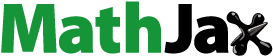
ABSTRACT
We study the impact of agglomeration effects on firms’ total factor productivity (TFP) for industry groups defined by technology intensity. This allows for non-uniform effects on firms depending on their technological level. We find that urban economies have the largest impact on firm productivity in high-technology industries, while they have no effect in low-technology industries. For firms in the latter industries, the diversity of the local economic structure is relevant. Localisation effects have a consistently positive and significant impact on TFP, with the effect increasing with the technology intensity of the industries.
1. INTRODUCTION
In line with neoclassical theory (Barro & Sala-i-Martin, Citation1995; Quah, Citation1996), the convergence process within the European Union still dominates due to the catching-up of Eastern European countries. At the same time, however, the regional disparities within the countries seem to increase considerably, in particular the growing gap between rural areas and big cities and their wider surroundings (Alcidi et al., Citation2018; Belitz et al., Citation2019; Goecke & Hüther, Citation2016). At first glance, our knowledge about the factors that lead to regional economic differences and what to do about it seems fairly strong. Already in the twentieth century, Marshall (Citation1920) and Weber (Citation1929) identified agglomeration effects as a driving force of regionally diverging economic developments. Although the empirical evidence at the micro-level is not unambiguous, it generally confirms the presumptions that firms and people benefit from agglomeration economies, be it in terms of more employment (Caragliu et al., Citation2016; Glaeser et al., Citation1992; Henderson, Citation1986), higher wages (Combes et al., Citation2008; Faberman & Freedman, Citation2016; Matano & Naticchioni, Citation2012), more innovativeness (Hervas-Oliver et al., Citation2018) or higher firm productivity (Ahrend et al., Citation2017; Cainelli et al., Citation2015; Cainelli & Ganau, Citation2018; Martin et al., Citation2011; Melo et al., Citation2009; Melo et al., Citation2017).
Based on the insights from regional economic research and other fields of economic research, European politics applies a broad policy mix and various instruments to counter or soften diverging regional economic developments (Capello & Perucca, Citation2018; European Committee of the Regions, Citation2021; Iammarino et al., Citation2019). This includes the Smart Specialisation Strategy as part of the reformed Cohesion policy (Foray, Citation2014; McCann & Ortega-Argilés, Citation2015). The strategy supports ‘knowledge-based investments and/or clusters’ and ‘embraces a broad view of innovation, supporting technological as well as practice-based and social innovation’, this way taking into account the importance of technology and knowledge for the economic development of regions and of the firms within the regions (European Commission, Citation2021).
However, the literature on innovation, R&D and knowledge-based capital (KBC) shows that firms differ substantially with respect to the effects of new knowledge, new technologies and innovation. These differences are systematic in the way that firms gain more in terms of higher productivity or stronger growth the more knowledge-intensive or R&D-intensive they are. The OECD summarises the existing evidence by stating that ‘the literature is in agreement that the positive effects of R&D and innovation are larger for high-tech firms and in high-tech or knowledge-intensive sectors’ (Tsvetkova et al., Citation2020, p. 19), while there are only small or even no benefits for low-tech industries (Kancs & Siliverstovs, Citation2016). Subsequent evidence further shows that the same holds for investments in intangible capital, which, besides R&D, includes spending on human capital, the investment in the organisation of firms, or digitisation efforts, etc. (DeRidder, Citation2019; Le Mouel & Schiersch, Citation2020). Put differently, the literature shows that gains from improvements in knowledge and technology increase with the knowledge-intensity of industries. Furthermore, we know that the various agglomeration effects are linked to benefits that come from improvements in knowledge and technology (Rocchetta et al., Citation2022).
Given this fact, it can be suspected that benefits from agglomerations differ in accordance with the knowledge-intensity of firms and industries. This is particularly important for the understanding of the relationship between agglomeration effects and total factor productivity (TFP), as the latter is driven by knowledge, innovation, technological improvements and competition. However, the technological intensity is mostly ignored in studies on the relationship between agglomeration effects and TFP to this day. This study addresses this gap by estimating the causal relationship between various indicators of agglomeration economies and firm-level TFP, while distinguishing between industries of different technological intensity.
The analysis is conducted using firm-level data from the German manufacturing industry that cover the 2003 to 2014 period. When ignoring technological intensities, we find all considered agglomeration effects to significantly affect TFP. However, competition effects and the diversity of the sector structure in a region is not statistically significant when high-tech industries are considered. Instead, growing concentration of other high-tech firms as well as benefits that come with urbanisation are relevant for productivity gains in high-tech industries. Furthermore, we find that the marginal effect of localisation and urbanisation decreases in magnitude, the lower the technological intensity of the industries. For the case of low-tech industries, the urbanisation variable loses statistical significance. Finally, and in contrast to high-tech industries, we also find that stronger competition seems to unfold negative effects in low-tech and medium low-tech industries. These differences show that agglomeration effects unfold very different effects depending on the technological intensity of firms.
The remainder of this paper is organised as follows. Section 2 discusses the literature and develops the main research question. Section 3 describes the empirical approach and Section 4 describes the data. The results are presented in Section 5, while Section 6 concludes with regard to regional policies such as the Smart Specialisation Strategy.
2. STATE OF THE LITERATURE ON AGGLOMERATION ECONOMIES AND FIRM TFP
The spatial concentration of firms is considered to positively affect productivity for a variety of reasons and mechanisms (Duranton & Puga, Citation2004; Rosenthal & Strange, Citation2004). Inter alia, firms benefit from sharing. For example, the companies benefit from using a better infrastructure. Moreover, the matching accuracy between the skills needed by companies and the skills of the job-seeking workforce is better, the larger the local labour market. Another positive externality of agglomeration areas is associated with learning. This refers to the creation, diffusion and accumulation of new knowledge within and between industries. For all these learning and innovation processes, agglomerations with their cultural diversity, diverse research institutions or the presence of international players offer advantages. To operationalise these influences in an empirical approach, several indicators are needed.
Following Marshall (Citation1920), Arrow (Citation1962) and Romer (Citation1986), the positive externalities associated with the specialisation of a region in a specific industry are known as MAR externalities or localisation economies. Consequently, the localisation economies variable measures the importance of a region for an industry within a country. Those benefits derived from density and urbanisation, such as the presence of local universities, research centres, cultural spaces and social climate, etc., are considered urbanisation economies and population density is regularly used as an indicator to capture this channel (Isard, Citation1956). Jacobs (Citation1969) argues that technological spillovers have a particularly large effect if innovation and new knowledge are created in one industry, but then adapted and used within a different industry. This refers to the learning effect outlined above. In order to capture this channel, the diversity of the sector structure in a region is calculated. The literature developed this approach further by differentiating between related and unrelated variety (Frenken et al., Citation2007). Finally, Porter (Citation1990) argues that innovation and knowledge transfer increases as a result of intense competition within regions, the competition effect. We aim to capture all of these effects in our analysis.
