Abstract
Background
Recent studies have suggested a potential causal association between Interleukins (ILs) and Colorectal Cancer (CRC), and thus, it is important to examine the causal relationship between them using a Mendelian randomization (MR) approach.
Methods
The instrumental variables were extracted for IL-1ra, IL-6, IL-6ra, IL-8, IL-16, IL-18, IL-27 from genome-wide association studies of European ancestry. Summary statistics of CRC were also retrieved. An inverse variance-weighted MR approach was implemented as the primary method to compute overall effects from multiple instruments. Additional MR approaches and sensitivity and heterogeneity pleiotropy analyses were also conducted respectively.
Results
Our analysis suggested a causal effect between an increase of IL-8 and a reduced risk of CRC (odds ratio 0.65; 95% confidence interval, 0.43–0.98; p = 0.041) and did not provide evidence for causal effects of IL-1ra, IL-6, IL-6ra, IL-16, IL-18, IL-27. Sensitivity analyses suggested the robustness of MR results and that they were unlikely to be affected by unbalanced pleiotropy or significant heterogeneity.
Conclusions
This study investigated the role of ILs in the development of CRC and we found a causal effect between an increase of IL-8 and a reduced risk of CRC but not found evidence for causal effects of IL-1ra, IL-6, IL-6ra, IL-16, IL-18, IL-27. Sensitivity analyses suggested the robustness of MR results and that they were unlikely to be affected by unbalanced pleiotropy or significant heterogeneity.
Introduction
Colorectal Cancer (CRC) is the fourth most common cancer and the second leading cause of cancer-related deaths in developed countries [Citation1]. It is a complex disease with multiple contributing factors, including environmental and genetic factors. Current research suggests that immune cells are an important component of the tumor microenvironment and that tumor infiltration of immune cells is an important trigger for tumor cell growth and development and for achieving immune escape [Citation2]. Interleukins (ILs), on the other hand, are a class of cytokines that regulate immune cells or are secreted by immune cells, they are tiny proteins that can be grouped into a number of families, each of which has more than 40 subfamilies [Citation3]. Inflammatory ILs from the same family that are found in the tumor can either stimulate or inhibit immune reactions to it. Most of cytokines in CRC cause a more tumorigenic effect [Citation4]. Some ILs, such as IL-2, IL-4 and IL-6, have been identified as having a role in the onset, progression and metastasis of CRC by influencing tumor growth and angiogenesis. Increased expression of IL-4, for instance, promotes tumor growth and angiogenesis in CRC [Citation5,Citation6]. IL-2 is also known to suppress quantitatively or qualitatively the immune response against CRC, while increased expression of IL-6 leads to stimulation of tumor growth in CRC by inducing pro-tumorigenic microenvironmental inflammation [Citation7–9]. Therefore, elevated IL levels could potentially be used as prognostic markers for CRC [Citation10]. Additionally, because ILs are involved in both the anti-tumor and pro-tumor activities of the immune system, efforts should be made to target specific ILs for cancer therapy, as this could potentially be an effective way of treating CRC [Citation11]. However, the causal relationship between ILs and CRC has yet to be established and as such, further investigation is needed.
Although progressing research has improved the understanding of the relationship between ILs and CRC, it is still not clear whether ILs are the consequent or causal factor of the CRC. This is the limitation of inferring Associations between Phenotypes and diseases. To overcome this limitation, GWAS and other advanced methods including Mendelian randomization (MR) analysis are in constant evolvement. MR is an instrumental variable (IV) based method that uses single nucleotide polymorphisms (SNPs), as an IV, as depicted in . SNPs are common genetic variants that can alter the functional consequences of proteins and contribute significantly to phenotypic variation in the general population [Citation12]. A strong association between an SNP and a disease can reveal that the SNP is related to the disease or that it is in linkage disequilibrium (LD) with related SNPs [Citation13]. SNPs are created before phenotypes and diseases, thus avoiding reverse causality. The IVs should meet two assumptions to conduct MR analysis [Citation14,Citation15]. The first condition is that SNPs should be robustly associated with the phenotype (ILs), the second is that SNPs can influence the disease only through the phenotype. Z (e.g. SNPs) represents IVs, X is the phenotype (e.g., ILs) and Y is the CRC.
