Abstract
Background and aim
Understanding treatment preferences in those patients who are not responding to corticosteroids for ulcerative colitis is important in informing treatment choices. This study aimed to assess the relative importance of treatment characteristics to patients by conducting a discrete-choice experiment.
Methods
Patients completed the questionnaire online. All data were collected between September and December 2020. Participants were shown 13 discrete-choice experiment tasks – a series of side-by-side comparisons of competing, hypothetical treatment characteristics and asked to select a preferred treatment. Survey responses were analysed using descriptive statistics and regression analyses.
Results
115 patients completed the study. Patient preferences were strongest for treatments with a lower chance of side effects, this attribute had the most influence on the choice of treatment patients preferred. The second most important attribute was an improvement in maintaining remission. Conversely, route and frequency of administration were least important on the choice of treatment patients preferred. Respondents were willing to make trade offs and accept treatment benefits to compensate them for receiving a treatment with a less desirable attribute level. Participants were willing to accept a larger benefit of 45% improvement in maintenance of remission to accept a treatment with a higher probability of side effects. The benefit required was smaller with a 10% improvement in remission required to accept a treatment with a lower probability of side effects.
Conclusion
Quantifying preferences helps to identify and prioritise treatment characteristics that are important to patients. The results highlight the importance of careful discussion of side effects, including the magnitude of risk, using visualisation tools during a patient consultation to support decisions.
Introduction
Ulcerative colitis is a chronic inflammatory condition that is characterised by debilitating symptoms of diarrhoea, rectal bleeding, abdominal pain, fatigue and impaired quality of life [Citation1]. Patients experiencing a disease flare are often treated with corticosteroids [Citation2]. However, just under 50% of patients do not respond fully to steroids [Citation3]. This requires further treatment and individuals are therefore faced with selecting a treatment in partnership with their clinician.
Treatment options include small molecules, biologic agents, thiopurines or surgery [Citation4]. Besides the effectiveness of treatments, other considerations may be important for patients when opting for treatment, including drug side effects, travel requirements to receive infusions and time off work [Citation5]. Treatment efficacy may also incorporate time to respond, steroid-free remission, and longer-term treatment success. The best treatment option for patients will depend on how they value the characteristics of treatments. Understanding these patient values is important to choose a treatment that closely aligns with these values [Citation6–8]. This information can then be used to facilitate the shared decision-making process between the patient and clinician [Citation9–11].
Discrete-choice experiments (DCEs) have been increasingly used to identify patient preferences in healthcare research [Citation12]. DCEs quantify the strength of preferences for the features of hypothetical treatments that are most important to patients. While studies have applied the DCE method to different types of ulcerative colitis [Citation13–17], evidence on preferences for the steroid-resistant population is limited. Consequently, this study aimed to understand the relative importance of treatment characteristics to patients with steroid-resistant ulcerative colitis by conducting a DCE.
Methods
Questionnaire design
The first section of the survey contained the DCE tasks (see Supplementary Data 1). At the start of the DCE section, we described a steroid-resistant ulcerative colitis scenario for participants to imagine. Then participants were shown a series of side-by-side comparisons of competing treatment profiles and were asked to select their preferred treatment profile (See ). The reason for describing an ulcerative colitis scenario for participants to imagine rather than asking patients to think about their own situation was to allow all patients to answer the DCE based on the same baseline. This context-setting is important because the clinical presentation of ulcerative colitis varies from patient to patient and otherwise would have introduced significant heterogeneity.
