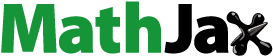
ABSTRACT
This paper aims to identify the future Industry 4.0 technology (I4T) centres of knowledge production in Europe. We expect I4Ts to thrive in regions where they can draw on local capabilities in I4T-related technologies. We use patent data to identify I4T-related technologies and find that I4Ts are positioned in the periphery of the knowledge space. The study shows that European regions with a high potential in terms of I4T-related technologies are more likely to diversify successfully in new I4Ts. We find huge differences across regions: some show high, but most regions show weak I4T potential.
INTRODUCTION
In the last two centuries, Europe has been subject to three industrial revolutions. These transformative periods opened new windows of opportunities of economic growth and prosperity, leading to the rise of new economic leaders (Perez & Soete, Citation1988). These revolutions led to major transformations in the geography of knowledge and innovation (Hall & Preston, Citation1988; Marshall, Citation1987). The Third Industrial Revolution led to the emergence of the Sunbelt states (and Silicon Valley, in particular) and the decline of manufacturing regions in the Rust Belt states in the United States (Lecuyer, Citation2006; Markusen et al., Citation1986; Scott, Citation1988). Europe underwent similar regional dynamics, where the South East in the UK and Bavaria in Germany presented themselves as the new centres of excellence and innovation.
Scholars have argued that we are currently undergoing a Fourth Industrial Revolution associated with Industry 4.0 (I4) (Liao et al., Citation2017; Lu, Citation2017; Ménière et al., Citation2017; Popkova et al., Citation2019). Key technologies such as cyber-physical systems, the Internet of Things (IoT), robotics and artificial intelligence (AI) are expected to affect a wide range of sectors. For instance, studies show that a broad range of jobs and job tasks may be automated, including non-routine tasks that use, for instance, big data to diagnose diseases or selecting job applicants for interviews. A lot of attention goes to possible negative effects, such as in the literature on automation that focuses on the types of jobs that might be displaced or under threat (e.g., Acemoglu & Restrepo, Citation2019; Autor, Citation2015; Bessen et al., Citation2019; Frey & Osborne, Citation2016).
I4 is expected to have effects on the geography of knowledge production and innovation in Europe. Scholars suggest that Industry 4.0 technologies (I4Ts) will open new windows of opportunities for regions, create new regional leaders and has the potential to shift the geography of knowledge production in Europe (Gotz & Jankowska, Citation2017; Gress & Kalafsky, Citation2015; Strange & Zucchella, Citation2017). Case studies of regions show how I4 is transforming local productive systems in some parts of Europe (Bellandi et al., Citation2020; De Propris & Bailey, Citation2020). A few papers indicate that the geography of I4T is highly uneven in Europe. Ciffolilli and Muscio (Citation2018) and Muscio and Ciffolilli (Citation2020) show the uneven participation of NUTS-2 regions in collaborative I4 research projects, while Ménière et al. (Citation2017) used patent applications to identify the top EU regions in I4T.
The main objective of the paper is to identify regions in Europe that have the potential to participate in new knowledge production in I4Ts. The novelty of the paper is that we use the relatedness framework (Boschma, Citation2017; Hidalgo et al., Citation2018) that has been proven useful to explain the potential of regions to diversify successfully in new industries (Neffke et al., Citation2011), new technologies (Rigby, Citation2015) and new jobs (Muneepeerakul et al., Citation2013). First, we use patent data from the Organisation for Economic Co-operation and Development’s (OECD) REGPAT database (March 2018 edition) and perform co-occurrence analysis to identify I4T-related technologies, that is, technologies that are frequently combined with I4Ts. This enables us to draw a knowledge space, a network representation that shows the relatedness between I4Ts and other technology fields. This study shows that I4Ts are not positioned in the core of the knowledge space. Some I4Ts are closely related to each other, while others are not.
Second, we use the knowledge space to estimate the impact of relatedness on the entry of I4Ts in 295 NUTS-2 regions in Europe. This helps us to understand to what extent the knowledge base of regions impacts their likelihood to become leaders in knowledge production in I4Ts. Our findings show that the local presence of I4-related technologies has a positive impact on regions to develop a new specialization in I4 technologies over the period 2002–16.
Third, we use the information of the geography of I4-related technologies in Europe to map the potentials of all European regions to become technological centres of knowledge production in I4.
The remainder of the paper is structured as follows. The next section gives a brief overview of the literature on I4Ts and its geography. The third section provides an analytical framework based on the principle of relatedness to identify the potential of regions to diversify into I4Ts, and it presents the I4 knowledge space. The fourth section presents a regional diversification model in Europe that estimates the impact of relatedness on the entry of I4Ts in EU regions. The fifth section maps the future I4 centres of knowledge production in Europe. The sixth section concludes.
INDUSTRY 4.0 TECHNOLOGIES AND THEIR GEOGRAPHY
New digital technologies around the IoT, robotics, AI and self-driving cars are expected to have a pervasive effect on a wide range of sectors (Liao et al., Citation2017; Lu, Citation2017). While previous industrial revolutions have been associated with the increasing automation of repetitive physical work, I4Ts are about the large-scale automation of entire groups of tasks, including repetitive intellectual or non-routine tasks (Ménière et al., Citation2017). Studies have attempted to assess the possible negative consequences of automation for jobs and workers, especially in terms of the number and types of jobs and the particular work tasks that are expected to be displaced or under threat. This has led to fierce and ongoing debates among scholars about the right empirical approach to assess the effect of automation on the labour market, initiated by a fundamental critique on the method proposed by Frey and Osborne (Citation2016) (Bessen et al., Citation2019; Nedelkoska & Quintini, Citation2018).