Figure 1. Study design of Mendelian randomization between interleukins and CRC. The black solid lines represent the association between the instrumental variables and exposure as well as the association between exposure and outcome. Dash lines with a cross means that the association meets two basic assumptions of Mendelian randomization: (1) the genetic variants are independent of confounders between exposure and outcomes, (2) the genetic variants only influence the outcome via exposure.
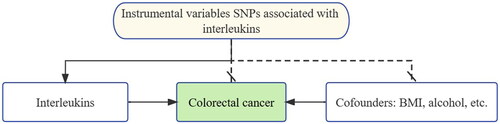
For this aim, we established an effective and reliable data processing framework, which made it possible to generate IVs for MR analysis. And then, using an inverse variance weighted (IVW) MR approach, which utilized a meta-analysis framework combining Wald estimates for each SNP to derive an overall estimate of the effect of biomarker levels on risk, we calculated the pool result based on IVs. To further guarantee the reliability of our method, Additional MR approaches (the weighted median approach and the MR-Egger regression) and leave-one-out validation were conducted in this step too. An analysis that leaves out one variant (i.e., removes one variant from the analysis and re-estimates the causal effect) can be valuable in assessing how dependent an MR analysis is on a particular variant. Moreover, Heterogeneity and pleiotropy tests were used to minimize false-positive conclusions and the risk of producing unreliable results. Finally, a full report was generated to provide an overview of the results of our study.
Methods
Instrumental variables
Summary-level data of GWAS dataset was the fundamental for MR analysis. IVs were extracted from ILs related GWAS dataset and CRC related GWAS dataset was then used in the further analysis. To meet the MR assumptions and reduce the bias, the summarized GWAS data was processed. Subsequently, MR analysis involving IVW MR approach, leave-one-out validation, MR-Egger analysis was used to comprehensively assess the causal effect of ILs on the risk of the development of CRC.
Data sources
ILs (IL-1ra, IL-6, IL-6ra, IL-8, IL-16, IL-18, IL-27, n = 19,178) were measured among a panel of 90 candidate biomarkers related to cardiovascular risk. Summary statistics were released by the SCALLOP consortium [Citation16]. We extracted relevant SNPs, effect allele, allele frequencies, beta coefficients and standard errors (SEs) to serve as summary data. Summary statistics data for CRC were available from a genome-wide association meta-analysis of 177,028 European-descent individuals (3022 cases and 174,006 noncases) from FinnGen.
We processed the summarized GWAS data for constructing IVs of MR analysis. Here IVs are genetic variants [Citation17]. Each SNP should be significantly associated with ILs and should be not associated with CRC. Thus, we extracted SNPs significantly associated with ILs and then removed these SNPs associated with CRC. We defined p < 5 × 10−8 as significant associated SNPs of ILs and we defined p-value more than 0.05 as not associated SNPs of CRC. To avoid over-precise estimates due to genetic pleiotropy, we should remove these SNPs with potential LD relationships. To remove SNPs with LDs, we ranked significant SNPs of ILs based on p-values. Finally, significant SNPs of ILs (IL-1ra, IL-6, IL-6ra, IL-8, IL-16, IL-18, IL-27) were identified and used to examine the possible relationship between ILs and CRC. Genetic variants associated with ILs at genome-wide significant significance (p < 5 × 10−8) and clumped at the threshold (r2 < 0.001 within a window of ±1 Mb, using the European population (EUR) from the 1000 Genomes Project Phase 3 as the reference panel) were selected as IVs (SI). To see how these data correlate with other clinical databases, we validated the results of the data against TCGA database using the Gepia2 tool (http://gepia2.cancer-pku.cn/).
Mendelian randomization
Using genetic variants as IVs, MR is a technique that is gaining popularity for determining the causal relationships between exposures and outcomes. In order to guarantee the accuracy of causal reasoning, MR is predicated on a number of essential premises. Assumption 1: There is a significant association between the genetic variation used as an IV and the relevant exposure. This premise is crucial because it guarantees that the genetic variation is a reliable substitute for the exposure, enabling the estimation of a causal impact. Estimates of the causal impact may be skewed if this assumption is broken. Assumption 2: The genetic variation is not linked to any confounding elements that might skew the relationship between exposure and result. The second fundamental tenet of MR is that there are no confounding variables linked with the genetic variant used as an IV that might skew the relationship between exposure and outcome. Third supposition: The genetic variant has no other impact on the exposure besides how it affects the outcome. The genetic variant used as an IV only influences the result through its impact on the exposure, and not through any other pathway, according to the third fundamental tenet of MR.