The DCE was developed by reviewing the literature, conducting qualitative interviews with patients, and consulting patient representatives. We conducted thirty-three interviews with patients to identify key treatment characteristics patients consider important when selecting a treatment [Citation18]. Thematic analysis of the qualitative interviews generated eight themes, which were ranked by the four patient and public involvement (PPI) group members. These members were patients with ulcerative colitis who have had medical or surgical treatments. Using a dot-voting technique [Citation19], the patients were given sixteen dots to distribute across the eight themes, where themes with the most dots revealed the most desirable treatment characteristics. This process helped to convert and reduce the eight themes to attributes. Upon discussions with the PPI group, one theme around the need for regular monitoring was dropped as this was deemed the least important theme compared to the others. Themes that were similar or correlated were merged. For example, route and frequency of administration were merged to create one single attribute. Similarly, quality of life and inducing a treatment response were merged as the two attributes were highly correlated to create another single attribute. The final five attributes focused on effectiveness, remission, speed of response, treatment administration and safety of the treatment (). The levels for the attributes were selected to reflect plausible values from the published clinical trials literature. For the side-effects attribute, we used the levels described in medical labels (i.e., very common, common) as patients are familiar with it.
Table 1. Treatment attribute descriptions and levels.
The DCE questions were generated using the NgeneTM software [Citation20]. The combination of attributes and levels (three attributes with three levels each and two attributes with four levels each) produced 432 possible treatment profiles, so to create a manageable DCE questionnaire computer software was used to select the treatment profiles to be included in the questionnaire (fractional factorial design). The software produced a D-optimal design that followed the principles of minimum overlap, orthogonality, and level balance [Citation21]. Only one version of the survey was generated using NgeneTM which contained 12 unlabelled DCE questions. One additional dominant question that was logically better was also included to test whether participants understood the DCE task [Citation22]. These 13 questions were displayed to the participants in a random order using the QualtricsTM survey platform. To create a realistic choice, participants were not given an opt-out option because treatment for ulcerative colitis is necessary to improve their length and quality of life.
Section two of the survey involved a ranking exercise where patients were asked to rank four commonly used treatments (adalimumab, infliximab, tofacitinib, and vedolizumab,) in order of preference from one to four. To aid this task, we provided comprehensive details of the treatments which included information on the effectiveness of the drug, speed of response to treatment, route of administration, side effects, and whether concomitant medication is needed [Citation23]. These treatment descriptions were developed using published literature with clinical input from the study team and presented to participants in a randomised order to reduce question order bias (see Supplementary Table 2).
In section three, we gathered sociodemographic details and the respondents’ personal history and severity of ulcerative colitis. The survey included two validated instruments, the IBD-Control-8 questionnaire and the EQ-5D-5L questionnaire. The IBD-Control-8 questionnaire captured disease control and impact from the respondents’ perspective. It generated a summary score ranging from 0 representing worst control to 16 representing best control of disease and a score of 13 and above representing quiescent disease (Cronbach's α = 0.86) [Citation24]. The EQ-5D-5L instrument captured respondents’ overall quality of life, generating a summary score between −0.59 and 1, where higher scores represented a better quality of life [Citation25]. Section four contained feedback questions about the survey.
To increase face validity, the survey was piloted by three patient representatives who were not involved in the first stage of the DCE design to check if it was feasible for patients to complete the survey. Upon receiving the pilot feedback, we simplified the way the DCE was presented by providing detailed explanations of the attributes at the start of the DCE exercise. A series of screens displayed instructions providing detailed descriptions of the attributes, for example, we explained risks qualitatively in the instruction screen (i.e., a drug that is 60% effective means that if 100 people had the same drug for ulcerative colitis, for 60 people the treatment would be effective but for 40 people treatment would not be effective) [Citation26] but in the DCE tables risks were shown numerically (i.e., 60 in 100, 60%) as it was quicker for the participants to read. In addition to the pilot, a review of responses was undertaken after 50 respondents completed the study to assess problems, including comprehension and dropouts.
