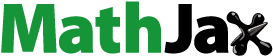
ABSTRACT
We assess the impact of regional policies on the economies of the 20 Italian regions for the period 1994–2016. We adopt a control function approach incorporating the funds’ allocation rules and ascertain the impact of various policy funds in four sectors (agriculture, industry, construction and services) through a multi-input multi-output transformation function. We find that European Structural and Investment Funds have a significant impact on various sectoral components of regional gross domestic product (GDP) per capita, with the European Regional Development Fund (ERDF) playing the strongest role. Nationally funded regional policies do not have an aggregate impact but affect the sectoral composition of GDP.
1. INTRODUCTION
Today, the very deep economic and social disparities that still exist both between countries and regions undermine the unity and cohesion of the European Union (EU). Hence, there is a need to evaluate the appropriateness and effectiveness of development policies implemented through the European Structural and Investment Funds. These Structural Funds (SFs) are, especially since the introduction of Agenda 2000, the EU’s primary tool for sustaining development in areas facing economic problems. For the new programming period 2021–27, €330.2 billion has been allocated in Europe for this policy, almost one-third (30.7%) of the total budget of the EU net of Next Generation EU, €1074.3 billion. All values are at 2018 prices (European Union, Citation2021, p. 29).
More specifically, in this paper we will focus on the following SFs: (1) the European Regional Development Fund (ERDF), created in 1975 with the aim of reducing regional imbalances in the EU; (2) the European Social Fund (ESF), created in 1957 with the aim of promoting training and educational attainment among the labour force, as well as other forms of active labour market policies; (3) the European Agricultural Fund for Rural Development (EAFRD) and the European Agricultural Guarantee Fund (EAGF), created in 2007 with the aim of facilitating the adjustment of agricultural structures and the development of rural areas by substituting the European Agricultural Guidance and Guarantee Fund (EAGGF), which dated back to 1962; and (4) the European Fisheries Fund (EFF), established in 2007 to support the national fishery programmes and substitute for the Financial Instrument for Fisheries Guidance (FIFG), which was created in 1994.Footnote1 It should be noted that, due to its relatively high gross domestic product (GDP) per capita, Italy does not qualify for the EU’s Cohesion Fund. On the other hand, we will fully consider the role of national co-financing. Indeed, in Italy, EU funding only finances up to 50% of the total cost of projects comprised within EU regional policy, with the remaining finance being provided by the central and regional governments under the principle of additionality.
The existence of relevant and persistent delays in development (Allen & Stevenson, Citation1974; Iuzzolino, Citation2009) in a country where significant financial resources have been dedicated to these policies raises issues about their effectiveness and makes Italy a particularly interesting (and worrying) case study. In light of the post COVID-19 stance of the EU, this analysis can help to provide some useful indications for the management of EU funding during the next programming period (2021–27) of the SFs.
Following up on some needs highlighted in the surveys by Fratesi (Citation2016, Citation2020), we stress the importance of identifying effective sectors of intervention and practices for cohesion policies. Hence, our empirical analysis is carried out by separately considering the outputs of four sectoral aggregates: agriculture, industry (which comprises energy and manufacturing), construction and services. A distinctive feature of this analysis is that the sectoral outputs are jointly modelled through a multi-input multi-output transformation function taken from production analysis (Coelli & Perelman, Citation1999; Kumbhakar, Citation2012, Citation2013). Furthermore, we consider, along with SFs, various types of nationally financed funds relevant for regional development.Footnote2 Among these national funds (collectively labelled as NFs) we include current account subsidies for firms, capital account expenditures divided into subsidies for firms and investment expenditures and an aggregate measure of nationally funded cohesion policies comprising the Fondo innovazione tecnologica, Fondo contributo imprese, Fondo solidarietà nazionale and Fondo per lo Sviluppo e la Coesione (FSC, formerly the Fondo per le Aree Sottoutilizzate – FAS).
Summing up, in this paper we assess the impact of SFs and NFs on the outputs of four economic sectors across the Italian regions, comparing their relative effectiveness. This analysis widens the scope of the literature on regional policies along two relatively underdeveloped dimensions (the evaluation of the sectoral effects of policies and the comparison of different policies) and yields clear policy implications with regard to which funds are effective in which sectors. While commenting on our results, we will also suggest some implications of the analysis that have a wider relevance, concerning the debate about the effectiveness of regional policies in the face of the century-old divide between northern and southern Italy. Moreover, we will consider to what extent the present analysis may also provide useful insights outside the Italian economy.
The remainder of the paper is organized as follows. Section 2 provides some background and clarifies the novelty of the present analysis. Section 3 illustrates the empirical procedures and the data, while the results of the empirical analysis are shown and commented on in section 4. Section 5 concludes and sets out some implications for future research.
2. LITERATURE AND BACKGROUND
A vast literature exists on the effectiveness of European regional policy. In most cases, this policy seems to have a positive impact on growth, but the significance of the results is far from uniform. A feature that emerges across various studies is that the policy impact depends on a series of conditioning factors (Fratesi, Citation2016). However, the impact of SFs across sectors has been very rarely analysed. Percoco (Citation2017) considers sectoral structure among the determinants of SFs’ impact on economic activity but does not directly analyse the effects of SFs on sectoral outputs.Footnote3 Coppola and Destefanis (Citation2007, Citation2015) measure the components of sectoral labour productivity change through a non-parametric Free Disposal Hull approach and subsequently regress these components on SFs and other variables. They find that the SFs have an impact on changes in labour productivity, with no systematic difference across sectors. However, their two-step procedure may entail some econometric problems.