In this study, we used the Two Sample MR (version 0.5.6) package in R 4.1.3 (R Foundation for Statistical Computing, Vienna, Austria) to conduct a MR analysis. We calculated the causal effect of ILs on site-specific CRC mediated by each individual instrumental SNP. Furthermore, we employed the IVW MR approach to generate overall estimates of the effect. Two complementary methods were also utilized: the weighted median approach, which is used to provide robust effect estimates when less than half the instruments are invalid, and the MR-Egger regression, which is used to detect unbalanced horizontal pleiotropy and generate pleiotropy-adjusted estimates. IVW estimates usually have more precise estimates of effect, whereas the weighted median and MR-Egger estimates were accompanied by wider confidence interval (CI) in the forest plots. The causal effects on CRC were presented per SD increase in circulating levels of ILs. In addition, to make a more accurate judgment about the relationship between ILs and CRC, we also used the Maximum likelihood and Unweighted regression two methods to strengthen the judgment.
Results
IVs of ILs based on SNPs
Summary statistics of instrumental SNPs as genetic IVs for ILs (IL-1ra, IL-6, IL-6ra, IL-8, IL-16, IL-18, IL-27) were presented in . These SNPs were not associated with CRC and have no LD associations. Corresponding associations with MS were extracted. Each line of the table included 10 items related to the SNP such as the SNPs, effect allele, chromosomal position, beta coefficients and SE of the SNP on the risk of the corresponding IL and CRC.
Table 1. Summary statistics of instrumental SNPs as genetic instrumental variables (IVs) for ILs (IL-1ra, IL-6, IL-6ra, IL-8, IL-16, IL-18, IL-27).
IL-8 as a potential anti-tumor agent in CRC
As shown in , our analysis suggested a causal effect between an increase of IL-8 and a reduced risk of CRC and did not provide evidence for causal effects of IL-1ra, IL-6, IL-6ra, IL-16, IL-18, IL-27. IL-8 levels may represent a decreased risk of CRC (odds ratio [OR] 0.65; 95% CI, 0.43–0.98; p = 0.041). Collectively, our results suggest that IL-8 may play an anti-tumor role in the development of CRC, and further studies are thus necessary to further explore this potential causal relationship.
Lack of causal association between IL-1ra, IL-6, IL-6ra, IL-16, IL-27 and CRC
MR results demonstrated that genetically-predicted levels of IL-1ra, IL-6, IL-6ra, IL-16, IL-27 were not associated with risk of CRC, IL-1ra (OR 0.95; 95% CI, 0.80–1.13; p =0 .543), IL-6 (OR 0.91; 95% CI, 0.70–1.20; p =0 .524), IL-6ra (OR 0.97; 95% CI, 0.92–1.02; p = 0.252), IL-16 (OR 0.96; 95% CI, 0.60–1.54; p = 0.862), IL-27 (OR 1.00; 95% CI, 0.93–1.08; p =0 .938), detailed information is provided in Figure S1.
Robustness of MR results: MR-Egger and heterogeneity tests
MR results were generally consistent among causal estimates given by IVW methods and two additional approaches. In MR analyses with three or more IVs, no horizontal pleiotropy was detected according to MR-Egger intercepts and no evident heterogeneity was identified, detailed information is provided in Table S1. In conclusion, our findings provide tentative evidence for a causal role of IL-8 in CRC, although further research is needed to confirm these results.
Leave-one-out analysis for MR results
The leave-one-out analysis was performed to assess the influence of individual SNPs on the estimates. The results demonstrate that the overall values obtained by the leave-one-out analysis were consistent with estimates obtained when all available SNPs were included in the analysis. Further information on the leave-one-out validation results can be found in Figure S2.