Participants
The study population included adults aged at least 18 years who had a diagnosis of ulcerative colitis. Participants were primarily recruited through two National Health Service (NHS) hospitals, in an outpatient (non-hospitalised) setting. Staff working in inflammatory bowel disease (IBD) clinics advertised the study by sending potential participants invitation letters. The study was also advertised on social media to recruit further participants from across the UK. If individuals decided to take part, they were able to access the online survey via the QualtricsTM platform and complete the survey after providing informed consent. We hoped to recruit up to 300 survey participants on the basis of precedence where the literature shows that the DCE sample size ranges between 100 to 300 participants [Citation27]. However, the minimum sample size required was N ≥ 83.3 (N = 500 × (4 [maximum number of levels])/(2 [# of alternatives] x 12 [# of tasks]) to estimate a model using the rule of thumb approach [Citation28].
Statistical analysis
Descriptive statistics were performed to analyse demographic data, IBD characteristics of the respondents and to rank the order of importance of medications. We performed conditional logistic regression models to analyse the DCE task data. All attributes were included as independent variables; attributes were first included as categorical variables, but after checking for linear relationships through visual inspection and model fit, speed and remission attributes were treated as continuous variables and effectiveness, administration and side effects as categorical variables in the model.
Parameter estimates from the conditional model were also used to calculate minimum acceptable benefit and to calculate the change in probability of uptake from a baseline scenario where all attributes are set to their worst level and then improved each attribute one at a time [Citation29] (see Supplementary Data 2 for an example calculation). All statistical analyses were conducted using STATATM v16.
Ethical considerations
The study was reviewed and approved by the NHS Research Ethics Committee – East Midlands Derby (19/EM/0011) and the Health Research Authority approved the research (IRAS ID: 255616).
Results
Study population
Seven hundred and twenty invitation letters were sent to eligible participants. Of the invited participants, 166 visited the QualtricsTM survey platform, 115 completed the survey and 5 people declined to complete the survey. Based on the respondents who visited the survey, the response rate was 69% however, based on the total invites sent, the response rate is 16% (see ). All data were collected between September and December 2020. presents the socio-demographic characteristics of the respondents and the clinical and treatment characteristics of the sample including current and previous medical treatments. The median age was 41 years and 52% of the responders were female. Our study sample is representative of the UK ulcerative colitis population in terms of gender and age [Citation30]. The majority of patients were white, employed and educated to a secondary school level or above. The median time from diagnosis was 10 years. The median IBD-Control-8 score was 13. Fifty-five percent of patients reported quiescent disease with a score of 13 and over. The median quality of life score was 0.77, which is lower than the UK general population's mean quality of life score of 0.85 [Citation31]. The majority of patients had previously received steroids (93%), thiopurines (70%) and biological therapies or tofacitinib (70%) – most commonly infliximab (51%).
Table 2. Patient characteristics and ulcerative colitis medication.
Patient preferences weights
All attributes had a significant influence on the choice of treatment patients preferred. shows the relative changes that make respondents more likely (positive coefficient) or less likely (negative coefficient) to take a treatment. A treatment having a lower likelihood of side effects (i.e., a change from very common to very rare (ß 2.937, p < .001) or very common to uncommon (ß 2.260, p < .001) or very common to common (ß 1.417, p < .001)) strongly increased the likelihood of the respondent choosing a treatment. Similarly, higher levels of the maintenance of remission attribute strongly influenced respondents’ choice of treatment (1% increase in remission: ß 0.065, p < .001). When induction of response was higher 50% or 60% (relative to 40%), respondents were also more likely to choose the treatment. However, patients were less likely to choose a treatment that takes longer to improve their symptoms (change per week: ß −0.145, p < .001). Orally administered medication, taken daily (ß 0.848, p < .001) and injections at home every 8 weeks (ß 0.541, p < .001) were preferred to infusions every 8 weeks at the hospital. Notably, a change from infusion at the hospital every 8 weeks to injection, every 2 weeks at home, was not significant (ß −0.029, p = .85) suggesting that patients found these two ways of administering the treatment comparable (see Supplementary Table 1).