The examination of the literature also reveals that EU Cohesion Policy is almost never analysed alongside national policies directed at regional growth and cohesion, probably because of data availability problems. Coppola et al. (Citation2020) and Psycharis et al. (Citation2020) provide the only studies comparing the effectiveness of EU and national cohesion policies with regard to Italian and Greek data, respectively. Interestingly, these two studies, which both ignore sectoral issues, find contrasting results. In Italy, SFs are found to have a much more significant impact on GDP per capita than NFs, while there is no indication that SFs impact regional growth in Greece. On the contrary, nationally funded public investments boosted growth in the Greek regions, at least before the Great Recession of 2008.
There is also a dearth of studies concerning the mechanisms presiding the allocation of funds across regions. As we argue in the following section, knowledge of these mechanisms should allow a better treatment of the selection bias. The allocation of funds may not be based solely on economic mechanisms but may also derive from redistributive motives of a political nature (Boldrin & Canova, Citation2001). Identifying the determination of the differences in the distribution of funds is conducive to a better understanding of the conditions under which they operate and to the development of policy proposals to improve their performance. However, scientific reflection has focused on this issue far less than on the impact of the funds themselves. Also note that the literature to be surveyed below almost exclusively focuses on EU funds. Yet, there is no reason why nationally funded policies should not be investigated using the same kind of analysis. In the following section, accordingly, we will consider the allocation mechanisms of both SFs and NFs.
For Kemmerling and Bodenstein (Citation2006), the political orientation of regional governments affects the amount of allocated funds. According to them, parties that have a regionalist rather than a national vocation are more effective in interacting directly with the European Commission through intense lobbying. Bouvet and Dall’erba (Citation2010) highlight the role of ‘political alignment’ between the orientation of the central government and the regional governments. Alignment facilitates the decision-making process directed at the appropriation and use of funds. Both Kemmerling and Bodenstein and Bouvet and Dall’erba also consider the role of sectoral variables in the allocation of funds across regions.
A related branch of the literature has focused on a more normative analysis of the allocation of EU funds across regions. Its main topic is the (lack of) alignment between an optimal spatial allocation of funds, reflecting the geography of structural disadvantages across EU regions, and the actual allocation of funds determined by EU regional policy. Crescenzi (Citation2009) finds a ‘spatial mismatch’ along these lines for both the programming periods 1994–99 and 2000–06, arguing that this misalignment may imply a reduction in the impact of the funds. According to Dotti (Citation2013) the multilevel governance of EU regional policy unleashes a bargaining process between the EU bodies, national governments, local governments and various pressure groups, which produces both the above-defined misalignment and an unstable allocation of funds over time. Crescenzi et al. (Citation2015) extend the analysis to include the allocation of EU regional, rural development and agricultural funds and to assess these resources’ territorial coordination as well as their consistency with the aim of territorial cohesion. According to their analysis, there are limited synergies among policies, and the distribution of overall community spending across policies and territories is only weakly compatible with the objective of territorial cohesion.
Del Bo and Sirtori (Citation2016) analyse the existence of a distorted allocation of funds across Italian regions, deviating from the distribution across regions or sectors that would have been achieved by a welfare maximizing social planner. Their results confirm, to some extent, the presence of regional and sectoral distortions. Del Bo and Sirtori also find strong substitution effects between European and NFs. Finally, also according to Crescenzi et al. (Citation2017) and Di Cataldo and Monastiriotis (Citation2020), the correspondence between the needs of a region and the actual policy implemented is an important determinant of policy success. Concentrating funds on the weak features of a region makes their expenditure more effective.
3. THE EMPIRICAL FRAMEWORK
3.1. Conceptual background
The basis of our empirical framework is an outcome equation, determining GDP per capita as a function of policy funds and other variables, which is compatible with both Solow’s neoclassical approach and with other growth models (on this, see Puigcerver-Peñalver, Citation2007). Following Coppola et al. (Citation2020), we deal with the selection bias problem inherent to policy evaluation along the lines of the control function approach (Heckman & Hotz, Citation1989; Wooldridge, Citation2004), assuming that policy funds are randomly allocated, conditional on a vector of observable covariates (that will be denoted here by Wit−1). Vector Wit−1 includes the variables presiding over the regional allocation of the funds, selected through the estimation of a set of auxiliary regressions in which SFs and NFs are posited to be a function of a list of potential determinants (sectoral and cyclical variables, politically based indicators). Wooldridge (Citation2004) demonstrates that an outcome equation can consistently estimate the average treatment effect of the policy if the treatments are continuous variables and are a linear homoscedastic function of Wit−1 and its other regressors. Given that we deal with continuous policy treatments, we can take continuity for granted and test for the other conditions (functional form, homoscedasticity) when we estimate the auxiliary regressions.
The control function approach is particularly convenient in our application for the following reasons. First, although, over time, there have been some explicit rules presiding the allocation of funds between regions (especially as far as the convergence objective is concerned), these rules have never fully governed the allocation of funds, even in the case of SFs. An important consequence of this situation is that there are no regions that do not receive any kind of funding in our sample. This is true for SFs and even more so for NFs. Hence, a counterfactual strategy based on the creation of a control group (for instance, one that does not receive any funding) cannot be enacted in our case.Footnote4 Besides, this approach is readily adapted to the modelling of multiple continuous treatments (the various policy funds, some of which we may want to jointly include in a regression).
The main element of novelty of the present paper is in its sectoral focus. We assess the effectiveness of SFs and NFs on the output of four sectoral aggregates: agriculture, industry, construction and services. Furthermore, sectoral outputs are modelled through a multi-input multi-output transformation function that allows for their joint determination. It could be thought that, to deal with the sectoral impact of regional policies, it is enough to replicate the outcome equation sector by sector. However, regressing sectoral output on SFs or NFs would assume away both the impact of the funds on the rest of the economy and the impact of the rest of the economy on the sector under scrutiny. Equally, only including in a sectoral equation the output of the rest of the economy along with the other regressors would not be a satisfactory way of modelling this nexus: we would implicitly assume that the rest of the economy is not affected by the funds. Following these considerations, we will estimate four multi-output multi-input transformation functions, with each of them modelling, as joint outputs, the value added of a given sector and the value added of the rest of the economy. For further details on the econometrics of multi-output multi-input transformation functions, see Coelli and Perelman (Citation1999) and Kumbhakar (Citation2012, Citation2013).
