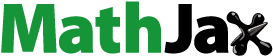
ABSTRACT
We examine the regional mortality rates associated with the spread of Covid-19 in Europe. In particular, we analyse the potential contribution of the country’s geographical and institutional features in shaping the virus's interregional spread and resulting local death rates. Our analysis is based on information from both pandemic waves from March to the end of November 2020. In a multilevel hierarchical regression estimation setting, covering information from over 600 OECD-TL3 areas, over 120 larger OECD-TL2 areas and 15 national-level areas, our findings suggest that local death rates are affected by the geographical and institutional features at the local, regional and national levels.
KEYWORDS:
INTRODUCTION
The aim of this paper is to examine the regional mortality rates associated with the spread of the Covid-19 pandemic across Europe. We analyse these death rates during the first major wave of the pandemic to hit Europe between the beginning of March and the end of the first week of June 2020, and then we also consider the longer term mortality rates, including the second wave of the pandemic until the end of the third week of November 2020. Our analysis covers the whole period from the onset of the pandemic in Europe right through to immediately before the appearance of the four newer and more contagious Alpha, Beta, Gamma and Delta variants of Covid-19 originating in the UK, South Africa, Brazil and India, respectively, and also predates the nationwide or worldwide rollout of any vaccines, which first commenced in the UK on 8 December 2020.
Our key objective is to examine how the geographical and institutional features of the country and the region may have shaped the interregional spread of the virus and resulting local death rates. Asking these types of questions in the European context becomes possible due to the geographical and institutional heterogeneity of European Union’s (EU) countries and regions. Even though so many countries are geographically close or even contiguous to each other, the variation in regional economic geography and institutional features ought to provide sufficient information on these different issues to understand the role which these various features have played in facilitating or inhibiting the incidence of Covid-19-related deaths across European regions. European countries exhibit large variations in population densities, urban scales, spatial patterns of urban systems and international travel accessibility, along with profoundly different governance systems in terms of the nature and quality of governance and different degrees of individualism and cooperative behaviour. At present, we are unclear as to the importance of the roles played by local, regional, national or international economic geography and institutional factors in explaining the scale and nature of the pandemic shocks faced by different countries and regions, and this is what this paper is designed to investigate.
Our paper is different from existing papers that examine these issues either at a very local level (Franch-Pardo et al., Citation2020), at a single regional level (Bourdin et al., Citation2021; Doerr & Gambacorta, Citation2020; European Observation Network for Territorial Development and Cohesion (ESPON), Citation2020; Meinen et al., Citation2021; Rodriguez-Pose & Burlina, Citation2021), or at a national scale (Pana et al., Citation2021). We explicitly consider that the spread of Covid-19 at a local scale is likely to be a multilevel phenomenon in which the local transmission and mortality effects of Covid-19 are associated with very local small regional factors, which themselves are to some extent conditioned on higher level broader regional factors, which in turn are likely to be conditioned by higher level nationwide factors. In other words, we posit that local Covid-19-related effects are nested within multilevel hierarchical sets of influences. As far as we are aware, no previous paper has examined the geography of Covid-19 in a cross-country, cross-region and multilevel setting.
Therefore, in order to examine the potential hierarchically nested nature of Covid-19 mortality effects, we assemble and integrate different sources of evidence that capture these geographical and institutional differences at different spatial scales, as they relate to the potential spread and mortality of the virus. We then examine these data using a series of multilevel hierarchical nested models to assess the extent to which local Covid-19 death rates relate to local, regional or national geographical or institutional factors. Our model results confirm that the data reflecting the geography of Covid-19 are indeed hierarchical in nature, and therefore multilevel nested hierarchical models are appropriate models for analysing these data.
Our findings suggest that the excess local death rates for European regions are a function of both geographical and institutional features at the local, regional and national levels. In particular, large metro areas are especially vulnerable, and there is also evidence that local death rates are also affected by the quality and effectiveness of national governments’ policy decisions. However, our results imply that while there is evidence that strong local governance may have helped reduce excess mortality, in general, neither the levels nor the quality of regional governance played any role in allaying Covid-19.
The rest of the paper is organized as follows. In the next section, we discuss the potential facilitators and inhibitors of the spread of Covid-19 across regions and nations. We move on to outline the data employed and outline the arguments justifying the use of each particular set of data, explaining how they reflect different aspects of the potential facilitators and inhibitors of the Covid-19-related deaths across EU regions. Then we report the results of the various specifications of the model estimated and consider which of these national and regional geographical and institutional features appear to contribute most to either the spread or mitigation of the pandemic-related deaths during 2020. Our final sections provide some insights and conclusions regarding the role played by economic geography in shaping the regional impacts of Covid-19.
THE REGIONAL INCIDENCE OF COVID-19 DEATHS
The nature and features of the Covid-19 pandemic crisis across Organisation for Economic Co-operation and Development (OECD) countries have been centre stage in almost all news bulletins published in every country. New insights, aspects and experiences of the crisis come to light almost daily, and the strains on all aspects of life are evident and ever-present. Yet, in marked contrast to other recent pandemics such as the 2002–04 severe acute respiratory syndrome (SARS) outbreak, the 2012 Middle East respiratory syndrome (MERS) outbreak, and the Ebola 2014–16 outbreak, industrialized OECD countries across the world bore the brunt of much of the first two waves of the Covid-19 impacts, and many still have amongst the highest per capita death rates. Industrialized societies have had to rapidly and profoundly adjust to the threats posed by the pandemic, and the economic shock effects associated with these adjustments on all aspects of economic and social activity have been profound. The nature, scale and speed of the pandemic in early 2020 caught most OECD countries out in terms of their levels of preparedness for such an eventuality, and this has resulted in many decisions being taken very quickly with only limited evidence on which to base those decisions. The health crisis has engendered an economic crisis, and the adverse economic shocks have been orders of magnitude beyond anything experienced by most living people. As such, as well as being on unprecedented scales, many of the policy and security responses have been rather blunt, as governments have moved quickly to implement profound changes to everyday life with no real prior experience of this type of situation (Smit et al., Citation2020). As such, countries have been sharing information and experiences in a bid to control the contagion and enhance cooperation as much as possible, given that the crisis is international as well as national.
The pandemic crisis is also very local and regional in nature, as well as being national and international (Bailey et al., Citation2020). There have been marked differences in the scale of the contagion within individual countries, with some regions facing much more severe health shocks than other regions. In particular, in many European countries, urban areas appear to have been particularly severely affected relative to rural regions, with cities such as Milan, Madrid, Barcelona, Paris and London facing major early contagion shocks. In countries such as the UK or the United States, the crisis initially appeared to most seriously affect the poorer parts of prosperous city-regions (Frey, Citation2020; Stier et al., Citation2020) as well as regions with major travel, hospitality and tourism industries (Muro et al., Citation2020), and this was followed by a spreading out to other largely economically weaker places and more remote regions (Berube, Citation2020; Nathan, Citation2020). However, across Europe, these patterns appear not to have been universal, with different types of places being affected differently in different countries (Bailey et al., Citation2020). There is some evidence that urban density may not, of itself, necessarily be a facilitator of viral transmission (Fang & Wahba, Citation2020) and that other contributory factors may be just as important, although as yet, our understanding of these issues is still limited.