Overall rank ordering of attribute importance
To compare the attributes that had the most or least importance on the choice of treatments patients preferred we calculated the change in predicted probabilities from a baseline scenario compared to a new scenario. In the baseline scenario, the treatment profile consisted of the worst possible levels for all the attributes, and in each new scenario, only one attribute level was improved at a time to find out how important that attribute was when selecting a treatment. shows the change in uptake rates of the improved treatment profiles. Larger changes in uptake rates indicate more favourable treatment attributes. Treatment with very rare side effects was ranked very favourably with a change in uptake rate of 90 percentage points (). Comparing the change in probabilities across these scenarios, the attributes can be compared in terms of priority, with side effect attributes having the most influence on the choice of treatment patients preferred followed by treatment with a 70% rate for maintaining remission. Conversely, route and frequency of administration were least important on the choice of treatment patients preferred.
Figure 3. Overall rank ordering of attribute importance. Note: Each vertical line represents the change in uptake rate of two treatments profiles. The baseline treatment profile was constant where induction of response was 40%, speed of response was 14 weeks, the remission rate was 35%, mode of administration was injection every 2 weeks at home, with very common side effects. One attribute level was improved from the base level in each improved treatment profile.
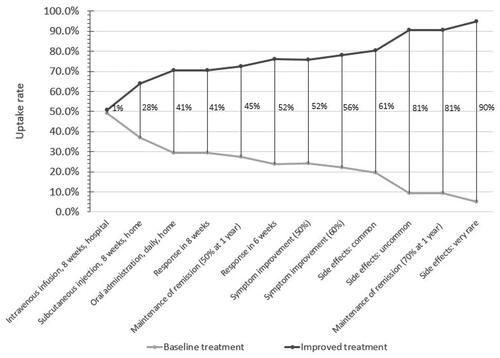
Minimum acceptable benefit
A minimum acceptable benefit was calculated to find the minimum change in benefit required by patients to compensate them for receiving a treatment with a less desirable attribute level, such as the chance of experiencing side effects. shows the percentage point increase in maintenance of remission required to compensate participants for the worsening levels of the chance of getting side effects. Participants would require a larger 45% improvement in maintenance of remission to accept the worst change in risk of side effects, an increase from very rare to very common. When the increase in the risk of side effects is smaller, from very rare to common, the participants are willing to accept a 23.22% improvement in maintenance of remission. Even smaller 10.35% improvement in remission was required to choose a treatment with change in risk from very rare to uncommon side-effect. Participants preferred route of administration was a pill but to switch from taking a pill to another mode of administration participants would require a larger improvement in maintenance of remission, specifically, a 13.39% increase in remission to administer an injection every two weeks or a 12.95% increase in remission to have an infusion every 8 weeks at the hospital or a 4.69% increase in remission to administer an injection every eight weeks.
Table 3. Minimum acceptable benefit.
Ranking treatments
When participants were asked to rank the four biological treatments in order of preference, where a ranking of ‘1′ equated to most preferred treatment and ‘4′ equated to least preferred, the most preferred treatments were infliximab (38%) and tofacitinib (38%), followed by vedolizumab (17%) and adalimumab (6%) (). The least preferred medication was adalimumab (54%), followed by vedolizumab (26%), infliximab (11%) and tofacitinib (9%). A subgroup (n = 35) of biologic-naive patients was analysed separately and compared to those who were on biologics. For the biologic-naive subgroup, the most preferred treatment ordering changed, with tofacitinib ranked first (46%), followed by infliximab (37%), vedolizumab (11%) and adalimumab (6%). The least preferred treatment was adalimumab (60%), vedolizumab (26%), infliximab (9%), and tofacitinib (6%). This ordering was similar to the full sample. Moreover, comparing the ranking treatments with modelled predictions of the DCE tasks showed that infliximab (46%) was the most preferred treatment followed by tofacitinib (42%), vedolizumab (6%) and adalimumab (5%) (see Supplementary Table 3). While both methods yielded similar treatment preference rankings, the proportion of shares of treatment was different.