3.2. Econometric specifications
We start from a standard panel specification:
(1)
(1) where i = 1, … , 20 refers to the regions, t = 1, … , n indicates the years and j = 1, … , m refers to the type of fund being considered; variables ai and at are, respectively, region and year fixed effects. The dependent variable yit is (the natural log of) regional GDP per capita; the lagged dependent variable yit−1 allows for the dynamic structure inherent in the data (as will be expounded at the outset in section 4, in the empirical work, we also experimented with more complex specifications). Besides yit, equation (1) includes gfiit, the regional gross fixed investment; sfit, the EU funds spent in a region; nfit, the national policy funds related to regional development accruing to a given region;Footnote5 and D.popit, the (natural log) variation in the population. All the lowercase variables are taken in natural logarithms. In equation (1), gfiit, sfit and nfit are natural log ratios of GDP. Variables
and
are country and year fixed effects, respectively, while
is, as usual, an independent and identically distributed error term. Vector Wit−1 includes the variables presiding over the regional allocation of the funds, selected through the estimation of a set of appropriate auxiliary regressions.
As stated above, the key empirical relationship of this paper links sectoral output per capita to policy funds through a multi-input multi-output transformation function. Accordingly, the outcome equation becomes the following:
(2)
(2) where xs is the natural log of the product of sector s divided by the total population, and xnons is the natural log of the product of all sectors of the economy, except for sector s. Both xs and xnons are divided by (the natural log of) the total population. We take f as a shorthand notation for any kind of policy fund, while gfi and D.pop have the same meaning as in equation (1). In the Cobb–Douglas transformation function (2), xs and xnons are outputs jointly produced by inputs gfi, D.pop and f. This joint determination is modelled by including the log difference (xnons – xs) in equation (2). Kumbhakar (Citation2013) shows that the apparent endogeneity of this log difference, which contains a left-hand variable, is not a problem if the other regressors are exogenous. The weak exogeneity of all the current (and forwarded) regressors included in the multi-output multi-input transformation functions shall therefore be thoroughly tested in our empirical analysis. A further important remark is that the transformation function relies on the variability of xnons to explain the variability of xs. Provided that xs and xnons are correlated, which is likely, this uses the available information in a very efficient way.
In equation (2), all the inputs affect xs and xnons homothetically. However, we are interested in ascertaining whether policy funds are particularly effective in some sectors. The multi-output multi-input transformation function framework can be extended to find out whether policy funds (as well as, eventually, gfi and D.pop) have different impacts across different sectors by adding to equation (2) an interaction term between any of the funds and the log difference (xnons it – xs it):Footnote6
(3)
(3) Using equation (3), we can see whether policy funds have a stronger impact on either xs or xnons. To observe this, we derive below the long-run solutions of (3) for each variable. For the sake of simplicity, we work on a shortened version of (3), including only xs, xnons and f. The derivation is detailed in the supplemental data online:
(4)
(4) In this case, funds favour xnons in the sense that a higher a52 increases the long-run impact of funds on this variable and dampens the long-run effect of funds on xs. Yet, the result would be the opposite if a52 had a negative sign in equation (3). Hence, our analysis can be used to identify sectors where policy intervention is particularly effective (or ineffective).
3.3. Data issues and sources
To carry out this analysis, we built a large dataset for the 20 Italian administrative regions concerning the period 1994–2016, covering, at least partially, four waves of the EU Cohesion Policy. It contains the value added and employment of four economic sectors (agriculture, industry, construction, services), other institutional and economic indicators (gross fixed capital formation, population, unemployment, etc.) and data on all the policy funds, extracted from the Spesa Pubblica Regionalizzata database of the General Accounting Office (Ragioneria Generale dello Stato) at the Italian Ministry of Economy and Finance (Ministero dell’Economia, Citationvarious years). Using the Spesa Pubblica Regionalizzata database allows us to rely on indicators based on the same methodology for both SFs and NFs.
SFs are measured in terms of disbursements to the regions by the Rotation Fund (Fondo di Rotazione), the Italian governmental institution responsible for raising funds from the EU. We consider the following SFs: the ERDF (labelled erdf when reporting the results, because taken in natural logarithms); the ESF (esf); the sum of the EAFRD, EAGF and EFF (othf);Footnote7 and the national co-financing (cofin), to which reference was made in section 2.Footnote8
Among the NFs going to a given region, we focus on current account subsidies for firms (cfsub) and capital account expenditures, which are divided into subsidies for firms (kfsub) and investments (kinv). We also measure national cohesion policies (ncf) through the sum of funds such as the Fondo innovazione tecnologica, Fondo contributo imprese, Fondo solidarietà nazionale and Fondo per lo Sviluppo e la Coesione (FSC, formerly the Fondo per le Aree Sottoutilizzate – FAS).
Our sample includes observations from 1994 to 2016. More information about all the data and their sources is provided in the supplemental data online, alongside a list of important variables and abbreviations.