Differential expression of ILs between CRC and normal cells
The Gepia2 tool was used to study the differential expression of IL genes (IL-1ra, IL-6, IL-6ra, IL-18) between CRC and normal cells. While the results showed that IL-1ra is upregulated and IL-6ra is downregulated in tumor tissues, indicating that these genes are involved in the development of CRC and have diagnostic significance, it is important to note that these findings do not establish a causal relationship between IL-1ra/IL-6ra and CRC. The results can be found in Figure S3.
Discussion
In recent years, the widely used statistics-based GWAS has yielded valuable insights into the field of complex disorder genetics [Citation18]. In human GWAS data, MR is a powerful tool for inferring causality [Citation19]. In this study, by using GWAS summary data and MR analysis, we determined the causal relationship between ILs and CRC. In previous studies, ILs such as IL-1 receptor antagonist (IL-1Ra), IL-2 receptor subunit (IL-2R), IL-6, IL-16, IL-17 and IL-18 have been revealed by GWAS studies to play roles in various diseases [Citation20–24]. Articles have also used the MR method to elucidate the potential causal effects of ILs in diseases such as prostate cancer, rheumatoid arthritis (RA), and obstructive sleep apnea (OSA) [Citation25–29]. However, MR has not been fully utilized for the study of the relationship between ILs and CRC. As a result, it is necessary to investigate the potential contribution of ILs to CRC using MR analysis, which may shed light on the genetics of the condition and inspire novel preventative and therapeutic approaches.
This study used MR analysis to determine whether ILs may play a causal role in CRC. Furthermore, the results did not support any causal effect of IL-1ra, IL-6, IL-6ra, IL-16, IL-18, IL-27. Sensitivity analyses revealed the robustness of the results and indicated that the results were unlikely to be affected by unbalanced pleiotropy or significant heterogeneity.
Many studies have shown that ILs play a very important role in the occurrence and development of CRC [Citation30]. In clinical studies, compared with healthy controls, CRC patients had increased concentrations of M2 polarization-related factors (e.g., IL-1β, IL-4), neutrophil chemotactic and N2 polarization-related cytokines (e.g., IL-8), and decreased concentrations of IL-2 and IFN-γ [Citation31]. Cancer stem cells (CSCs), also known as tumor-initiating cells, are a subpopulation of tumor cells that exhibit self-renewal capacity and are an important factor in the pathogenesis of CRC [Citation32]. It has been shown that the type, location and density of adaptive immune cells present in the tumor microenvironment represent an independent prognostic factor for CRC patients [Citation33]. The increase of inflammatory cells and cytokines in the tumor microenvironment promotes malignant cell proliferation, metastasis, and epithelial-mesenchymal transition (EMT), as well as the loss of the acquired immune response [Citation34]. The ILs molecules IL-6 and IL-8 play a role in the interaction between CSCs and their microenvironment in CRC [Citation35]. IL-6 is an inflammatory factor with many biological effects, including tumor promotion [Citation36]. In CRC cells, IL-6 expression is regulated by nuclear factor-κB (NF-κB), which is involved in regulating the transcription of genes related to tumor proliferation [Citation37]. Among these, tumor-infiltrating T cells are a predictor of prognosis in CRC patients, and the multiple actions of IL-2 on T cells make its role in the tumor immune microenvironment very important [Citation38]. It has been suggested that IL-2 has a potential negative impact in the tumor immune response and may have implications for the future immunotherapy of CRC patients [Citation39]. IL-1β belongs to the IL-1 family, and IL-1 receptor antagonist (IL-1RA) is a compound that binds to the cell surface IL-1 receptor (IL-1R), the same receptor that binds to members of the IL-1 family, and prevents IL-1 from signaling to cells [Citation40]. In CRC patients, IL-1β and IL-1RA are highly expressed and regulated in vitro by IL-1β/1RA axis through autophagy for EMT, invasion, migration, clone formation, proliferation and apoptosis [Citation41]. IL-18 is one of the best characterized members of the IL-1 family. IL-18 has been shown to be a mediator product of the activation of the inflammasome/caspase-1 pathway by the NOD-like receptor pyridine-containing domain protein 3 (NLRP3) [Citation42]. It is a key regulator of signaling pathways involved not only in various immune responses but also in antitumor processes in various cancers [Citation43].