Understanding and engagement
All participants completed all the choice tasks and for the remainder of the survey, missing data was low and did not exceed 5%. The majority of (97%) survey participants answered the dominant choice question (where one treatment profile was logically better) correctly. Only four participants failed this internal validity test and the modelling results did not change when the four participants were excluded from the analysis (results not reported), which suggests that participants were able to understand the DCE tasks and the probabilities presented in them (see Supplementary Table 4). Moreover, participants’ feedback about the survey showed that the majority of respondents understood the DCE (92%) and ranking tasks (91%) and agreed that the information provided in the DCE task was appropriate (70%) (see Supplementary Table 4).
Discussion
This study was conducted to elicit patient preferences in the treatment of steroid-resistant ulcerative colitis. In this cohort of patients with ulcerative colitis, choices of treatment were most influenced by fewer side effects compared to other attributes in their decision making. Improvement in maintaining remission was also valued but less than treatments with fewer side effects. It is possible that the conduct of the study during the COVID-19 pandemic influenced patients prioritising side effects over symptom improvement because of heightened fears about infections from hospital attendance or as a result of immunosuppressive medication. The result highlights the importance of careful discussion of side effects, including the magnitude of risk, for example using visualisation tools during a patient consultation to support decisions.
Infliximab and tofacitinib were rated as the most preferred treatments by this sample of patients. In the UK infliximab and adalimumab are the most widely used biologics. Tofacitinib is more recently available and prescribing is less frequent [Citation32]. The findings suggest that the place of tofacitinib earlier in the treatment pathway for steroid-resistant ulcerative colitis needs evaluation. This will be affected by factors including specific toxicities that were not part of the DCE such as thromboembolic disease and herpes zoster infection.
This is the first DCE study to focus on patients with steroid-resistant ulcerative colitis conducted in the UK, though other studies have used DCEs to quantify patient preferences for IBD treatments [Citation13–15,Citation33,Citation34]. Our finding, that treatments with fewer side effects are most important to patients, contrasts with the study by Bewtra, where patients with IBD were prepared to accept relatively high risks of lymphoma to avoid a disease relapse over the next 5 years [Citation35]. Almario et al. also found that efficacy was the most important attribute for patients with ulcerative colitis, but that side effects were the key priority for patients with Crohn’s disease [Citation15]. Boeri et al. reported that symptom improvement and risk of malignancy were the two most important attributes. Similar to our study, Boeri et al. reported that patients preferred oral modes of administration compared to subcutaneous or intravenous administration [Citation14]. Our results also showed that less frequent administration of subcutaneous injections were preferred. Feedback from a patient panel indicated that frequent injections could be intrusive or a reminder of their illness.
This study has several strengths. The DCE was conducted to a high standard following published best practice guidelines [Citation22]. The study included an internal validity check to test if participants understood the DCE task. Only four people failed the internal validity DCE question and the findings did not change when the four participants were excluded from the analysis. Also, the majority of participants reported that they understood the survey questions and the information provided in the DCE tasks was deemed sufficient. Additionally, the survey was developed by conducting qualitative interviews with patients [Citation18] and accompanied by iterative rounds of piloting, with the help of patient and clinical representatives to ensure that information was accurate and easily understandable.
This study also has some limitations. First, while every effort was made to objectively describe the four treatments, interpretation of the ranking of treatments should take into consideration the uncertainty in the evidence base. While there is a significant amount of data assessing treatment safety and effectiveness there are several uncertainties in the evidence base [Citation36]. The majority of the evidence comes from placebo-controlled efficacy trials with only limited head-to-head trials and some real-world evidence from registries [Citation36]. This is further complicated by different definitions of outcomes, which are not always measured with the same instrument or scale [Citation36]. Thus, developing an accurate treatment profile was challenging. Nevertheless, to ensure rigour and objectivity, the descriptions were compiled with key statistics which were calculated using systematic reviews and with input from experienced consultant gastroenterologists. Due to the significant heterogeneity that exists in the literature, it is possible that different researchers could use different statistics, and until the gaps in the evidence base are met by well-conducted head-to-head trials this problem is likely to persist.
