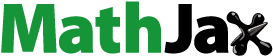
ABSTRACT
Regional capabilities are regarded a pillar of Smart Specialisation Strategy (S3). There is yet little focus in S3 policy on the role of interregional linkages. This study on 292 NUTS-2 regions in Europe finds that interregional linkages have a positive effect on the probability of regions to diversify, especially in peripheral regions. What matters is not being connected to other regions per se, but being connected to regions that provide complementary capabilities. Finally, we propose a new indicator that enables regions to identify other regions as strategic partners in their S3 policy, depending on the presence of complementary capabilities in other regions.
INTRODUCTION
A large body of literature shows that regions build on existing capabilities to develop new activities (Balland et al., Citation2019; Boschma, Citation2017; Hidalgo et al., Citation2018). Local capabilities have been regarded as a source of regional change in Smart Specialisation Strategy (S3) in the European Union (EU) from its very start (Foray, Citation2015; Radosevic et al., Citation2018). The objective is building competitive advantage in new domains in which regions possess capabilities (Foray et al., Citation2009; McCann & Ortega-Argilés, Citation2015). What attracted little attention is that S3 also required regions to identify potential links of their privileged domains with other regions in Europe. Despite these S3 guidelines, there is little focus on the role of interregional linkages in Smart Specialisation policy (Barzotto et al., Citation2019; Iacobucci & Guzzini, Citation2016; Radosevic & Ciampi Stankova, Citation2015; Santoalha, Citation2019; Sörvik et al., Citation2016; Thissen et al., Citation2013; Uyarra et al., Citation2014, Citation2018; Varga et al., Citation2020).
Interregional linkages are considered to give regions access to external knowledge that can tackle or avoid the tendency of regions to get locked in (Ascani et al., Citation2020; Bathelt et al., Citation2004; Boschma & Iammarino, Citation2009; Camagni, Citation1991; Eriksson & Lengyel, Citation2019; Grabher, Citation1993; Grillitsch & Nilsson, Citation2015; Hassink, Citation2005; Hesse & Fornahl, Citation2020; Miguelez & Moreno, Citation2018; Tavassoli & Carbonara, Citation2014). However, there is little understanding of how interregional linkages may affect the development of new activities in regions, and if found important, to what extent interregional linkages can compensate for weak or missing capabilities in a region. Studies on regional diversification have primarily focused on regional capabilities, but neglected the role of interregional linkages (Boschma, Citation2017; Whittle et al., Citation2020). The same applies to the literature on new path development that paid little to no attention to interregional links (Trippl et al., Citation2018). This may be one of the reasons why interregional connectivity is poorly implemented in Smart Specialisation policy in Europe. Scholars have also mentioned that a systematic, well-accepted and easy-to-apply method to identify relevant links between regions is still missing or weakly developed (Iacobucci & Guzzini, Citation2016).
This paper makes a first step to fill these gaps in the scientific as well as the S3 literature. The first objective is to investigate the impact of interregional ties on technological diversification in 292 NUTS-2 regions in Europe for the period 1992–2016, on top of the impact of regional capabilities. Using patent data from the Organisation for Economic Co-operation and Development’s (OECD) REGPAT data set (2019 version), we estimate the entry probability of a new technology in a region in all EU-28 countries and four European Free Trade Association (EFTA) countries. Following Boschma and Iammarino (Citation2009), we expect linkages giving access to additional capabilities in other regions that are related to existing capabilities in a region will have a stronger impact on the ability of regions to diversify. We do indeed find strong evidence for that: what matters is not being connected to other regions per se, but being connected to regions that provide complementary capabilities. Having complementary interregional linkages significantly increases the probability of regions in Europe to develop new technological specializations. We also examined whether interregional linkages are more important for diversification in peripheral regions, as these regions are often perceived to have weak capabilities (Grillitsch & Nilsson, Citation2015; Rodríguez-Pose & Wilkie, Citation2019). Our study shows that peripheral regions tend to diversify less, but their capacity to diversify increases significantly when connecting to regions with complementary capabilities.
The second objective of the paper is to propose a new indicator that can identify for each region and for each technology to what extent other regions in Europe have complementary capabilities that are missing in a region. This information can be used by regions to design an S3 strategy in which strategic partnerships are developed with other regions, given their capabilities.
The remainder of the paper is structured as follows. The next section gives a short literature review. We then describe the data set and variables used. We present the empirical findings on the effect of different types of interregional linkages on regional diversification in Europe. We also present an indicator that regions in their S3 policy can use to map complementary capabilities in other regions. The final section concludes.
INTERREGIONAL LINKAGES AND REGIONAL DIVERSIFICATION
To develop new economic activities and upgrade existing activities in regions is a principal objective of S3 policy. Balland et al. (Citation2019) argue that the S3 policy should target economic activities with high growth potential that can build on existing capabilities in regions. The idea is that regions are more likely to develop activities related to existing local activities because these provide similar (but not identical) capabilities, such as knowledge, skills and institutions. Consequently, new capabilities needed to develop new and upgrade existing activities are easier to acquire when close to local capabilities (D’Adda et al., Citation2019). By contrast, unrelated diversification requires a complete transformation of local capabilities, which is accompanied by high costs and a high risk of failure, and thus unlikely to happen.
This claim has found confirmation in empirical research in the last decade (Boschma, Citation2017). The product space literature provided evidence that the emergence of new export products in countries are not random events but strongly embedded in capabilities at the national scale (Hidalgo et al., Citation2007). The economic geography literature found a similar tendency of regions to diversify into new activities related to existing capabilities (Kogler et al., Citation2013; Muneepeerakul et al., Citation2013; Neffke et al., Citation2011; Rigby, Citation2015). Despite different research layouts, empirical studies find that related diversification is the rule: local capabilities condition which new activities are more likely to develop in regions. This principle of relatedness seems to hold, no matter what types of activities (industries, technologies, occupations or scientific fields) are considered, what relatedness measures (skill relatedness, product relatedness, technological relatedness, input–output relatedness, etc.) are employed, or at what spatial scales (local, regional, national, global) studies are conducted (Hidalgo et al., Citation2018).
However, these studies primarily focus on regional (and national) capabilities. As a result, they draw little attention to interregional linkages that could also affect regional diversification (Boschma, Citation2017; Whittle et al., Citation2020). Also, the path development literature pays little attention to the role of interregional linkages for new path creation (Trippl et al., Citation2018). This is remarkable because scholars have claimed for long that extra-regional linkages are crucial for regional development and prevent regions from ending up in a lock-in situation (e.g., Asheim & Isaksen, Citation2002; Bathelt et al., Citation2004; Camagni, Citation1991; Giuliani & Bell, Citation2005; Hassink, Citation2005; Vicente et al., Citation2011).