In the extensive literature on I4, there exists no consensus on what I4T stands for (Alcacer & Cruz-Machado, Citation2019; Chiarello et al., Citation2018; Dallasega et al., Citation2018; Kamble et al., Citation2018; Liao et al., Citation2017; Lu, Citation2017; Oztemel & Gursev, Citation2020; Popkova et al., Citation2019; Valdes & Ilja Rudyk, Citation2017; Xu et al., Citation2018). There is no formal, well-accepted and easy-to-apply classification of I4 activities. Some scholars employ a broad definition of I4Ts. Here, I4T stands for the massive deployment of the IoT, in which objects with computing capabilities that are connected to a data network operate autonomously, based on their own data collection, or through data exchange with other objects. Ménière et al. (Citation2017), for instance, distinguish three activities in which I4Ts are found: (1) core technologies (hardware, software, connectivity); (2) enabling technologies (analytics, security, AI, position determination, power supply, 3D systems, user interfaces); and (3) application domains (home, personal, enterprise, manufacturing, infrastructure, vehicles). Other scholars use a narrower definition, such as Ciffolilli and Muscio (Citation2018), in which I4 is connected to smart factories in which new digital and other technologies transform value chains. Here, the focus is on the digitization of manufacturing industries that gets integrated with new information and communication technologies (ICTs).
No matter what definition is used, scholars tend to agree that I4Ts will have an impact of the geography of knowledge production (Ciffolilli & Muscio, Citation2018; Ménière et al., Citation2017), the geography of manufacturing (Gress & Kalafsky, Citation2015; Muro et al., Citation2019), and the location and organization of activities within value chains (Strange & Zucchella, Citation2017). Case studies of regions show how I4 is transforming local productive systems (Bellandi et al., Citation2020; De Propris & Bailey, Citation2020). Muro et al. (Citation2019) found there are differences in the United States between metropolitan areas and between rural and urban communities with regard to their exposure to automation. A few papers indicate that the geography of I4T is highly uneven in Europe. Ciffolilli and Muscio (Citation2018) examined collaborative research in I4 in 294 NUTS-2 regions and found it is highly concentrated in regions in south Germany (Baden-Württemberg and Bavaria), North Rhine-Westphalia, Rhône-Alpes, Île-de-France and Lombardia. Eastern and Southern Europe participate in I4 collaborative research only to a limited extent. Ménière et al. (Citation2017) used data on patent applications to identify the location of I4Ts in Europe. They found that the top I4T regions are also ranked high as top EU regions of the Third Industrial Revolution.
Many regions have expressed the ambition to participate fully in I4Ts (Reischauer, Citation2018; Santos et al., Citation2017). However, there is little understanding which regions have actually the potential to diversify into and develop the new technologies of the Fourth Industrial Revolution. This begs the question: What capabilities do regions need to diversify successfully in I4 technologies? The regional diversification literature (Hidalgo et al., Citation2007; Neffke et al., Citation2011) claims that regional capabilities condition which new activities will be feasible to develop in regions, and which ones will not: regions are more likely to develop new activities related to their existing activities. This insight has been replicated in empirical studies (Boschma, Citation2017). Neffke et al. (Citation2011) found that an industry is more likely to enter a region when technologically related to pre-existing industries in that region. Similar findings have been reported for NUTS-2 regions in the EU (Cortinovis et al., Citation2017), and for prefectures in China (Guo & He, Citation2017; He et al., Citation2018).
Scholars have used patent data to investigate the potential of regions to diversify successfully in new technologies. Rigby (Citation2015) and Boschma et al. (Citation2015) used measures of relatedness between patent classes to describe the rise of new technologies in US cities. They constructed a knowledge space to determine relatedness between technologies that is based either on co-occurrence of technology classes on a patent document, or on citations across technology classes. Studies find systematic evidence that technologies related to pre-existing technologies had a higher probability to enter metropolitan regions in the United States (Boschma et al., Citation2015; Kogler et al., Citation2013; Rigby, Citation2015) and NUTS-2 regions in the EU (Balland et al., Citation2019). The thesis that new technologies do not start from scratch has also been confirmed for specific technologies, such as green technologies (Corradini, Citation2019; Montresor & Quatraro, Citation2019; Santoalha & Boschma, Citation2021; van den Berge & Weterings, Citation2014), renewable energy technologies (Li, Citation2020), fuel cell technology (Tanner, Citation2016), nanotechnologies (Colombelli et al., Citation2014) and biotechnologies (Boschma et al., Citation2014). Without exception, these studies confirm the importance of the principle of relatedness (Hidalgo et al., Citation2018). However, this has not yet been tested for regional diversification into I4Ts.
This paper builds on the related diversification framework to identify potentials of regions to diversify and participate in I4Ts. We examine whether the ability of European regions to develop new technologies associated with I4 is depending on capabilities related to their pre-existing technological specializations. We test the extent to which I4Ts build on existing local capabilities, or whether they develop from scratch. We then use the relatedness framework to identify the future potentials of European regions to develop I4Ts by looking at the extent to which they possess the required capabilities to do so.
MAPPING THE INDUSTRY 4.0-KNOWLEDGE SPACE
To map the geography of I4T potentials requires the identification of the key technologies of I4. As outlined above, this is a daunting task and a huge challenge because there is no widely agreed and well-accepted classification of I4 activities. For instance, Ciffolilli and Muscio (Citation2018) investigated the Seventh Framework Programs of the EU and identified eight enabling technologies linked to I4. Their classification of I4T’s builds on a taxonomy of key research areas based on expert peer reviews. Chiarello et al. (Citation2018) identified a total of eight clusters of I4Ts using Wikipedia data.
