available, and the use of work flow data aggregated to census tracts. In the first case, other exposure simulation models, which produced a single aggregate distribution for an urban population, have been evaluated when sufficient personal monitoring data existed for the same population (CitationOtt et al. 1988; CitationLaw et al. 1997; CitationHanninen et al. 2003; CitationKruize et al. 2003; CitationBruinen de Bruin et al. 2004). The study design of this research requires exposure estimates for a large number of census tracts, making validation via personal monitoring impractical. The SESM is still useful in that numerous scenarios and groups can be simulated, allowing for the identification of relative similarities and differences based on the input data, with the results being used to delineate specific spatial variations within the study area that could more readily be investigated empirically in future studies.
Second, input data for the SESM were collected at different times and over different periods. The time-activity patterns in CHAPS were collected in 1994 and 1995 (CitationLeech et al. 1997). The work flow data from Statistics Canada are based on a survey conducted on 15 May 2001. The annual average NO2 estimate is based on data from 2003. Property assessment and parcel data were collected in 2004 and 2005. With respect to the work flow data, more current data are not available. It is therefore implicitly assumed that overall urban and residential development patterns have not changed enough between 2001 and 2004 and 2005 (the date of the property-related data) to affect the SESM results. Similarly, it is assumed that the spatial patterns of traffic-related pollution did not change dramatically between 2001 (the work flow data date), 2003 (the LUR estimate date) and 2004 and 2005 (the property-related data date). With respect to the currency of the time-activity patterns from CHAPS, no additional time-activity surveys have since been conducted for the cities included in the survey, so it was not possible to ascertain how the amount of time spent in different MEs might have changed since 1994 and 1995. Studies that reported time spent in MEs analogous to those used in the SESM were reviewed and the results are reported in . No clear trends were obvious with respect to changes in the amount of time spent in different MEs over time, although different geographic locations and city sizes made direct comparison difficult. In the model's favor, the general proportions of time spent in each ME were similar in magnitude across cities and over time. For the SESM, given the lack of other data, it is assumed that the time-activity patterns collected for CHAPS in 1994 and 1995 remain relevant for 2001, the date of the work flow survey.
Table 2 Summary of time spent in microenvironments for a range of locations and years
Third, work flow data were acquired for census tracts, making the SESM results susceptible to the modifiable areal unit problem. It is possible that the use of boundaries other than census tracts, and thus potentially differently aggregated work flow data, could produce different results. We used data at the finest scale practical and so are less concerned about the effects of using larger and therefore fewer boundaries. The potential effects of using different or more, smaller boundaries (i.e., dissemination areas) on the results remain unknown, primarily because (a) the individual-level data are not available to evaluate the effect of using different boundaries at the same scale, and (2) we are unable to practically model several million possible combinations of home–work pairs using dissemination areas. Hypothetically, spatial variability in work flow data within each census tract would have to be high and have a distinct spatial pattern within the census tract to greatly affect the existing results (i.e., workers in one area of the census tract having significantly different work locations than workers in another area of the same census tract). With 87 percent of the census tracts in the study area having an area of 5 km2 (i.e., a 2.5 × 2.5 km square), it is likely reasonable to assume that subpopulations within each census tract are homogeneous, at least in terms of work flow patterns.
In conclusion, the SESM results suggest that, in general, there is not significant spatial variation in the estimates of annual average total exposure to traffic-related NO2 between working females and males as simulated in this study, but there is observable spatial variation when looking specifically at estimated exposures associated with time spent at work. These results provide directions for future research. For example, including personal monitoring of working females and males in suburban areas might be fruitful in determining whether these differences are empirically observable and aid in identifying the associated contributing factors. Other approaches, such as multilevel modeling, might also be useful to further explore the determinants of exposure, and the SESM results suggest that incorporating place of work into such models could be informative. Importantly, these results are specific to NO2 in the GVRD and may be different for other pollutants, particularly those with spatial patterns that differ from those observed for NO2, as well as for other urban areas with different spatial distributions of residential and commercial and industrial buildings and road networks.
About the Author
Her research interests include exposure assessment for environmental epidemiology and methods for incorporating high-resolution data sets in estimating exposure to environmental pollutants.
His research interests focus on the advancement of the geographic information sciences for better decision making with a focus on diverse application including the fields of health, tourism, and natural resources.