It may be that these differences in part reflect the different economic geographies in different countries (Committee of the Regions (CoR), Citation2020). Some parts of Europe are very densely populated by international standards, whereas other parts of Europe exhibit very low population densities by OECD standards. The opportunities for interpersonal transmission of the virus are therefore likely to vary enormously according to population densities. At the same time, many European countries do not contain cities that would be regarded as being large in, for example, the United States or Japan. Across the whole of Europe, there are only two European cities whose functional economic areas are over 10 million people, and only five urban centres for more than 5 million people, with the majority of Europe’'s cities being of the order of 1 million or fewer inhabitants. On the one hand, this may suggest that interpersonal virus transmission opportunities may be fewer than in very large city cases. On the other hand, and differently to the North American urban context, is the fact that many parts of Europe are connected by dense high-speed cross-border railway networks as well as dense air and sea passenger transport routes. These densely integrated transport systems potentially offer widespread opportunities for virus transmission between countries.
Various pieces of research have found that local air pollution enhances Covid-19 death rates (Bourdrel et al., Citation2021), but what is not known is whether this is also a consequence of population density or of city size, given that pollution is also associated with both of these features. A further feature of a country’'s economic geography that may influence the transmission of the virus relates to the interregional economic inequalities. Countries with more unbalanced interregional economic systems may also exhibit greater inequalities in terms of their ability to address the challenges (Institute of Health Equity (IHE), Citation2021). Greater spatial concentrations of poverty, poor housing and poor health may exacerbate the pandemic’'s local and national mortality rates (Bailey et al., Citation2020). This is because the local overloading of health and social care systems due to localized institutional bottlenecks may lead to wider systemic failures, also putting severe pressure on other parts of the health and social care systems.
The variegated economic geography of Europe may therefore have played a part in the differential national experiences of Covid-19 mortality, due to different population densities, different numbers of international travellers and differences in interregional inequalities. As such, we might expect that higher population densities, higher mobility flows and higher interregional inequalities may all be associated with higher Covid-19-related transmission and death rates.
At the same time, the ability of a country or region to respond effectively to the Covid-19 pandemic, and thereby to either reduce the virus transmission rates or to improve the healthcare responses for any level of transmission rates, may also be conditioned and constrained by various features of the national and regional institutional and governance systems. In some cases, decisions made regarding the health and social care provision along with security, policing and public enforcement can be made primarily at a local or regional level based on detailed local knowledge (CoR, Citation2020). In contrast, these decisions are made at a more centralized level in other countries with less local information informing decisions. European countries differ markedly in terms of their governance and institutional systems, ranging from large federal or quasi-federal states such as Germany and Spain to large centralized states such as the UK and Poland, through to small centralized states such as Estonia and Ireland along with small decentralized federal states such as Austria and Belgium. In terms of institutional and governance issues, Europe is a patchwork quilt. As such, the ability to make policy decisions in a manner which is appropriate for the local context and local emergency challenges – dependent on the availability of information and the ability to act on that information – may therefore be institutionally constrained, and this may affect the efficacy of regional as well as national government responses to the pandemic.
Meanwhile, the efficacy of decision-making is also likely to depend on the quality of the governance system and its structure. We know that the effectiveness of government at the national level (Kaufmann et al., Citation2010) and the quality of government at the regional level (Charron et al., Citation2019) varies significantly across Europe, as well as the architecture of governance (Hooghe et al., Citation2016; Ladner et al., Citation2019) and that there are differences in the quality of governance even at these different institutional tiers. These differences may also account in part for differences in how different countries have approached the pandemic, with the ability to deploy different responses being dependent not only on decision-making structures, powers and autonomy but also on the quality of these decision-making roles. As such, the ways that different countries have handled the crisis at the local level (OECD, Citation2020) may depend on the balance between national and local decision-making systems.
In some cases, such as Australia or Germany, devolved federal types of governance systems would appear to have been a major advantage in the responses to Covid-19 during its early waves, although devolved governance is no guarantee of more a successful response. In terms of governance architectures, the United States is far more devolved than New Zealand, but during 2020, New Zealand's response to the crisis would appear to have been far superior to that of the United States. As such, many other country features may also play a role in determining how countries and regions have responded to the crisis.
On this point, two other features could potentially be crucial in determining the regional and national patterns of Covid-19-related deaths: the severity and stringency of the ‘lockdown’ decisions made and enforced by governments, and also the societal responses to these decisions. There is some evidence that the extent of the stringency of which countries have imposed these measures may be related to their success in containing the spread of the virus (Chaudhry et al., Citation2020), although the extent to which this is the case is still being debated.Footnote1 However, there are also arguments to suggest that the timing of the imposition of such measures is just as important as their severity (Kochańczyk & Lipniacki, Citation2021), with the earlier implementation of these measures being more effective than other measures imposed later. As such, both stringency and timing of the lockdown measures may be crucial in influencing the incidence of Covid-19-related deaths.
In addition, societal responses to any pandemic measures introduced are also crucial. If sufficiently large numbers of the population refuse to fully comply with the imposed social restrictions, then the efficacy of any policy restrictions can potentially be undermined. Cohesive societies, and societies accustomed to working together to achieve commonly shared societal goals, might potentially be expected to respond more constructively to any imposed measures for the common good; in contrast, more individualistic societies where cooperation is more difficult to achieve, might be expected to find it harder to ensure that any imposed measures are effective (OECD, Citation2012).
As the Prime Minister of New Zealand, Jacinda Ardern, famously stated on 14 March 2020: New Zealand must ‘go hard and go early’ to overcome the virus threat (Jamieson, Citation2020). Indeed, alongside Taiwan (Spencer, Citation2021), New Zealand implemented amongst the most stringent lockdown measures of any OECD country, including immediately banning all foreign travellers; both countries are regarded internationally to have successfully managed the crisis. Yet, while the mortality figures and widespread international recognition suggests that New Zealand's and Taiwan's responses to Covid-19 have been excellent, it is unclear whether their success was primarily due to these specific lockdown measures, or to the high social cohesiveness of their societies, or to being island nations. As well as being an island nation, in terms of reducing internal viral transmissions, New Zealand may have the advantage of having generally small urban settlements which are isolated from each other and with low population densities. Iceland also has similar features and has managed the crisis well. However, Taiwan has both large urban settlements and high population densities, and it still has managed the crisis just as effectively as either New Zealand or Iceland, as has Singapore, another densely populated island nation. Yet, another country that has successfully handled the crisis is South Korea, a country which, like Taiwan, has very large urban populations and very high population densities and, given that its only land border is North Korea, is effectively also an island nation. At the same time, all these countries are also rather centralized and top-down in terms of governance. Yet, being an island with a highly centralized governance system is no guarantee of success in handling Covid-19, as the example of the UK clearly demonstrates. Conversely, Australia is an island nation with a highly decentralized governance system and is regarded as having handled the first two waves of the crisis well, as has Germany, a decentralized and largely landlocked country, along with Austria, a decentralized and totally landlocked country. As such, anecdotal examples suggest that different countries with different economic geographies and governance features appear to have both successfully and unsuccessfully managed the crisis. This begs the question regarding the relative roles that different aspects of economic geography, institutional and governance systems play in shaping the geography of the Covid-19 pandemic.