To determine the major technologies of I4, we examined key references on I4, most of which are literature reviews of I4 in specialized technology journals (Alcacer & Cruz-Machado, Citation2019; Chiarello et al., Citation2018; Ciffolilli & Muscio, Citation2018; Dallasega et al., Citation2018; Kamble et al., Citation2018; Liao et al., Citation2017; Lu, Citation2017; Ménière et al., Citation2017; Oztemel & Gursev, Citation2020; Popkova et al., Citation2019; Reischauer, Citation2018; Santos et al., Citation2017; Valdes & Ilja Rudyk, Citation2017; Xu et al., Citation2018). Based on an extensive literature review, we distinguish 10 I4Ts: (1) additive manufacturing; (2) AI; (3) augmented reality; (4) autonomous robots; (5) autonomous vehicles; (6) cloud computing; (7) cybersecurity; (8) machine tools; (9) quantum computers; and (10) system integration. Doing so, we follow the broad definition of I4 that includes but also goes beyond the digitalization of manufacturing industries and global value chains.
To map the geography of I4 knowledge production in Europe, we need to (1) identify precisely the I4 technological domains, (2) geolocate the regions where they are being developed, and (3) measure how they connect to other technologies, based on our relatedness framework. We use the OECD’s REGPAT database (March 2018 edition) to identify I4T patents, assign them to technology classes and compute the degree of relatedness between technology classes. OECD-REGPAT is a regionalized patent dataset derived from PATSTAT (Maraut et al., Citation2008). It contains patent applications to the European Patent Office (EPO) from 1977 to 2017. Patent applications are regionalized at the NUTS-2 level by inventor addresses.
First, we linked the 10 I4Ts directly or indirectly to the Cooperative Patent Classification (CPC). The CPC is one of the most precise technological classifications, breaking down technologies in approximately 250,000 categories. For some I4Ts, this is straightforward to do, as for cloud computing for instance, because all patents that make an invention claim to the technology of grid computing is classified under the category G06F9/5072. For other I4Ts, we were able to reconstruct them indirectly by combining subcategories, such as for the category of autonomous vehicles that combines subcategories such as cruise control (B60W30/14) and the lidar system (G01S17), which are key components of autonomous vehicles technologies. Other I4 technologies, such as the IoT or blockchain technologies, were very hard to identify accurately and could not be isolated as separate categories.
shows the number of patents at EPO in each of the 10 I4T categories for the period 2002–16.Footnote1 What can be observed first is that there was a lot of patenting in I4Ts already at the beginning of the 21st century. This confirms other studies that the first I4Ts emerged already decades ago (Popkova et al., Citation2019; Valdes & Ilja Rudyk, Citation2017). Second, patenting in I4Ts increased in the decade 2002–11, despite the 2008 crisis, but after that, it more or less stabilized. Third, patenting in cybersecurity is the most intense by far, followed by machine tools and autonomous vehicles. Fourth, most I4T categories witness a strong increase in number of patents, with the exception of cybersecurity and machine tools. The sharp drop in patenting in quantum computers in Europe strikes the eye in particular.
Table 1. Industry 4.0 technology (I4T) patent count per period.
The next step is to determine the degree of relatedness between I4Ts, and between each I4T and all other technologies. This information is needed to test the idea that I4 technologies will only thrive if they connect to an ecosystem of related technologies that will nurture their growth by drawing on the specific knowledge bases in regions (Balland et al., Citation2019; Rigby, Citation2015). There are different ways of assessing the relatedness between activities (Boschma, Citation2017; Freire, Citation2017; Hidalgo et al., Citation2007).
To measure technological relatedness between patent classes, we use the distribution of knowledge claims by CPC class on each patent application to the EPO, following Boschma et al. (Citation2015) and Rigby (Citation2015). This is done by counting the number of EU patents for a given period that contains a co-class pair, say i and j, and then standardizing this count by the total number of patents that record knowledge claims in I4T/CPC classes i and j. Relatedness is, therefore, a standardized measure of the frequency with which two I4T/CPC classes appear on the same patent document. We use a standardization method (Steijn, Citation2017) based on Van Eck and Waltman (Citation2009), as implemented in the relatedness function of the EconGeo R package (Balland, Citation2017). Once we have calculated relatedness between technologies, we can formalize relatedness between technologies as a network, the so-called ‘knowledge space’. The knowledge space is an n*n network where the individual nodes i (i = 1, … , n) represent technological categories (I4T or CPC classes), and the links between them indicate their degree of relatedness. We compute relatedness between each pair of technologies i and j for three non-overlapping periods: 2002–06, 2007–11 and 2012–16.
is a graph visualization of the knowledge space for the last period. I4Ts tend to locate at the periphery of this network, signalling that they build on capabilities that are not generic, and not easy to find in other technologies. It also indicates that I4Ts are novel technologies that emerge outside the core of the network where most technologies are positioned. Most I4Ts tend to cluster around similar technologies, while some I4Ts are more isolated. Additive manufacturing stands out in particular because it is located a great distance from all the other I4Ts in the knowledge space.
explores the relatedness between the 10 I4Ts in more detail. On the left-hand side, relatedness is displayed as a network (), while on the right-hand side relatedness is displayed as a heat map, with more intense colours meaning a higher level of relatedness. The highest levels of relatedness appear to be between quantum computers and AI, and between machine tools and autonomous robots. By and large, there is one knowledge cluster around computer-related I4Ts (quantum computers, AI, cloud computing, cybersecurity and system integration), and a second knowledge cluster around machine tools, autonomous robots and autonomous vehicles.Footnote2 AI seems to link the two clusters to some extent. Augmented reality and additive manufacturing tend to be more disconnected from the other I4Ts. In other words, I4 contains many dimensions that are not easy to describe under one unifying label. The relatedness framework reveals which of these dimensions are more tightly linked to each other in terms of shared competences, and which to a lesser extent.
MODELLING THE ENTRY OF I4Ts IN EUROPEAN REGIONS
Using the information on the knowledge space, we identify the I4 knowledge structure of all NUTS-2 regions in the EU-27 plus Iceland, Norway, Switzerland and the UK. We are particularly interested in exploring the I4-related knowledge cores of all regions, or how much of the technology produced within each NUTS-2 region (as captured by patents) tends to cluster around I4Ts, which would signal a strong local environment for new knowledge production in I4Ts.