Neutrophils are the target cells for IL-8 activation [Citation44]. Knockdown of non-erythroid spectrin αII (SPTAN1) in CRC cell lines led to a significant increase in IL-8 expression at both the mRNA and protein levels, which in turn induced the migration of neutrophils. The study also suggests that CRC cells with low SPTAN1 expression may release increased quantities of IL-8, leading to increased immune cell infiltration in MLH1-deficient CRC [Citation45]. Meanwhile, considering its capacity to chemoattract polymorphonuclear leukocytes, IL-8 was the first chemokine to be identified [Citation46,Citation47]. IL-8 acts on its cognate receptors, CXCR1 and CXCR2, and is an important driver of inflammation [Citation48]. IL-8 is one of the key factors in tumors, because it can attract macrophages and granulocytes to the milieu of the tumor tissue, allowing them to function as myeloid-derived suppressor cells [Citation49,Citation50]. Additionally, it has been demonstrated that IL-8 induces trophic effects on cancer cells and CSCs and functions as a direct proangiogenic factor on tumor vasculature [Citation51,Citation52]. All in all, almost all studies have concluded that IL8 is pro-tumor. However, the results of our analysis are contrary to these results. The results of the analysis indicated that IL-8 has a causal effect between an increase of IL-8 and a reduced risk of CRC. In contrast, the analysis did not provide evidence for the causal effects of the other ILs (IL-1ra, IL-6, IL-6ra, IL-16, IL-18, IL-27) on CRC. This result may also be the result of fewer IV. In a nutshell the roles of these ILs in CRC may be more complex and require further investigation.
Using the most up-to-date IL data set and the largest available GWAS data set of CRC cases, we were able to leverage summary-level data from large-scale genetic consortia in order to ascertain weak associations between circulating ILs and CRC risk. This allowed us to have high statistical power and a reliably large sample size to study the novel relationships studied. But there are some limitations in this study that should be considered. First, while we looked at the effect of certain circulating ILs on CRC, it was not possible to explore potential nonlinear relationships with CRC. It is also noteworthy that ILs such as IL-2, -4 and -5, which have been previously linked to an increased risk of CRC, were not included in this study. Another limitation is that the associations between ILs and CRC were derived from a European cohort, making it difficult to extrapolate to other populations. Moreover, this study was limited to the effect of circulating ILs and did not evaluate intracellular function of ILs. To further understanding of the possible effects of ILs on CRC, larger and more comprehensive MR studies are warranted.
Conclusions
In summary, the results of this study suggested a significant causal between genetically predicted IL-8 and the risk of CRC. Due to the limitations of MR, we were not able to identify causal relationships between other ILs (IL-1ra, IL-6, IL-6ra, IL-16, IL-18, IL-27) and CRC, suggesting that they play complex roles and complex interactions between them, which also influence tumorigenesis and progression. To better elucidate the causal relationship between ILs and CRC, more large-scale prospective studies and mechanistic studies are needed.
Author contributions
Conceptualization: HF.J. and YM.L.; Methodology, HF.J.; Software, YM.L.; Validation, HF.J., YM.L. and R.Z.; Formal analysis, HF.J.; Investigation, YM.L.; Resources, R.Z.; Data Curation, R.Z.; Writing—original draft preparation, R.Z.; writing—review and editing, L.Y.; Visualization, L.Y.; Supervision, Y.F.; Project administration, Y.F.; Funding acquisition, Y.F. and L.Y. All authors have read and agreed to the published version of the manuscript.
Supplemental Material
Download MS Word (9.3 MB)Acknowledgments
The authors express their gratitude to the participants and investigators of the FinnGen study. The authors thank all investigators for sharing this data.
Disclosure statement
No potential conflict of interest was reported by the author(s).
Additional information
Funding
References
- Lim EB, Oh HS, Kim KC, et al. Identification and functional validation of HLA-C as a potential gene involved in colorectal cancer in the Korean population. BMC Genomics. 2022;23(1):261. doi: 10.1186/s12864-022-08509-5.
- Gajewski TF, Schreiber H, Fu YX. Innate and adaptive immune cells in the tumor microenvironment. Nat Immunol. 2013;14(10):1014–1022. doi: 10.1038/ni.2703.