In regions there are strong forces at work that make individuals, firms and networks prone to lock-in. Access to information is limited and, above all, firms have limited capacity to absorb external information (Simon, Citation1955). To reduce this uncertainty, firms tend to search for new knowledge close by (Nelson & Winter, Citation1982), that is, in their own cognitive domains, in their own networks and in their own local surroundings. Access to distant knowledge is poor in general, the more so when knowledge is tacit (Gertler, Citation2003). Therefore, knowledge diffusion is often geographically bounded (Balland & Rigby, Citation2017; Balland et al., Citation2020; Jaffe et al., Citation1993). The potential downside of this tendency is too much inward orientation. Knowledge exchange with the same local actors may lose value over time (Maskell & Malmberg, Citation1999) and can lead to cognitive lock-in a region (Nooteboom, Citation2000). This means there is a tendency of regions to get locked in and become over-specialized (Boschma & Lambooy, Citation1999; Grabher, Citation1993).
This lock-in process may be avoided or overcome by linking to agents in other regions to obtain access to external knowledge pools (Crespo et al., Citation2014; Maskell & Malmberg, Citation2007). Bathelt et al. (Citation2004) argued that cluster firms need to supplement local knowledge with external knowledge, and for that purpose build global pipelines. Network studies have shown that well-performing firms in clusters connect to bodies of knowledge residing outside clusters (e.g., Giuliani & Bell, Citation2005). Scholars have also stressed the crucial role of gatekeepers that link local actors to knowledge outside their regions (Breschi & Lenzi, Citation2015; Broekel & Mueller, Citation2018; Graf, Citation2011; Morrison, Citation2008; Morrison et al., Citation2013). However, there is yet little understanding of the role of interregional knowledge sourcing during the emergent phase of clusters (Henn, Citation2013; Ter Wal & Boschma, Citation2011; Vicente, Citation2018).
There is evidence that lagging regions, in particular, rely on non-local linkages when innovating because their own capabilities and local networks tend to be weak (Fitjar & Rodríguez-Pose, Citation2011; Grillitsch & Nilsson, Citation2015; Vale & Carvalho, Citation2013). De Noni et al. (Citation2018) showed, for instance, that firms in lagging regions are more innovative when engaged in collaborative linkages with knowledge-intensive regions. Barzotto et al. (Citation2019) find that the level of extra-regional collaboration is often higher in peripheral regions where it promotes innovation. This suggests that regions with weaker capabilities benefit more from non-local knowledge inputs.
Scholars also explore how interregional trade linkages may be connected to S3 policy (Brennan & Rakhmatullin, Citation2018; Radosevic & Ciampi Stankova, Citation2015). Thissen et al. (Citation2013) examined trade linkages between regions and how they affect demand-led growth (growth of markets in region’s export destinations) and structural growth (development of new export products) in regions. The global value chain literature (Gereffi et al., Citation2005) has shown how regions play different roles in global networks: some regions act as centres of corporate control and host high-end activities such as research and development (R&D), while other regions function primarily as branch plant economies. This has resulted in a division of labour in which regions may be locked-in in certain activities at the global scale (Blažek, Citation2016). This makes relevant the question of whether regions have possibilities to upgrade their position in existing value chains and enter new value chains (Los et al., Citation2017). This literature on trade linkages has been less explicit, however, on how interregional linkages contribute to new path development (Trippl et al., Citation2018).
Few studies have assessed the importance of extra-regional linkages for new path development. Bahar et al. (Citation2014) and Boschma et al. (Citation2017) found that regions are more likely to develop new export industries in which their neighbour regions are already specialized. Neighbouring regions also tend to have more similar export structures when they are connected. Andersson et al. (Citation2013) found that the entry of new high-quality export products in regions is positively affected by high-quality imports. Santoalha (Citation2019) looked at the role of cooperation inside regions and across regions. He concluded that both forms of cooperation boost regional diversification in Europe. Whittle et al. (Citation2020) found that interregional collaboration networks had a positive effect on regional (related) diversification in Europe, especially when external knowledge sourcing is done in a diverse set of regions. What these studies do not account for is the nature of interregional linkages in terms of relatedness that may affect regional diversification.
Indeed, what these studies tend to neglect is that regions require absorptive capacity to exploit and benefit from external knowledge (Boschma & Iammarino, Citation2009; Miguelez & Moreno, Citation2015). This means that not all non-local knowledge is of immediate relevance and not all linkages to other regions matter for the capacity of a region to diversify (Boschma et al., Citation2009). Using trade data, Boschma and Iammarino (Citation2009) found that regional growth is not simply affected by extra-regional linkages. Instead, they found a positive effect of extra-regional linkages when the proximity between the knowledge base of a region and the external knowledge flowing into a region is neither too small nor too large. Boschma et al. (Citation2014) looked at the impact of relatedness between local knowledge and the inflow of non-local knowledge on new biotech activities in cities. Barzotto et al. (Citation2019) applied a relatedness framework while examining the role of extra-regional linkages for technological development in EU regions. Miguelez and Moreno (Citation2018) examined the effect of extra-regional linkages on the type of innovations in regions. They found that extra-regional knowledge linkages have a higher impact on innovation in a region when the similarity between external knowledge flows and local knowledge is higher. Extra-regional linkages promoted radical innovations when the extra-regional knowledge is related, rather than similar to the existing knowledge base in the region.
What all these studies did not look at, however, is the effect of extra-regional linkages on regional diversification. Moreover, the question is still open whether access to non-local capabilities is important for regional diversification when relevant capabilities are missing in a region (Whittle et al., Citation2020). Grillitsch and Nilsson (Citation2015) claim that non-local linkages do not necessarily complement local knowledge spillovers and can even compensate for the lack of local knowledge spillovers in peripheral regions. De Noni et al. (Citation2017) argued instead that local and non-local linkages in tandem are crucial for knowledge creation and productivity in regions.
In sum, few studies to date have estimated the effect of interregional linkages on regional diversification, and no study yet has examined the extent to which the nature of interregional linkages (in terms of relatedness) has had an impact on the diversification process in regions. To address this gap in the literature, we examine the importance of interregional linkages for regional diversification in new technologies in Europe, while controlling for local capabilities. We develop a new measure of complementarity in interregional linkages to assess whether linkages that give a region access to additional capabilities in other regions that are related to existing capabilities in the region have an impact on regional diversification. We also show that this new complementarity measure enables a region to identify capabilities in other regions that might help the region to diversify in new activities.
DATA, VARIABLES AND METHOD
This section explains what data we used, what variables were constructed and what method was employed to analyse the impact of regional capabilities and interregional ties on technological diversification in 292 NUTS-2 regions in Europe for the period 1992–2016.