METHODOLOGY AND DATA
In the preceding sections, we have outlined the various arguments which have been put forward as to the potential or likely relationships and associations between key variables and Covid-19 local death rates. However, the rapid and unprecedented nature of the pandemic has meant that many of these arguments are necessarily partial and somewhat speculative, based on different bits of evolving evidence in different contexts. As such, in the rest of the paper, we adopt an inductive, exploratory empirical approach utilizing the most detailed data available across countries and regions. Along with many other papers, our suggestions as to the likely relationships are also somewhat partial. Therefore, we do not have any strong priors or ex-ante hypotheses as to how local Covid-19 rates are related to either economic geography or institutions, but rather we examine the data on each of these points in order to identify which relationships appear to be the most relevant. In order to do this, we develop a multilevel nested hierarchical model. These models are sometimes criticized by economists because of the difficulties involved in identifying causality. However, in the case of Covid-19, there is nothing behavioural in the interpersonal transmission mechanisms in the way that social scientists investigating causality might think, for example, about sorting processes, self-selection mechanisms or rational expectations-type behaviour. The transmission mechanisms and the competition between variant mutations are purely biochemical in nature, although the scale of transmission and consequent mortality rates may be enhanced by the geographical and institutional context, exactly as we are investigating here. In this context, a multilevel mixed-effects model with random intercepts but fixed slope coefficients is the most appropriate approach. The models we estimate are specified as three-level hierarchical models (models 2, 4 and 6) and two-level hierarchical models (models 1, 3 and 5), respectively.
The three-level hierarchical models take the form of a simple regression developed for each local (small regions) area i. The model assumes that we have data grouped in different levels j (large regions); and k (countries) with a different number of individuals in each group nj and nk:
where Yij is the dependent response variable measured for ith local area unit (i = 1, … , ni) nested within the jth regional area unit (j = 1, … , nj) and the kth national area unit (k = 1, … , nk); Xij is the value on the level-2 predictor (regional level); Xik is the value on the level-3 (country level) predictor; β0 is the intercept; β1 is the regression coefficient associated with Xijk for the ith level-1 unit (small regions or local level); β2 is the regression coefficient associated with Xjk for the jth level-2 unit (large regions level); β3 is the regression coefficient associated with Xk for the kth level-3 unit (national level); eijk is the random error associated with the individual level residual ith level-1 unit (local area); ujk is the random error associated with the meso level residual jth level-2 unit (regional area); and υk is the random error associated with the kth level-3 unit (national area).
Our empirical approach uses a multilevel hierarchical model to capture the effects of different factors operating at different spatial scales that are nested within one another (Knoben et al., Citation2016; van Oort et al., Citation2012). This approach is appropriate because, as we have seen from the above discussion, there are arguments that suggest that different factors operating at the national, regional and local scales may simultaneously influence the deaths associated with the local spread of the virus. Hierarchical multilevel modelling allows us to model these simultaneous influences, thereby taking into account both the higher order contextuality of local responses (Knoben et al., Citation2016; van Oort et al., Citation2012) and any unobserved heterogeneity random coefficients. While standard regression models are designed to model the mean, hierarchical or multilevel models are designed to explicitly model variances (De Leeuw & Meijer, Citation2007; Goldstein, Citation2003). In the particular context of the regional impacts of Covid-19, it is necessary to use multilevel hierarchical models because the grouped data violate the assumption of the independence of all observations (Corrado & Fingleton, Citation2012; van Oort et al., Citation2012). We proceed with a multilevel mixed-effects model with random intercepts, and the model statistics of the resulting estimated models confirm that the data are indeed hierarchically structured and that a multilevel model is the appropriate approach. Our results also incorporate grand mean centring predictors to avoid potential multicollinearity problems between predictor variables. The resulting raw and centred models are equivalent, but multilevel models with centred predictors tend to be more stable, and estimates from these models can be treated as more or less independent from each other, which is desirable in our applied setting.
lists our variables; provides the summary statistics. The data we use are framed in the context of the OECD territorial levels (TL) spatial classification of regions. We employ data at the OECD-Territorial Level 1 (OECD-TL1), representing the national-level data; at OECD-TL2, which represents the large subnational regions; and at OECD-TL3, which represents the small subnational regions. The definition of OECD-TL3 regions is identical to the EU NUTS-3 regions,Footnote2 whereas OECD-TL2 regions differ from EU NUTS-2 regions.Footnote3 For our response variables of Covid-19 death rates, we examine data at OECD-TL3, the smallest comparable spatial units across European countries. These 1293 OECD-TL3 areas are nested within 203 larger OECD-TL2 areas, which are nested within 25 national-level areas. In the various estimated models, we also integrate data at both the OECD-TL2 regional and OECD-TL1 national area levels along with OECD-TL3 data for our independent explanatory variables.
Table 1. Definition of variables.
Table 2. Descriptive statistics
In order to run our models examining the regional levels of mortality associated with Covid-19 across OECD-TL3 European regions during the first half of 2020, it is necessary to integrate different types of data. In each case, we use the most recently published and spatially disaggregated data applicable to and consistent across all European countries and regions. The geographical and institutional influences on the spread and mortality rates of the pandemic are likely to relate both to the national economic and governance systems as well as to the local economic and governance characteristics.
We employ different sets of dependent response variables. The first dependent variable to be estimated is the standard deviation of the number of weekly excess deaths at the OECD-TL3 regional levels during the period from 1 January 2020 (week 1) to the end of the first week of June 2020 (week 24), and also from 1 March 2020 (week 10) to the end of the first week of June 2020 (week 24). These data capture the effects of the first wave of the pandemic. More specifically, we use the relative age-standardized mortality rates (rASMRs) as expressed as the percentage change per week in 2020 from the average annual age-standardized mortality rate in 2015–19. We then calculate the standard deviation of these weekly percentage changes for both periods of weeks 1–24 of 2020 and also of weeks 10–24 of 2020. The data we employ are compiled by Eurostat and by the UK Office for National Statistics (ONS),Footnote4 and the excess deaths are standardized considering the age distribution of the country and the region, and the average age-standardized mortality rates for each week of the year exhibited between 2015 and 2019. These data are denoted in as ‘sd_01_24’ and ‘sd_10_24’, respectively.