There is a limited, but growing, literature on the relationship between TFP at firm-level and agglomeration economies that essentially started with the seminal paper by Martin et al. (Citation2011).Footnote1 Using the data of French manufacturing firms that cover the 1996 to 2004 period, they estimate the effect of localisation economies, industry diversity, intensity of competition and urbanisation on the TFP of firms. Using ordinary least squares (OLS) and the generalised method of moments (GMM), urbanisation and localisation are found to positively affect TFP in most regressions. In contrast, the coefficients of the competition variable and of industry diversity are ambiguous. In some specifications they are negative and significant, but positive and significant in others, while even insignificant in some robustness checks.
Martin et al. (Citation2011) is followed by a series of studies that, although similar in nature to some extent, provide mixed results with respect to the individual agglomeration effects. Using French data, Combes et al. (Citation2012) find that firms in denser populated areas have higher TFP, which points to positive effects from urbanisation, while selection and learning due to stronger competition is statistically irrelevant. This is partly in line with the findings of Martin et al. (Citation2011) on urban economies and competition. Using German plant level data, Ehrl (Citation2013) finds no effect for urbanisation, sectoral specialisation or sectoral diversity. Only labour market pooling, which is captured by occupational information at the county level, is significant. DiGiacinto et al. (Citation2014), using data from Italian manufacturing firms and defining three regions that capture urban centres, industry districts and all others, find that firms in the first two regions have significantly higher TFP. This is interpreted as support for the beneficial effects of urban economies and location economies. Cainelli et al. (Citation2015) also analyses data from Italian manufacturing firms and uses identical definitions as Martin et al. (Citation2011) for the variables capturing competitive intensity, localisation, urbanisation and diversity. In contrast to the latter, they constantly find positive effects for competition while the coefficients for location economies are only positive and significant in a very few specifications. The coefficients for urbanisation and diversity are usually not significantly different from zero. In a later study, Cainelli and Ganau (Citation2018) uses distances to define regions. The study analyses the effect of intra-industry and inter-industry externalities on TFP and find a positive coefficient for the intra-industry density measure on short and medium distances, but a negative one for the inter-industry density measure on the distances up to 15 km. This is interpreted as positive effects from localisation economies and negative ones from variety. Harris and Moffat (Citation2015) analyse the relationship between the population density of regions in the UK, which serves as an indicator for urban economies, and TFP growth. They find a positive relationship. In a further study, Harris and Moffat (Citation2022, p. 1725) show that ‘agglomeration economies are indeed an important source of London’s productivity advantage’. Distinguishing between localisation and diversification, they find Marshallian localisation is an important factor. Finally, Holl (Citation2016) uses data on Spanish manufacturing firms when analysing the relationship between the TFP of firms and employment density as well as the population density at the municipal level and the distance to the next highway. A nearby highway and a higher density are found to increase TFP. The latter points to the positive effects of urbanisation.
Apart from differences in the data and the results, the aforementioned studies share a number of common characteristics,Footnote2 of which one is most relevant for our research question: All of the studies apply a two-stage approach. The first stage foresees the estimation of a Cobb–Douglas production function estimation, usually at the level of two-digit industries, from which the firm-level TFP are obtained. In a second stage, all observations are pooled across industries and TFP is regressed on agglomeration economies indicators and additional control variables. Note that such two-stage procedures can cause econometric issues, which we discuss in detail in Section A of the online Appendix. More importantly, however, the pooling of observations in the second stage of the process imposes the strong assumption that agglomeration effects are uniform across all industries. In other words, by pooling across all sectors, one expects that canned soup manufacturers and producers of computer chips benefit in the same way and to the same extent from being located in an agglomeration.
However, we already noted that firms do not gain uniformly from new knowledge, new technologies and innovations, as shown by, inter alia, Kancs and Siliverstovs (Citation2016). Their study analyses the effect of R&D, as a proxy for innovation, on TFP at the firm-level using an international firm-level data set. The main take away from that study is that of large heterogeneity in the effect of R&D across firms, which, however, is not random. Instead, the effect increases with the level of technological intensity of industries. The effect is even negative for small investments by low-tech companies. This is consistent with other studies on the differences between low-tech and high-tech industries in terms of the impact of R&D and knowledge spillovers, as Tsvetkova et al. (Citation2020) show in their survey study.
Firms engage in much more than just R&D in order to stay competitive; they seek to improve their knowledge, to enhance their technology, and, ultimately, to become more productive. This includes investments in computerised information, innovative properties, and economic competencies, what has become known as intangible capital or knowledge based capital.Footnote3 Thus, intangible capital captures many aspects that are linked to agglomerations as emphasised by the OECD (Tsvetkova et al., Citation2020). The latest Schumpeterian growth models predict that firms differ in their ability to make productive use of intangible capital, i.e., they differ in their ability to benefit from new knowledge, which results in productivity dispersion (Aghion et al., Citation2019; DeRidder, Citation2019). First empirical evidence confirms that the effect of intangible capital on TFP is heterogeneous and generally stronger in high-tech industries than medium-tech or low-tech industries (Le Mouel & Schiersch, Citation2020). In sum, the literature on R&D and other intangible assets suggests a positive relationship between the technology-intensity of industries and the effect of innovation, improved knowledge and the like.
Therefore, we drop the implicit assumption of identical effects of agglomeration economies across industries as applied in previous studies and postulate the following research question: Do agglomeration effects differ systematically between industries of different technology intensity? We suspect this to be the case. Given the aforementioned findings, we further suspect that the agglomeration effects to be stronger in more knowledge-intensive or technological-intensive sectors. Addressing this question adds new insights into the relationship between TFP at firm-level and agglomeration effects, thus closing an existing gap in the literature on this topic.
To the best of our knowledge, this question is not examined in existing studies on the relationship between firm-level TFP and agglomeration effects with one notable exception: As part of a robustness check, Ehrl (Citation2013) presents results from a second stage in which the firms are not pooled across all industries, as was the case for the main result, but across four groups of industries. One group consists of all the service sectors in the dataset. The remaining three groups capture high-tech, the medium-tech and the low-tech manufacturing industries. Ehrl (Citation2013) finds that the main result only holds for the medium-tech industry and the service sector. In contrast, labour market pooling is not statistically significant for high-tech or low-tech industries. However, this is neither discussed nor explored any further.
3. MODEL AND ESTIMATION STRATEGY
The above presented literature consistently uses a two-step approach. We present this standard model and discuss its main issues in Section A of the online Appendix. Below, we present the econometric method that is used in this analysis and that overcomes the issues of the two-stage approach. In our analysis, we follow the literature insofar as we assume that a production function exists that transforms labour and capital inputs into outputs, as well as a function
that explains TFP and how it is affected by relevant variables. The function to be estimated, in logs, is shown in Equation (1):
(1)
(1) where
and
capture the firm and time dimensions, respectively, to account for the panel structure of the data.
