Abstract
Background and Aims
There is no golden standard for the diagnosis of autoimmune hepatitis which still dependent on liver biopsy currently. So, we developed a noninvasive prediction model to help optimize the diagnosis of autoimmune hepatitis.
Methods
From January 2017 to December 2019, 1739 patients who had undergone liver biopsy were seen in the second hospital of Nanjing, of which 128 were here for consultation. Clinical, laboratory, and histologic data were obtained retrospectively. Multivariable logistic regression analysis was employed to create a nomogram model that predicting the risk of autoimmune hepatitis. Internal and external validation was both performed to evaluate the model.
Results
A total of 1288 patients with liver biopsy were enrolled (1184 from the second hospital of Nanjing, the remaining 104 from other centers). After the univariate and multivariate logistic regression analysis, nine variables including ALT, IgG, ALP/AST, ALB, ANA, AMA, HBsAg, age, and gender were selected to establish the noninvasive prediction model. The nomogram model exhibits good prediction in diagnosing autoimmune hepatitis with AUROC of 0.967 (95% CI: 0.776–0.891) in internal validation and 0.835 (95% CI: 0.752–0.919) in external validation.
Conclusions
ALT, IgG, ALP/AST, ALB, ANA, AMA, HBsAg, age, and gender are predictive factors for the diagnosis of autoimmune hepatitis in patients with unexplained liver diseases. The predictive nomogram model built by the nine predictors achieved good prediction for diagnosing autoimmune hepatitis.
Introduction
The incidence of autoimmune hepatitis (AIH) has been increasing, and it has now become the most common cause of noninfective liver disease. According to a Chinese study [Citation1], AIH accounted for 19.1% of patients with unknown liver disease who underwent liver biopsy, which became a major component of unidentified unknown liver disease. The onset of AIH may be hidden or acute, and even progress to cirrhosis at diagnosis, which can eventually progress to liver failure or liver cancer. It is therefore important to diagnose and treat AIH as early as possible. At present, the etiology and pathogenesis of AIH remain unclear, and it is believed to be associated with a combination of genetic susceptibility and environmental factors. T cells and B cells in the immune system are thought to play a crucial role in the development of AIH, leading to liver tissue damage and inflammatory responses.
The International Autoimmune Hepatitis Group (IAIHG) proposed the descriptive diagnostic scoring system in 1993 [Citation2] and later released the revised original criteria in 1999 [Citation3], as well as a simplified version in 2008 [Citation4]. Currently, the latter two criteria are the commonly used diagnostic standards for AIH. A multicenter study [Citation5] involving 405 patients was conducted in China. In which, the simplified criteria demonstrated a sensitivity of 90% and specificity of 95% for the diagnosis of probable AIH. This compares well with the revised original criteria, which had sensitivity and specificity of 100% and 93%, respectively, for probable AIH. For definite AIH, the simplified criteria exhibited a sensitivity of 62% and specificity of 99%, whereas the revised original criteria had a sensitivity of 64% and specificity of 100%. The diagnosis of AIH in this study was based on the descriptive scoring system, so both the revised criteria and the simplified criteria for AIH have yielded promising outcomes. Due to its complexity, the revised criteria are not widely used in clinical practice. Then, the simplified criteria have become the preferred scoring system in clinic. However, it was found [Citation5] that some atypical patients, such as patients with low titers of autoantibodies or low serum immunoglobulins were easily missed by the simplified score. Currently, there are no clinically specific biomarkers [Citation6,Citation7] available for the reliable diagnosis of AIH. And, existing diagnostic criteria still heavily depend on the histologic evaluation obtained through liver biopsy. However, liver biopsy is an invasive diagnostic examination with its risks including infection, pain, and bleeding [Citation8,Citation9]. Currently, several noninvasive models have been developed to predict the degree of liver inflammation, fibrosis or cirrhosis in AIH [Citation10–12]. The number of cases included in these studies is limited, and the accuracy of these models can still be further improved. Thus, the objective of this study was to develop an optimal diagnostic model using noninvasive clinical variables for predicting the risk of AIH in patients.