Following other papers on regional technological diversification (Boschma et al., Citation2015; Rigby, Citation2015), we estimate an entry model that assesses the probability that a region specializes in a new technology. We use patent data that are derived from the OECD’s REGPAT data set (2019 version). In the study, we assigned patents to 654 technological classes (four-digit Cooperative Patent Classification – CPC), and to 292 European regions (at the NUTS-2 level), based on the address of inventors. These include regions in all EU-28 countries and the four EFTA countries (Iceland, Liechtenstein, Norway and Switzerland).
The dependent variable is the entry, or not, of a new specialization in a technology in a region. Following other studies (e.g., Balland et al., Citation2019; Rigby, Citation2015), we calculate the relative technological advantage (RTA) to assess whether a region becomes specialized in a technology that is new to the region. RTA is a binary variable that takes the value 1 when a region possesses a greater share of patents in technology class i than in the reference category (the EU as a whole), and 0 otherwise. In other words, a region r has an RTA in the production of technological knowledge i (r = 1, … , n; i = 1, … , k) such that if:
As other studies on regional diversification (Boschma, Citation2017), a linear probability model is estimated to assess the probability that a region develops an RTA (i.e., RTA > 1) in a new technology. The entry probability of a new technology is assessed for time windows of five years. As we have patent data for the period 1992–2016, we calculate this entry probability for five subsequent periods (1992–96, 1997–2001, 2002–06, 2007–11 and 2012–16).
All independent variables are measured in the period before the time window of five years. Our first main variable of interest is the role of relevant regional capabilities. As other studies (e.g., Boschma et al., Citation2015; Hidalgo et al., Citation2007; Rigby, Citation2015), we construct a relatedness density (RD) measure to assess the effect of regional capabilities on technological diversification in European regions. RD is measured as the degree of proximity (relatedness) between a technology and the technological portfolio of a region. This requires two steps.
First, we calculate the degree of relatedness between each pair of technologies for a total of 654 CPC technology classes using co-occurrence analysis. This measures the frequency of occurrence of combinations of two technology classes on a patent document. We normalized these co-occurrences using the cosine similarity index. Second, this relatedness information is used to calculate the RD measure. That is, for each region r, we calculated the density of technology production in the vicinity of individual technologies i. Following Hidalgo et al. (Citation2007) and Boschma et al. (Citation2015), the density of knowledge production around a given technology i in region r at time t is derived from the technological relatedness of technology i to all other technologies j in which the region has an RTA, divided by the sum of technological relatedness of technology i to all the other technologies j in the reference region (Europe as a whole) at time t. RD takes a minimum value of 0 when there are no technologies related to technology i present in region r at time t, while it takes a maximum value of 100 when all technologies related to i are present in region r at time t:
The other main variable of interest is the role of interregional linkages. We developed two measures to capture the effect of interregional linkages on regional diversification. The first indicator is based on co-inventors residing in multiple regions, following other studies using patent data (Le Gallo & Plunket, Citation2020; Whittle et al., Citation2020; van der Wouden, Citation2018). It measures the number of linkages (NL) inventors in a region have with inventors in other NUTS-2 regions.
The second indicator captures complementarities in interregional linkages. As discussed above, we expect that linkages that give access to additional capabilities in other regions that are related to existing local capabilities to have a stronger impact on regional diversification. We constructed a new variable called complementary interregional linkages (CL) that measures for each potential new technology i the extent to which a region r is linked with other regions s that are specialized in technologies j to which the new technology i is related, but that are missing in region r (i.e., in which region r is not specialized). In other words, this variable aims to capture relevant capabilities that are missing in a region but which might enhance the probability of the region to diversify into a new technology when the region has access to these relevant capabilities through co-inventor linkages with other regions.
It takes six steps to construct this variable CL for a region r and a technology i. The first step is to measure the RD around technology i in all regions s, as explained above. The second step is to determine for region r which technologies j are missing in region r (RTA < 1) to which technology i is related. The third step is to determine which regions s are specialized in these technologies j (RTA > 1) related to technology i that are missing in region r. The fourth step is to sum all RD scores around technology i for all regions s (as defined in the first step) that have a specialization in technologies j (RTA > 1) in which region r is not specialized (as defined in the third step). This is called RD added: it measures the amount of RD that can potentially be added by other regions to the RD of region r in that technology i because these regions are specialized in technologies j related to technology i that are missing in region r. The fifth step is to determine the number of co-inventor ties a region r has with each region s and multiply it with the RD added of each region s. Therefore, the more ties with another region, the more relevant the complementary capabilities from that other region will be for region r. The sixth step is to sum the scores in the fifth step for all regions s, so we obtain a total score of CL for region r in technology i, based on the RD added multiplied by the number of interregional ties.Footnote1 The score of CL will be higher, the lower the RD in region r and the more it is connected to regions that can add high amounts of RD to region r. The score of CL will be 0 when region r has a maximum of RD in technology i (so no need to connect to other regions), and when it has no interregional ties with regions that could potentially add RD to region r.
We take a fictional example. Assume that technology i is related to two technologies m and n, and that there are three regions r, s and t. Region r has an RTA in technology m, region s has no RTA in both technologies m and n, and region t has an RTA in both m and n. Region s will add 0% RD to region r (0/2), while region t will add 50% RD to region r in technology i (1/2). We then use information on the links (co-patents) between regions to see if region r actually collaborates or not with regions that can add RD. If region r actively collaborates with other regions that have a high potential of complementarity, CL will go up. Assume region r has 10 ties with region s, and five ties with region t. The CL (total) of region r around technology i will then be = 0*10 + 50*5 = 250. Therefore, the CL variable sums all RD added by other regions multiplied by the number of ties with these regions.
So far we defined the role of regional capabilities and interregional ties. We also include two control variables. Data are taken from Eurostat. First, we include gross domestic product (GDP) per capita to account for the level of economic development in a region. We expect the higher the GDP per capita, the higher the probability of a region to diversify. Second, we take the level of population (log) to account for different population sizes of regions. Again, we expect a positive effect. Third, we included time-fixed effects. Appendix A in the supplemental data online provides summary statistics for all variables.
MAIN FINDINGS: INTERREGIONAL TIES AND TECHNOLOGICAL DIVERSIFICATION IN REGIONS
presents the findings of the regional entry model. What can first be noticed in model 1 is that the coefficients of our control variables are positive and, in most cases, significant: population size and GDP per capita tend to increase the entry probability of new technologies in regions. Moreover, as expected, we find a positive effect of RD in all specifications. If the level of RD for a given technology in a given region increases by 10 points (+10%), the entry probability of this technology in this region during the next period increases by about 40% (0.004*10/0.1). This is a confirmation of earlier studies on the importance of relatedness for technological diversification of regions (Balland et al., Citation2019; Boschma et al., Citation2015; Kogler et al., Citation2013; Rigby, Citation2015).