Second, our intention was to recruit 300 participants, however, due to the pandemic we were unable to achieve this target as staff at NHS trusts were working under extreme pressures and the data collection period was shortened. Nevertheless, the sample size in our study was larger than the minimum sample required according to the rule of thumb approach to estimate a model and was sufficient to run the regression analyses and find statistically significant results. Larger sample size would also have enabled better subgroup analyses. We estimated models to assess preference heterogeneity using interaction terms for gender, ethnicity, age groups but we did not find statistically significant differences, probably due to the small sample size in the subgroups (results not reported). The response rate in this study was also low. Possible reasons include the inability to recruit from a face-to-face clinic attendance with the switch to remote clinics during the CoViD-19 pandemic. It is possible that the respondents filling the survey differ from non-respondents but we do not have data on those who did not complete the survey and therefore firm conclusions cannot be drawn.
A third challenge is surrounding the content of the DCE. The task involved trading hypothetical treatment profiles which were defined by five attributes. The selection of attributes contained a trade off, the more attributes we include in the DCE task, the higher the likelihood that the task will become unwieldy contributing to poor quality of data [Citation37]. Consequently, a few attributes were excluded from the DCE, for example, the time burden involved with treatment monitoring or the option to have or avoid surgery. It is unclear the impact of excluding these attributes from the DCE and how respondents would behave differently when these factors are introduced in the real world. The study was planned and undertaken before the availability of ustekinumab for ulcerative colitis in the UK and ustekinumab was therefore not included in the ranking exercise. Whilst this might have affected the uptake rates for particular agents, the drug attributes prioritised by patients are still applicable in terms of efficacy and toxicity.
Understanding patient preferences have implications for decision-making. By quantifying the importance of different treatment characteristics, this study helps to facilitate discussions between patients and clinicians when reviewing treatment options. Results highlight the importance of clearly communicating risks of treatment using visualisation tools to have more informed discussions during a patient consultation. Also, selecting treatments that are effective but fast-acting, and providing patients the opportunity to select how they would like to administer the treatments should be encouraged where possible. Improving communication between clinicians and patients about different treatment characteristics could lead to better therapeutic decision-making. Treatments that align closely to patient preferences are likely to increase adherence to treatment, improve shared decision making and improve patient satisfaction [Citation9–11]. Non-adherence to treatment not only impairs patient recovery but also costs valuable resources [Citation38]. Prioritising treatment characteristics that patients value when considering treatments could lead to better-shared decision-making and better outcomes. Future research could evaluate the trade offs involved with choosing surgery vs medical therapies, facilitators for prescribing tofacitinib and improving ways to provide personalised treatments.
Ethics statement
The study was reviewed and approved by the NHS Research Ethics Committee – East Midlands Derby (19/EM/0011) and the Health Research Authority approved the research (IRAS ID: 255616).
Supplemental Material
Download PDF (764.9 KB)Acknowledgments
We would like to thank all participants who took part in this research. Many thanks to Professor Arne Risa Hole, Dr. Laura Ternent, Professor Tariq Iqbal, Dr. Gabrielle Thorpe and Mrs. Jayne Kranat for providing guidance during this study.
Disclosure statement
Dr. Lee was a member of the medical research panel for Crohn’s and Colitis UK and reports funding from Crohn’s and Colitis UK. Dr. Probert reports non-financial support from Vifor, and personal fees from Dr. Falk Pharma, Celltrion, Galapagos and Janssen. Dr. Sebastian reports grants from Takeda, Amgen, Pfizer, AbbVie, Tillott Pharma, and personal fees from AbbVie, Celgene, Takeda, Janssen, Tillots Pharma, Falk Pharma, Amgen, and Pharmcocosmos. Dr. Lobo reports personal fees from and Advisory Board membership for Takeda, personal fees from Janssen, Advisory Board membership for Vifor Pharma, Celltrion and Medtronic, and grants from CCUK and grants from Health Foundation. All other authors report no competing interests.