Materials and methods
We followed the TRIPOD for Abstracts (Transparent Reporting of Multivariable Prediction Models in Journal and Conference Abstracts) statement for reporting on the development and validation of the multivariate prediction model [Citation13,Citation14].
Patient population
Between January 2017 and December 2019 at the Second Hospital of Nanjing (Nanjing, China), patients with liver diseases who had undergone liver biopsy were seen. Inclusion criteria included the following: (1) undergone liver biopsy; (2) confirmed cases with diagnosis of liver diseases. Exclusion criteria consisted of the following: (1) incomplete clinical data; (2) incomplete laboratory data. And then a total of 1184 individuals who had complete clinical and laboratory data were enrolled in this retrospective study. The 1184 patients were divided into two cohorts randomly, 70% (n = 829) for serving as a model derivation group and the remaining 30% (n = 355) for internal validation group [Citation15]. We set up the external validation cohort from the patients (n = 104) from other hospitals who came for consultation. This study was approved by the ethics committee at the Second Hospital of Nanjing. Of the 1288 (1184 + 104) patients, 235 (191 + 44) individuals were diagnosed with AIH based on histopathology and clinical features, and the others (n = 1053) composed of primary biliary cirrhosis (PBC), AIH-PBC overlap syndrome, drug induced liver injury, nonalcoholic fatty liver disease, nonalcoholic steatohepatitis(NASH), chronic hepatitis B, and so on. The enrolled patients including the AIH and the not AIH were divided into two cohorts: the training group and the validation cohort ().
Data collection
Data on the patients’ clinical variables, such as age, gender and the time of first visit, were retrospectively collected. Laboratory test data at diagnosis were collected including serum biochemistry (TBIL, DBIL, ALB, GLO, ALT, AST, γ-GT, ALP, LDH, and TBA), blood coagulation function test (PT, INR, and FIB), serum immunoglobulins (IgA, IgG, and IgM), viral hepatitis serum markers (HBsAg, HBV DNA, HCV-Ab, and HCV RNA), routine blood test (RBC, WBC, Hb, and PLT), and serum autoantibodies including autoimmune antibodies containing antinuclear antibodies (ANA), smooth muscle antibodies (SMA), antimitochondrial antibodies (AMA), antibodies against AMA-M2, liver kidney microsomes type1 (LKM-1), and soluble liver antigen/liver-pancreas antigen (SLA/LP).
In this study, liver biopsies were performed on all patients. The liver tissues were fixed in formalin, embedded in paraffin, and then sectioned and deparaffinized. After staining with hematoxylin-eosin, the liver tissue specimens were examined and recorded by two experienced pathologists and one liver specialist who had expertise in liver pathology. Liver histology was evaluated using the Scheuer scoring system to assess inflammation and fibrosis, while the degree of steatosis was assessed using the NAS scoring system.
Statistical analyses
Descriptive statistics were applied to all variables, which displayed in form of mean, standard deviation, percentile for continuous variables while the frequency and percentage for categorical factors. Univariate analysis was performed to evaluate the differences between AIH and Not AIH, student’s t-test or Wilcoxon rank sum test were used to compare continuous variables, while for classified variables chi-square test was used. A P-value threshold of <0.1 was used in univariate analysis for inclusion of suspicious predictive factors into the multivariate logistic regression analysis in which P-value <0.05 was used. Using the RMS software package of R software, the nomogram model was established according to the factors enrolled in the multivariate logistic regression analysis. The internal and external validation on performance of AIH diagnosis model were based on discrimination and calibration [Citation16,Citation17]. The discrimination of the nomogram model was verified by the receiver operating characteristic curve (ROC curve) while the accuracy verified by the calibration curve (a 45° curve represents a flawless prediction). A P-value <0.05 was considered statistically significant. All analyses were operated using SPSS (version 26, IBM, America) and R (version 2.13.1, The R Foundation for Statistical Computing, Vienna, Austria; packages used: Design and ROCR).