- Askoura M, Abbas HA, Al Sadoun H, et al. Elevated levels of IL-33, IL-17 and IL-25 indicate the progression from chronicity to hepatocellular carcinoma in hepatitis C virus patients. Pathogens. 2022;11(1):57. doi: 10.3390/pathogens11010057.
- Braumüller H, Mauerer B, Andris J, et al. The cytokine network in colorectal cancer: implications for new treatment strategies. Cells. 2022;12(1):138. doi: 10.3390/cells12010138.
- Leonard WJ, Lin J-X, O’Shea JJ. The γc family of cytokines: basic biology to therapeutic ramifications. Immunity. 2019;50(4):832–850. doi: 10.1016/j.immuni.2019.03.028.
- Zhu X, Liang R, Lan T. Tumor-associated macrophage-specific CD155 contributes to M2-phenotype transition, immunosuppression, and tumor progression in colorectal cancer. J ImmunoTher Cancer. 2022;10(9):e004219. doi: 10.1136/jitc-2021-004219.
- Sun Q, Shang Y, Sun F, et al. Interleukin-6 promotes epithelial-mesenchymal transition and cell invasion through integrin β6 upregulation in colorectal cancer. Oxid Med Cell Longev. 2020;2020:8032187. doi: 10.1155/2020/8032187.
- Ye D, Liu H, Zhao G, et al. LncGMDS-AS1 promotes the tumorigenesis of colorectal cancer through HuR-STAT3/Wnt axis. Cell Death Dis. 2023;14(2):165. doi: 10.1038/s41419-023-05700-8.
- Albrecht HC, Gustavus D, Schwanemann J, et al. Generation of colon cancer-derived tumor-infiltrating T cells (TILs) for adoptive cell therapy. Cytotherapy. 2023;25(5):537–547. doi: 10.1016/j.jcyt.2023.01.009.
- Acevedo-León D, Gómez-Abril SÁ, Sanz-García P, et al. The role of oxidative stress, tumor and inflammatory markers in colorectal cancer patients: a one-year follow-up study. Redox Biol. 2023;62:102662. doi: 10.1016/j.redox.2023.102662.
- Shibabaw T, Teferi B, Ayelign B. The role of Th-17 cells and IL-17 in the metastatic spread of breast cancer: as a means of prognosis and therapeutic target. Front Immunol. 2023;14:1094823. doi: 10.3389/fimmu.2023.1094823.
- Alzahrani FA, Ahmed F, Sharma M, et al. Investigating the pathogenic SNPs in BLM helicase and their biological consequences by computational approach. Sci Rep. 2020;10(1):12377. doi: 10.1038/s41598-020-69033-8.
- Schaub MA, Kaplow IM, Sirota M, et al. A classifier-based approach to identify genetic similarities between diseases. Bioinformatics. 2009;25(12):i21–i29. doi: 10.1093/bioinformatics/btp226.
- Liang YY, Chen J, Peng M, et al. Association between sleep duration and metabolic syndrome: linear and nonlinear mendelian randomization analyses. J Transl Med. 2023;21(1):90. doi: 10.1186/s12967-023-03920-2.
- Li J, Li C, Huang Y, et al. Mendelian randomization analyses in ocular disease: a powerful approach to causal inference with human genetic data. J Transl Med. 2022;20(1):621. doi: 10.1186/s12967-022-03822-9.
- Folkersen L, Gustafsson S, Wang Q, et al. Genomic and drug target evaluation of 90 cardiovascular proteins in 30,931 individuals. Nat Metab. 2020;2(10):1135–1148. doi: 10.1038/s42255-020-00287-2.
- Zhao H, Jin X. Causal associations between dietary antioxidant vitamin intake and lung cancer: a Mendelian randomization study. Front Nutr. 2022;9:965911. doi: 10.3389/fnut.2022.965911.
- Lim AJW, Tyniana CT, Lim LJ, et al. Robust SNP-based prediction of rheumatoid arthritis through machine-learning-optimized polygenic risk score. J Transl Med. 21;92.
- Bou Sleiman M, Jha P, Houtkooper R, et al. The gene-regulatory footprint of aging highlights conserved central regulators. Cell Rep. 2020;32(13):108203. doi: 10.1016/j.celrep.2020.108203.