To capture the potential of each region in Europe to develop I4Ts, we calculated for each region the density of technological knowledge production in the vicinity of I4T i. Following Hidalgo et al. (Citation2007), Rigby (Citation2015) and Balland et al. (Citation2019), the density of knowledge production around a given I4T i in region r at time t is derived from the technological relatedness of technology i to all other technologies j (I4Ts and non-I4Ts) in which the region has a relative technological advantage (RTA), divided by the sum of technological relatedness of technology i to all the other technologies j in the reference region at time t:
RTA is a binary variable that takes a value of 1 when a region has a greater share of patents in technology class i than the reference group (all European regions), and 0 otherwise. A region r has RTA in the production of technological knowledge i (r = 1, … , n; i = 1, … , k) such that RTAr,i,t = 1 if:
The higher the region’s score on relatedness density, the closer, on average, its existing set of technologies are to I4T. In other words, relatedness density reflects an overall average score of the potential of a region to develop a specific I4T. Below, we test this assumption and assess whether relatedness density indeed has a positive effect on the probability of a region to diversify into a new I4T.
Because we are interested in the entry of a new specialization in a I4T, we estimate the probability that a region develops a new RTA in a I4T. Following Boschma et al. (Citation2015) and Balland et al. (Citation2019), our main variable of interest is the relatedness density between a I4T and the overall technological portfolio of a region. We include control variables at the regional level that may affect the entry probability of an I4T in a region: gross domestic product (GDP) per capita, population (log), educational attainment (share of those aged 30–34 that have completed tertiary education) and R&D (gross expenditures for research and development). Data are taken from Cambridge Econometrics and Eurostat. summarizes the descriptives statistics for the variables.
Table 2. Summary statistics.
All specifications are estimated at the region technology period level. We use a linear probability model to assess the probability that a region specializes in a new I4T (entry) using the following specification:
If a region already has RTA in I4T i in t – 1, then the observation is removed from the analysis, because the respective I4T i cannot enter by definition. If the region does not already have RTA in I4T i in t – 1 but it does in t, then entryr,i,t = 1 (entryr,i,t = 0 otherwise). Regionsr,t–1 is a vector that summarizes the range of observable time-varying regional characteristics (GDP per capita, population, education and R&D). The baseline specification is a two-way fixed-effects model where
is a I4T fixed effect,
is a time fixed effect and
is a regression residual. Our panel consists of data for 295 NUTS-2 regions and 10 I4Ts over the period 2002–16. We average the data over non-overlapping five-year periods, denoted by t. To dampen potential endogeneity issues, all independent variables are lagged by one period, denoted by t – 1.
presents the main findings. In all entry model specifications, we find that relatedness density has a positive and significant effect on the probability that a region specializes (RTA > 1) in a new I4T, which is expected and consistent with other studies (Balland et al., Citation2019; Boschma et al., Citation2015; Rigby, Citation2015). The effect of relatedness is also strong: an increase of 10% in relatedness density in a region is associated with a 52–63% relative increase in the entry probability of a given I4T in a region.Footnote3 We also find that regions with a larger population base are more likely to enter in I4T. This is consistent with findings that more complex technologies (such as I4T) disproportionally concentrate in large cities (Balland & Rigby, Citation2017; Balland et al. Citation2020). GDP per capita and R&D in regions do not seem to matter, while the coefficient of tertiary education is only positive and significant in model 4. In column 4, we also include country dummies to control for country-specific features such as national institutions, policy, etc.
Table 3. Diversification model (Industry 4.0 technologies – I4Ts).
MAPPING THE FUTURE INDUSTRY 4.0 CENTRES OF KNOWLEDGE PRODUCTION IN EUROPE
The diversification model showed that relatedness density is a good predictor for the entry of a new I4T in a region in Europe. This makes us confident that relatedness density can be used as a measure to map the potential of all EU regions regarding their future diversification opportunities in I4Ts.
shows a map of Europe with respect to relatedness density in all I4Ts. Relatedness density is computed for the most recent period 2012–16. There are huge differences across European regions with respect to their potential future participation in I4Ts. shows the top 20 European regions with the highest potential in I4Ts. More than half of all top 20 regions in I4Ts comes from only three countries in Europe, that is, Germany, France and the UK. The top three consists of Oberbayern (Munich), Île-de-France (Paris) and Berlin, followed closely by Östra Mellansverige and Outer London.
Table 4. Top 20 European Union regions: relatedness density around all Industry 4.0 technologies (I4Ts).a
There are also significant differences regarding the regions with the highest potentials in Europe between the 10 I4Ts. and show for all European regions their scores on relatedness density in autonomous vehicles and cybersecurity, respectively. The two maps look quite different: for instance, German regions score high on autonomous vehicles but low on cybersecurity. This is because they represent two very different I4Ts positioned far away from each other in the knowledge space (). This means the two I4Ts rely on different related technologies that are also concentrated in different regions in Europe. This explains why there is no single geography of I4T in Europe.
How important it is to distinguish between the 10 I4Ts is also shown in . It shows how different the geographies of future knowledge production in the 10 I4Ts in Europe look like, reflecting the geographical distributions of their most relevant regional capabilities. Appendix A in the supplemental data online shows maps for all I4Ts. The findings on relatedness density for cloud computing and quantum computers need to be interpreted with great caution, due to the low patent activity in these two I4Ts in Europe (). Broadly speaking, German regions such as Oberbayern and Stuttgart tend to dominate and show high potentials in autonomous vehicles, machine tools, autonomous robots, additive manufacturing and system integration. UK regions tend to score high on relatedness density in augmented reality. Potential in knowledge production in artificial intelligence is high in UK regions, but also fairly distributed across other European countries, often found in their capital city regions, such as Île-de-France and Comunidad de Madrid. High potentials in cybersecurity are found in regions in France, UK and Finland in particular. At the same time, many European countries are rarely mentioned, or not mentioned at all in these top rankings, suggesting a weak technological potential to develop I4Ts in the near future.