The second set of dependent variables we employ is the standard deviation of excess deathsFootnote5 during the whole pandemic from January to 22 November 2020 (week 47),Footnote6 reported in terms of percentages of the local OECD-TL3 population. We then calculate the standard deviation of the weekly excess deaths as a percentage of the local population for the period weeks 1–47 of 2020. These data are generated by the European Data Journalism programme building on Eurostat and other sources (Guibourg, Citation2020),Footnote7 and these data allow us to consider the combination of both the first wave and also the onset of the subsequent second wave of the pandemic, by which time countries had learned a great deal about how to cope with Covid-19, and they also had the possibility to adjust their institutional, governance and security responses to the pandemic. These data are denoted in the empirical estimations reported in as ‘sd_Excess Deaths’.
Our dependent variables are all calculated on the basis of slightly different definitions of excess death rates. and we report estimations using each of these different definitions. The calculation of Covid-19 deaths varies across countries due to different death registration rules and processes, whereas using excess deaths, and in our case various different definitions of excess deaths, provides directly comparable measures that are comparable across countries and are also very consistent with other measures.Footnote8 Moreover, this measure also allows for the fact that the indirect and unintended consequences of lockdowns measures will also cause additional deaths in some cases and lower deaths in other cases, thereby providing a holistic measure of the true impact of both the pandemic and also societal responses to it.
For all our dependent variables, and in order to allow for the different patterns of the initial and largely unanticipated pandemic shocks on each region, we calculate the standard deviation of the reported weekly excess deaths during the earlier periods of the first wave of the pandemic. We prefer to examine the standard deviation of the excess deaths rather than simply the total excess deaths because this index of dispersion provides more subtle information regarding the performance of a country or region in tackling the epidemic during the rapid and largely unanticipated surges and spikes of the first two waves of the pandemic. The pandemic was characterized by rapid upswings and spikes in excess death rates, spikes which took place at different scales and times in different places and the standard deviation captures these types of differential changes. This is important because it is the primarily the severity of the upswings and the spikes in Covid-19 cases which puts the severe pressure on public and social infrastructure and disrupts normal life and also spurs governance responses.
For our OECD-TL3 independent explanatory variables, each of the OECD-TL3 regions is classified using the OECD’s Urban–Rural TypologyFootnote9 into one of five categories describing the nature of the locality: metro large; metro; non-metro close to small city; non-metro close to metro; and non-metro remote. We might expect that the larger metropolitan areas are more susceptible to Covid-19 transmission and mortality rates than smaller and more sparely located places (Kulu & Dorey, Citation2021). At OECD-TL3, we also use gross domestic product (GDP) per capita in US$ at constant prices and constant purchasing power parity (PPP), the base year 2015. This is our index of the local economy’'s strength and local prosperity. In some countries (IHE, Citation2021; Kulu & Dorey, Citation2021) there is evidence that poorer places exhibit higher Covid-19 death rates, so with this argument we might expect Covid-19 death rates to be inversely related to GDP per capita. We use both the local OECD-TL3 population density and the number of in-migrants per year into each OECD-TL3 area, calculated as both the percentage of newcomers relative to the population and the net migration flowsFootnote10 as a share of the population. These indicators capture the local viral transmission potential based on the intra-regional face-to-face intensity of interactions and interregional openness. We might expect that higher values of these indicators are associated with higher transmission and death rates. Finally, in the OECD-TL3 area, we use the share of activity and employment in 11 local sectors. For each OECD-TL3 area, we calculate the Herfindahl indices of both local output and employment across 11 industries in order to capture the local industrial structures. This is in order to see if structural effects play any role in terms of mobility responses to lockdown (Heroy et al., Citation2021). At the higher OECD-TL2s in which all OECD-TL3 areas nested, in addition, we use data on two social exclusion indicators, namely, the percentage of school-leavers not in employment, education or training (NEETs), as well as the percentage of local early school leavers. In principle, one might expect that regions with higher social exclusion levels or inequality might see lower levels of civic cooperation in achieving communal goals relating to fighting the pandemic.
Meanwhile, at OECD-TL2, in order to capture social capital, social cohesion and citizens’ willingness to act constructively and cooperatively to achieve common goals, we use the share of voter turnout in national elections (Putnam, Citation1993, Citation1995). In terms of the level of health service provision at the OECD-TL2 regional level, we use the number of doctors per 1000 population, on the simple assumption that higher numbers of doctors may help to reduce death rates. The quality of the environment may also be important in terms of the prevalence of respiratory-related illnesses, of which Covid-19 appears to be a contributor. To capture this, for each OECD-TL2 area we use the levels of air pollution, as defined in terms of the index of tiny airborne particles or droplets of a width of ≤ 2.5 µm, the major form of pollution-related mortality (The Guardian, Citation2021). We might expect that higher local pollution scores will be associated with higher local death rates. Finally, for each OECD-TL2 region, use the regional quality of government (RQG) index (Charron et al., Citation2019)Footnote11 for 2017. This provides us with a detailed quantitative assessment of the quality of local and regional government institutions. These data complement the various other regional autonomy indices we employ as described below, in that they focus on the quality of the subnational institutions rather than the structure of the subnational government system. If decentralized governance is an important feature of Covid-19 responses, then one might expect that a higher quality of subnational government is associated with lower local death rates.
In terms of our national-level variables, there are various geographical features of a country that we might expect to be positively associated with the spread of Covid-19 in all regions, namely the overall national population density and the country’'s openness. Therefore, we include the 2019 national population density as a country-specific variable, as a proxy for the virus's ability to be transmitted interpersonally. We also incorporate international transport data to capture the potential scale and speed with which the virus is transmitted across borders (Pana et al., Citation2021). We can break down international passenger travel by modes of transportation, namely the number of annual international air transport passengers (WATS-IATA, Citation2019), the annual number of international rail transport,Footnote12 and sea transport passengers (Eurostat, Citation2020). For both the WATS-IATA and EU passenger transportation data, the most recent comprehensive data are for 2018. In order to allow for the potential nationwide effects of concentrations of deprivation shaping both interregional and national impacts (IHE, Citation2021), we employ the 2017 indices of interregional inequality for each European country using a Theil index calculated at OECD-TL3 (Carrascal-Incera et al., Citation2020), which accounts for both the between and within local inequalities. If interregional inequality is associated with higher Covid-19 death rates, this should be picked up by the Theil index. In terms of the ability of the country’s health service to successfully treat people to overcome the virus and to reduce mortality, we employ the 2016 health accessibility and quality (HAQ) index, as reported by Lozano et al. (Citation2018). This index takes into account both the quality of healthcare present in the country and the ability of individuals to access these services across the country. The most recent data are available for 2016, and we might expect that lower HAQs will be associated with higher local Covid-19 death rates.