- Von Essen MR, Søndergaard HB, Petersen ERS, et al. IL-6, IL-12, and IL-23 STAT-Pathway genetic risk and responsiveness of lymphocytes in patients with multiple sclerosis. Cells. 2019;8(3):285. doi: 10.3390/cells8030285.
- Kühnapfel A, Horn K, Klotz U, et al. Genetic regulation of cytokine response in patients with acute community-acquired pneumonia. Genes. 2022;13(1):111. doi: 10.3390/genes13010111.
- Wang QJ, Shen YE, Wang X, et al. Concomitant memantine and Lactobacillus plantarum treatment attenuates cognitive impairments in APP/PS1 mice. Aging. 2020;12(1):628–649.
- Ye X, Li J, Cheng Y, et al. Genome-wide association study of resistance to stripe rust (Puccinia striiformis f. sp. tritici) in Sichuan wheat. BMC Plant Biol. 2019;19(1):147. doi: 10.1186/s12870-019-1764-4.
- Zhuang H, Han J, Cheng L, et al. A positive causal influence of IL-18 levels on the risk of T2DM: a Mendelian randomization study. Front Genet. 2019;10:295.
- Li BH, Yan SY, Luo LS, et al. Ten interleukins and risk of prostate cancer. Front Oncol. 2023;13:1108633. doi: 10.3389/fonc.2023.1108633.
- Yi M, Zhao W, Fei Q, et al. Causal analysis between altered levels of interleukins and obstructive sleep apnea. Front Immunol. 2022;13:888644. doi: 10.3389/fimmu.2022.888644.
- Yuan S, Li X, Lin A, et al. Interleukins and rheumatoid arthritis: bi-directional Mendelian randomization investigation. Semin Arthritis Rheum. 2022;53:151958. doi: 10.1016/j.semarthrit.2022.151958.
- Lu H, Wu PF, Zhang W, et al. Circulating interleukins and risk of multiple sclerosis: a Mendelian randomization study. Front Immunol. 2021;12:647588. doi: 10.3389/fimmu.2021.647588.
- Yuan S, Lin A, He QQ, et al. Circulating interleukins in relation to coronary artery disease, atrial fibrillation and ischemic stroke and its subtypes: a two-sample Mendelian randomization study. Int J Cardiol. 2020;313:99–104. doi: 10.1016/j.ijcard.2020.03.053.
- Cui G, Liu H, Laugsand JB. Endothelial cells-directed angiogenesis in colorectal cancer: interleukin as the mediator and pharmacological target. Int Immunopharmacol. 2023;114:109525. doi: 10.1016/j.intimp.2022.109525.
- Li W, Chen F, Gao H, et al. Cytokine concentration in peripheral blood of patients with colorectal cancer. Front Immunol. 2023;14:1175513. doi: 10.3389/fimmu.2023.1175513.
- Liu QQ, Li CM, Fu LN, et al. Enterotoxigenic Bacteroides fragilis induces the stemness in colorectal cancer via upregulating histone demethylase JMJD2B. Gut Microbes. 2020;12(1):1788900. doi: 10.1080/19490976.2020.1788900.
- Trimaglio G, Tilkin-Mariamé AF, Feliu V, et al. Colon-specific immune microenvironment regulates cancer progression versus rejection. Oncoimmunology. 2020;9(1):1790125. doi: 10.1080/2162402X.2020.1790125.
- Huang C, Yang G, Jiang T, et al. The effects and mechanisms of blockage of STAT3 signaling pathway on IL-6 inducing EMT in human pancreatic cancer cells in vitro. Neoplasma. 2011;58(5):396–405. doi: 10.4149/neo_2011_05_396.
- Kim B, Seo Y, Kwon JH, et al. IL-6 and IL-8, secreted by myofibroblasts in the tumor microenvironment, activate HES1 to expand the cancer stem cell population in early colorectal tumor. Mol Carcinog. 2021;60(3):188–200. doi: 10.1002/mc.23283.
- Liu Y, Han Z P, Zhang S S, et al. Effects of inflammatory factors on mesenchymal stem cells and their role in the promotion of tumor angiogenesis in colon cancer. J Biol Chem. 2011;286(28):25007–25015. doi: 10.1074/jbc.M110.213108.