Table 5. Top 10 European regions: relatedness density around each Industry 4.0 technology (I4T).
This uneven opportunity to develop I4T is further illustrated in , which maps development opportunities in all 10 I4Ts in the French region of Île-de-France and the Italian region of Piemonte. Relatedness density is shown on the x-axis, while the y-axis indicates the number of patents (log) in each I4T in the whole of Europe. What can be observed is that Île-de-France has a low I4T potential (low relatedness density) in machine tools, additive manufacturing and system integration, but it has high potential in cybersecurity and AI. The case of Piemonte tells a different story: this region has I4T potential in autonomous robots, autonomous vehicles and machine tools, which partly reflects its techno-industrial past, but Piemonte has low potential in other I4Ts. This illustration shows how important it is to develop an I4T policy that defies a ‘one-size-fits-all’ framework, but instead is tailor-made and targets I4-related capabilities in order to increase the probability of successful policy intervention.
CONCLUSIONS
This paper aimed to identify the future I4T centres of knowledge production in Europe. A thorough exploration of the I4 literature showed there is no consensus on what I4T stands for. There is also no formal classification or list of I4T technologies. We made an extensive literature review of key publications on I4 that were often literature reviews on I4 themselves. We followed a broad definition of I4 that includes but also goes beyond the digitalization of industries and value chains. We identified and distinguished 10 I4Ts: (1) additive manufacturing; (2) AI; (3) augmented reality; (4) autonomous robots; (5) autonomous vehicles; (6) cloud computing; (7) cybersecurity; (8) machine tools; (9) quantum computers; and (10) system integration.
The study aims to apply insights from the regional diversification literature (Boschma, Citation2017; Hidalgo et al., Citation2018) to the study of the geography of I4 (De Propris & Bailey, Citation2020). Linking the two literatures, we expected I4Ts to thrive in regions where they can draw on resources from I4-related technologies available at the regional scale. We identified I4T-related technologies, that is, technologies that are frequently combined with I4Ts in patent documents. This resulted in the construction of a knowledge space, in which the level of relatedness between I4Ts and all other technological fields is shown. We found that I4Ts are located at the periphery of the knowledge space. Some I4Ts tend to cluster around similar technologies, while other I4Ts such as additive manufacturing are more isolated. Broadly speaking, we identified a knowledge cluster around computer-related I4Ts, and another knowledge cluster centred on manufacturing-related I4 technologies. This tends to be in line with our broad take on I4 that includes many dimensions, and which is hard to unite under a single, unifying label.
We used this I4-knowledge space to estimate the impact of relatedness on the entry of I4Ts in NUTS-2 regions in 31 European countries (EU-27 plus Iceland, Norway, Switzerland and the UK) during the period 2002–16. As expected, we found that relatedness has a strong and positive effect on the probability that a region specializes in a new I4T. This shows that regions with a high potential in terms of a strong local presence of I4T-related technologies are more likely to diversify successfully in new I4Ts. We also found that regions with a larger population are more likely to enter new I4Ts.
Because relatedness seemed to be a good predictor of new knowledge production in I4Ts in European regions in the past (2002–16), we also used the relatedness measure to map the potential of European regions to contribute to and participate in future knowledge production in I4Ts. The map of future I4T centres of knowledge production in Europe shows huge differences across regions. German, French and UK regions tend to show the highest probabilities to develop I4Ts in the future, while many European regions show a weak potential to contribute to new knowledge production in I4Ts. There are also significant differences between the 10 I4Ts. We found no single geography of I4T in Europe. Instead, we found many. Our study shows this is because I4Ts rely on different related technologies that are also located in different regions in Europe. In other words, the geographies of specific I4Ts in Europe tend to reflect the geographical distributions of their most relevant regional capabilities.
Our findings imply that public policy intervention that aims to develop I4Ts should take as a point of departure the particular I4T potentials that region possess. Regions with a low or no I4T potential should think twice to invest public funds in I4Ts, because the risk of policy failure is high. Public policy should target those regions that have related I4T capabilities, as these provide local assets that might be exploited to make policy effective (Hallward-Driemeier et al., Citation2020).
The paper is not without limitations, however. First, we used patent data to capture potentials of regions to contribute to new knowledge production in I4Ts. While patents are a rich source of information, there might be other sources of knowledge (embodied in R&D and skills of people) that are highly relevant for I4Ts that are not taken up by patent data.
Second, to identify regional potentials in new I4Ts, there might be other factors besides relevant local capabilities that might be crucial for regions to participate fully in I4. Local university–industry linkages might be a precondition to exploit fully local capabilities (D’Este et al., Citation2013; Reischauer, Citation2018), but also knowledge links with other regions that provide access to complementary capabilities might enhance the ability of regions to contribute to new knowledge creation in I4Ts (Balland & Boschma, Citation2021; Neffke et al., Citation2018).
Third, it might be the case that some relevant capabilities are locally available, but regions might still lack the critical mass to participate fully in I4Ts. This certainly applies to the two emerging I4Ts of cloud computing and quantum computers in which Europe is lagging behind China and the United States. But even in these cases, a thorough understanding of where relevant local capabilities are concentrated is crucial to develop an effective I4T policy strategy.
Fourth, to complement this study, there is a need to look more closely at the fields of economic application of I4Ts in regions. While we expect some overlap between the locations of technology production of I4 identified in this paper and the locations of industrial production and application of I4 in Europe, this is not necessarily the case. This should be taken up in future research. This would bring important insights in factors that may influence the rate of economic application of new I4Ts, which is a key topic in the I4 literature (De Propris & Bailey, Citation2020).