As already mentioned, different countries have different institutional and governance systems that can act as mediators in how the central and regional or local governments interact and how policies are developed and deployed. To capture these differences across European countries, we employ three different measures. Firstly, we use the 2014 local autonomy index (LAI) of the decision-making capabilities of local levels of subnational government (Ladner et al., Citation2019; OECD, Citation2019). If enhanced very local governance powers increase our ability to respond to the Covid-19 shocks at the local level, then higher LAIs ought to be associated with lower Covid-19 death rates. Second, we use the newly published Version 3 of the regional authority index (RAI) (Marks, Citation2020). The construction of the RAI 2016.V2 (Hooghe et al., Citation2016; Hooghe & Marks, Citation2016) has very recently been updated and adjusted to account for different subnational domains of power and effectiveness in the new 2018 RAI V3 (Shair-Rosenfield et al., Citation2020). The newly updated RAI gives us an overall measure of the extent to which subnational government institutions at the wider regional level have decision-making power in a variety of different roles. As our explanatory variable, we take the (natural log of the) ratio of the national population divided by 1 + RAI to reflect the levels of regional governance decentralization relative to population. In general, larger countries tend to be more devolved and decentralized than smaller countries, but there are exceptions to this pattern. If more devolved subnational governance relative to the size of the country helps to better respond to local Covid-19 shocks, then a lower value of the (log of) population divided by 1 + RAI will be associated with lower local Covid-19 death rates, whereas if more centralized societies are better able respond, then a higher value will be associated with lower excess death rates. Third, we use the 2019 World Bank indices of national government effectiveness (Kaufman & Kraay, Citation2020). For each country, the Covid-19 pandemic is a national emergency, and national governments that are more effective might be expected to be better able to respond to the emergency than less effective governments. As such, we would expect that higher levels of national government effectiveness are likely to be associated with lower Covid-related deaths.
Besides national geographical and institutional-governance indicators, we also employ four measures that capture the severity of the Covid-19 pandemic lockdown’ measures instituted by national governments across a range of social, legal and security dimensions. These data come from the Oxford stringency index (OSI)Footnote13 produced by the Coronavirus Government Response Tracker Project at the Blatavnik School of Government at the University of Oxford (Petherick et al., Citation2020). The data are reported daily for each country from the onset of the pandemic crisis, and we use these data in various ways. First, we calculate the average daily OSI between weeks 4 and 24 of 2020. The beginning of week 4, namely Monday, 20 January, was the first date on which any European country, namely the UK, imposed any form of anti-pandemic measure. All other interventions across Europe were subsequent to this. We also use the OSI for each country on 31 March 2020, because this is the first date by which all European countries had implemented their most stringent regime. In addition, we count the number of days before 31 March 2020 by when a country instituted its most stringent lockdown regime, and we also count the number of days before 31 March 2020 when a country first instituted some lockdown-related restrictions. As of 1 March 2020, no country in Europe had Covid-19-related deaths of more than one person per 1 million population (Financial Times, Citation2020), but the following weeks the pandemic deaths escalated across Europe at an astonishing speed. Finally, we calculate the average daily OSI between weeks 4 and 47, that is, from 20 January to 22 November 2020. Therefore, these four data elements provide information about both the severity and the speed of the national response to the threat of the virus. In principle, we might expect that a more stringent lockdown regime implemented at an earlier date would be associated with fewer excess deaths, but this may also depend on the timing of when interventions were first imposed or the speed with which measures became more stringent. These data capture each of these institutional dimensions.
EMPIRICAL RESULTS
provides the first sets of multilevel results based on a multilevel mixed-effects model with random intercepts, for which all the variables are available at each of the three nested tiers of spatial units.
Table 3. Multilevel hierarchical OECD-TL3 area models of European-wide regional Covid-19-related excess deaths.
In models 1 and 2 of , the dependent variable is the standard deviation of the daily excess deaths at the local OECD-TL3 region from 1 January 2020 (week 1) to the end of the first week of June 2020 (week 24). In models 3 and 4, the dependent variable is the standard deviation of the daily excess deaths at the local OECD-TL3 region from 1 March 2020 (week 10) to the end of the first week of June 2020 (week 24). In models 5 and 6, the dependent variable is the standard deviation of the total weekly excess deaths at the local OECD-TL3 region level as a percentage of the total local population between 1 January and 22 November 2020 (week 47). Models 1, 3 and 5 estimate the hierarchical multilevel model by combining information at OECD-TL2 and OECD-TL3, while models 2, 4 and 6 estimate the models at OECD-TL1–TL3 combined. These different models allow us to identify the extent to which national-level influences change what is observed at lower levels.
In , we see that many variables are not significant. Most of the coefficient signs are largely as would be expected, but many of the individual coefficients are not significant, and this may simply reflect the ubiquity of the Covid-19 virus. At OECD-TL3, there is evidence that more specialized regions exhibit lower death rates (models 1, 3 and 5). In terms of urban or rural structures, from week 10 onwards, large metros (models 4–6) display significantly higher per capita excess deaths than other types of regions.Footnote14 Meanwhile, in models 1–6, the excess death rates are also negatively related to net flows. In other words, regions which before the coronavirus pandemic enjoyed relatively large net annual population inflows appear to display lower excess death rates during the first wave of the pandemic, whereas those with population outflows tend to experience higher excess deaths. Across Europe, high net out-migration tends to be associated with low local social well-being (Faggian et al., Citation2012; Faggian & Royuela, Citation2010; Rijnks et al., Citation2018). In other words, places with lower social well-being are associated with higher death rates.
At the higher OECD-TL2 region scale, the number of physicians (doctors) per 1000 local population is negatively related to excess deaths in models 1 and 3, although these effects disappear once we control for OECD-TL1 national HAQ scores. In terms of social deprivation, in models 2 and 4, the share of NEETS is negatively related to total local Covid-19-related excess deaths during the early stages of 2020, and pollution (PM2.5) is seen to be positively related to Covid-19 deaths in models 2, 5 and 6.
At the national OECD-TL1 level, the HAQ index is positively associated with excess deaths in the pandemic’'s first two waves (models 2, 4 and 6), as is the number of international travellers per capita (models 2 and 4). In terms of the stringency of the national lockdown restrictions, the highest value of stringency (Max OSI) is associated with lower death rates (models 2 and 4), although there is tentative evidence that earlier interventions (Days Before Max OSI Index; Days to First OSI Index Intervention) appear to be associated with higher death rates (model 1). This is likely to reflect the response to the emerging crisis as much as any causal relationship. Meanwhile, better national governance effectiveness is clearly associated with lower death rates (models 2, 4 and 6), and there is also strong evidence that higher local governance autonomy (LAI) is associated with lower death rates (models 2, 4 and 6). Finally, higher (Theil) interregional inequality also appears to be associated with lower death rates (models 2 and 4).
We can now repeat precisely the same exercises as reported in , but this time we use a grand mean-centred response variable (Paccagnella, Citation2006). The results are reported in . We see that when we move from non-centred multilevel models to grand mean centred models, the results for OECD-TL3 and OECD-TL2 variables are barely affected at all; almost the exact same selection of variables remains significant, and their coefficients only differ very slightly. Only the number of doctors per 1000 local population changes being significant from models 1 and 3 to models 2 and 4.
Table 4. Grand mean centred OECD-TL3 area multilevel hierarchical models of European-wide regional Covid-19-related excess deaths.