We deviate from the aforementioned studies by following the productivity literature for modelling and estimating the relationship between TFP and the covariates that capture the characteristics of agglomerations. As in Aw et al. (Citation2011), Doraszelski and Jaumandreu (Citation2013) and De Loecker et al. (Citation2016), covariates that directly affect TFP are included in the law of motion explaining TFP. As shown subsequently, the elasticities of functions and
are then estimated jointly using a control function approach along the lines of Ackerberg et al. (Citation2015) (ACF procedure). In the following description of the econometric technique, we omit the index for the industry groups
for brevity.
Since the seminal paper of Levinsohn and Petrin (Citation2003), control function approaches utilise the assumption of an intermediate input demand function () with certain characteristics. These are, inter alia, that the intermediary input
is a fully flexible input, TFP
is the only unobserved state variable in
and
is strictly monotonic in
. Because of these assumptions,
is invertible and allows a function to be obtained that serves as a proxy for the unobserved TFP, i.e.,
.Footnote4 Apart from the intermediate input, capital and labour are also included in the control function. The latter dates back to the seminal papers of Ackerberg et al. (Citation2006, Citation2015) in which the authors prove that ‘
is functionally dependent of
,
, and
’ (Ackerberg et al., Citation2015, p. 2423), which is why identification of the labour coefficient in the first step fails, as in Levinsohn and Petrin (Citation2003). Moreover, De Loecker and Warzynski (Citation2012) states that all variables observed and relevant to the TFP should be included in the proxy function. This leads to the proxy function
, where the vector
captures additional variables. Furthermore, the ‘exact variables to be included in
depend on the application but will definitely capture variables leading to differences in optimal input demand across firms’ (De Loecker & Warzynski, Citation2012, p. 2446). If the general premise is correct, that agglomeration economies affect companies and their productivity in many different ways, then the respective agglomeration variables belong in
.
By substituting the unobserved TFP with , the function to be estimated in the first-step of the control function framework is:
(2)
(2) With
. Note that we also include R&D in function
, because the firms’ own R&D efforts positively affect its TFP, as shown by Aw et al. (Citation2011), Doraszelski and Jaumandreu (Citation2013), Kancs and Siliverstovs (Citation2016) and others.Footnote5 This also takes into account that ‘firms are neither equally equipped to receive knowledge nor homogeneously willing to serve as sources of spillover’ (Cainelli & Ganau, Citation2018, p. 923). By including R&D, our analysis actually controls for this ability and willingness. This goes beyond previous studies on the relationship between agglomeration economies and the TFP of firms. At this stage, additional control variables
are included, such as legal form, year and an East/West dummy.Footnote6
Equation (2) is estimated as the first step of the control function approach using OLS. Because the functional form is unknown, we follow the literature and use a polynomial as a proxy. As in all control function approaches, the first step is only conducted to overcome the endogeneity issue that comes from the fact that TFP is an unobserved variable that is correlated with the production inputs. Thus, we do not obtain input coefficients or calculate TFP at the end of the first step. Note that the input coefficients obtained from this estimation are not identified, thus requiring a second step.Footnote7 Control function approaches impose the assumption that TFP in
is determined by past experiences summarised in the information set
and a shock to productivity
. The shock
is assumed to be random, which is why it is uncorrelated with the information set (
). Following the literature,
is governed by a first-order Markov process and is also driven by other relevant variables. Assuming a linear relationship between R&D effort, agglomerations economies and TFP, this yields:
(3)
(3) Estimating Equation (3) is hampered by the fact that TFP is still unknown. Yet, from
and
follows
(4)
(4)
Equation (4) is estimated by means of GMM in an iterative process that minimises the error term using starting values for input coefficients and calculated in the first step of the process. Note that by estimating Equation (4), not only are the input coefficients
obtained, but also, simultaneously, the coefficients for the agglomeration variables
.
For the coefficients to be consistent, the variables need to be orthogonal to , which is unobserved and, therefore, part of the error term. Identification is based on timing assumptions regarding the firms’ decisions for the different inputs. Ackerberg et al. (Citation2015) show that contemporaneous labour is a rather flexible input and that this creates an issue: even if labour is considered less flexible than materialFootnote8 and even if firms decide upon its use after
but before
, hence at
with
, it is still at least partly influenced by
. Therefore, the authors advise to use lagged labour as an instrument because the decision regarding its use is taken at
and, thus,
is not correlated with
. The same reasoning holds for R&D (see Aw et al., Citation2011; Doraszelski & Jaumandreu, Citation2013; Kancs & Siliverstovs, Citation2016). Since Olley and Pakes (Citation1996), it is assumed that a firm’s decision on investing is taken in
but fully implemented in
, which is why
is uncorrelated with
. Finally, we impose the assumption that location is exogenous to the firm, which is why all contemporaneous agglomeration variables are orthogonal to
. Hence, the following moment conditions apply:
with
. Following the bulk of the literature,
is assumed to be a Cobb–Douglas function.
While the econometric technique used ensures a correct estimation of agglomeration effects on TFP, it does not directly answer the research question. To this end, our estimation strategy foresees several separate estimations. First, we follow the literature and estimate the effect for the entire manufacturing sector without distinguishing between technology intensities. In this way, we can compare our results with those in the literature and put them in context. We then conduct the analysis separately for the four groups of industries. The firms are assigned to the high-tech, medium high-tech, medium low-tech, and low-tech industries in accordance with the Eurostat definition.Footnote9 The estimations are conducted separately for each group.Footnote10 The results for each group are then compared with the results of the estimations based on pooled data. From this, conclusions can be drawn as to whether agglomeration effects differ systematically between industries with different technological intensities.
4. DATA
4.1. Datasets
The analysis uses the AFiD-Panel manufacturing firms, which contains the Cost Structure Survey for manufacturing firms (CS), the Investment Census for manufacturing firms (IC) and the Annual Report for manufacturing firms (AR). The data are collected and processed by the German Federal Statistical Office and the Statistical Offices of the Länder.Footnote11 The three datasets are combined by the Statistical Office using unique firm IDs. Konold (Citation2007), Koch (Citation2007) and Fritsch et al. (Citation2004) provide a detailed discussions of the datasets.Footnote12 The combined dataset covers the 2003 to 2014 period. It is enriched with deflator series for material, value added, and capital as well as deprecation rates at the two-digit industry level. These data are provided by the Statistical Office. For privacy reasons, firm-level data do not contain the individual address of firms. Yet, the data include the so called ‘Amtlicher Gemeindeschlüssel’ (AGS). This is an eight-digit code that is unique for each municipality. Thus, each firm can be assigned to a municipality and each municipality to a region. The georeference codes of municipalities, the assignment of communities to the individual administrative units, i.e., NUTS-2 or NUTS-3 regions, are provided by the Federal Institute for Research on Building, Urban Affairs and Spatial Development (BBSR).Footnote13
The unprocessed dataset contains 467,397 observations for the 2003 to 2014 period. These are mostly observations from the Annual Report. This dataset is a census of all manufacturing and quarrying firms with at least 20 employees, containing only a few items, of which one is the number of persons employed.Footnote14 We use it to construct the indicators for agglomeration economies.