Future studies should also focus on the consequences of I4 for spatial inequalities in Europe. A promising avenue is to study and assess the role of big companies where crucial I4 knowledge is developed, applied and concentrated. Balland et al. (Citation2019) showed that complex knowledge (also in I4) is heavily spatially concentrated and contributes to increasing spatial inequality. Ménière et al. (Citation2017) estimated that 25 global ICT companies take up about half of all patent applications in I4Ts, especially in core technologies. Such research sheds light on the role of power and big businesses that are active in new I4Ts, and which are expected to shape new spatial inequalities in Europe and beyond to a considerable degree (Iammarino et al., Citation2019). Finally, this relates to more fundamental questions to how society and public policy should respond to I4. Concerns have been raised regarding the monopoly power of giant companies (Feldman et al., Citation2019) because they block innovation, undermine workers’ rights and make jobs obsolete, but also because they have negative impacts on society at large, such as violating privacy and subverting democratic processes through information distortion and electoral interference (Cooke, Citation2019). This is part of a much larger debate in society which in the end will impact the extent to which, and what kinds of I4T’s will be produced and implemented, and where and how.
Supplemental Material
Download PDF (540 KB)ACKNOWLEDGEMENT
This paper is partly based on a background paper produced for the Europe 4.0 flagship project of the World Bank (Hallward-Driemeier et al., Citation2020).
DISCLOSURE STATEMENT
No potential conflict of interest was reported by the authors.
Additional information
Funding
Notes
1. The year 2016 is our final year, because there is a significant drop in patents after 2016 because many patents are still ‘under review’.
2. There are some interesting key links between machine tools and digital technologies. It all relates to the transition towards intelligent machine tools (IMT) or smart machine tools (SMT). The key components of this transition build heavily on recent development in AI and the role of autonomous sensing, autonomous connection (machine-to-machine, in particular), autonomous learning, autonomous optimization and autonomous execution. This is part of the ‘physical AI’ wave, as Lee (Citation2018) dubbed it.
3. The mean of entry is 0.12. In model 1, for instance, the probability of entry increases (in absolute terms) by about 0.008 for any additional unit of relatedness density (scale 0–100). Therefore, an additional 10 points in relatedness density boosts entry by about (10*0.008)/0.126 = 63% (relative increase).
REFERENCES
- Acemoglu, D., & Restrepo, P. (2019). Automation and new tasks: How technology displaces and reinstates labor. Journal of Economic Perspectives, 33(2), 3–30. https://doi.org/https://doi.org/10.1257/jep.33.2.3
- Alcacer, V., & Cruz-Machado, V. (2019). Scanning the Industry 4.0: A literature review on technologies for manufacturing systems. Engineering Science and Technology, 22(3), 899–919. https://doi.org/https://doi.org/10.1016/j.jestch.2019.01.006
- Autor, D. H. (2015). Why are there still so many jobs? The history and future of workplace automation. Journal of Economic Perspectives, 29(3), 3–30. https://doi.org/https://doi.org/10.1257/jep.29.3.3
- Balland, P. A. (2017). EconGeo: Computing key indicators of the spatial distribution of economic activities, R package version 1.3. https://github.com/PABalland/EconGeo
- Balland, P., & Boschma, R. (2021). Complementary inter-regional linkages and Smart Specialisation. An empirical study on European regions. Regional Studies. https://doi.org/https://doi.org/10.1080/00343404.2020.1861240.
- Balland, P. A., Boschma, R., Crespo, J., & Rigby, D. (2019). Smart specialization policy in the EU: Relatedness, knowledge complexity and regional diversification. Regional Studies, 53(9), 1252–1268. https://doi.org/https://doi.org/10.1080/00343404.2018.1437900
- Balland, P. A., Jara-Figueroa, C., Petralia, S., Steijn, M., Rigby, D., & Hidalgo, C. (2020). Complex economic activities concentrate in large cities. Nature Human Behaviour, 4(3), 248–254. https://doi.org/https://doi.org/10.1038/s41562-019-0803-3
- Balland, P. A., & Rigby, D. (2017). The geography of complex knowledge. Economic Geography, 93(1), 1–23. https://doi.org/https://doi.org/10.1080/00130095.2016.1205947
- Bellandi, M., Santini, E., Vecciolini, C., & de Propris, L. (2020). Industry 4.0: Transforming local productive systems in the Tuscany region. In L. De Propris, & D. Bailey (Eds.), Industry 4.0 and regional transformations (pp. 84–96). Routledge.
- Bessen, J., Goos, M., Salomons, A., & van den Berge, W. (2019). Automatic reaction – What happens to workers at firms that automate? (CPB Discussion Paper). Netherlands Bureau for Economic Policy Analysis.