Data availability statement
The data underlying this article will be shared on reasonable request to the corresponding author
Correction Statement
This article has been corrected with minor changes. These changes do not impact the academic content of the article.
Additional information
Funding
References
- Brooks AJ, Rowse G, Ryder A, et al. Systematic review: psychological morbidity in young people with inflammatory bowel disease – risk factors and impacts. Aliment Pharmacol The. 2016;44(1):3–15.
- European Medicines Agency. Guideline on the development of new medicinal products for the treatment of Crohn’s Disease; 2016 [cited 2020 Mar 10]. Available from: http://www.ema.europa.eu/docs/en_GB/document_library/Scientific_guideline/2016/07/WC500211430.pdf.
- Ho G-T, Chiam P, Drummond H, et al. The efficacy of corticosteroid therapy in inflammatory bowel disease: analysis of a 5-year UK inception cohort. Aliment Pharmacol Ther. 2006;24(2):319–330.
- Lamb CA, Kennedy NA, Raine T, et al. British Society of Gastroenterology consensus guidelines on the management of inflammatory bowel disease in adults. Gut. 2019;68(Suppl 3):s1–s106.
- Louis E, Ramos-Goñi JM, Cuervo J, et al. A qualitative research for defining meaningful attributes for the treatment of inflammatory bowel disease from the patient perspective. Patient. 2020;13(3):317–325.
- Baars JE, Markus T, Kuipers EJ, et al. Patients’ preferences regarding shared decision-making in the treatment of inflammatory bowel disease: results from a patient-empowerment study. Digestion. 2010;81(2):113–119.
- Arseneau KO, Sultan S, Provenzale DT, et al. Do patient preferences influence decisions on treatment for patients with steroid-refractory ulcerative colitis? Clin Gastroenterol Hepatol. 2006;4(9):1135–1142.
- Casellas F, Guinard Vicens D, García-López S, et al. Consensus document on the management preferences of patients with ulcerative colitis: points to consider and recommendations. Eur J Gastroenterol Hepatol. 2020;32(12):1514–1522.
- Bager P, Julsgaard M, Vestergaard T, et al. Adherence and quality of care in IBD. Scand J Gastroenterol. 2016;51(11):1326–1331.
- Lindfors P, Axelsson E, Engstrand K, et al. Online education is non-inferior to group education for irritable bowel syndrome: a randomized trial and patient preference trial. Clin Gastroenterol Hepatol. 2021;19(4):743–751. e1.
- Abraham NS, Naik AD, Street RL. Shared decision making in GI clinic to improve patient adherence. Clin Gastroenterol Hepatol. 2012;10(8):825–827.
- de Bekker‐Grob EW, Ryan M, Gerard K. Discrete choice experiments in health economics: a review of the literature. Health Econ. 2012;21(2):145–172.
- Bewtra M, Johnson FR. Assessing patient preferences for treatment options and process of care in inflammatory bowel disease: a critical review of quantitative data. Patient. 2013;6(4):241–255.
- Boeri M, Myers K, Ervin C, et al. Patient and physician preferences for ulcerative colitis treatments in the United States. Clin Exp Gastroenterol. 2019;12:263–278.
- Almario CV, Keller MS, Chen M, et al. Optimizing selection of biologics in inflammatory bowel disease: development of an online patient decision aid using conjoint analysis. Am J Gastroenterol. 2018;113(1):58–71.
- Patel DB, van Deen WK, Almario CV, et al. Assessing patient decision-making on biologic and small-molecule therapies in inflammatory bowel diseases: insights from a conjoint analysis in the United States, Canada, and the United Kingdom. Inflammatory Bowel Dis. 2021;27(10):1593–1601.
- Wijnands AM, te Groen M, Peters Y, et al. Patients prioritize a low-volume bowel preparation in colitis-associated colorectal cancer surveillance: a discrete choice experiment. Inflammatory Bowel Dis. 2021;27(9):1–8.