4. THE EVIDENCE
One important observation should be immediately made regarding the dynamic specification of the regressions. Following Coppola et al. (Citation2020) and Destefanis et al. (Citation2022), the dynamic specification of our model reflects the institutional mechanism presiding to the allocation of SFs. These funds are paid out from the EU to the regions with a lag of approximately one year relative to the spending decisions, meaning that the SF expenditures written down for year t have already been spent by regions in year t – 1. Accordingly, the SF variables included in our estimates are forwarded by one year, although, for simplicity, we adopt the convention of dating SFs one year back when presenting the results.Footnote9
and provide the main evidence obtained applying the empirical framework presented in the previous section. relates to equation (1), while various specifications of equations (2) and (3) are included in . We only present the estimated coefficients for the variables of interest. All the specifications include, however, the log variation of the population and the Wit−1 vector.Footnote10 The auxiliary regressions that select the relevant variables within the Wit−1 vector are presented and commented on in Table A1 in the supplemental data online. It turns out that the Wit−1 vector includes lagged values of GDP per capita, gross fixed investment, and various SFs and NFs, as well as of other structural variables, such as the (female) rate of unemployment, sectoral shares of output and employment and unit labour costs of services. Political variables have very marginal effects, arguably because, unlike in Kemmerling and Bodenstein (Citation2006) or Bouvet and Dall’erba (Citation2010), we account for unobserved heterogeneity through fixed-effect panel estimates.
Table 1. Equation (1), total economy. The impact of funds on gross domestic product (GDP) per capita, dependent variable: y. Specifications with and without nationally financed policy funds; N = 420.
Table 2. Equations (2) and (3). Various sectors, impact of funds on sectoral value added per capita. Dependent variable: xs. Specifications only include ERDF; N = 420.
The diagnostic tests for the estimates in and are generally very good. There is only some evidence of endogeneity for the capital account subsidies for firms (). Accordingly, we proceed to discuss the main economic features of our evidence. SFs have a significant impact on GDP per capita. However, this impact is mainly observed with regard to the ERDF. , relating to the determination of regional GDP per capita, conveys this message well. A, say, doubling of the ERDF/GDP share increases the steady-state level of GDP per capita by a sizeable proportion (about one-seventh) of the same proportional increase of gross fixed investment/GDP. However, this impact vanishes when we take the other components of SFs into account (the ESF, agriculture- and fishery-related funds, and national co-financing; the latter, however, has a respectable size and approaches significance at the 10% level; the restriction that erdf and cofin have the same – significant – coefficient is easily accepted by the data). Equally, no nationally financed policy fund (nationally funded cohesion policies, capital account investment expenditures or either current or capital account subsidies for firms) has a significant impact on aggregate GDP per capita.
These findings suggest restricting the presentation in to only sectoral estimates that are centred around the role of the ERDF. We always report estimates for equations (2) and (3), first only including the ERDF (), then including the ERDF and other SFs () and, finally, including the ERDF and nationally funded regional development policies (). There are, of course, many other sets of estimates that could be presented, but we believe that the reported results convey, as simply as possible, the main findings of our study.
Table 3. Equations (2) and (3). Various sectors, impact of funds on sectoral value added per capita. Dependent variable: xs. Specifications include ERDF and other SFs; N = 420.
Table 4. Equations (2) and (3). Various sectors, impact of funds on sectoral value added per capita. Dependent variable: xs, Specifications including ERDF and nationally financed policy funds, N = 420.
We immediately note, from comparing the r2 and Rss in and , that the transformation function uses the available information more efficiently than aggregate estimates. However, the most important remark is that the hierarchies established in are, by and large, confirmed. In the estimates of equation (2), the ERDF maintains its widespread significance, while the other SFs are not significant, and the same is true for the NFs. However, the estimation of equation (3) provides interesting insights resulting from the interaction terms involving the policy funds and sectoral outputs.
In the estimates for equation (3) regarding agriculture and construction, the ERDF is not significant because of the high correlation between the erdf standalone and the interaction terms. Indeed, this fund affects, to the same extent, the sector under scrutiny and the rest of the economy. On the other hand, the impact of the ERDF is stronger for industry and weaker for services, as compared with the rest of the economy (this can be seen by looking at the, respectively, negative and positive signs of the relevant interaction terms). This result is very robust and occurs throughout all the specifications of interest.
In equation (3), the esf and cofin coefficients always become significant. From the signs of the interaction effects for these funds, one can infer that they have a stronger impact in the agricultural sector. Hence, in general, the impact of SFs is weaker in services and stronger in agriculture and industry. A remarkable feature of the estimates from is that funds related to agriculture and fishery are never significant, with or without interactions. We will return to this result when commenting on our evidence.
Some significant interaction terms also emerge for NFs from the estimation of equation (3). The impact of nationally funded cohesion policies (ncf) is stronger in industry and (much less significantly) in construction and is weaker in services. These interaction effects are particularly strong and follow, to some extent, the same pattern in terms of the impact of the ERDF. The impact of capital account subsidies (kfsub) is significantly weaker in agriculture and shows some signs of being weaker in industry and stronger in services (the equations for agriculture and services are, however, the least reliable of our estimates on account of the significant values of the weak exogeneity tests for capital account subsidies). Also, the impact of nationally funded public investments (kinv) is marginally stronger in services.
As far as SFs are concerned, our results echo the evidence of Percoco (Citation2017), who finds that a high share of services in the economy is associated with a weaker impact of SFs on economic activity. Percoco does not directly analyse the effects of SFs on sectoral variables and surmises that his evidence could reflect the impact of the stage of development of an economy upon the effectiveness of SFs. To investigate this issue, we carried out Chow’s first test of parameter stability for all the specifications of . We partitioned our sample across areas, separating the Mezzogiorno regions (including Abruzzo, Molise, Campania, Puglia, Basilicata, Calabria, Sicilia and Sardegna) from the rest of Italy. We could never reject at the 10% significance level the null of parameter stability and thus conclude that our results reflect structural differences in the sectoral impact of SFs, rather than being driven by factors linked to the development of the regional economy. Having established this, one can ask whether the size and sign of the interaction terms between policies and sectoral outputs depend on the sectoral allocation of the funds. Some information about the latter can be obtained for the 2007–13 programming cycle and is presented in .Footnote11
Table 5. Sectoral percentage shares; number of projects, funding, value added, employment.