Table 1. Diversification model.
How about interregional linkages once we control for this effect of regional capabilities? In model 2, we find a positive impact of the number of interregional connections (NL). Being connected to other regions shows a positive relationship with technological diversification. Therefore, our findings suggest that both regional capabilities and interregional linkages tend to contribute to the entry of new technologies in European regions.
In model 3, we add our new variable of complementarity in interregional linkages (CL). As expected, we found a strong and positive relationship: a new technology has a higher probability to enter a region when this region has connections with other regions that provide complementary (and thus relevant) capabilities concerning this new technology. Complementarity of interregional linkages is not only statistically but also economically significant. A 10% increase in the CL variable is associated with a 2.7% relative increase of the mean probability of a technology entering the region.Footnote2 What is also interesting to observe is that the positive effect of NL per se turns into a negative effect, once we control for CL. This might suggest that NL are costly: adding connections with other regions that are not relevant to the technological structure of regions comes with a price. Therefore, our findings tend to suggest that it matters to whom you connect (in terms of access to complementary capabilities), instead of being connected per se.
In model 4, we have added an interaction variable between local relatedness and complementary interregional ties (RD*CL) to test whether the two are substitutes or complements. A negative relationship might suggest that weak capabilities in a region (as reflected by a low RD) could be compensated by strong interregional linkages. Our findings show that the interaction effect is positive and significant: the higher the internal relatedness in a region is, the stronger the possible impact of external relatedness. Therefore, CL tend to reinforce, not weaken, the effect of regional capabilities on technological diversification in regions in Europe.Footnote3
This finding requires further exploration of the possible role of weak capabilities in regions in combination with interregional linkages. We take the example of peripheral regions, as scholars (e.g., Fitjar & Rodríguez-Pose, Citation2011; Grillitsch & Nilsson, Citation2015) have suggested that access to non-local capabilities is especially important for innovation in peripheral regions where strong and relevant capabilities are often found missing. We estimated the same diversification model as in , but added several variables for peripheral regions. For simplicity, we define peripheral regions as those that include all ‘less developed’ regions (regions with a GDP per capita < 75% of the EU average) and ‘transition’ regions (GDP per capita between 75% and 90%), following the criteria of EU Cohesion Policy. In the estimations reported in , we include the peripheral regions as a dummy variable.
Table 2. Diversification model: specification for peripheral regions
shows that peripheral regions tend to diversify less in new technologies. We then constructed variables interacting with Peripheral. We do not find evidence that the possible effect of regional capabilities (RD) is stronger or weaker in peripheral regions: the coefficient of the interaction term RD*Peripheral is positive but not significant. However, as expected, model 2 shows the possible effect of interregional linkages on diversification is stronger in peripheral regions. This is in line with literature stressing that peripheral regions tend to rely more on interregional linkages because their own capabilities might be weaker. Model specifications 3 and 4 in show there is also a positive and significant relationship with CL in peripheral regions. This is interesting because it suggests that while peripheral regions tend to diversify less in general, once they connect to regions that provide capabilities complementary to existing capabilities in peripheral regions, they will increase the probability of peripheral regions to diversify in new technologies.
INTERREGIONAL LINKAGES IN AN S3 FRAMEWORK
The question is how to incorporate this role of (complementary) interregional linkages in an S3 framework (Iacobucci & Guzzini, Citation2016). One way of doing that is to identify regions that face similar technological challenges and opportunities. Regions that would look more similar should then connect to exploit economies of scale and to learn from each other. One could calculate a technological similarity measure to identify regions with a similar technological structure: this measures how many technologies two regions have in common (e.g., Autant-Bernard, Citation2001; Moreno et al., Citation2005). However, similarity does not necessarily indicate complementarity. What we need instead is a complementarity measure that is technology-specific, so it can assess for each technology that a region wants to prioritize in its S3 which other regions can provide complementary capabilities. This measure can be used by European regions to identify regional strategic partnerships, given the capabilities in other regions.
This complementary indicator maps for each region to what extent other regions in Europe have complementary capabilities that are missing in the respective region. This is illustrated further in . The S3 of region A has the objective to develop a new technology i (such as biotech) that is highly complex. Assume biotech is related to 10 other technologies, and region A has strong expertise in five out of these 10 technologies, leading to an RD of 50%. Analysing the technological portfolio of other European regions would reveal that region B has strong expertise in three technologies related to biotech in which region A has no expertise. Therefore, region A could develop a strategy in which it connects to region B. Through the creation of this interregional link, the RD of region A would increase by 30%. Therefore, it would make sense for region A to connect to region B in its S3 because it would significantly increase its probability to diversify successfully into a new biotech activity.
Take another example, but this time based on real data. shows to which European regions the Île-de-France region (marked in black) could connect if it aims to develop new technologies in hydrogen as part of its S3. This requires the identification of those technologies that can be considered related to hydrogen technology, followed by an identification of regions in which these technologies related to hydrogen are concentrated in Europe. shows the potential increase of RD in the Île-de-France region concerning hydrogen technology if it would connect to other European regions. It shows that European regions such as the French Lorraine region, the Brussels region of Belgium and the German region of Dresden could be interesting strategic partners for Île-de-France to develop new hydrogen technologies, as these regions possess large amounts of complementary capabilities that are missing in Île-de-France. Then it is for Île-de-France to find out if it is already connected to these regions and how that works in practice. If any deficiencies in these interregional linkages do exist and the Île-de-France region could something about it, the region could consider making it part of its S3.
Figure 2. European regions with complementary capabilities for the Île-de-France region in new hydrogen technologies.
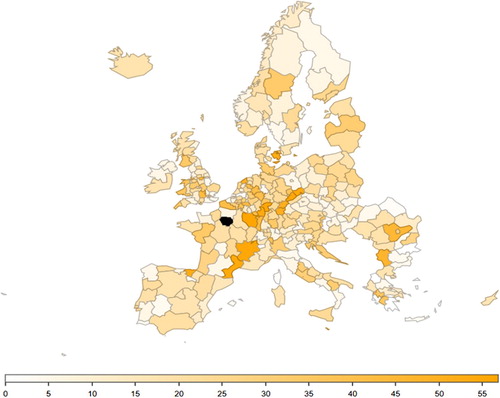
This complementarity measure enables regions to develop an S3 in which interregional linkages are incorporated. This measure helps to assess diversification opportunities of regions by identifying complementarities in capabilities across European regions. It aims to identify other regions that could act as strategic partners to develop new and more complex activities in a region as part of its S3. With this measure, it is possible to map for each technology (645 technologies) in each European region (292 NUTS regions) to what extent other regions in Europe have complementary capabilities that are missing in a region. This information is useful for regions to identify and select other regions as the most relevant strategic partners because of complementarities in their capabilities. This sheds a new light on the need to make connections between regions in Smart Specialisation policy: it makes the policy focus shift from making interregional connections per se to the exploitation of complementarities in other regions through the establishment of interregional linkages that give access to those.