The only coefficients that differ in their significance are all in the OECD-TL1 national variables. If we compare with , we see that in the grand mean-centred models, at the national level, the number of international travellers per capita and the HAQ index of health accessibility and quality both change signs from positive to negative while remaining significant. National population density now also becomes positively related to local excess deaths. The number of days before 31 March 2020 that the first lockdown interventions were instituted now also becomes negatively related to excess deaths instead of the maximum level of lockdown stringency. Similarly, a more centralized subnational governance structure relative to population now also becomes associated with higher death rates.
In and , we estimate a two-level hierarchical model (non-centred and grand mean centred, respectively), with the lowest level being OECD-TL2 regions. This now allows us also to include Germany in the analysis because Covid-19-related regional death rates are only reported in Germany at OECD-TL2s of the German Länder. When comparing and with and at OECD-TL2, we see that broadly the same variables remain significant. Meanwhile, at the OECD-TL1 national level, precisely the same variables remain significant and with the same coefficient signs, except for the per capita number of international travellers, the HAQ index and the log of the ratio of the population over the regional authority index, all of which are now insignificant.Footnote15 Obviously, these OECD-TL2 area two-level models are much less detailed than the OECD-TL3 area three-level models, but at least these comparisons confirm high levels of stability in the models and the overall applied hierarchical framework.
Table 5. OECD-TL2 area multilevel hierarchical models of European-wide regional Covid-19-related excess deaths.
Table 6. OECD-TL2 area grand mean centred multilevel hierarchical models of European-wide regional Covid-19-related excess deaths.
All our models display chi-squared scores that are significant, which means that the data display the nested hierarchical structure, such that multilevel hierarchical models are indeed appropriate for analysing these data.
One of the issues we observe is that the RQG index is not found to be significant in any of our models, although the degrees of subnational regional governance authority and also the decentralized powers of local government are significantly associated with lower Covid-19-related deaths. In order to further examine these issues, we estimate another 88 multilevel models of different forms where we allow for multilevel interactions (Knoben et al., Citation2016; van Oort et al., Citation2012) at the TL2 and TL1 levels between RQG and a range of other national-level variables. The results of these 88 additional models are reported in the supplemental data online, and they demonstrate that our findings reported here are very robust and stable. Therefore, it would appear that national government effectiveness, the levels of devolved subnational regional governance and also the levels of local government autonomy dominate any regional RQG effects. This would be consistent with the argument that in this type of crisis, the role and performance of the national government is critical, as is the ability also to make decisions both regionally and very locally.
CONCLUSIONS
For European countries, the excess local death rates associated with the Covid-19 pandemic are found to be a function of both geographical and institutional features at the local, regional and national levels. Broadly, a mixture of low local levels of urbanization, effective national governance, good local health service provision, decentralized subnational and local governance, low air pollution, and the early implementation of severe lockdown measures, all combine to reduce Covid-19 related excess deaths. On the basis of our results for regional GDP per capita and Theil regional inequality, we do not find any relationship between excess death rates and regional incomes, a finding which is consistent with the national-level findings of Deaton (Citation2021). Nor do we find any role for the quality of regional government, but rather the effectiveness of national government and the levels of regional and local governance devolution appear to dominate. The usual caveats regarding multilevel approaches remain, especially relating to causality. However, the novelty, unprecedented scale and speed of the Covid-19 pandemic have meant that our causality understanding of many aspects of the pandemic is still only emerging slowly. Yet, whereas most of the focus of the media attention in different countries tends to be on national policy decisions, our results also imply that the interactions between economic geography and the institutional set-up are crucial determinants of the regional patterns of deaths, and that a multilevel hierarchical approach is the appropriate methodology for examining these issues. National-level factors shape the role of regional level features, which in turn shape the impacts of very local characteristics. As such, purely national-, regional- or local-level analyses or narratives fail to fully capture the geography of Covid-19, which is genuinely multilevel in nature.
Supplemental Material
Download PDF (916.5 KB)DISCLOSURE STATEMENT
No potential conflict of interest was reported by the authors.
Additional information
Funding
Notes
2. OECD-TL3 regions and EU NUTS-3 regions all coincide exactly for 22 European countries. There are 1067 OECD-TL3 regions within the EU, plus 179 OECD-TL3 regions in the UK, 26 in Switzerland, 18 in Norway and two in Iceland. The average size of European OECD-TL3 regions is a population of just over 400,000 people, and for European OECD-TL2 regions is just over 2.5 million people, whereas the average for EU NUTS-2 regions is just below 2 million people and for EU NUTS-1 regions is 5.6 million.
3. The figures for Germany are only reported at OECD-TL2. The OECD-TL2 regions coincide exactly with the EU NUTS regions, except for Belgium, France, the UK and Germany, in which cases the OECD-TL2 regions coincide with the EU NUTS-1 regions.
5. The data are reported as the percentage excess deaths for the three periods of spring, summer and autumn 2020, relative to the population. To calculate the standard deviation using these percentage scores, we use the mean variation across all three time periods and all regions along with the three individual seasonal deviations for each region.
6. The data are reported to up to week 47 for all regions in Spain, France, the UK, Sweden, Lithuania, Portugal and Belgium, except for Italy (week 40), Finland (week 46), Denmark (week 48), the Netherlands (week 46), Latvia (week 48), Slovakia (week 43), Poland (week 44) and the Czech Republic (week 44).
7. The data are available at https://gitlab.com/newsworthy/jpp.2005.excess.deaths.data/.
8. For example, in the case of the UK, as of 27 January 2021, there were 99,211 mortalities calculated on the basis of excess deaths, 100,162 deaths within 28 days of a positive Covid-19 test, and 103,602 deaths which mentioned Covid-19 on the death certificate; see https://www.bbc.co.uk/news/uk-55820178/.
10. Net migration flows equal inflows minus outflows divided by the population, for the most recent year available immediately preceding the pandemic.
11. The EU Quality of Government (QOG) data are calculated at the EU NUTS-2 level regions, which correspond exactly to the OECD-TL2 regions in 19 countries and 143 regions, except for the cases of the UK, Germany, France and Belgium, where the OECD-TL2 regions coincide with the EU NUTS-1 regions. In the UK, there are 12 OECD-TL2 regions and 39 NUTS-2 regions; in Germany, there are 16 OECD-TL2 regions and 38 NUTS-2 regions; in France, there are 13 OECD-TL2 regions and 22 NUTS-2 regions; and in Belgium, there are three OECD-TL2 regions and 11 NUTS-2 regions. The OECD-TL2 and TL3 regions are based on subnational governance systems, whereas the NUTS-2 regions are based on EU Cohesion Policy funding allocation rules. For present purposes in this paper, the OECD-TL2 and OECD-TL3 regions are therefore superior unit of analysis, given that governance is one of the central issues we are investigating. In these four country cases of the UK, Germany, France and Belgium, in order to translate the NUTS-2 QOG data to OECD-TL2 data, we simply compute the average QOG scores for the NUTS-2 regions nested within the OECD-TL2 regions, weighting them by population.
12. The international rail passenger data for the Netherlands is not published by the semi-private Dutch rail operator NS, so our Netherlands rail data come from the NS report; see https://nieuws.ns.nl/vier-miljoen-reizigers-in-2019-per-trein-de-grens-over/.