The percentages from make clear that the sectoral allocation of the policy variables can provide, at most, only a partial explanation of the differences in the interaction terms, because a sizeable share of the funds for which data are available is simply not attributed to any given sector. Having said this, it is true that the proportions of the ERDF’s projects and funding attributed to industry are closer to the sectoral shares of value added and employment than those of the other sectors. However, a stronger impact of funds in favour of industry also emerged in our estimates for nationally funded cohesion policies where, at least based on the information available in , the sectoral policy endowment of industry is much lower that the value added or employment share of this sector. It is probably more sensible to conclude that the priority areas of the ERDF (that have consistently privileged innovation and research and support for small and medium-sized enterprises) have made this fund particularly effective in industry. Unfortunately, the available information cannot provide any insight about the differentiated impact of capital account expenditures, especially capital account subsidies.
The priority areas that characterize the ERDF may also help explain why this fund achieves the strongest impact on value added per capita across the board. Indeed, according to Fratesi and Perucca (Citation2019), firm assistance is the most effective axis of intervention in regions lacking territorial capital (Fratesi & Perucca, Citation2016, show that a very large share of the Italian territory is characterized by a relatively low endowment of territorial capital). On the other hand, the ESF and capital account subsidies (kfsub) only tilt the sectoral distribution of the economy, respectively, in favour of and against agriculture. A similar effect is achieved by the nationally funded cohesion policies (ncf) in favour of industry and against services.
The lack of significance of NFs on GDP per capita may be rationalized on several grounds. According to a first argument, NFs are more intensive in non-core items, such as education and health.Footnote12 As already noted by Coppola et al. (Citation2020, p. 92) problems with data availability, and with difficulties in distinguishing core and non-core expenditures among the SFs, make it difficult to empirically assess this argument. At any rate, based on the information presented in the supplemental data online, the composition of expenditure may explain why the ERDF is more effective than the ESF, but not why current account subsidies to firms, nationally funded cohesion policies or public investments are so ineffective. As far as public investments are concerned, Coppola et al. (Citation2020, p. 92) observe that a more promising explanation may rely on the analysis developed by Golden and Picci (Citation2005) about the mismanagement of public works in Italy. To this it should be added that the regionalization of infrastructure spending brought about by various institutional reforms is likely to have entailed a less efficient use of resources, especially for transport infrastructure (on this, see Di Giacinto et al., Citation2012). Both these issues must, however, be left for future research as they require a proper treatment of the relationships between infrastructure expenditures and outcomes, as well as of network and spillover effects across regions.
The issue of policy governance is another often-evoked argument. Abundant literature (see Coppola et al., Citation2020, for a survey) blames the problematic outcome of regional policies in Italy mainly on the lack of effective governance, especially at the local level, and on the fragmentation of interventions. The different governance across SFs has often been evoked as a cause of their different effectiveness: the fragmentation of interventions, especially within the ESF, is often blamed as a source of ineffectiveness (Marinuzzi & Tortorella, Citation2016), and we already noted that the ESF may be biased towards non-core expenditures. Admittedly, all this means that the peculiarly strong effect of the ESF in agriculture is a bit of a puzzle. Maietta et al. (Citation2019) point out that nowadays an important channel for productivity improvement in agriculture is increased specialization in more productive farming activities. Hence, local investments in human capital, even outside agriculture, could help this kind of favourable transition. We readily acknowledge that this is only a tentative explanation. It is, on the other hand, possible to find a more solid justification for the lack of effectiveness of othf. Crescenzi et al. (Citation2015) find much room for improvement in rural development policies and demand greater coordination between them and EU Cohesion Policy. Maietta et al. (Citation2019), surveying the relevant literature and providing an analysis of the Common Agricultural Policy (CAP) reform, highlight that the impact of CAP instruments (such as the agriculture-related funds that we analyse) on agricultural productivity is highly heterogeneous across instruments and environments. Subsequently, it is not surprising that an insignificant effect of agriculture- and fishery-related funds is observed. This finding calls for future research that considers in greater detail the impact of different types of funds on productivity. In this sense, separating agriculture and fishery funds could also be appropriate.
It is also interesting to compare the gist of our evidence with that of Psycharis et al. (Citation2020). Indeed, while they find that nationally funded policies boosted growth in the Greek regions, at least before the Great Recession of 2008, and that SFs were basically ineffective, our results basically tell the opposite story. It is impossible to carry out a thorough comparison of the two analyses, given some differences in the basic framework (the estimated models as well as the econometric analyses). However, we must say that the lower effectiveness of SFs in Greece is at least partly consistent with our findings, given the much smaller share of industry in the Greek economy (with our coefficients, the coefficient of the ERDF would be about a half of what we observe here). The reasons for the higher effectiveness of nationally funded public investments in Greece are less clear a priori. However, from Eurostat’s annual government finance statistics, it turns out that the share of public investment managed by the central government in Greece was (between 1995 and 2021) 78%, versus 36% in Italy. According to the considerations made above, this could lead to a more effective governance of these funds in Greece.