The production function estimations, however, are limited to those firms that are simultaneously part of the investment census and the cost structure survey, as these datasets contain the required production function inputs and the value added. This limits the number of observations that can be used in the production function estimation to roughly 186,000 (see Table C.2 (column CS&IS) in the online Appendix). From this dataset, some industries are dropped, such as mining, mainly because of too few observations.Footnote15
Following Cainelli et al. (Citation2015), those observations that are below the 0.5 percentile or above the 99.5 percentile in terms of the labour and capital productivity are dropped as outliers. This is done separately for each two-digit industry. Finally, all observation with missing observations in the relevant variables are also dropped. Material, value added and capital (the respective investments) are deflated with respective series per two-digit industry. The final dataset used in the production function estimations is an unbalanced panel with 174,863 observations. Table C.3 in the Appendix shows the main descriptive statistics.
We make use of administratively defined regions, namely the 96 ‘Raumordnungsregionen’ (ROR).Footnote16 These regions are made up of NUTS-3 regions and are constructed such that they capture an economic centre and its surroundings. The affiliation of a municipality to an ROR is determined by commuter flows, political structures, central services, etc., and set by the BBSR. The BBSR provides the table containing the municipalities per ROR.Footnote17 Each firm is assigned to one of the 96 ROR, based on the eight-digit AGS code of the municipality where the firm is located.
4.2. Indicators of agglomeration economies
The following indicators are constructed to capture the different effects of agglomerations, regardless of the spatial definition applied.
4.2.1. Localisation
The variable is supposed to capture the potential benefit that comes with a high concentration of firms from the same industry in a region. The variable is defined as
(5)
(5) where
is the number of employees in firm
at time
in a two-digit industry
and region
. The denominator is the sum of all employees working in industry
at time
in the entire country. In other words, the variable measures the region’s share of employment in an industry, corrected for the number of employees in the firm under consideration and varies by firm, time, region and two-digit industry.
4.2.2. Competition
The variable captures regional competition intensity among firms in an industry
at time
in region
. It is calculated by means of the inverse of the Herfindahl index of employment concentration:
(6)
(6) where
is the sum of all employees working in industry
at time
in region
. The variable varies across regions, industries, and time, but is identical for all firms within a specific two-digit industry in a region at time
.
4.2.3. Urbanisation
Population density, measured as inhabitants per square kilometre, is regularly used as indicator for urbanisation economies. For firm at time
that is located in region
and active in the two-digit industry
, the urbanisation economies variable is defined as:
(7)
(7) Given this definition, all firms in a region, regardless of their industry affiliation, face the same population density. Thus, the variable varies across time and regions.
4.2.4. Diversity
In the spirit of Frenken et al. (Citation2007) and in line with Basile et al. (Citation2017), we distinguish between related and unrelated variety. The related variety is measured by means of the inverse of the Herfindahl index of employment using the shares of the four-digit industries within a two-digit industry
in a region
at time
. Accordingly, the variable is defined as:
(8)
(8) where
is the sum of employment in each four-digit industry
in region
at time
, whereby only those four-digit industries
are considered that belong the two-digit industry
that firm
belongs to. Simply put, it measures the diversity within a two-digit industry in a region. The variable varies over time, region and two-digit industry. The unrelated variety uses the shares of two-digit industries
in region
at time
:
(9)
(9) where
is the sum of all employees working in region
at time
, whereas
is the sum of all employees working in industry
at time
in region
. It measures the diversity of two-digit industries within a region. The variable varies over time and regions.
5. RESULTS
shows the estimation results for our main specification, i.e., when the agglomeration indicators are constructed based on RORs. The first two rows show the elasticities of labour and capital from the production function. The coefficients for labour and capital are significant and have an order of magnitude that is within a reasonable range in all estimations.Footnote18 This assures that the estimations provide reasonable results. The remaining rows contain the coefficients for the variables in the law of motion ().
Table 1. Elasticities of production inputs and variables in the law of motion; technology groups; and regions: ROR.
In a first step, we estimate the effect of agglomeration on the TFP of firms for the entire manufacturing sector. In doing so, we follow the standard approach in the literature, which uses pooled data. The first column in contains the corresponding results. We find that firms’ own R&D activity is an important driver of TFP, even when knowledge effects arising from agglomeration externalities are taken into account. Hence, supporting firms in their R&D activities is a meaningful economic policy. This finding is in line with the literature on the effects of R&D on TFP.Footnote19
However, column (1) also shows that all indicators of agglomeration effects have significant coefficients. The magnitudes of the elasticities are lower than those for R&D, but this is in line with expectations, since their own R&D efforts have a targeted effect on firms’ TFP, while agglomeration effects have a more general and more diffuse effect. We find that urbanisation and localisation positively affect the firms’ TFP, a result consistent with the bulk of the literature. We also see that diversity, whether of related or unrelated varieties, has a positive effect, although the magnitude is small and the significance is only at the 10% level in the case of the latter. Surprisingly, competition seems to unfold negative effects. In contrast, most studies find no significant effect or, as in the case of Martin et al. (Citation2011), the negative effect is not stable. Taken together, our results for manufacturing largely confirm that knowledge effects arising from agglomeration externalities are an important driver of TFP, even when firms’ own knowledge creation is taken into account.
In the following, we assess the heterogeneity of the effects between firms with different technological intensities and, in the process, also identify the source of the negative elasticity for competition in column (1). To begin with, heterogeneity is found with respect to the factors directly under control of the firms: the TFP in low-tech sectors is mostly determined by the previous period TFP, while TFP has a lower persistence in high-tech sectors. This is to be expected because firms in low-tech industries are less reliant on new technologies and knowledge than firms in high-tech industries, thus productivity in low-tech industries is less dynamic and more determined by past productivity. Consistent with this argument, we find that a company’s own research and development activities have, by far, the strongest effect on TFP in high-tech industries. In medium high-tech sectors, the impact of R&D is lower than in high-tech sectors, but still noticeably higher than in low-tech and medium low-tech industries. Both results show that the effect of firms’ own knowledge creation varies considerably between firms with different technological intensity.Footnote20
Large differences between industry groups are also found for the urbanisation variable. The degree of urbanisation has no effect on firms in low-technology sectors, while the largest effect on TFP is found for firms in high-technology industries. Moreover, the coefficient for the latter industry group (Table 1, column (5)) is three times larger than the coefficient estimated for manufacturing as a whole (column (1)). The coefficients for the variable that captures localisation economies have a similar sectoral pattern, with the strongest effect in high-tech industries (column (5)) and the lowest coefficient for low-tech sectors (column (2)). This confirms our assumption that firms with different technological intensities benefit to different degrees from agglomeration externalities.