Her research interests include investigating geographies of health, specifically related to healthy aging for older adults and those living in rural environments.
His research interests focus on environmental and spatial epidemiology, with a particular focus on air pollutants.
Acknowledgments
Notes
a n = 84.
b n = 94.
aTotals may not add to 100 percent due to rounding. SESM = spatial exposure simulation model.
*This work was supported by Health Canada as part of the Border Air Quality Study and by the University of Victoria via a graduate student fellowship. Special thanks are due to the Lead Investigator, Dr. Michael Brauer (University of British Columbia), and to numerous data providers, including Dr. Judy Leech (Canadian Human Activity Pattern Survey) and the individual municipalities and jurisdictions that freely provided property data. We also extend our thanks to Dr. Ray Copes (University of British Columbia) and Dr. Les Foster (University of Victoria) for comments and guidance during the development of this research and for the anonymous reviewers whose comments on this article were very much appreciated.
1. In the U.S. EPA HAPEM model, a fixed value for the penetration factor is employed for each of five ME groups (indoor at residence, indoor at other site, outdoors near a road, outdoors away from a road, and in a vehicle). The fixed values are based on a literature review, and set using the following criteria: (1) If a reviewed study reported an I/O ratio with a median or mean less than or equal to one, the median was used first, then the mean if that is all that was reported. If more than one study reported the median or mean, the average of the values was used. (2) If only the range of I/O values was reported and the range extended below one, the difference between the minimum and one was split and the resulting value used. (3) When no I/O ratios were reported as less than one, one was used as a default, the idea being to exclude indoor sources from consideration (CitationICF Consulting and TRJ Environmental Inc. 2000).
Literature Cited
- Adams , H. S. , Nieuwenhuijsen , M. J. , Colvile , R. N. , McMullen , M. A. S. and Khandelwal , P. 2001 . Fine particle (PM2.5) personal exposure levels in transport microenvironments, London, UK . Science of the Total Environment , 279 ( 1–3 ) : 29 – 44 .
- Akland , G. G. , Hartwell , T. D. , Johnson , T. R. and Whitmore , R. W. 1985 . Measuring human exposure to carbon-monoxide in Washington, DC, and Denver, Colorado, during the winter of 1982–1983 . Environmental Science & Technology , 19 ( 10 ) : 911 – 18 .
- ArcGIS, Version 9.1 , Redlands, CA : ESRI .
- Blondeau , P. , Iordache , V. , Poupard , O. , Genin , D. and Allard , F. 2005 . Relationship between outdoor and indoor air quality in eight French schools . Indoor Air , 15 ( 1 ) : 2 – 12 .
- Blumen , O. 1994 . Gender differences in the journey to work . Urban Geography , 15 ( 3 ) : 223 – 45 .
- Brauer , M. , Henderson , S. , Jerrett , M. and Beckerman , B. 2005 . Land use regression modeling of nitrogen oxides and fine particulate matter in the Greater Vancouver Regional District , Blaine, WA : International Perspectives on Environmental Management .
- Briggs , D. J. , Collins , S. , Elliott , P. , Fischer , P. , Kingham , S. , Lebret , E. , Pryl , K. , Van Reeuwijk , H. , Smallbone , K. and Van der Veen , A. 1997 . Mapping urban air pollution using GIS: A regression-based approach . International Journal of Geographical Information Science , 11 ( 7 ) : 699 – 718 .
- Briggs , D. J. , de Hoogh , C. , Gulliver , J. , Wills , J. , Elliott , P. , Kingham , S. and Smallbone , K. 2000 . A regression-based method for mapping traffic-related air pollution: Application and testing in four contrasting urban environments . The Science of the Total Environment , 253 : 151 – 67 .
- Bruinen de Bruin , Y. , Hanninen , O. , Carrer , P. , Maroni , M. , Kephalopoulos , S. , di Marco , G. S. and Jantunen , M. 2004 . Simulation of working population exposures to carbon monoxide using EXPOLIS-Milan microenvironment concentration and time-activity data . Journal of Exposure Analysis and Environmental Epidemiology , 14 ( 2 ) : 154 – 63 .
- Brunekreef , B. and Holgate , S. T. 2002 . Air pollution and health . Lancet , 360 ( 9341 ) : 1233 – 42 .