Finally, the following remarks can be made regarding the contribution of our evidence to the wider debate on the effectiveness of cohesion policies in a country where the divide between Mezzogiorno and the rest of the country has widened since the inception of EU Cohesion Policy. Fratesi (Citation2020) points out that, by and large, we should expect to find from the literature on SFs that these funds are more effective in less developed regions. This is exemplified by the findings in Pellegrini et al. (Citation2013) and Aiello and Pupo (Citation2012). The latter, in a set-up like that of the present paper, both in terms of its panel structure and the choice of GDP per capita as the key outcome variable, find a stronger impact of SFs on GDP per capita in the Mezzogiorno regions. These results are hard to reconcile with the persistent territorial divides in Italy. In this paper, when we applied a Chow test to our estimates, we did not find any structural differences between Mezzogiorno and the rest of the country. It thus appears that when proper allowance is made for the composition of the SF expenditure, its dynamic specification and its sectoral impact, the factors that would lead, in the Mezzogiorno, to a lower or higher effectiveness of the SFs counterbalance each other. Interestingly, the discrepancy between our results and those obtained by Aiello and Pupo (Citation2012) for GDP per capita can be at least partially explained by the fact that during their estimation period the GDP share of industry, not modelled in their framework, decreased less quickly in the southern than in the northern regions (1.35 versus 3.34 percentage points). In other circumstances, the verdict of a lower effectiveness of regional policies in the Mezzogiorno may have stemmed from the opposite kind of situation. In light of these considerations, we believe that in this paper we have shown that the sectoral impact of regional policies is an element that must be considered when evaluating the design and outcome of these measures.
5. CONCLUSIONS
In this paper we investigate the impact of SFs and NFs on the sectoral components of GDP of the Italian regions across four waves of the EU Cohesion Policy (relating to the 1994–2016 period). We consider, through a control function approach, the selection bias potentially resulting from the fund allocation mechanism and assess separately the funds’ effects on four sectors (agriculture, manufacturing, construction, services) of the regional economies through a multi-input multi-output transformation function.
Our evidence implies that SFs, in particular the ERDF, has a significant impact on sectoral products as well as on aggregate GDP per capita. SFs are particularly effective in industry and (to a lesser extent) agriculture, while being less effective for services. NFs are never significant in the aggregate. Yet, nationally funded cohesion policies have, respectively, a stronger and weaker impact in industry and services. There is also some evidence for capital account expenditures being more effective in the service sector (and less effective elsewhere, especially in agriculture).
Given that in this paper we stress the need to identify effective practices and sectors of intervention, its analysis can help to provide some useful indications for the management of EU funding during the new programming period (2021–27) of the SFs, also in light of the post-COVID-19 stance of the EU. In the last decade, EU regional policy has embraced the place-based approach (Barca, Citation2009; Barca et al., Citation2012), according to which policies are tailored to exploit the potentialities of any given region. During the 2014–20 programming period, this approach was mainly represented by the Smart Specialisation Strategy (Capello & Kroll, Citation2016). To have access to EU funds for Axis 1 (‘Strengthening research, technological development and innovation’), each region must ascertain and document its competitive strengths and weaknesses. This orientation also characterizes EU Cohesion Policy in the new 2021–27 programming cycle (Italy’s Partnership Agreement, January 2022 draft). In support of this strategy, Crescenzi et al. (Citation2017) and Di Cataldo and Monastiriotis (Citation2020) suggest that concentrating funds on regional needs makes policies more successful. The evidence from this paper provides further support for this strategy, at least for the Italian regions. Gearing EU funds to the industrial sector and responding to regional needs (related to research into technological development and innovation, transport infrastructure, human resources and business support) are largely overlapping aims, at least in the Mezzogiorno. It is likely that satisfying the needs that currently exist in the Mezzogiorno would make the impact of the ERDF on industry even more effective.
Place-based strategies are also very demanding in terms of governance capability. We have already noted that the relatively poor performance of the ESF and of nationally funded cohesion policies, and the even poorer performance of the other funds studied, can be explained by a lack of such capability. In this respect, we must acknowledge that the Smart Specialisation agenda has encouraged regions to combine ESF and ERDF expenditures with the aim of obtaining synergic effects between these funds. The 2021–27 programming strategy also stresses the need to enhance the complementarities and integrations among the EU funds to strengthen their effectiveness (Partnership Agreement of Italy, January 2022 draft). More generally, two policy instruments, a national technical assistance programme called ‘Capacità per la coesione’ and administrative regeneration plans (PRigA), address the problem of the lack of governance capability.
Future research in this field should contemplate applying our empirical framework to regional data for a group of EU economies. This would dramatically increase the sample size and allow a more granular treatment of the structural differences among different territories, including a more articulate comparison of the Mezzogiorno with other less developed regions. On the other hand, the analysis of EU data would also make it difficult to obtain information on nationally funded regional development policies.
Supplemental Material
Download PDF (399.7 KB)ACKNOWLEDGEMENTS
We are thankful to the editors and referees, to Cristina Vaquero Pineiro, and to the participants at the SIE 2018, ERSA 2019 and AISRE 2019 meetings for helpful comments on previous drafts of this paper. The usual disclaimer applies.
DISCLOSURE STATEMENT
No potential conflict of interest was reported by the authors.
Additional information
Funding
Notes
1. Strictly speaking, the EAFRD, EAGF and EFF are no longer ranked among the European Structural and Investment Funds after the 2007 reform. However, we chose to include them in our empirical analysis, taking a wider view of EU Cohesion Policy.
2. To the best of our knowledge, only Coppola et al. (Citation2020) and Psycharis et al. (Citation2020) have conducted a cogent comparison of the relative effectiveness of EU and national funds. Neither study analyses the effects of these funds on sectoral outputs.
3. In a recent paper, Scotti et al. (Citation2022) define as sectors what most other authors define as pillars (or axes) of EU Cohesion Policy: human resources, research and development (R&D), transport infrastructure, etc. They subsequently compare the effectiveness of SFs across these pillars.
4. Notable examples of this kind of analysis, mostly based on a cross-sectional regression discontinuity design, include Becker et al. (Citation2010, Citation2012, Citation2013), Pellegrini et al. (Citation2013), Giua (Citation2017) and Crescenzi and Giua (Citation2018). In these papers, policy identification is typically achieved by focusing on a finer level of territorial disaggregation than in the present paper and neglecting the possibility of multiple treatments. Hence, we believe that our counterfactual analysis can usefully complement this existing evidence.