With regard to competition, the results are more unambiguous. Increasing competition seems to have negative effects in low- and medium low-tech industries, but no statistically significant effects in high-tech industries. We interpret this as an indication that regional or local competitive intensity is rather unimportant for the mostly export-oriented and globally active high-tech firms. In contrast, the phenomenon of cut-throat competition seems to play out in more domestically oriented medium- and low-tech industries. Note that these differences also affect the results for manufacturing as a whole (column (1)). The negative coefficient for competition there is driven by firms from the medium- and low-tech industries, which make up the majority of the firms.
The findings for the two diversity variables also indicate large differences between industry groups. Like Martin et al. (Citation2011), however, no clear pattern emerges. A large intra-sectoral (RV) and inter-sectoral (URV) variety has a positive impact on firms in low-tech industries. As these are industries with rather simple technical and less knowledge-demanding requirements, they might actually gain from knowledge created by others and then adapted for use in a much more simplistic form given their technologically less demanding operations. At the same time, the indicators for related and unrelated variety are not significantly different from zero in the high-tech industries. This might be driven by the fact that these are usually firms with very specific technologies that are active in niche markets. For these firms, own knowledge creation will be more important.
Several problems could affect our results, in particular the issue of reverse causality and the modifiable area unit problem (MAUP) (Openshaw, Citation1984). The first is addressed by using historical employment data to create instruments and by modifying the empirical approach such that we are able to incorporate these instruments within our structural approach. The second issue is addressed by adapting the idea of Cainelli and Ganau (Citation2018) and using radii to define regions. For reasons of space, the corresponding econometric results and their discussion are presented in Section B of the online Appendix. That section further includes a discussion of other econometric issues and limitations of our study. By and large, however, the robustness tests support the results of our main specification.
In summary, the results of our analysis are consistent with most of the literature and confirm that agglomeration externalities are relevant factors for firms’ TFP development. Moreover, and more importantly, they show that agglomeration externalities have significantly different effects on firms operating with different technology intensities. This confirms our presumption formulated in Section 2. In addition, the differences in effects are partly consistent with the conjecture made there: positive knowledge effects arising from agglomeration externalities, in particular from urbanisation and localisation, tend to be stronger in more knowledge- or technologically-intensive industries.
6. CONCLUSION
Existing studies show that the productivity of firms benefits from agglomeration externalities. The existing empirical literature, however, implicitly assumes that the effects are identical across firms and industries. Building on insights from the literature on productivity effects of innovation, R&D and intangible capital, we drop this implicit assumption and study whether the effects of agglomeration differ between firms that operate with different technological intensity.
The analysis uses firm-level data from the German manufacturing industry covering the 2003 to 2014 period. Firms are assigned to high-tech, medium high-tech, medium low-tech and low-tech industry classifications following the definition provided by Eurostat. We apply an econometric approach that overcomes the econometric issue of the methods used so far. Specifically, we do not separate the estimation of the production function from the estimation of the effects of agglomeration externalities on TFP. In addition, our estimation function accounts for the impact of intra-firm knowledge creation by incorporating R&D into the law of motion that governs TFP.
We first follow the literature and consider the manufacturing sector as a whole. The results are consistent with the literature in confirming that agglomeration effects are important determinants of firms’ TFP. Going beyond these average effects, our analysis examines, for the first time to the our best of our knowledge, the heterogeneity of benefits from agglomeration across sectors with different technology intensities. First, such heterogeneity is found with respect to the factor directly under the control of firms: their own research and development activities have the largest impact on TFP in high-technology industries by far. In medium high-tech sectors, the impact of R&D is lower than in high-tech sectors, but still noticeably higher than in low-tech and medium low-tech industries. In other words, our analysis is in line with the recent literature that postulates heterogeneous effects of R&D across firms.
Importantly, we show that such heterogeneity can also be found with respect to agglomeration advantages. To start with, the level of urbanisation has the largest impact on TFP in high-tech industries and the smallest effect on low-tech sectors. This suggests that, for example, public research and education activities, which are concentrated in large population centres with high population densities, are likely to generate positive productivity effects. Although we cannot quantify the importance of the individual factors, the same presumably applies to the influence of cultural diversity or the presence of international actors, which also vary with spatial density. In turn, this would mean that the aforementioned benefits of urbanisation do not affect the TFP of firms in low-tech industries.
We further find substantial heterogeneity in the effect of increased concentration of firms of similar industries within regions, i.e., benefits due to localisation economies. The pattern is similar to the pattern found for urbanisation, with the strongest effect in high-tech industries and the lowest coefficient for low-tech sectors.
A large heterogeneity of the effects is also observed for the remaining indicators for agglomeration economies. However, the patterns are less unidirectional, as in the case of localisation. We find that stronger local competition seems to unfold negative effects in low-tech and medium low-tech industries, while changes in local competition are irrelevant for high-tech firms. The diversity of the local structure within the manufacturing sector has a positive impact on the TFP of firms in low-tech industries. In high-tech industries, on the other hand, the TFP of firms seems not to profit from an increased industrial diversity in a region, as measured by the related and unrelated variety.
These results show that firms benefit to varying degrees from diverse agglomeration effects and that these effects are not inherently present in all industries. This has implications for regional economic policy, especially when integrated into the framework of the Smart Specialisation Strategy introduced by the European Union. In general, the fact that the localisation variable is consistently positive and significant supports the formation of clusters. However, not every type of industry-cluster will develop successfully in every region. Our results support the formation of high-tech clusters in high-density regions. At the same time, complementary industrial structures do not need to be already in place for the successful development of the high-tech firms in these cluster. This is shown by the insignificance of the related and unrelated variety variables. Conversely, it also follows from our results that promoting the development of high-tech clusters in less densely populated regions – even if this may seem politically opportune – is less meaningful, because the firms cannot exploit their full productivity potential and will ultimately remain less competitive.
That said, our results also point to development opportunities for less densely populated areas. The results suggest that clusters of low- and medium low-tech industries can be particularly successful in less densely populated areas. For success, however, it is necessary that the newly developed industrial clusters fit into pre-existing structures – in some cases even in a complementary way. This is in line with previous studies on the success factor of the Smart Specialisation Strategy, which emphasise that the regional context is always decisive for the functionality of Smart Specialisation Strategies (Foray et al., Citation2021; Panori et al., Citation2022; Ruhrmann et al., Citation2022).