- Brunekreef , B. , Janssen , N. A. H. , deHartog , J. , Harssema , H. , Knape , M. and vanVliet , P. 1997 . Air pollution from truck traffic and lung function in children living near motorways . Epidemiology , 8 ( 3 ) : 298 – 303 .
- Burke , J. M. , Zufall , M. J. and Ozkaynak , H. 2001 . A population exposure model for particulate matter: Case study results for PM2.5 in Philadelphia, PA . Journal of Exposure Analysis and Environmental Epidemiology , 11 ( 6 ) : 470 – 89 .
- Chao , C. Y. H. and Law , A. 2000 . A study of personal exposure to nitrogen dioxide using passive samplers . Building and Environment , 35 ( 6 ) : 545 – 53 .
- Chau , C. K. , Tu , E. Y. , Chan , D. W. T. and Burnett , C. J. 2002 . Estimating the total exposure to air pollutants for different population age groups in Hong Kong . Environment International , 27 ( 8 ) : 617 – 30 .
- Clench-Aas , J. , Bartonova , A. , Gronskei , K. E. , Hagen , L. O. , Braathen , O. A. and Walker , S. E. 1999 . Air pollution exposure monitoring and estimation: Part VI. Ambient exposure of adults in an industrialised region . Journal of Environmental Monitoring , 1 ( 4 ) : 341 – 47 .
- Crane , R. and Crepeau , R. 1998 . Does neighborhood design influence travel?: A behavioral analysis of travel diary and GIS data . Transportation Research Part D-Transport and Environment , 3 ( 4 ) : 225 – 38 .
- Cullen , A. C. and Frey , H. C. 1998 . Probabilistic techniques in exposure assessment: A handbook for dealing with variability and uncertainty in models and inputs , New York : Plenum .
- Duan , N. 1982 . Models for human exposure to air pollution . Environment International , 8 : 305 – 09 .
- Ekberg , L. E. 1995 . Concentrations of NO2 and other traffic related contaminants in office buildings located in urban environments . Building and Environment , 30 ( 2 ) : 293 – 98 .
- Georgoulis , L. B. , Hanninen , O. , Samoli , E. , Katsouyanni , K. , Kunzli , N. , Polanska , L. , Bruinen de Bruin , Y. , Alm , S. and Jantunen , M. 2002 . Personal carbon monoxide exposure in five European cities and its determinants . Atmospheric Environment , 36 ( 6 ) : 963 – 74 .
- Gilbert , N. L. , Woodhouse , S. , Stieb , D. M. and Brook , J. R. 2003 . Ambient nitrogen dioxide and distance from a major highway . Science of the Total Environment , 312 ( 1–3 ) : 43 – 46 .
- Greater Vancouver Regional District (GVRD) . 2006a . Regional development key facts—GVRD population estimates 1995–2005 , Vancouver, BC, , Canada : Greater Vancouver Regional District .
- Greater Vancouver Regional District (GVRD) . 2006b . Regional development key facts—GVRD population projections by local health areas , Vancouver, BC, , Canada : Greater Vancouver Regional District .
- Greater Vancouver Regional District (GVRD) . 2006c . Regional development key facts—Total number of registered vehicles 1994–2004 , Vancouver, BC, , Canada : Greater Vancouver Regional District .
- Greater Vancouver Transportation Authority . 2004 . 2005–2007 three-year plan & ten year outlook , Vancouver, BC, , Canada : TransLink .
- Guo , H. , Lee , S. C. and Chan , L. Y. 2004 . Indoor air quality investigation at air-conditioned and non-airconditioned markets in Hong Kong . Science of the Total Environment , 323 ( 1–3 ) : 87 – 98 .
- Handy , S. L. 1996 . Understanding the link between urban form and nonwork travel behavior . Journal of Planning Education and Research , 15 ( 3 ) : 183 – 98 .
- Hanninen , O. , Kruize , H. , Lebret , E. and Jantunen , M. 2003 . EXPOLIS simulation model: PM2.5 application and comparison with measurements in Helsinki . Journal of Exposure Analysis and Environmental Epidemiology , 13 ( 1 ) : 74 – 85 .
- Hanson , S. and Pratt , G. 1995 . Gender, work, and space , London and New York : Routledge .
- Health Effects Institute . 2004 . Special report: 15 health effects of outdoor air pollution in developing countries of Asia: A literature review , Boston : Health Effects Institute International Scientific Oversight Committee .