5. In empirical analysis, this specification can easily be generalized to allow for more than one category of either EU or national policy funds.
6. In what follows we abstract from the sectorally differentiated effects of gross fixed investment and the variation in the population, which never turned out to be significant when tested during the empirical analysis.
7. Before 2007 this is actually the sum of the EAGGF and FIFG.
8. Unfortunately, our dataset only includes an aggregate measure of co-financing throughout the sample period. The analysis of co-financing disaggregated across funds must thus be deferred to future research.
9. As already noted by Destefanis et al. (Citation2022), the European Commission (Citation2020) provides measures of SF expenditures ‘taking place on the ground’ that closely follow the evolution over time of our forwarded SFs. We stick, however, to our SF measures since the European Commission’s indicators do not include national co-financing and are available for fewer years.
10. Tables presenting the complete estimates are available from the authors upon request. The log variation of the population always gives estimates with the negative sign expected a priori. Among the variables included in the Wit–1 vector, only the unit labour cost in services consistently achieves significance (with a positive sign). Sectoral shares of output and employment are occasionally significant with varying signs. None of these features matters for the validity of the control function approach or for the economic and policy implications of our estimates.
11. EU regulations imply that the EAGF, EAFRD and EFF (and the EAGGF and FIFG before 2007) in their entirety are spent within agriculture.
12. Ever since the work of Aschauer (Citation1989), it has been held that the main impact of public capital on GDP comes from so-called productive or core infrastructure (roads, railways, ports, airports, etc.).
REFERENCES
- Aiello, F., & Pupo, V. (2012). Structural funds and the economic divide in Italy. Journal of Policy Modeling, 34(3), 403–418. doi:10.1016/j.jpolmod.2011.10.006
- Allen, K., & Stevenson, A. (1974). An introduction to the Italian economy. Martin Robertson.
- Aschauer, D. A. (1989). Is public expenditure productive? Journal of Monetary Economics, 23(2), 177–200. doi:10.1016/0304-3932(89)90047-0
- Barca, F. (2009). An agenda for a reformed Cohesion Policy. A place-based approach to meeting European Union challenges and expectations. Independent Report for Danuta Hübner, Commissioner for Regional Policy.
- Barca, F., McCann, P., & Rodríguez-Pose, A. (2012). The case for regional development intervention: Place-based versus place-neutral approaches. Journal of Regional Science, 52(1), 134–152. doi:10.1111/j.1467-9787.2011.00756.x
- Becker, S. O., Egger, P. H., & von Ehrlich, M. (2010). Going NUTS: The effect of EU structural funds on regional performance. Journal of Public Economics, 94(9–10), 578–590. doi:10.1016/j.jpubeco.2010.06.006
- Becker, S. O., Egger, P. H., & von Ehrlich, M. (2012). Too much of a good thing? On the growth effects of the EU’s regional policy. European Economic Review, 56(4), 648–668. doi:10.1016/j.euroecorev.2012.03.001
- Becker, S. O., Egger, P. H., & von Ehrlich, M. (2013). Absorptive capacity and the growth and investment effects of regional transfers: A regression discontinuity design with heterogeneous treatment effects. American Economic Journal: Economic Policy, 5(4), 29–77. doi:10.1257/pol.5.4.29
- Boldrin, M., & Canova, F. (2001). Europe’s regions, income disparities and regional policies. Economic Policy, 32, 207–253. doi:10.1111/1468-0327.00074
- Bouvet, F., & Dall’erba, S. (2010). European regional structural funds: How large is the influence of politics on the allocation process?. JCMS: Journal of Common Market Studies, 48(3), 501–528. doi:10.1111/j.1468-5965.2010.02062.x
- Capello, R., & Kroll, H. (2016). From theory to practice in smart specialization strategy: Emerging limits and possible future trajectories. European Planning Studies, 24(8), 1393–1406. doi:10.1080/09654313.2016.1156058
- Coelli, T., & Perelman, S. (1999). A comparison of parametric and non-parametric distance functions: With application to European railways. European Journal of Operational Research, 117(2), 326–339. doi:10.1016/S0377-2217(98)00271-9
- Coppola, G., & Destefanis, S. (2007). Fondi strutturali, produttività e occupazione. Uno studio sulle regioni italiane. Rivista di economia e statistica del territorio, (2), 85–113.
- Coppola, G., & Destefanis, S. (2015). Structural funds and regional convergence: Some sectoral estimates in Italy. In C. Mussida & F. Pastore (Eds.), Geographical labor market imbalances (pp 307–333). Springer.
- Coppola, G., Destefanis, S., Marinuzzi, G., & Tortorella, W. (2020). European Union and nationally based cohesion policies in the Italian regions. Regional Studies, 54(1), 83–94. doi:10.1080/00343404.2018.1447099
- Crescenzi, R. (2009). Undermining the principle of concentration? European union regional policy and the socio-economic disadvantage of European regions. Regional Studies, 43(1), 111–133. doi:10.1080/00343400801932276
- Crescenzi, R., De Filippis, F., & Pierangeli, F. (2015). In tandem for cohesion? Synergies and conflicts between regional and agricultural policies of the European Union. Regional Studies, 49(4), 681–704. doi:10.1080/00343404.2014.946401
- Crescenzi, R., Fratesi, U., & Monastiriotis, V. (2017). The EU cohesion policy and the factors conditioning success and failure: Evidence from 15 regions. Regions Magazine, 305(1), 4–7. doi:10.1080/13673882.2017.11868994
- Crescenzi, R., & Giua, M. (2018). One or many cohesion policies of the European Union? On the diverging impacts of cohesion policy across member states (SERC DP No. 230).