- Boschma, R. (2017). Relatedness as driver behind regional diversification: A research agenda. Regional Studies, 51(3), 351–364. https://doi.org/https://doi.org/10.1080/00343404.2016.1254767
- Boschma, R., Balland, P. A., & Kogler, D. F. (2015). Relatedness and technological change in cities: The rise and fall of technological knowledge in U.S. metropolitan areas from 1981 to 2010. Industrial and Corporate Change, 24(1), 223–250. https://doi.org/https://doi.org/10.1093/icc/dtu012
- Boschma, R., Heimeriks, G., & Balland, P. A. (2014). Scientific knowledge dynamics and relatedness in biotech cities. Research Policy, 43(1), 107–114. https://doi.org/https://doi.org/10.1016/j.respol.2013.07.009
- Chiarello, F., Trivelli, L., Bonaccorsi, A., & Fantoni, G. (2018). Extracting and mapping industry 4.0 technologies using Wikipedia. Computers in Industry, 100, 244–257. https://doi.org/https://doi.org/10.1016/j.compind.2018.04.006
- Ciffolilli, A., & Muscio, A. (2018). Industry 4.0: National and regional comparative advantages in key enabling technologies. European Planning Studies, 26(12), 2323–2343. https://doi.org/https://doi.org/10.1080/09654313.2018.1529145
- Colombelli, A., Krafft, J., & Quatraro, F. (2014). The emergence of new technology-based sectors in European regions: A proximity-based analysis of nanotechnology. Research Policy, 43(10), 1681–1696. https://doi.org/https://doi.org/10.1016/j.respol.2014.07.008
- Cooke, P. (2019). Responsible research and innovation? From FinTech’s ‘flash crash’ at Cermak to digitech’s Willow Campus and Quayside. European Planning Studies, 27(12), 2376–2393. https://doi.org/https://doi.org/10.1080/09654313.2018.1556610
- Corradini, C. (2019). Location determinants of green technological entry: Evidence from European regions. Small Business Economics, 52(4), 845–858. https://doi.org/https://doi.org/10.1007/s11187-017-9938-7
- Cortinovis, N., Xiao, J., Boschma, R., & van Oort, F. (2017). Quality of government and social capital as drivers of regional diversification in Europe. Journal of Economic Geography, 17(6), 1179–1208. https://doi.org/https://doi.org/10.1093/jeg/lbx001
- Dallasega, P., Rauch, E., & Linder, C. (2018). Industry 4.0 as an enabler of proximity for construction supply chains: A systematic literature review. Computers in Industry, 99, 205–225. https://doi.org/https://doi.org/10.1016/j.compind.2018.03.039
- De Propris, L., & Bailey, D. (Eds.). (2020). Industry 4.0 and regional transformations. Routledge.
- D’Este, P., Guy, F., & Iammarino, S. (2013). Shaping the formation of university–industry research collaborations: What type of proximity does really matter? Journal of Economic Geography, 13(4), 537–558. https://doi.org/https://doi.org/10.1093/jeg/lbs010
- Feldman, M., Guy, F., & Iammarino, S. (2019, October. Regional income disparities, monopoly & finance (Working Paper). University of London.
- Freire, C. (2017). Diversification and structural economic dynamics. Boekenplan.
- Frey, C. B., & Osborne, M. (2016). The future of employment: How susceptible are jobs to computerisation? Technological Forecasting and Social Change, 114, 254–280. https://doi.org/https://doi.org/10.1016/j.techfore.2016.08.019
- Gotz, M., & Jankowska, B. (2017). Clusters and Industry 4.0 – Do they fit together? European Planning Studies, 25(9), 1633–1653. https://doi.org/https://doi.org/10.1080/09654313.2017.1327037
- Gress, D. R., & Kalafsky, R. V. (2015). Geographies of production in 3D: Theoretical and research implications stemming from additive manufacturing. Geoforum; Journal of Physical, Human, and Regional Geosciences, 60, 43–52. https://doi.org/https://doi.org/10.1016/j.geoforum.2015.01.003
- Guo, Q., & He, C. (2017). Production space and regional industrial evolution in China. Geojournal, 82(2), 379–396. https://doi.org/https://doi.org/10.1007/s10708-015-9689-4
- Hall, P., & Preston, P. (1988). The carrier wave. New information technology and the geography of innovation 1846–2003. Unwin Hyman.
- Hallward-Driemeier, M., Nayyar, G., Fengler, W., Aridi, A., & Gill, I. (2020). Europe 4.0. addressing the digital Dilemma. World Bank.
- He, C., Yan, Y., & Rigby, D. (2018). Regional industrial evolution in China. Papers in Regional Science, 97(2), 173–198. https://doi.org/https://doi.org/10.1111/pirs.12246
- Hidalgo, C. A., Klinger, B., Barabasi, A. L., & Hausmann, R. (2007). The product space and its consequences for economic growth. Science, 317(5837), 482–487. https://doi.org/https://doi.org/10.1126/science.1144581
- Hidalgo, C., Balland, P. A., Boschma, R., Delgado, M., Feldman, M., Frenken, K., Glaeser, E., He, C., Kogler, D., Morrison, A., Neffke, F., Rigby, D., Stern, S., Zheng, S., & Zhu, S. (2018). The principle of relatedness. In Springer proceedings in complexity. Springer. Paper originally presented at the International Conference on Complex Systems (ICCS) (pp. 451–457). Cambridge, MA, USA, July 22–27.
- Iammarino, S., Rodriguez-Pose, A., & Storper, M. (2019). Regional inequality in Europe: Evidence, theory and policy implications. Journal of Economic Geography, 19(2), 273–298. https://doi.org/https://doi.org/10.1093/jeg/lby021
- Kamble, S. S., Gunasekaran, A., & Gawankar, S. A. (2018). Sustainable Industry 4.0 framework: A systematic literature review identifying the current trends and future perspectives. Process Safety and Environmental Protection, 117, 408–425. https://doi.org/https://doi.org/10.1016/j.psep.2018.05.009
- Kogler, D. F., Rigby, D. L., & Tucker, I. (2013). Mapping knowledge space and technological relatedness in US cities. European Planning Studies, 21(9), 1374–1391. https://doi.org/https://doi.org/10.1080/09654313.2012.755832
- Lecuyer, C. (2006). Making Silicon Valley. Innovation and the growth of high tech, 1930–1970. MIT Press.
- Lee, K. F. (2018). AI superpowers: China, Silicon Valley and the new world order. Houghton Mifflin Harcourt.
- Li, D. (2020). Place dependence of renewable energy technologies. Connecting local and global scale [PhD thesis]. Utrecht University, Utrecht.
- Liao, Y., Deschamps, F., de Freitas Rocha Loures, E., & Felipe Pierin Ramos, L. (2017). Past, present and future of Industry 4.0 – A systematic literature review and research agenda proposal. International Journal of Production Research, 55(12), 3609–3629. https://doi.org/https://doi.org/10.1080/00207543.2017.1308576
- Lu, Y. (2017). Industry 4.0: A survey on technologies, applications and open research issues. Journal of Industrial Information Integration, 6, 1–10. https://doi.org/10.1016/j.jii.2017.04.005
- Markusen, A., Hall, P., & Glasmeier, A. (1986). High tech America: The what, how, where and why of the sun-rise industries. Allen & Unwin.