- Henderson , S. B. , Beckerman , B. , Jerrett , M. and Brauer , M. 2007 . Application of land use regression to estimate long-term concentrations of traffic-related nitrogen oxides and fine particulate matter . Environmental Science & Technology , 41 ( 7 ) : 2422 – 28 .
- Hoek , G. , Brunekreef , B. , Goldbohm , S. , Fischer , P. and van den Brandt , P. A. 2002 . Association between mortality and indicators of traffic-related air pollution in The Netherlands: A cohort study . Lancet , 360 ( 9341 ) : 1203 – 09 .
- ICF Consulting and TRJ Environmental Inc . 2000 . Development of microenvironmental factors for the HAPEM4 in support of the National Air Toxics Assessment (NATA) external review draft report, Office of Air Quality Planning and Standards , Research Triangle Park, NC : US Environmental Protection Agency .
- Janelle , D. G. , Goodchild , M. F. and Klinkenberg , B. 1988 . Space-time diaries and travel characteristics for different levels of respondent aggregation . Environment and Planning A , 20 ( 7 ) : 891 – 906 .
- Janssen , N. A. H. , van Vliet , P. H. N. , Aarts , F. , Harssema , H. and Brunekreef , B. 2001 . Assessment of exposure to traffic related air pollution of children attending schools near motorways . Atmospheric Environment , 35 ( 22 ) : 3875 – 84 .
- Jerrett , M. , Arain , A. , Kanaroglou , P. , Beckerman , B. , Potoglou , D. , Sahsuvaroglu , T. , Morrison , J. and Giovis , C. 2005 . A review and evaluation of intraurban air pollution exposure models . Journal of Exposure Analysis and Environmental Epidemiology , 15 ( 2 ) : 185 – 204 .
- Jo , W. K. and Park , K. H. 1999 . Commuter exposure to volatile organic compounds under different driving conditions . Atmospheric Environment , 33 ( 3 ) : 409 – 17 .
- Johnson , T. , Capel , J. and McCoy , M. 1996 . Estimation of ozone exposures experienced by urban residents using a probabilistic version on NEM and 1990 population data , Durham, NC : U.S. Environmental Protection Agency .
- Johnson , T. , Mihlan , G. , Lapointe , L. J. , Fletcher , K. and Capel , J. 1999 . Estimation of carbon monoxide exposures and associated carboxyhemoglobin levels in Denver residents using pNEM/CO (Version 2.0) , Research Triangle Park, NC : U.S. Environmental Protection Agency . Draft report
- Kain , J. F. 1968 . Housing segregation, negro employment, and metropolitan decentralization . Quarterly Journal of Economics , 82 ( 2 ) : 175 – 97 .
- Kim , J. J. , Smorodinsky , S. , Lipsett , M. , Singer , B. C. , Hodgson , A. T. and Ostro , B. 2004 . Traffic-related air pollution near busy roads—The East Bay children's respiratory health study . American Journal of Respiratory and Critical Care Medicine , 170 ( 5 ) : 520 – 26 .
- Klepeis , N. E. 1999 . An introduction to the indirect exposure assessment approach: Modeling human exposure using microenvironmental measurements and the recent National Human Activity Pattern Survey . Environmental Health Perspectives , 107 : 365 – 74 .
- Kousa , A. , Monn , C. , Rotko , T. , Alm , S. , Oglesby , L. and Jantunen , M. J. 2001 . Personal exposures to NO2 in the EXPOLIS-study: Relation to residential indoor, outdoor and workplace concentrations in Basel, Helsinki and Prague . Atmospheric Environment , 35 ( 20 ) : 3405 – 12 .
- Kramer , U. , Koch , T. , Ranft , U. , Ring , J. and Behrendt , H. 2000 . Traffic-related air pollution is associated with atopy in children living in urban areas . Epidemiology , 11 ( 1 ) : 64 – 70 .
- Kruize , H. , Hanninen , O. , Breugelmans , O. , Lebret , E. and Jantunen , M. 2003 . Description and demonstration of the EXPOLIS simulation model: Two examples of modeling population exposure to particulate matter . Journal of Exposure Analysis and Environmental Epidemiology , 13 ( 2 ) : 87 – 99 .
- Lai , H. K. , Kendall , M. , Ferrier , H. , Lindup , I. , Alm , S. , Hanninen , O. Jantunen , M. 2004 . Personal exposures and microenvironment concentrations of PM2.5, VOC, N02 and CO in Oxford, UK . Atmospheric Environment , 38 ( 37 ) : 6399 – 410 .