CONCLUSIONS
Regional capabilities are regarded as a key pillar of Smart Specialisation policy in the EU, as they supply (related) internal capabilities in which a region can tap in to diversify into new activities and upgrade existing activities. However, there is yet little focus in S3 on the role of interregional linkages, and how to incorporate that in policy. A plausible reason is that there still is little understanding of how interregional linkages may give access to relevant capabilities in which a region can tap into to diversify, how they might affect the development of new activities in regions and to what extent these interregional linkages may compensate for a lack of relevant capabilities in regions. To fill this gap in the scientific and S3 policy literature, this paper estimated the possible effects of regional capabilities and interregional linkages on the ability of European regions to diversify into new technologies. We developed a new indicator of complementary interregional linkages (CL) that accounts for the fact that regions require absorptive capacity to exploit and benefit from the inflow of external knowledge through interregional linkages. This indicator aims to capture the impact of co-inventor linkages with other regions that provide access to relevant capabilities that are missing in a region, and which could increase its ability to diversify into a new technology.
The main findings can be summarized as follows. First, we find a positive relationship between interregional linkages and the probability of regions to diversify, on top of regional capabilities. This is especially true for interregional linkages that give access to capabilities not present in the region but related to existing capabilities in the region. In other words, a new technology has a higher probability to enter a region when this region has connections with other regions that provide complementary capabilities for this new technology. Second, the study has shown that it matters to whom a region connects in terms of access to complementary capabilities, while just being connected to regions does not seem to matter. This is in line with the philosophy of S3 policy that focuses on relevant capabilities, but also extends it to the targeting of interregional linkages that provide access to complementary capabilities. Third, we find that these CL tend to strengthen, not weaken the effect of regional capabilities on the ability of regions to enter new technologies. This seems to imply that having no capabilities whatsoever, or having very weak capabilities in a region, cannot be compensated for by connecting to other regions, even when interregional linkages would give a region access to relevant (i.e., complementary) capabilities. Fourth, our analysis shows that peripheral regions tend to diversify less in new technologies. However, once peripheral regions connect to regions that provide capabilities that are complementary to their own capabilities, we found this increased the capacity of peripheral regions to develop new technologies. Finally, we constructed an indicator that can map for each region and for each possible technology the extent to which other regions in Europe have complementary capabilities that are missing in a region. This information is useful for a region because it gives an additional rationale to select and connect to specific regions as part of its S3, given the availability of complementary capabilities in other regions. Doing so, the policy focus of Smart Specialisation shifts from making interregional connections per se, to the exploitation of complementarities available in other regions through interregional linkages.
Needless to say, this paper also has some limitations. First, there are the usual caveats of using patent data to estimate diversification potentials in regions. This applies especially to peripheral or less urbanized regions where other types of capabilities in low- and medium-tech may be more important for diversification. This requires additional analyses using data on industries or occupations that have proven to be useful to identify diversification potentials in peripheral regions (Balland & Boschma, Citation2019). These data can be used to conduct a similar analysis on the role of interregional linkages for regional diversification, using trade data (Boschma & Iammarino, Citation2009) or labour flow data (Boschma et al., Citation2009), for instance.
Second, when looking for other regions as strategic partners in S3, it could also be relevant, besides complementary capabilities, to take into consideration other factors, such as geographical or cultural proximity. That is, once a set of regions has been identified based on their complementary capabilities, a region might prefer to connect to regions that are geographically near, belong to the same country (so national policy instruments could be adopted), or that share the same language or similar norms and values. We see our paper as a first step to analyse in more detail how other forms of proximity besides cognitive proximity may affect the selection of other regions as potential partners in Smart Specialisation policy.
Our study did not look at the role of national and regional institutions. Weak institutions, such as low quality of government, or bonding social capital, could impact negatively on the diversification opportunities of regions, especially in peripheral regions (Cortinovis et al., Citation2017; Rodríguez-Pose & Di Cataldo, Citation2015). Therefore, even when the right regional capabilities and interregional linkages would be in place, a weak institutional structure might still prevent a region to diversify in new and promising activities. Moreover, one has to take into consideration that the degree of political autonomy of regions differs between and within countries in Europe to a considerable degree (Muringani et al., Citation2019). In Germany, for example, political power is more concentrated at the NUTS-1 level (Länder) rather than the NUTS-2 regions (Regierungsbezirke). This may have implications for the implementation of Smart Specialisation policy more in general, and for the capacity of regions to link to other regions in particular.
Finally, there is a need to explore how the role of interregional linkages can be incorporated in the design and implementation of S3 (Barzotto et al., Citation2019; Iacobucci & Guzzini, Citation2016; Santoalha, Citation2019; Sörvik et al., Citation2016; Uyarra et al., Citation2014), and what kind of policy instruments might be effective to exploit interregional linkages to enhance diversification in European regions (Bednarz & Broekel, Citation2019; Uyarra et al., Citation2018). In this paper, we restricted our attention to the selection of regions that could act as strategic partners in Smart Specialisation policy, based on their complementary capabilities. The next step is to think about the design and implementation of S3 once potential partners have been identified. There might be many mechanisms through which policy could facilitate interregional linkages, such as publicly funded collaborative research projects in which partners in different regions are selected based on their complementarities (Uhlbach et al., Citation2017). This also requires considerations in S3 of how to cope with perceived barriers to collaborations between regions in Europe (Uyarra et al., Citation2018).
Supplemental Material
Download PDF (44 KB)DISCLOSURE STATEMENT
No potential conflict of interest was reported by the authors.
Additional information
Funding
Notes
1. An option is to divide this by the total number of interregional ties region r has, so we derive a CL score that takes the average of the RD added per interregional tie. We have used this alternative CL variable in the estimations, and the findings remain the same.
2. The coefficients provided in indicate the impact of a 1-unit change on the probability of entry, and the CL variable is expressed in a logarithmic form. A 1-unit change of the log-transformed variable therefore indicates the average change in entry probability when the raw variable increases by 170%. A change in the log of 1 corresponds to a 170% increase in the raw variable, that is, multiplied by 2.7. For instance, log(1000) = 6.9 and log(2700) = 7.9. Since the mean of entry is equal to 0.1, we can say that a 170% increase in the CL variable can be associated with a 27% (0.027/0.1) relative increase of the mean probability of a technology entering the region. One should keep in mind the non-linear properties of the log-transformation. Doubling CL for instance – that is, +0.7 in the log – is associated with a 18.9% (0.7*0.027/0.1) relative increase. Since the log of 0.1 corresponds to a 10% increase in the raw variable – that is, log(1000) = 6.9 and log(1100) = 7 – a 10% increase in the CL variable is associated with a 2.7% (0.1*0.027/0.1) relative increase of the mean probability of a technology entering the region.