Supplemental Material
Download PDF (485.6 KB)ACKNOWLEDGMENTS
We gratefully acknowledge funding received by the Hans-Böckler-Stiftung as part of the research project ‘Industry in the City’ (project number 2016-973-1). We thank the Advisory Board of this project for the valuable suggestions and comments. We are grateful to the team at the Research Data Center of the Berlin-Brandenburg State Statistical Office for their extensive support. Finally, we wish to thank two anonymous reviewers and the editor for their valuable comments and suggestions, which significantly improved the paper. An earlier version of this paper circulated as a working paper under the title ‘Agglomeration Economies and Firm TFP: Different Effects across Industries’.
DISCLOSURE STATEMENT
No potential conflict of interest was reported by the authors.
Notes
1. We abstain from presenting studies using solely aggregated data, whether aggregated at the sector level, the regional level, or both, such as Siller et al. (Citation2021), Beugelsdijk et al. (Citation2018) or Antonelli et al. (Citation2011). Studies including agglomeration variables as standard inputs into a production function are also not discussed here (see, inter alia, Harris, Citation2021; Maré & Graham, Citation2013; Melo et al., Citation2009). The reason is that, regardless of whether the dependent variable is gross output, value added or labour productivity, the underlying assumption in such models is that agglomeration economies directly affect the output of firms, just like labour or capital. By definition, this means that they do not affect TFP.
2. These other characteristics are: First, almost all of the studies use the method proposed by Levinsohn and Petrin (Citation2003) for estimating the production functions. However, this method does not solve the endogeneity problem that is inherent to production function estimations (for a discussion see Ackerberg et al., Citation2007). Second, the agglomeration variables are mostly ignored when estimating the production functions and calculating TFP, causing the estimations and the obtained TFP to suffer from the omitted variable bias (see Section A in the online Appendix for more). Third, and with the exception of Cainelli and Ganau (Citation2018), all studies use administratively defined regions and mostly ignore the well-known modifiable areal unit problem (MAUP) (Openshaw, Citation1984). Finally, all studies ignore R&D as an independent explanation and covariate in the analysis of the effect of agglomeration variables on TFP, while it is well known that R&D is a driving factor of TFP development (see for example Doraszelski & Jaumandreu, Citation2013). If some of the agglomeration variables are correlated with R&D, as can be expected in the case of urbanisation, the analysis will suffer from the omitted variable bias.
3. These are the three main categories, of which each contains various knowledge based assets. See Corrado et al. (Citation2005, Citation2009, Citation2016) for an overview.
4. See Levinsohn and Petrin (Citation2003) for the proof of invertibility.
5. There is a large literature on the relationship between R&D and the productivity of firms as well as the direct effect of R&D on firm performance that is not discuss here. Ugur et al. (Citation2016) and Møen and Thorsen (Citation201Citation7) provide an overview and conduct meta analyses confirming the beneficial effect of R&D.
6. The East/West dummy is needed to control for the substantial differences that still exist in all economic parameters between East and West German regions.
7. For a comprehensive description of the reasons, see Ackerberg et al. (Citation2007).
8. This is a necessary assumption.
10. See Table C.1 for the list of industries and their assignment to the different groups.
11. In all estimations, industry dummies for two-digit industries are included as additional controls in X.
12. Hereafter, we use the term Statistical Office for simplicity.
13. See also www.forschungsdatenzentrum.de for details on the data and for data access.
14. For more information, see https://www.bbr.bund.de/BBR/EN/Home/_node.html.
15. In some industries, the threshold is 10 employees because of the size structure of the respective industries. The share of employees working in firms with fewer than 10 total employees in the German manufacturing industry is 7%.
16. We follow Richter and Schiersch (Citation2017) and drop observations from the mining industries (B5-B9), from the manufacturing of tobacco products (C12) and from the manufacturing of refined petroleum products (C19). The number of observations is insufficient in these industries, which leads to conflicts with the privacy policy rules applied in the Statistical Offices. We also drop manufacturing of other transport equipment (C30). The industry is quite heterogeneous as it includes shipbuilding, train manufacturing, aircraft construction, truck construction, as well as construction of rockets and satellites. This results in implausible estimates for labour and capital.
17. Figure A.2 in Gornig and Schiersch (Citation2019) provides a visual overview of the ROR.
18. The ROR are largely compatible with the German metropolitan areas as defined by the OECD (Citation2012).
19. Compare, for example, with the findings in Duso et al. (Citation2021).
20. See, among others, Ugur et al. (Citation2016) for a review of the relationship between productivity and R&D.
REFERENCES
- Ackerberg, D., Benkard, C., Berry, S., Pakes, A. (2007). Econometric tools for analyzing market outcomes. In J. J. Heckman & E. E. Leamer (Eds.), Handbook of econometrics. Vol. 6A (Ch. 63, pp. 4171–4276). North-Holland.
- Ackerberg, D., Caves, K., & Frazer, G. (2006). Structural identification of production functions. MPRA Paper No. 38349.
- Ackerberg, D. A., Caves, K., & Frazer, G. (2015). Identification properties of recent production function estimators. Econometrica, 83(6), 2411–2451. https://doi.org/10.3982/ECTA13408
- Aghion, P., Bergeaud, A., Boppart, T., Klenow, P. J., & Li, H. (2019). A theory of falling growth and rising rents. NBER Working Paper No. 26448.
- Ahrend, R., Farchy, E., Kaplanis, I., & Lembcke, A. C. (2017). What makes cities more productive? Agglomeration economies and the role of urban governance: Evidence from 5 OECD countries. OECD Productivity Working Papers, No. 6.
- Alcidi, C., Ferrer, J. N., Musmeci, R., DiSalvo, M., & Pilati, M. (2018). Income Convergence in the EU: Within-country regional patterns. CEPS Commentary, 5. February. https://www.ceps.eu/ceps-publications/income-convergence-eu-within-country-regional-patterns/
- Antonelli, C., Patrucco, P. P., & Quatraro, F. (2011). Productivity growth and pecuniary knowledge externalities: An empirical analysis of agglomeration economies in European regions. Economic Geography, 87(1), 23–50. https://doi.org/10.1111/j.1944-8287.2010.01104.x
- Arrow, K. J. (1962). The rate and direction of inventive activity: Economic and social factors. Princeton University Press, Ch. Economic welfare and the allocation of resources for invention, pp. 609–626.
- Aw, B. Y., Roberts, M. J., & Xu, D. Y. (2011). R&D investment, exporting, and productivity dynamics. American Economic Review, 101(4), 1312–1344. https://doi.org/10.1257/aer.101.4.1312
- Barro, R. J., & Sala-i-Martin, X. (1995). Economic growth theory. McGraw-Hill.