- Law , P. L. , Lioy , P. J. , Zelenka , M. P. , Huber , A. H. and McCurdy , T. R. 1997 . Evaluation of a probabilistic exposure model applied to carbon monoxide (pNEM/CO) using Denver personal exposure monitoring data . Journal of the Air & Waste Management Association , 47 ( 4 ) : 491 – 500 .
- Lee , S. C. , Chan , L. Y. and Chiu , M. Y. 1999 . Indoor and outdoor air quality investigation at 14 public places in Hong Kong . Environment International , 25 ( 4 ) : 443 – 50 .
- Leech , J. A. , Wilby , K. , McMullen , E. and Laporte , K. 1997 . The Canadian human activity pattern survey: Report of methods and population surveyed . Chronic Diseases in Canada , 17 ( 3 ) http://www.phac-aspc.gc.ca/publicat/cdic-mcc/17-3/d_e.html(last accessed 6 November 2009)
- Le Tertre , A. , Medina , S. , Samoli , E. , Forsberg , B. , Michelozzi , P. , Boumghar , A. Vonk , J. M. 2002 . Short-term effects of particulate air pollution on cardiovascular diseases in eight European cities . Journal of Epidemiology and Community Health , 56 ( 10 ) : 773 – 79 .
- Levy , J. I. 1998 . Impact of residential nitrogen dioxide exposure on personal exposure: An international study . Journal of the Air & Waste Management Association , 48 ( 6 ) : 553 – 60 .
- Liao , S. S. T. , Bacon-shone , J. and Kim , Y. S. 1991 . Factors influencing indoor air-quality in Hong-Kong—Measurements in offices and shops . Environmental Technology , 12 ( 9 ) : 737 – 45 .
- Maruo , Y. Y. , Ogawa , S. , Ichino , T. , Murao , N. and Uchiyama , M. 2003 . Measurement of local variations in atmospheric nitrogen dioxide levels in Sapporo, Japan, using a new method with high spatial and high temporal resolution . Atmospheric Environment , 37 ( 8 ) : 1065 – 74 .
- McCurdy , T. 1995 . Estimating human exposure to selected motor vehicle pollutants using the NEM series of models: Lessons to be learned . Journal of Exposure Analysis and Environmental Epidemiology , 5 ( 4 ) : 533 – 50 .
- McLafferty , S. and Preston , V. 1996 . Spatial mismatch and employment in a decade of restructuring . Professional Geographer , 48 ( 4 ) : 420 – 31 .
- Monn , C. , Brandli , O. , Schindler , C. , Ackermann-Liebrich , U. and Leuenberger , P. 1998 . Personal exposure to nitrogen dioxide in Switzerland . Science of the Total Environment , 215 ( 3 ) : 243 – 51 .
- Nethery , E. , Leckie , S. E. , Teschke , K. and Brauer , M. 2008 . From measures to models: An evaluation of air pollution exposure assessment for epidemiological studies of pregnant women . Occupational and Environmental Medicine , 65 ( 9 ) : 579 – 86 .
- Nyberg , F. , Gustavsson , P. , Jarup , L. , Bellander , T. , Berglind , N. , Jakobsson , R. and Pershagen , G. 2000 . Urban air pollution and lung cancer in Stockholm . Epidemiology , 11 ( 5 ) : 487 – 95 .
- Ott , W. R. 1985 . Total human exposure . Environmental Science & Technology , 19 ( 10 ) : 880 – 86 .
- Ott , W. , Thomas , J. , Mage , D. and Wallace , L. 1988 . Validation of the simulation of human activity and pollutant exposure (shape) model using paired days from the Denver, CO, carbon-monoxide field-study . Atmospheric Environment , 22 ( 10 ) : 2101 – 13 .
- Ozkaynak , H. , Zufall , M. , Burke , J. , Xue , J. and Zidek , J. 1999 . A probabilistic population exposure model for PM10 and PM2.5 . Epidemiology , 10 ( 4 ) : S79 – S79 .
- Partti-Pellinen , K. , Marttila , O. , Ahonen , A. , Suominen , O. and Haahtela , T. 2000 . Penetration of nitrogen oxides and particles from outdoor into indoor air and removal of the pollutants through filtration of incoming air . Indoor Air-International Journal of Indoor Air Quality and Climate , 10 ( 2 ) : 126 – 32 .