14. Rural regions are the baseline category, and the coefficients are increasing with the scale of the urban settlement.
15. We have also run estimations in which the average OSI score (OECD, Citation2021) until November 2020 is also included, but these were not significant in any OECD-TL3 or OECD-TL2 specifications, although they do reduce the importance of both government effectiveness and local governance autonomy.
REFERENCES
- Bailey, D., Clark, J., Colombelli, A., Corradini, C., De Propris, L., Derudder, B., Fratesi, U., Fritsch, M., Harrison, J., Hatfield, M., Kemeny, T., Kogler, D. F., Lagendijk, A., Lawton, P., Ortega-Argilés, R., Otero, C. I., & Usai, S. (2020). Regions in a time of pandemic. Regional Studies, 54(9), 1163–1174. https://doi.org/https://doi.org/10.1080/00343404.2020.1798611
- Berube, A. (2020, April 29). Which city economies did COVID-19 damage first? https://www.brookings.edu/blog/the-avenue/2020/04/29/which-city-economies-did-covid-19-damage-first/?utm_campaign=Brookings%20Brief&utm_source=hs_email&utm_medium=email&utm_content=87187919
- Bourdin, S., Jeanne, L., Nadou, F., & Noiret, G. (2021). Does lockdown work? A spatial analysis of the spread and concentration of covid-19 in Italy. Regional Studies, 55(7), 1182–1193. https://doi.org/https://doi.org/10.1080/00343404.2021.1887471
- Bourdrel, T., Annesi-Maesano, I., Alahmad, B., Maesano, C. N., & Bind, M. A. (2021). The impact of outdoor air pollution on COVID-19: A review of evidence from in vitro, animal, and human studies. European Respiratory Review, 30(159), 200242. https://doi.org/https://doi.org/10.1183/16000617.0242-2020
- Carrascal-Incera, A., McCann, P., Ortega-Argilés, R., & Rodriguez-Pose, A. (2020). UK interregional inequality in a historical and international comparative context. National Institute Economic Review, 253, R4–R17.
- Charron, N., Lapuente, V., & Annoni, P. (2019). Measuring quality of government in EU regions across space and time. Papers in Regional Science, 98(5), 1925–1953. https://doi.org/https://doi.org/10.1111/pirs.12437
- Chaudhry, R., Dranitsaris, G., Mubashir, T., Bartoszko, J., & Riazi, S. (2020). A country level analysis measuring the impact of government actions, country preparedness and Socioeconomic factors on COVID-19 mortality and related health outcomes. eClinical Medicine, 25), https://doi.org/https://doi.org/10.1016/j.eclinm.2020.100464
- Committee of the Regions (CoR). (2020, October 2). EU annual regional and local barometer: Full Report. European Committee of the Regions.
- Corrado, L., & Fingleton, B. (2012). Where is the economics in spatial econometrics? Journal of Regional Science, 52(2), 210–239. https://doi.org/https://doi.org/10.1111/j.1467-9787.2011.00726.x
- De Leeuw, J., & Meijer, E. (Eds.). (2007). Handbook of multilevel analysis. Springer.
- Deaton, A. (2021). Covid-19 and global income inequality. Institute for Fiscal Studies. https://www.ifs.org.uk/inequality/covid-19-and-global-income-inequality/
- Doerr, S., & Gambacorta, L. (2020, May 15). Covid-19 and regional employment in Europe. BIS Bulletin No. 16. Bank of International Settlements (BIS). https://www.bis.org/publ/bisbull16.htm
- European Observation Network for Territorial Development and Cohesion (ESPON). (2020, December). Geography of COVID-19 outbreak and first policy answers in European regions and cities. Policy Brief, December. ESPON.
- Eurostat. (2020). Passenger transport statistics: Statistics Explained 2020–07. https://ec.europa.eu/eurostat/statistics-explained/index.php?title=Passenger_transport_statistics&oldid=503247
- Faggian, A., Olfert, M. R., & Partridge, M. D. (2012). Inferring regional well-being from individual revealed preferences: The ‘voting with your feet’ approach. Cambridge Journal of Regions, Economy and Society, 5(1), 163–180. https://doi.org/https://doi.org/10.1093/cjres/rsr016
- Faggian, A., & Royuela, V. (2010). Migration flows and quality of life in a metropolitan area: The case of Barcelona, Spain. Applied Research in Quality of Life, 5(3), 241–259. https://doi.org/https://doi.org/10.1007/s11482-010-9108-4
- Fang, W., & Wahba, S. (2020, April 20). Urban density is not an enemy in the coronavirus fight: Evidence from China. https://blogs.worldbank.org/sustainablecities/urban-density-not-enemy-coronavirus-fight-evidence-china
- Financial Times. (2020). Coronavirus tracked. Financial Times. https://ig.ft.com/coronavirus-chart/?areas=usa&areas=gbr&areasRegional=usny&areasRegional=usca&areasRegional=usfl&areasRegional=ustx&areasRegional=usnd&areasRegional=ussd&byDate=1&cumulative=0&logScale=1&per100K=1&values=deaths
- Franch-Pardo, I., Napoletano, B. M., Rosete-Verges, F., & Billa, L. (2020). Spatial analysis and GIS in the study of COVID-19. A review. Science of the Total Environment, 739, article 140033. https://doi.org/https://doi.org/10.1016/j.scitotenv.2020.140033
- Frey, W. H. (2020, April 10). Who lives in the places where coronavirus is hitting the hardest? Brookings. https://www.brookings.edu/blog/the-avenue/2020/04/10/who-lives-in-the-places-where-coronavirus-is-hitting-the-hardest/?utm_campaign=brookings-comm&utm_source=hs_email&utm_medium=email&utm_content=86280769
- Goldstein, H. (2003). Multilevel statistical models (3rd Ed.). Edward Arnold.
- Guibourg, C. (2020). A fraction of European regions account for a majority of Covid deaths. https://medium.com/newsworthy-se/a-fraction-of-european-regions-account-for-a-majority-of-covid-deaths-778e546765a9
- Heroy, S., Loaiza, I., Pentland, A., & O’Clery, N. (2021). COVID-19 policy analysis: Labour structure dictates lockdown mobility behaviour. Journal of the Royal Statistical Society: Interface, 18(176), article 20201035. https://doi.org/https://doi.org/10.1098/rsif.2020.1035
- Hooghe, L., & Marks, G. (2016). Community, scale, and regional governance: A postfunctionalist theory of governance, Volume II. Oxford University Press.
- Hooghe, L., Marks, G., Schakel, A. H., Niedzwiecki, S., Chapman-Osterkatz, S., & Shair-Rosenfield, S. (2016). Measuring regional authority: A postfunctionalist theory of governance, Volume I. Oxford University Press.
- Institute of Health Equity (IHE). (2021). Build back fairer: The COVID-19 Marmot review. The pandemic, socioeconomic and health inequalities in England. IHE.