3. As a robustness check, we split the period in two subperiods: pre-crisis (1992–2006) and post-crisis (2007–16). We rerun all models for the two periods separately. As shown in Appendix A in the supplemental data online, the main results remain the same. We also estimated zero-inflated negative binomial models that generated the same results, with the exception of the interaction term which was not significant anymore. For replication purposes, we followed other studies in the regional diversification literature that define regional entry as new specialization, based on RTA > 1. As a robustness check, we also ran the same estimations, defining regional entry as a new specialization, based on RTA > 2. In these same estimations, we also used this new threshold of RTA > 2 in the construction of our key variables RD and CL. The findings for our key variables remained the same.
REFERENCES
- Andersson, M., Bjerke, L., & Karlsson, C. (2013). Import flows: Extraregional linkages stimulating renewal of regional sectors? Environment and Planning A: Economy and Space, 45(12), 2999–3017. https://doi.org/10.1068/a45732
- Ascani, A., Bettarelli, L., Resmini, L., & Balland, P. A. (2020). Global networks, local specialization and regional patterns of innovation (Papers in Evolutionary Economic Geography (PEEG) No. 20.02). Utrecht University, Department of Human Geography and Spatial Planning.
- Asheim, B. T., & Isaksen, A. (2002). Regional innovation systems: The integration of local ‘sticky’ and global ‘ubiquitous’ knowledge. Journal of Technology Transfer, 27(1), 77–86. https://doi.org/10.1023/A:1013100704794
- Autant-Bernard, C. (2001). The geography of knowledge spillovers and technological proximity. Economics of Innovation and New Technology, 10(4), 237–254. https://doi.org/10.1080/10438590100000010
- Bahar, D., Hausmann, R., & Hidalgo, C. A. (2014). Neighbors and the evolution of the comparative advantage of nations: Evidence of international knowledge diffusion? Journal of International Economics, 92(1), 111–123. https://doi.org/10.1016/j.jinteco.2013.11.001
- Balland, P. A., & Boschma, R. (2019). Smart Specialization: Beyond patents. European Commission, DG Regional and Urban Policy.
- Balland, P. A., Boschma, R., Crespo, J., & Rigby, D. (2019). Smart Specialization policy in the EU: Relatedness, knowledge complexity and regional diversification. Regional Studies, 53(9), 1252–1268. https://doi.org/10.1080/00343404.2018.1437900
- Balland, P. A., Jara-Figueroa, C., Petralia, S. G., Steijn, M. P., Rigby, D. L., & Hidalgo, C. A. (2020). Complex economic activities concentrate in large cities. Nature Human Behaviour, 4, 248–254.
- Balland, P. A., & Rigby, D. (2017). The geography of complex knowledge. Economic Geography, 93(1), 1–23. https://doi.org/10.1080/00130095.2016.1205947
- Barzotto, M., Corradini, C., Fai, F. M., Labory, S., & Tomlinson, P. R. (2019). Enhancing innovative capabilities in lagging regions: An extra-regional collaborative approach to RIS3. Cambridge Journal of Regions, Economy and Society, 12(2), 213–232. https://doi.org/10.1093/cjres/rsz003
- Bathelt, H., Malmberg, A., & Maskell, P. (2004). Clusters and knowledge: Local buzz, global pipelines and the process of knowledge creation. Progress in Human Geography, 28(1), 31–56. https://doi.org/10.1191/0309132504ph469oa
- Bednarz, M., & Broekel, T. (2019). The relationship of policy induced R&D networks and inter-regional knowledge diffusion. Journal of Evolutionary Economics, 29(5), 1459–1481. https://doi.org/10.1007/s00191-019-00621-2
- Blažek, J. (2016). Towards a typology of repositioning strategies of GVC/GPN suppliers: The case of functional upgrading and downgrading. Journal of Economic Geography, 16(4), 849–869. https://doi.org/10.1093/jeg/lbv044
- Boschma, R. (2017). Relatedness as driver of regional diversification: A research agenda. Regional Studies, 51(3), 351–364. https://doi.org/10.1080/00343404.2016.1254767
- Boschma, R., Balland, P. A., & Kogler, D. (2015). Relatedness and technological change in cities: The rise and fall of technological knowledge in US metropolitan areas from 1981 to 2010. Industrial and Corporate Change, 24(1), 223–250. https://doi.org/10.1093/icc/dtu012
- Boschma, R., Eriksson, R., & Lindgren, U. (2009). How does labour mobility affect the performance of plants? The importance of relatedness and geographical proximity. Journal of Economic Geography, 9(2), 169–190. https://doi.org/10.1093/jeg/lbn041
- Boschma, R., Heimeriks, G., & Balland, P. A. (2014). Scientific knowledge dynamics and relatedness in biotech cities. Research Policy, 43(1), 107–114. https://doi.org/10.1016/j.respol.2013.07.009
- Boschma, R., Martin, V., & Minondo, A. (2017). Neighbour regions as the source of new industries. Papers in Regional Science, 96(2), 227–245. https://doi.org/10.1111/pirs.12215
- Boschma, R. A., & Iammarino, S. (2009). Related variety, trade linkages and regional growth. Economic Geography, 85(3), 289–311. https://doi.org/10.1111/j.1944-8287.2009.01034.x
- Boschma, R. A., & Lambooy, J. G. (1999). Evolutionary economics and economic geography. Journal of Evolutionary Economics, 9(4), 411–429. https://doi.org/10.1007/s001910050089
- Brennan, L., & Rakhmatullin, R. (2018). Transnationalizing Smart Specialization Strategy. In S. Radosevic, A. Curaj, R. Gheorghiu, L. Andreescu, & I. Wade (Eds.), Advances in the theory and practice of Smart Specialization (pp. 250–269). Academic Press.
- Breschi, S., & Lenzi, C. (2015). The role of external linkages and gatekeepers for the renewal and expansion of US cities’ knowledge base, 1990–2004. Regional Studies, 49(5), 782–797. https://doi.org/10.1080/00343404.2014.954534
- Broekel, T., & Mueller, W. (2018). Critical links in knowledge networks – What about proximities and gatekeeper organisations? Industry and Innovation, 25(10), 919–939. https://doi.org/10.1080/13662716.2017.1343130
- Camagni, R. (Ed.). (1991). Innovation networks: Spatial perspectives. Belhaven.