- Xu J, Qian J, Zhang W, et al. LYPD8 regulates the proliferation and migration of colorectal cancer cells through inhibiting the secretion of IL‑6 and TNF‑α. Oncol Rep. 2019;41(4):2389–2395. doi: 10.3892/or.2019.7034.
- Waldmann TA. The biology of interleukin-2 and interleukin-15: implications for cancer therapy and vaccine design. Nat Rev Immunol. 2006;6(8):595–601. doi: 10.1038/nri1901.
- Taylor ES, McCall JL, Shen S, et al. Prognostic roles for IL-2-producing and CD69+ T cell subsets in colorectal cancer patients. Int J Cancer. 2018;143(8):2008–2016.
- Masters SL, Dunne A, Subramanian SL, et al. Activation of the NLRP3 inflammasome by islet amyloid polypeptide provides a mechanism for enhanced IL-1β in type 2 diabetes. Nat Immunol. 2010; 11(10):897–904.
- Chen Y, Yang Z, Deng B, et al. Interleukin 1β/1RA axis in colorectal cancer regulates tumor invasion, proliferation and apoptosis via autophagy. Oncol Rep. 2020;43(3):908–918. doi: 10.3892/or.2020.7475.
- Xie Q, Shen WW, Zhong J, et al. Lipopolysaccharide/adenosine triphosphate induces IL‑1β and IL-18 secretion through the NLRP3 inflammasome in RAW264.7 murine macrophage cells. Int J Mol Med. 2014;34(1):341–349. doi: 10.3892/ijmm.2014.1755.
- Feng X, Zhang Z, Sun P, et al. Interleukin-18 is a prognostic marker and plays a tumor suppressive role in colon cancer. Dis Markers. 2020;2020:6439614. doi: 10.1155/2020/6439614.
- Chen KQ, Li D Z, Chen Z B, et al. Quanzhenyiqitang reverses LPS-Induced inflammation via inhibiting PYK2/p38MAPK/HDAC2/CK2 signaling pathway in rat alveolar macrophage. Evid Based Complement Alternat Med. 2022;2022:7857022. doi: 10.1155/2022/7857022.
- Ackermann A, Lafferton B, Plotz G, et al. Expression and secretion of the pro‑inflammatory cytokine IL‑8 is increased in colorectal cancer cells following the knockdown of non‑erythroid spectrin αII. Int J Oncol. 2020;56(6):1551–1564. doi: 10.3892/ijo.2020.5026.
- Van Damme J, Van Beeumen J, Opdenakker G, et al. A novel, NH2-terminal sequence-characterized human monokine possessing neutrophil chemotactic, skin-reactive, and granulocytosis-promoting activity. J Exp Med. 1988;167(4):1364–1376. doi: 10.1084/jem.167.4.1364.
- Baggiolini M, Walz A, Kunkel SL. Neutrophil-activating peptide-1/interleukin 8, a novel cytokine that activates neutrophils. J Clin Invest. 1989;84(4):1045–1049. doi: 10.1172/JCI114265.
- D’Agostino G, García-Cuesta EM, Gomariz RP, et al. The multilayered complexity of the chemokine receptor system. Biochem Biophys Res Commun. 2020;528(2):347–358. doi: 10.1016/j.bbrc.2020.02.120.
- Olivera I, Sanz-Pamplona R, Bolaños E, et al. A therapeutically actionable protumoral axis of cytokines involving IL-8, TNFα, and IL-1β. Cancer Discov. 2022;12(9):2140–2157. doi: 10.1158/2159-8290.CD-21-1115.
- Teijeira A, Garasa S, Ochoa MC, et al. IL8, neutrophils, and NETs in a collusion against cancer immunity and immunotherapy. Clin Cancer Res. 2021;27(9):2383–2393. doi: 10.1158/1078-0432.CCR-20-1319.
- Waugh DJJ, Wilson C. The interleukin-8 pathway in cancer. Clin Cancer Res. 2008;14(21):6735–6741. doi: 10.1158/1078-0432.CCR-07-4843.
- Alfaro C, Sanmamed MF, Rodríguez-Ruiz ME, et al. Interleukin-8 in cancer pathogenesis, treatment and follow-up. Cancer Treat Rev. 2017;60:24–31. doi: 10.1016/j.ctrv.2017.08.004.