- Basile, R., Pittiglio, R., & Reganati, F. (2017). Do agglomeration externalities affect firm survival? Regional Studies, 51(4), 548–562. https://doi.org/10.1080/00343404.2015.1114175
- Belitz, H., Gornig, M., & Schiersch, A. (2019). Productivity: Urban-rural differences affect productivity more than east-west differences. DIW Weekly Report, 43, 387–393. https://doi.org/10.18723/diw_dwr:2019-43-1
- Beugelsdijk, S., Klasing, M. J., & Milionis, P. (2018). Regional economic development in Europe: The role of total factor productivity. Regional Studies, 52(4), 461–476. https://doi.org/10.1080/00343404.2017.1334118
- Cainelli, G., Fracasso, A., & Marzetti, G. V. (2015). Spatial agglomeration and productivity in Italy: A panel smooth transition regression approach. Papers in Regional Science, 94(Supplement 1), S40–S67. https://doi.org/10.1111/pirs.12103
- Cainelli, G., & Ganau, R. (2018). Distance-based agglomeration externalities and neighbouring firms’ characteristics. Regional Studies, 52(7), 922–933. https://doi.org/10.1080/00343404.2017.1360482
- Capello, R., & Perucca, G. (2018). Understanding citizen perception of European Union cohesion policy: The role of the local context. Regional Studies, 52(11), 1451–1463. https://doi.org/10.1080/00343404.2017.1397273
- Caragliu, A., de Dominicis, L., & de Groot, H. L. (2016). Both marshall and jacobs were right!. Economic Geography, 92(1), 87–111. https://doi.org/10.1080/00130095.2015.1094371
- Combes, P.-P., Duranton, G., & Gobillon, L. (2008). Spatial wage disparities: Sorting matters!. Journal of Urban Economics, 63(2), 723–742. https://doi.org/10.1016/j.jue.2007.04.004
- Combes, P.-P., Duranton, G., Gobillon, L., Puga, D., & Roux, S. (2012). The productivity advantages of large cities: Distinguishing agglomeration from firm selection. Econometrica, 80(6), 2543–2594. https://doi.org/10.3982/ECTA8442
- Corrado, C., Haskel, J., Jona-Lasinio, C., & Iommi, M. (2016). Investment and investment finance in Europe: Financing productivity growth. European Investment Bank, Ch. Growth, tangible and intangible investment in the EU and US before and since the Great Recession, pp. 73–103.
- Corrado, C., Hulten, C., & Sichel, D. (2005). Measuring Capital in a New Economy. National Bureau of Economic Research and University of Chicago Press, Ch. Measuring Capital and Technology: An Expanded Framework, pp. 11–46.
- Corrado, C., Hulten, C., & Sichel, D. (2009). Intangible capital and the US economy. The Review of Income and Wealth, 55(3), 661–685. https://doi.org/10.1111/j.1475-4991.2009.00343.x
- De Loecker, J., Goldberg, P. K., Khandelwal, A. K., & Pavcnik, N. (2016). Prices, markups, and trade reform. Econometrica, 84(2), 445–510. https://doi.org/10.3982/ECTA11042
- De Loecker, J., & Warzynski, F. (2012). Markups and firm-level export status. American Economics Review, 102(6), 2437–2471. https://doi.org/10.1257/aer.102.6.2437
- DeRidder, M. (2019). Market power and innovation in the intangible economy. Mimeo.
- DiGiacinto, V., Gomellini, M., Micucci, G., & Pagnini, M. (2014). Mapping local productivity advantages in Italy: Industrial districts, cities or both? Journal of Economic Geography, 14(2), 365–394. https://doi.org/10.1093/jeg/lbt021
- Doraszelski, U., & Jaumandreu, J. (2013). R&D and productivity: Estimating endogenous productivity. The Review of Economic Studies, 80(4), 1338–1383. https://doi.org/10.1093/restud/rdt011
- Duranton, G., & Puga, D. (2004). Microfoundations of urban agglomeration economies. In J. V. Henderson, & J.-F. Thiss (Eds.), Handbook of regional and urban economics. Vol. 4 (Ch. 48, pp 2063–2117). North-Holland.
- Duso, T., Nardotto, M., & Schiersch, A. (2021). Broadband and productivity: Structural estimates for Germany. DIW Discussion Paper 1988.
- Ehrl, P. (2013). Agglomeration economies with consistent productivity estimates. Regional Science and Urban Economics, 43(5), 751–763. https://doi.org/10.1016/j.regsciurbeco.2013.06.002
- European Commission. (2021). The smart specialisation concept. EU-smart specialisation platform. https://s3platform.jrc.ec.europa.eu/what-we-do.
- European Committee of the Regions. (2021). Smart specialisation strategies are a cornerstone of EU’s sustainable recovery. https://cor.europa.eu/en/news/Pages/Smart-specialisation-strategies-as-a-cornerstone-of-EU-policies.aspx.
- Faberman, J. R., & Freedman, M. (2016). The urban density premium across establishments. Journal of Urban Economics, 93, 71–84. https://doi.org/10.1016/j.jue.2016.03.006
- Foray, D. (2014). From smart specialisation to smart specialisation policy. European Journal of Innovation Management, 17(4), 492–507. https://doi.org/10.1108/EJIM-09-2014-0096
- Foray, D., Eichler, M., & Keller, M. (2021). Smart specialization strategies—Insights gained from a unique European policy experiment on innovation and industrial policy design. Review of Evolutionary Political Economy, 2(1), 83–103. https://doi.org/10.1007/s43253-020-00026-z
- Frenken, K., Oort, F. V., & Verburg, T. (2007). Related variety, unrelated variety and regional economic growth. Regional Studies, 41(5), 685–697. https://doi.org/10.1080/00343400601120296
- Fritsch, M., Görzig, B., Hennchen, O., & Stephan, A. (2004). Cost structure surveys for Germany. Schmollers Jahrbuch: Journal of Applied Social Science Studies/Zeitschrift für Wirtschafts- und Sozialwissenschaften, 124(4), 557–566.
- Glaeser, E. L., Kallal, H. D., Scheinkman, J. A., & Shleifer, A. (1992). Growth in cities. Journal of Political Economy, 100(6), 1126–1152. https://doi.org/10.1086/261856
- Goecke, H., & Hüther, M. (2016). Regional convergence in Europe. Intereconomics, 51(3), 165–171. https://doi.org/10.1007/s10272-016-0595-x
- Gornig, M., & Schiersch, A. (2019). Agglomeration economies and firm tfp: different effects across industries. DIW Discussion Paper 1788.