- Cortinovis, N., Xiao, J., Boschma, R., & van Oort, F. (2017). Quality of government and social capital as drivers of regional diversification in Europe. Journal of Economic Geography, 17(6), 1179–1208. https://doi.org/10.1093/jeg/lbx001
- Crespo, J., Suire, R., & Vicente, J. (2014). Lock-in or lock-out? How structural properties of knowledge networks affect regional resilience. Journal of Economic Geography, 14(1), 199–219. https://doi.org/10.1093/jeg/lbt006
- D’Adda, D., Iacobucci, D., & Palloni, R. (2019). Relatedness in the implementation of Smart Specialisation Strategy: A first empirical assessment. Papers in Regional Science, https://doi.org/10.1002/pirs.12492
- De Noni, I., Ganzaroli, A., & Orsi, L. (2017). The impact of intra- and inter-regional knowledge collaboration and technological variety on the knowledge productivity of European regions. Technological Forecasting and Social Change, 117, 108–118. https://doi.org/10.1016/j.techfore.2017.01.003
- De Noni, I., Orsi, L., & Belussi, F. (2018). The role of collaborative networks in supporting the innovation performances of lagging-behind European regions. Research Policy, 47(1), 1–13. https://doi.org/10.1016/j.respol.2017.09.006
- Eriksson, R., & Lengyel, B. (2019). Co-worker networks and agglomeration externalities. Economic Geography, 95(1), 65–89. https://doi.org/10.1080/00130095.2018.1498741
- Fitjar, R. D., & Rodríguez-Pose, A. (2011). When local interaction does not suffice: Sources of firm innovation in urban Norway. Environment and Planning A: Economy and Space, 43(6), 1248–1267. https://doi.org/10.1068/a43516
- Foray, D. (2015). Smart Specialisation: Opportunities and challenges for regional innovation policy. Routledge.
- Foray, D., David, P. A., & Hall, B. H. (2009). Smart Specialization. The concept (Knowledge Economists Policy Brief No. 9, June). European Commission.
- Gereffi, G., Humphrey, J., & Sturgeon, T. (2005). The governance of global value chains. Review of International Political Economy, 12(1), 78–104. https://doi.org/10.1080/09692290500049805
- Gertler, M. S. (2003). Tacit knowledge and the economic geography of context, or the undefinable tacitness of being (there). Journal of Economic Geography, 3, 75–99. doi:10.1093/jeg/3.1.75
- Giuliani, E., & Bell, M. (2005). The micro-determinants of meso-level learning and innovation: Evidence from a Chilean wine cluster. Research Policy, 34(1), 47–68. https://doi.org/10.1016/j.respol.2004.10.008
- Grabher, G. (1993). The weakness of strong ties – The lock-in of regional development in the Ruhr area. In G. Grabher (Ed.), The embedded firm (pp. 255–277). Routledge.
- Graf, H. (2011). Gatekeepers in regional networks of innovators. Cambridge Journal of Economics, 35(1), 173–198. https://doi.org/10.1093/cje/beq001
- Grillitsch, M., & Nilsson, M. (2015). Innovation in peripheral regions: Do collaborations compensate for a lack of local knowledge spillovers? Annals of Regional Science, 54(1), 299–321. https://doi.org/10.1007/s00168-014-0655-8
- Hassink, R. (2005). How to unlock regional economies from path dependency? From learning region to learning cluster. European Planning Studies, 13(4), 521–535. https://doi.org/10.1080/09654310500107134
- Henn, S. (2013). Transnational entrepreneurs and the emergence of clusters in peripheral regions: The case of the diamond cutting cluster in Gujarat (India). European Planning Studies, 21(11), 1779–1795. https://doi.org/10.1080/09654313.2012.753690
- Hesse, K., & Fornahl, D. (2020). Essential ingredients for radical innovations? The role of (un-)related variety and external linkages in Germany (Papers in Evolutionary Economic Geography (PEEG) No. 20.07). Utrecht University, Department of Human Geography and Spatial Planning.
- Hidalgo, C., Balland, P. A., Boschma, R., Delgado, M., Feldman, M., Frenken, K., Glaeser, E., He, C., Kogler, D., Morrison, A., Neffke, F., Rigby, D., Stern, S., Zheng, S., & Zhu, S. (2018). The principle of relatedness. In Springer proceedings in complexity (pp. 451–457). Springer. Paper originally presented at the International Conference on Complex Systems (ICCS), Cambridge, MA, USA, July 22–27 2018.
- Hidalgo, C. A., Klinger, B., Barabasi, A. L., & Hausmann, R. (2007). The product space conditions the development of nations. Science, 317(5837), 482–487. https://doi.org/10.1126/science.1144581
- Iacobucci, D., & Guzzini, E. (2016). Relatedness and connectivity in technological domains: Missing links in S3 design and implementation. European Planning Studies, 24(8), 1511–1526. https://doi.org/10.1080/09654313.2016.1170108
- Jaffe, A. B., Trajtenberg, M., & Henderson, R. (1993). Geographic localization of knowledge spillovers as evidenced by patent citations. Quarterly Journal of Economics, 108(3), 577–598. https://doi.org/10.2307/2118401
- Kogler, D. F., Rigby, D. L., & Tucker, I. (2013). Mapping knowledge space and technological relatedness in US cities. European Planning Studies, 21(9), 1374–1391. https://doi.org/10.1080/09654313.2012.755832
- Le Gallo, J., & Plunket, A. (2020). Regional gatekeepers, inventor networks and inventive performance: Spatial and organizational channels. Research Policy. https://doi.org/10.1016/j.repolx.2020.100007
- Los, B., Lankhuizen, M., & Thissen, M. (2017). New measures of regional competitiveness in a globalizing world. In P. McCann, F. van Oort, & J. Goddard (Eds.), The empirical and institutional dimensions of Smart Specialisation (pp. 105–126). Routledge.
- MacKinnon, D., Dawley, S., Steen, M., Menzel, M. P., Karlsen, A., Sommer, P., Hopsdal Hansen, G., & Endresen Normann, H. (2018). Path creation, global production networks and regional development: A comparative international analysis of the offshore wind sector (Papers in Evolutionary Economic Geography (PEEG) No. 18.10). Utrecht University, Department of Human Geography and Spatial Planning.