- Pedersen , C. B. , Raaschou-Nielsen , O. , Hertel , O. and Mortensen , P. B. 2004 . New directions: Air pollution from traffic and schizophrenia risk . Atmospheric Environment , 38 ( 22 ) : 3733 – 34 .
- Phillips , M. L. , Hall , T. A. , Esmen , N. A. , Lynch , R. and Johnson , D. L. 2001 . Use of global positioning system technology to track subject's location during environmental exposure sampling . Journal of Exposure Analysis and Environmental Epidemiology , 11 ( 3 ) : 207 – 15 .
- Quackenboss , J. J. , Spengler , J. D. , Kanarek , M. S. , Letz , R. and Duffy , C. P. 1986 . Personal exposure to nitrogen-dioxide—Relationship to indoor outdoor air-quality and activity patterns . Environmental Science & Technology , 20 ( 8 ) : 775 – 83 .
- Riediker , M. , Williams , R. , Devlin , R. , Griggs , T. and Bromberg , P. 2003 . Exposure to particulate matter, volatile organic compounds, and other air pollutants inside patrol cars . Environmental Science & Technology , 37 ( 10 ) : 2084 – 93 .
- Roorda-Knape , M. C. , Janssen , N. A. H. , de Hartog , J. J. , van Vliet , P. H. N. , Harssema , H. and Brunekreef , B. 1998 . Air pollution from traffic in city districts near major motorways . Atmospheric Environment , 32 ( 11 ) : 1921 – 30 .
- Ross , Z. , English , P. B. , Scalf , R. , Gunier , R. , Smorodinsky , S. , Wall , S. and Jerrett , M. 2006 . Nitrogen dioxide prediction in Southern California using land use regression modeling: Potential for environmental health analyses . Journal of Exposure Science and Environmental Epidemiology , 16 ( 2 ) : 106 – 14 .
- Rotko , T. , Kousa , A. , Alm , S. and Jantunen , M. 2001 . Exposures to nitrogen dioxide in EXPOLIS-Helsinki: Microenvironment, behavioral and sociodemographic factors . Journal of Exposure Analysis and Environmental Epidemiology , 11 ( 3 ) : 216 – 23 .
- Rouwendal , J. and Nijkamp , P. 2004 . Living in two worlds: A review of home-to-work decisions . Growth and Change , 35 ( 3 ) : 287 – 303 .
- Scoggins , A. , Kjellstrom , T. , Fisher , G. , Connor , J. and Gimson , N. 2004 . Spatial analysis of annual air pollution exposure and mortality . The Science of the Total Environment , 321 : 71 – 85 .
- Sekine , K. , Shima , M. , Nitta , Y. and Adachi , M. 2004 . Long term effects of exposure to automobile exhaust on the pulmonary function of female adults in Tokyo, Japan . Occupational and Environmental Medicine , 61 ( 4 ) : 350 – 57 .
- Setton , E. 2007 . Probabilistic estimates of variability in exposure to traffic-related air pollution in the Greater Vancouver Regional District: A spatial perspective , Victoria, BC, , Canada : University of Victoria .
- Shearmur , R. 2006 . Travel from home: An economic geography of commuting distances in Montreal . Urban Geography , 27 ( 4 ) : 330 – 59 .
- Smargiassi , A. , Baldwin , M. , Pilger , C. , Dugandzic , R. and Brauer , M. 2005 . Small-scale spatial variability of particle concentrations and traffic levels in Montreal: A pilot study . Science of the Total Environment , 338 ( 3 ) : 243 – 51 .
- Spengler , J. , Schwab , M. , Ryan , P. B. , Colome , S. , Wilson , A. L. , Billick , I. and Becker , E. 1994 . Personal exposure to nitrogen-dioxide in the Los Angeles Basin . Journal of the Air & Waste Management Association , 44 ( 1 ) : 39 – 47 .
- U.S. Environmental Protection Agency . 2006 . Further technical details about HAPEM4 http://www.epa.gov/ttnatw01/nata/hapem4-followup2.html(last accessed 6 November 2009)
- Wyly , E. K. 1998 . Containment and mismatch: Gender differences in commuting in metropolitan labor markets . Urban Geography , 19 ( 5 ) : 395 – 430 .