- Harris, R. (2021). The importance of spatial differences in total factor productivity: New Zealand, 2001–2016. Regional Studies, 55(7), 1209–1227. https://doi.org/10.1080/00343404.2020.1869204
- Harris, R., & Moffat, J. (2015). Total factor productivity growth in local enterprise partnership regions in Britain, 1997–2008. Regional Studies, 49(6), 1019–1041. https://doi.org/10.1080/00343404.2013.799770
- Harris, R., & Moffat, J. (2022). The geographical dimension of productivity in Great Britain, 2011–18: The sources of the London productivity advantage. Regional Studies, 56(10), 1713–1728. https://doi.org/10.1080/00343404.2021.2004308
- Henderson, J. V. (1986). Efficiency of resource usage and city size. Journal of Urban Economics, 19(1), 47–70. https://doi.org/10.1016/0094-1190(86)90030-6
- Hervas-Oliver, J.-L., Sempere-Ripoll, F., Alvarado, R. R., & Estelles-Miguel, S. (2018). Agglomerations and firm performance: Who benefits and how much? Regional Studies, 52(3), 338–349. https://doi.org/10.1080/00343404.2017.1297895
- Holl, A. (2016). Highways and productivity in manufacturing firms. Journal of Urban Economics, 93, 131–151. https://doi.org/10.1016/j.jue.2016.04.002
- Iammarino, S., Rodriguez-Pose, A., & Storper, M. (2019). Regional inequality in Europe: Evidence, theory and policy implications. Journal of Economic Geography, 19(2), 273–298. https://doi.org/10.1093/jeg/lby021
- Isard, W. (1956). Location and space-economy; a general theory relating to industrial location, market areas, land use, trade, and urban structure. Technology Press of Massachusetts Institute of Technology.
- Jacobs, J. (1969). The economy of cities. Vintage.
- Kancs, A., & Siliverstovs, B. (2016). R&D and non-linear productivity growth. Research Policy, 45(3), 634–646. https://doi.org/10.1016/j.respol.2015.12.001
- Koch, A. (2007). Neue Datenquelle “Unternehmensregister” Mehr Informationen über den Mittelstand ohne neue Bürokratie. Institut für Mittelstandsforschung.
- Konold, M. (2007). New possibilities for economic research through integration of establishment-level panel data of German official statistics. Schmollers Jahrbuch: Journal of Applied Social Science Studies/Zeitschrift für Wirtschafts- und Sozialwissenschaften, 127(2), 321–334.
- Le Mouel, M., & Schiersch, A. (2020). Knowledge-based capital and productivity divergence. DIW Discussion Paper 1868.
- Levinsohn, J., & Petrin, A. (2003). Estimating production functions using inputs to control for unobservables. Review of Economic Studies, 70(2), 317–341. https://doi.org/10.1111/1467-937X.00246
- Maré, D. C., & Graham, D. J. (2013). Agglomeration elasticities and firm heterogeneity. Journal of Urban Economics, 75, 44–56. https://doi.org/10.1016/j.jue.2012.12.002
- Marshall, A. (1920). Principles of economics. Macmillan and Co., Ltd.
- Martin, P., Mayer, T., & Mayneris, F. (2011). Spatial concentration and plant-level productivity in France. Journal of Urban Economics, 69(2), 182–195. https://doi.org/10.1016/j.jue.2010.09.002
- Matano, A., & Naticchioni, P. (2012). Wage distribution and the spatial sorting of workers. Journal of Economic Geography, 12(2), 379–408. https://doi.org/10.1093/jeg/lbr013
- McCann, P., & Ortega-Argilés, R. (2015). Smart specialization, regional growth and applications to European union cohesion policy. Regional Studies, 49(8), 1291–1302. https://doi.org/10.1080/00343404.2013.799769
- Melo, P. C., Graham, D. J., Levinson, D., & Aarabi, S. (2017). Agglomeration, accessibility and productivity: Evidence for large metropolitan areas in the US. Urban Studies, 54(1), 179–195. https://doi.org/10.1177/0042098015624850
- Melo, P. C., Graham, D. J., & Noland, R. B. (2009). A meta-analysis of estimates of urban agglomeration economies. Regional Science and Urban Economics, 39(3), 332–342. https://doi.org/10.1016/j.regsciurbeco.2008.12.002
- Møen, J., & Thorsen, H. S. (2017). Publication bias in the returns to R&D literature. Journal of the Knowledge Economy, 8(3), 987–1013. https://doi.org/10.1007/s13132-015-0309-9
- OECD. (2012). Redefining “urban”: A new way to measure metropolitan areas. OECD Publishing.
- Olley, S., & Pakes, A. (1996). The dynamics of productivity in the telecommunications equipment industry. Econometrica, 64(6), 1263–1297. https://doi.org/10.2307/2171831
- Openshaw, S. (1984). The modifiable areal unit problem. Geo Books.
- Panori, A., Kakderi, C., & Dimitriadis, I. (2022). Combining technological relatedness and sectoral specialization for improving prioritization in smart specialisation. Regional Studies, 56(9), 1454–1467. https://doi.org/10.1080/00343404.2021.1988552
- Porter, M. E. (1990). The competitive advantage of nations. Free Press.
- Quah, D. T. (1996). Regional convergence clusters across Europe. European Economic Review, 40(3–5), 951–958. https://doi.org/10.1016/0014-2921(95)00105-0
- Richter, P. M., & Schiersch, A. (2017). CO2 emission intensity and exporting: Evidence from firm-level data. European Economic Review, 98(C), 373–391. https://doi.org/10.1016/j.euroecorev.2017.07.011
- Rocchetta, S., Ortega-Argilés, R. F., & Kogler, D. (2022). The non-linear effect of technological diversification on regional productivity: Implications for growth and smart specialisation strategies. Regional Studies, 56(9), 1480–1495. https://doi.org/10.1080/00343404.2021.1939292
- Romer, P. M. (1986). Increasing returns and long-run growth. The Journal of Political Economy, 94(5), 1002–1037. https://doi.org/10.1086/261420
- Rosenthal, S. S., & Strange, W. C. (2004). Evidence on the Nature and Sources of Agglomeration Economies. Vol. 4. Elsevier, Ch. 49, pp. 2243–2291.
- Ruhrmann, H., Fritsch, M., & Leydesdorff, L. (2022). Synergy and policy-making in German innovation systems: Smart specialisation strategies at national, regional, local levels? Regional Studies, 56(9), 1468–1479. https://doi.org/10.1080/00343404.2021.1872780
- Siller, M., Schatzer, T., Walde, J., & Tappeiner, G. (2021). What drives total factor productivity growth? An examination of spillover effects. Regional Studies, 55(6), 1129–1139. https://doi.org/10.1080/00343404.2020.1869199
- Tsvetkova, A., Ahrend, R., Martins, J. O., Lembcke, A., Knutsson, P., Jong, D., & Terzidis, N. (2020). The spatial dimension of productivity: Connecting the dots across industries, firms and places. OECD Regional Development Working Papers 2020/1.
- Ugur, M., Trushin, E., Solomon, E., & Guidi, F. (2016). R&D and productivity in OECD firms and industries: A hierarchical meta-regression analysis. Research Policy, 45(10), 2069–2086. https://doi.org/10.1016/j.respol.2016.08.001
- Weber, A. (1929). Theory of the location of industries. Cersity Press.