- Barr A, et al. P96 factors influencing treatment preferences in steroid resistant ulcerative colitis–a qualitative interview study. Gut. 2021;70:A90-A91.
- Dalton J. Great big agile: dot voting. Waterford: Springer; 2019. p. 165–166.
- Metrics C. Ngene 1.2 user manual & reference guide. Sydney, Australia: Choice Metrics; 2018.
- Carlsson F, Martinsson P. Design techniques for stated preference methods in health economics. Health Econ. 2003;12(4):281–294.
- Lancsar E, Louviere J. Conducting discrete choice experiments to inform healthcare decision making: a user's guide. Pharmacoeconomics. 2008;26(8):661–677.
- Bonovas S, Lytras T, Nikolopoulos G, et al. Systematic review with network meta-analysis: comparative assessment of tofacitinib and biological therapies for moderate-to-severe ulcerative colitis. Aliment Pharmacol Ther. 2018;47(4):454–465.
- Ormerod CR. The IBD control questionnaire: the development and psychometric validation of a questionnaire for measuring inflammatory bowel disease control from the patient's perspective. Liverpool (UK): University of Liverpool; 2019.
- Devlin NJ, Shah KK, Feng Y, et al. Valuing health-related quality of life: An EQ-5D-5L value set for England. Health Econ. 2018;27(1):7–22.
- Ryan M, Gerard K, Amaya-Amaya M. Using discrete choice experiments to value health and health care. Vol. 11. Netherland: Springer Science & Business Media; 2007.
- de Bekker-Grob EW, Donkers B, Jonker MF, et al. Sample size requirements for discrete-choice experiments in healthcare: a practical guide. Patient. 2015;8(5):373–384.
- Orme B. Getting started with conjoint analysis: strategies for product design and pricing research second edition. Madison: Research Publishers; 2010.
- Lancsar E, Louviere J, Flynn T. Several methods to investigate relative attribute impact in stated preference experiments. Soc Sci Med. 2007;64(8):1738–1753.
- King D, Reulen RC, Thomas T, et al. Changing patterns in the epidemiology and outcomes of inflammatory bowel disease in the United Kingdom: 2000-2018. Aliment Pharmacol Ther. 2020;51(10):922–934.
- Janssen MF, Szende A, Cabases J, et al. Population norms for the EQ-5D-3L: a cross-country analysis of population surveys for 20 countries. Eur J Health Econ. 2019;20(2):205–216.
- Kotwani P, Terdiman J, Lewin S. Tofacitinib for rescue therapy in acute severe ulcerative colitis: a real-world experience. J Crohns Colitis. 2020;14(7):1026–1028.
- Bewtra M, Kilambi V, Fairchild AO, et al. Patient preferences for surgical versus medical therapy for ulcerative colitis. Inflamm Bowel Dis. 2014;20(1):103–114.
- Bewtra M, Reed SD, Johnson FR, et al. Variation among patients with Crohn's disease in benefit vs risk preferences and remission time equivalents. Clin Gastroenterol Hepatol. 2020;18(2):406–414. e7.
- Bewtra M, Fairchild AO, Gilroy E, et al. Inflammatory bowel disease patients' willingness to accept medication risk to avoid future disease relapse. Am J Gastroenterol. 2015;110(12):1675–1681.
- Gordon JP, McEwan PC, Maguire A, et al. Characterizing unmet medical need and the potential role of new biologic treatment options in patients with ulcerative colitis and Crohn's disease: a systematic review and clinician surveys. Eur J Gastroenterol Hepatol. 2015;27(7):804–812.
- Ratcliffe J, Longworth L. Investigating the structural reliability of a discrete choice experiment within health technology assessment. Int J Technol Assess Health Care. 2002;18(1):139–144.
- Selinger CP, Robinson A, Leong RW. Clinical impact and drivers of non-adherence to maintenance medication for inflammatory bowel disease. Expert Opin Drug Saf. 2011;10(6):863–870.