- Maskell, P., & Malmberg, A. (1999). The competitiveness of firms and regions: Ubiquitification and the importance of localized learning. European Urban and Regional Studies, 6(1), 9–25. https://doi.org/10.1177/096977649900600102
- Maskell, P., & Malmberg, A. (2007). Myopia, knowledge development and cluster evolution. Journal of Economic Geography, 7(5), 603–618. https://doi.org/10.1093/jeg/lbm020
- McCann, P., & Ortega-Argilés, R. (2015). Smart Specialization, regional growth and applications to European Union Cohesion Policy. Regional Studies, 49(8), 1291–1302. https://doi.org/10.1080/00343404.2013.799769
- Miguelez, E., & Moreno, R. (2015). Knowledge flows and the absorptive capacity of regions. Research Policy, 44(4), 833–848. https://doi.org/10.1016/j.respol.2015.01.016
- Miguelez, E., & Moreno, R. (2018). Relatedness, external linkages and regional innovation in Europe. Regional Studies, 52(5), 688–701. https://doi.org/10.1080/00343404.2017.1360478
- Moreno, R., Paci, R., & Usai, S. (2005). Spatial spillovers and innovation activity in European regions. Environment and Planning A: Economy and Space, 37(10), 1793–1812. https://doi.org/10.1068/a37341
- Morrison, A. (2008). Gatekeepers of knowledge within industrial districts: Who they are, how they interact? Regional Studies, 42(6), 817–835. https://doi.org/10.1080/00343400701654178
- Morrison, A., Rabellotti, R., & Zirulia, L. (2013). When do global pipelines enhance the diffusion of knowledge in clusters? Economic Geography, 89(1), 77–96. https://doi.org/10.1111/j.1944-8287.2012.01167.x
- Muneepeerakul, R., Lobo, J., Shutters, S. T., Gomez-Lievano, A., & Qubbaj, M. R. (2013). Urban economies and occupation space: Can they get ‘there’ from ‘here’? PLoS One, 8(9), e73676. https://doi.org/10.1371/journal.pone.0073676
- Muringani, J., Fitjar, R. D., & Rodríguez-Pose, A. (2019). Decentralisation, quality of government and economic growth. Revista de Economía Mundial, 51, 25–50.
- Neffke, F., Henning, M., & Boschma, R. (2011). How do regions diversify over time? Industry relatedness and the development of new growth paths in regions. Economic Geography, 87(3), 237–265. https://doi.org/10.1111/j.1944-8287.2011.01121.x
- Nelson, R. R., & Winter, S. G. (1982). An evolutionary theory of economic change. Belknap.
- Nooteboom, B. (2000). Learning and innovation in organizations and economies. Oxford University Press.
- Radosevic, S., & Ciampi Stankova, K. (2015). External dimensions of Smart Specialization. Opportunities and challenges for trans-regional and transnational collaboration in the EU-13 (Joint Research Centre S3 Working Paper Series No. JRC96030).
- Radosevic, S., Curaj, A., Gheorghiu, R., Andreescu, L., & Wade, I. (Eds.). (2018). Advances in the theory and practice of Smart Specialization. Academic Press.
- Rigby, D. (2015). Technological relatedness and knowledge space: Entry and exit of US cities from patent classes. Regional Studies, 49(11), 1922–1937. https://doi.org/10.1080/00343404.2013.854878
- Rodríguez-Pose, A., & Di Cataldo, M. (2015). Quality of government and innovative performance in the regions of Europe. Journal of Economic Geography, 15(4), 673–706. https://doi.org/10.1093/jeg/lbu023
- Rodríguez-Pose, A., & Wilkie, C. (2019). Innovating in less developed regions: What drives patenting in the lagging regions of Europe and North America. Growth and Change, 50(1), 4–37. https://doi.org/10.1111/grow.12280
- Santoalha, A. (2019). Technological diversification and Smart Specialisation: The role of cooperation. Regional Studies, 53(9), 1269–1283. https://doi.org/10.1080/00343404.2018.1530753
- Simon, H. A. (1955). A behavioral model of rational choice. Quarterly Journal of Economics, 6, 99–118.
- Sörvik, J., Midtkandal, I., Marzocchi, C., & Uyarra, E. (2016). How outward looking is Smart Specialization? Results from a survey on inter-regional collaboration in Smart Specialisation Strategies, S3 (Policy Brief Series No. 16/2016, EUR 27795 EN). Publications Office of the European Union. https://doi.org/10.2791/943671
- Tavassoli, S., & Carbonara, N. (2014). The role of knowledge variety and intensity for regional innovation. Small Business Economics, 43(2), 493–509. https://doi.org/10.1007/s11187-014-9547-7
- Ter Wal, A., & Boschma, R. (2011). Co-evolution of firms, industries and networks in space. Regional Studies, 45(7), 919–933. https://doi.org/10.1080/00343400802662658
- Thissen, M., van Oort, F., Diodato, D., & Ruijs, A. (2013). Regional competitiveness and Smart Specialization in Europe: Place-based development in international economic networks. Edward Elgar.
- Trippl, M., Grillitsch, M., & Isaksen, A. (2018). Exogenous sources of regional industrial change: Attraction and absorption of non-local knowledge for new path development. Progress in Human Geography, 42(5), 687–705. https://doi.org/10.1177/0309132517700982
- Uhlbach, W. H., Balland, P., & Scherngell, T. (2017). R&D policy and technological trajectories of regions: Evidence from the EU framework programmes (Papers in Evolutionary Economic Geography (PEEG) No. 17.22). Utrecht University, Section Economic Geography, Utrecht.
- Uyarra, E., Marzocchi, C., & Sorvik, J. (2018). How outward looking is Smart Specialisation? Rationales, drivers and barriers. European Planning Studies, 26(12), 2344–2363. https://doi.org/10.1080/09654313.2018.1529146
- Uyarra, E., Sorvik, J., & Midtkandal, I. (2014). Inter-regional collaboration in research and innovation strategies for Smart Specialisation (JRC Technical Reports, S3 Working Paper Series No. 6/2014). IPTS.
- Vale, M., & Carvalho, L. (2013). Knowledge networks and processes of anchoring in Portuguese biotechnology. Regional Studies, 47(7), 1018–1033. https://doi.org/10.1080/00343404.2011.644237
- Van der Wouden, F. (2018). Exploring the changing structures of inventor collaboration in US cities between 1836 and 1975. PhD thesis, UCLA.
- Varga, A., Sebestyen, T., Szabo, N., & Szerb, L. (2020). Estimating the economic impacts of knowledge network and entrepreneurship development in Smart Specialization policy. Regional Studies, 54(1), 48–59. https://doi.org/10.1080/00343404.2018.1527026
- Vicente, J. (2018). Economics of clusters: A brief history of cluster theories and policy. Palgrave Pivot.
- Vicente, J., Balland, P. A., & Brossard, O. (2011). Getting into networks and clusters: Evidence from the Midi-Pyrenean Global Navigation Satellite Systems (GNSS) collaboration network. Regional Studies, 45(8), 1059–1078. https://doi.org/10.1080/00343401003713340
- Whittle, A., Lengyel, B., & Kogler, D. F. (2020). Understanding regional branching knowledge diversification via inventor collaboration networks (Papers in Evolutionary Economic Geography (PEEG) No. 20.06). Utrecht University, Department of Human Geography and Spatial Planning.