- Marshall, M. (1987). Long waves of regional development. Macmillan.
- Maraut, S., Dernis, H., Webb, C., Spiezia, V., & Guellec, D. (2008). The OECD REGPAT database: A presentation (OECD Science, Technology and Industry Working Paper No. 2008/02). OECD Publ.
- Ménière, Y., Rudyk, I., & Valdes, J. (2017). Patents and the fourth industrial revolution. The inventions behind digital transformation. European Patent Office (EPO).
- Montresor, S., & Quatraro, F. (2019). Green technologies and Smart Specialisation Strategies: A European patent-based analysis of the intertwining of technological relatedness and key enabling technologies. Regional Studies, 54(10), 1354–1365. https://doi.org/https://doi.org/10.1080/00343404.2019.1648784
- Muneepeerakul, R., Lobo, J., Shutters, S. T., Gomez-Lievano, A., & Qubbaj, M. R. (2013). Urban economies and occupation space: Can they get ‘there’ from ‘here’? PLoS ONE, 8(9), e73676. https://doi.org/https://doi.org/10.1371/journal.pone.0073676
- Muro, M., Maxim, R., & Whiton, J. (2019). Automation and artificial intelligence. How machines are affecting people and places. Metropolitan Policy Program, Brookings Institution.
- Muscio, A., & Ciffolilli, A. (2020). What drives the capacity to integrate Industry 4.0 technologies? Evidence from European R&D projects. Economics of Innovation and New Technology, 29(2), 169–183. https://doi.org/https://doi.org/10.1080/10438599.2019.1597413
- Nedelkoska, L., & Quintini, G. (2018). Automation, skill use and training, OECD social, employment and migration (Working Papers No. 2018/3). Organisation for Economic Co-operation and Development (OECD) Publ.
- Neffke, F., Hartog, M., Boschma, R., & Henning, M. (2018). Agents of structural change. The role of firms and entrepreneurs in regional diversification. Economic Geography, 94(1), 23–48. https://doi.org/https://doi.org/10.1080/00130095.2017.1391691
- Neffke, F., Henning, M., & Boschma, R. (2011). How do regions diversify over time? Industry relatedness and the development of new growth paths in regions. Economic Geography, 87(3), 237–265. https://doi.org/https://doi.org/10.1111/j.1944-8287.2011.01121.x
- Oztemel, O., & Gursev, S. (2020). Literature review of Industry 4.0 and related technologies. Journal of Intelligent Manufacturing, 31(1), 127–182. https://doi.org/https://doi.org/10.1007/s10845-018-1433-8
- Perez, C., & Soete, L. (1988). Catching up in technology: Entry barriers and windows of opportunity. In G. Dosi, C. Freeman, R. Nelson, G. Silverberg, & L. Soete (Eds.), Technical change and economic theory (pp. 458–479). Pinter.
- Popkova, E. G., Ragulina, Y. V., & Bogoviz, A. V. (Eds.). (2019). Industry 4.0: Industrial revolution of the 21st century. Springer.
- Reischauer, G. (2018). Industry 4.0 as policy-driven discourse to institutionalize innovation systems in manufacturing. Technological Forecasting and Social Change, 132, 26–33. https://doi.org/https://doi.org/10.1016/j.techfore.2018.02.012
- Rigby, D. (2015). Technological relatedness and knowledge space. Entry and exit of US cities from patent classes. Regional Studies, 49(11), 1922–1937. https://doi.org/https://doi.org/10.1080/00343404.2013.854878
- Santoalha, A., & Boschma, R. (2021). Diversifying in green technologies in European regions: Does political support matter? Regional Studies, 55(2), 182–195. https://doi.org/https://doi.org/10.1080/00343404.2020.1744122.
- Santos, C., Mehrsai, A., Barros, A. C., Araújo, M., & Ares, E. (2017). Towards Industry 4.0: An overview of European strategic roadmaps. Procedia Manufacturing, 13, 972–979. https://doi.org/https://doi.org/10.1016/j.promfg.2017.09.093
- Scott, A. (1988). New industrial spaces: Flexible production organization and regional development in North America and Western Europe. Pion.
- Steijn, M. P. A. (2017). Improvement on the association strength: Implementing probability measures based on combinations without repetition (Working Paper). Utrecht University.
- Strange, R., & Zucchella, A. (2017). Industry 4.0, global value chains and international business. Multinational Business Review, 25(3), 174–184. https://doi.org/https://doi.org/10.1108/MBR-05-2017-0028
- Tanner, A. N. (2016). The emergence of new technology-based industries: The case of fuel cells and its technological relatedness to regional knowledge bases. Journal of Economic Geography, 16(3), 611–635. https://doi.org/https://doi.org/10.1093/jeg/lbv011
- Valdes, Y. M., & Ilja Rudyk, J. (2017). Patents and the fourth industrial revolution (EPO). European Patent Office (EPO), Handelsblatt Research Institute.
- van den Berge, M., & Weterings, A. (2014). Relatedness in eco-technological development in European regions (Papers in Evolutionary Economic Geography No. 14.13). Utrecht University.
- Van Eck, N. J., & Waltman, L. (2009). How to normalize co-occurrence data? An analysis of some well-known similarity measures. Journal of the American Society for Information Science and Technology, 60(8), 1635–1651. https://doi.org/10.1002/asi.21075
- Xu, L. D., Lu, E. L., & Li, L. (2018). Industry 4.0: State of the art and future trends. International Journal of Production Research, 56(8), 2941–2962. https://doi.org/https://doi.org/10.1080/00207543.2018.1444806