- Del Bo, C. F., & Sirtori, E. (2016). Additionality and regional public finance – evidence from Italy. Environment and Planning C: Government and Policy, 34(5), 855–878. doi:10.1177/0263774X15614682
- Destefanis, S., Di Serio, M., & Fragetta, M. (2022). Regional multipliers across the Italian regions. Journal of Regional Science, 62(4), 1179–1205. doi:10.1111/jors.12592
- Di Cataldo, M., & Monastiriotis, V. (2020). Regional needs, regional targeting and regional growth: An assessment of EU cohesion policy in UK regions. Regional Studies, 54(1), 35–47. doi:10.1080/00343404.2018.1498073
- Di Giacinto, V., Micucci, G., & Montanaro, P. (2012). Network effects of public transport infrastructure: Evidence on Italian regions. Papers in Regional Science, 91(3), 515–541. doi:10.1111/j.1435-5957.2012.00446.x
- Dotti, N. F. (2013). The unbearable instability of structural funds’ distribution. European Planning Studies, 21(4), 596–614. doi:10.1080/09654313.2012.722956
- European Commission. (2020). Historic EU payments – Regionalised and modelled. https://cohesiondata.ec.europa.eu/Other/Historic-EU-payments-regionalised-and-modelled/tc55-7ysv
- European Union. (2021). The EU’s 2021–2027 long-term budget and NextGeneration EU. Publications Office of the European Union.
- Fratesi, U. (2016). Impact assessment of European cohesion policy: Theoretical and empirical issues. In S. Piattoni, & L. Polverari (Eds.), Handbook on cohesion policy in the EU (pp. 443–460). Edward Elgar.
- Fratesi, U. (2020). Contextualizing regional policy impact: A contribution to more effective policy-making. Scienze Regionali, 19(3), 453–476. doi:10.14650/98287
- Fratesi, U., & Perucca, G. (2016). Territorial capital and EU cohesion policy. In J. Bachtler, P. Berkowitz, S. Hardy, & T. Muravska (Eds.), EU cohesion policy. Reassessing performance and direction (pp. 255–270). Routledge.
- Fratesi, U., & Perucca, G. (2019). EU regional development policy and territorial capital: A systemic approach. Papers in Regional Science, 98(1), 265–281. doi:10.1111/pirs.12360
- Giua, M. (2017). Spatial discontinuity for the impact assessment of the EU regional policy: The case of Italian Objective 1 regions. Journal of Regional Science, 57(1), 109–131. doi:10.1111/jors.12300
- Golden, M., & Picci, L. (2005). Proposal for a new measure of corruption, illustrated with Italian data. Economics and Policy, 17(1), 37–75. doi:10.1111/j.1468-0343.2005.00146.x
- Heckman, J., & Hotz, V. J. (1989). Alternative methods for evaluating the impact of training programs. Journal of the American Statistical Association, 84(804), 862–887. doi:10.1080/01621459.1989.10478848
- Iuzzolino, G. (2009). I divari territoriali di sviluppo in Italia nel confronto internazionale. In L. Cannari (Ed.), Mezzogiorno e politiche regionali (pp. 427–478). Banca d’Italia.
- Kemmerling, A., & Bodenstein, T. (2006). Partisan politics in regional redistribution. Do parties affect the distribution EU structural funds across regions? European Union Politics, 7(3), 373–392. doi:10.1177/1465116506066264
- Kumbhakar, S. C. (2012). Specification and estimation of primal production models. European Journal of Operational Research, 217(3), 509–518. doi:10.1016/j.ejor.2011.09.043
- Kumbhakar, S. C. (2013). Specification and estimation of multiple output technologies: A primal approach. European Journal of Operational Research, 231(2), 465–473. doi:10.1016/j.ejor.2013.05.019
- Maietta, O. W., De Devitiis, B., Destefanis, S., & Suppa, D. (2019). Human capital and rural development policy: Evidence from European FADN regions. Bio-based and Applied Economics, 8(3). doi:10.13128/bae-8888
- Marinuzzi, G., & Tortorella, W. (2016). Chi è l’asso pigliatutto del Fesr 2007–2013? Quotidiano Enti Locali & Pa. Sole24Ore, 20 April.
- Ministero dell’Economia. (various years). La spesa statale regionalizzata. Rome.
- Pellegrini, G., Busillo, F., Muccigrosso, T., Tarola, O., & Terribile, F. (2013). Measuring the effects of European regional policy on economic growth: A regression discontinuity approach. Papers in Regional Science, 92(1), 217–233. doi:10.1111/j.1435-5957.2012.00459.x
- Percoco, M. (2017). Impact of European cohesion policy on regional growth: Does local economic structure matter? Regional Studies, 51(6), 833–843. doi:10.1080/00343404.2016.1213382
- Psycharis, Y., Tselios, V., & Pantazis, P. (2020). The contribution of cohesion funds and nationally funded public investment to regional growth: Evidence from Greece. Regional Studies, 54(1), 95–105. doi:10.1080/00343404.2018.1525696
- Puigcerver-Peñalver, M. C. (2007). The impact of structural funds policy on European regions’ growth: A theoretical and empirical approach. European Journal of Comparative Economics, 4(2), 179–208.
- Scotti, F., Flori, A., & Pammolli, F. (2022). The economic impact of structural and cohesion funds across sectors: Immediate, medium-to-long term effects and spillovers. Economic Modelling, 111, 105833. doi:10.1016/j.econmod.2022.105833
- Wooldridge, J. M. (2004). Estimating average partial effects under conditional moment independence assumptions (Working Papers No. CWP03/04). Centre for Microdata Methods and Practice (CeMMAP), Institute for Fiscal Studies (IFS).