- Jamieson, T. (2020). ‘Go hard, Go early’: Preliminary lessons from New Zealand’s response to COVID-19. American Review of Public Administration, 50(6–7), 598–605. https://doi.org/https://doi.org/10.1177/0275074020941721
- Kaufman, D., & Kraay, A. (2020). The worldwide governance indicators (WGI) project. World Bank. http://info.worldbank.org/governance/wgi/
- Kaufmann, D., Kraay, A., & Mastruzzi, M. (2010). The worldwide governance indicators: A summary of methodology, data and analytical issues. World Bank Policy Research Working Paper No. 5430. http://papers.ssrn.com/sol3/papers.cfm?abstract_id=1682130
- Knoben, J., Arikan, A., van Oort, F., & Raspe, O. (2016). Agglomeration and firm performance: One firm’s medicine is another firm’s poison. Environment and Planning A: Economy and Space, 48(1), 132–153. https://doi.org/https://doi.org/10.1177/0308518X15602898
- Kochańczyk, M., & Lipniacki, T. (2021). Pareto-based evaluation of national responses to COVID-19 pandemic shows that saving lives and protecting economy are non-trade-off objectives. Nature: Scientific Reports, 11(1), 2425. https://doi.org/https://doi.org/10.1038/s41598-021-81869-2
- Kulu, H., & Dorey, P. (2021). Infection rates from covid-19 in Great Britain by geographical units: A model-based estimation from mortality data. Health and Place, 67), https://doi.org/https://doi.org/10.1016/j.healthplace.2020.102460
- Ladner, A., Keuffer, N., Baldersheim, H., Hlepas, N., Swianiewicz, P., Steyvers, K., & Navarro, C. (2019). Patterns of local autonomy in Europe. Palgrave Macmillan.
- Lozano, R., et al. (2018, May 23). Measuring performance on the Healthcare Access and Quality Index for 195 countries and territories and selected subnational locations: A systematic analysis from the Global Burden of Disease Study 2016. The Lancet, 391(10136), 2236–2271. https://doi.org/https://doi.org/10.1016/S0140-6736(18)30994-2
- Marks, G. (2020, November 23). Regional authority. https://garymarks.web.unc.edu/ and http://garymarks.web.unc.edu/data/regional-authority/
- Meinen, P., Serafini, R., & Papagalli, O. (2021). Regional economic impact of Covid-19: The role of sectoral structure and trade linkages. Working Paper No. 2528, February. European Central Bank (ECB).
- Muro, M., Maxim, R., & Whiton, J. (2020, March 17). The places a COVID-19 recession will likely hit hardest. Brookings Metropolitan Program. https://www.brookings.edu/blog/the-avenue/2020/03/17/the-places-a-covid-19-recession-will-likely-hit-hardest/?utm_campaign=brookings-comm&utm_source=hs_email&utm_medium=email&utm_content=84875880
- Nathan, M. (2020). The city and virus. Medium, May 15. https://medium.com/@maxnathan/the-city-and-the-virus-db8f4a68e404
- Organisation for Economic Co-operation and Development (OECD). (2012). Perspectives on global development: Social cohesion in a shifting world. OECD.
- Organisation for Economic Co-operation and Development (OECD). (2019). Making decentralisation work: A handbook for policy makers (OECD Multi-level Governance Studies). OECD.
- Organisation for Economic Co-operation and Development (OECD). (2020). The territorial impact of COVID-19: Managing the crisis across levels of government. OECD.
- Organisation for Economic Co-operation and Development (OECD). (2021). Implications of remote working adoption on place based policies: A focus on G7 countries. OECD.
- Oshchepkov, A., & Shirokanova, A. (2020). Multilevel modeling for economists: Why, when and how. Basic Research Program, Working Papers Series: Economics WP BRP No. 233/EC/2020. National Research University, Higher School of Economics. https://papers.ssrn.com/sol3/papers.cfm?abstract_id=3637907&download=yes
- Paccagnella, O. (2006). Centering or not centering multilevel models: The role of the group mean and the assessment of group effects. Evaluation Review, 30(1), 66–85. https://doi.org/https://doi.org/10.1177/0193841X05275649
- Pana, T. A., Bhattacharya, S., Gamble, D. T., Pasdar, Z., Szlachetka, W. A., Perdomo-Lampignano, J. A., Ewers, K. D., McLernon, D. J., & Myint, P. K. (2021). Country-level determinants of the severity of the first global wave of the COVID-19 pandemic: An ecological study. BMJ Open, 11, article e042034. https://doi.org/https://doi.org/10.1136/bmjopen-2020-042034
- Petherick, A., Kira, B., Hale, T., Phillips, T., Webster, S., Cameron-Blake, E., Hallas, L., Majumdar, S., & Tatlow, H. (2020, November 24). Variation in government responses to COVID-19. Blavatnik School Working Paper. https://www.bsg.ox.ac.uk/research/publications/variation-government-responses-covid-19
- Putnam, R. (1993). Making democracy work. Princeton University Press.
- Putnam, R. (1995). Bowling alone: America’s declining social capital. Journal of Democracy, 6(1), 65–78. https://doi.org/https://doi.org/10.1353/jod.1995.0002
- Rijnks, R., Koster, S., & McCann, P. (2018). Spatial heterogeneity in amenity and labor market migration. International Regional Science Review, 41(2), 183–209. https://doi.org/https://doi.org/10.1177/0160017616672516
- Rodriguez-Pose, A., & Burlina, C. (2021). Institutions and the uneven geography of the first wave of the covid-19 pandemic. Journal of Regional Science. https://doi.org/https://doi.org/10.1111/jors.12541
- Shair-Rosenfield, S., Schakel, A. H., Niedzwiecki, S., Marks, G., Hooghe, L., & Chapman-Osterkatz, S. (2020). Language difference and regional authority. Regional and Federal Studies. https://doi.org/https://doi.org/10.1080/13597566.2020.1831476.
- Smit, S., Hirt, M., Buehler, K., Lund, S., Greenberg, E., & Govindarajan, A. (2020). Safeguarding our lives and our livelihoods: The imperative of our time. McKinsey.
- Spencer, D. (2021, February 2). Taiwan’s ban on foreigners is tough but necessary. Taiwan News. https://www.taiwannews.com.tw/en/news/4092030
- Stier, A. J., Berman, M. G., & Bettencourt, L. M. A. (2020). COVID-19 attack rate increases with city size. https://ssrn.com/abstract=3564464
- The Guardian. (2021, February 9). ‘Invisible killer’: Fossil fuels caused 8.7m deaths globally in 2018, research finds. The Guardian. https://www.theguardian.com/environment/2021/feb/09/fossil-fuels-pollution-deaths-research
- van Oort, F., Burger, M. J., Knoben, J., & Raspe, O. (2012). Multilevel approaches and the firm – Agglomeration ambiguity in economic growth studies. Journal of Economic Surveys, 26(3), 468–491. https://doi.org/https://doi.org/10.1111/j.1467-6419.2012.00723.x
- WATS-IATA. (2019). WATS world air transport statistics 2019. International Air Transport Association (IATA). https://www.iata.org/en/publications/store/world-air-transport-statistics/