Results
Demographic and clinical characteristics
In the Second Hospital of Nanjing, a total of 1184 patients with complete clinical and laboratory data were enrolled in this retrospective study, which were all underwent liver biopsy. Of the total subjects, 58.8% were female and 41.2% were male. The mean age at first time of visit was 48(38 ∼ 55) years old. 191 subjects (16.1% of all subjects) had biopsy-proven AIH, and 993 subjects (83.87% of all subjects) were without AIH.
describes the clinical and laboratory characteristics of the patients. There is a higher proportion of female in the AIH group. The levels of TB, DB, GLO, ALT, AST, GGT, LDH, TBA, PT, INR, IgG, and ANA were significantly higher in AIH subjects versus not-AIH. However, values of RBC, Hb, PLT, ALB, FIB, AMA, AMA M2, and HBsAg were found lower in the AIH group.
Table 1. Demographic and clinical characteristics of patients.
Histological features
summarizes the histological characteristics of the patients. As expected, individuals with AIH had a higher percentage of interface hepatitis, lymphocyte/plasma cell predominance, rosettes, and emperipolesis, which were the characteristic histological features of AIH. The incidence of lymphocyte/plasma cell predominance in AIH group was significantly higher than that in not-AIH group, which were 74.87% and 22.76%, respectively (P < 0.001). Rosettes was present in 50.79% of patients with AIH as compared to 4.93% of patients without AIH. The presence of interface hepatitis was significantly higher in patients with AIH as compared to those without NASH: 96.86% vs. 24.2%. Emperipolesis was seen in 2.09% of AIH patients which was much lower than the actual incidence, while none in not-AIH group.
Table 2. Histological features of patients.
Logistic regression analysis
To determine the predictors for diagnosis of AIH in patients with liver diseases, 23 variables related with AIH were included in the univariate statistical analysis that revealed the following factors were potential predictors: ALT, IgG, ALP/AST, AST, GLO, ALB, DB, TB, TBA, LDH, IgA, INR, PT, FIB, ANA, AMA, SMA, HBsAg, age, and gender (). Then, these factors were enrolled in the multivariate analysis, and we narrowed down to the seven independent predictors including IgG (adjusted OR (AOR): 2.08, 95% CI: 1.03–4.23), ALP/AST (AOR: 0.19, 95% CI: 0.11–0.34), HBsAg (AOR: 0.31, 95% CI: 0.12–0.69), AMA (AOR: 0.22, 95% CI: 0.10–0.43), ALB (AOR: 0.92, 95% CI: 0.88–0.96), age (AOR: 1.03, 95% CI: 1.02–1.05), and female (AOR: 3.26, 95% CI: 2.06–5.27). According to the results, IgG, age, and female were risk parameters, while ALP/AST, HBsAg, AMA, and ALB were protective parameters.
Table 3. Predictors of autoimmune hepatitis diagnosis determined by univariate and multivariate logistic regression analysis.
Nomogram model
According to statistics, ALT and ANA are not related to the prediction of AIH, but considering their clinical role in the diagnosis of AIH [Citation18, Citation19], we incorporate both them into the nomogram model. Eventually, the model for the prediction of AIH was built by nine clinical variables consist of ALT, IgG, ALP/AST, ALB, ANA, AMA, HBsAg, age, and gender.
According to the values of clinical and laboratory indexes, the nomogram model is applied by locating the position on the scale of each prediction variable. Each scale position corresponds to a prognostic point (top axis). Determine the point value of each prediction variable and add it to calculate the total point value. Then locate this value in the bottom axis and draw a straight line there to determine the probability of diagnosing AIH () [Citation15,Citation20].
Figure 2. Nomogram model for predicting the presence of autoimmune hepatitis in patients with liver diseases.
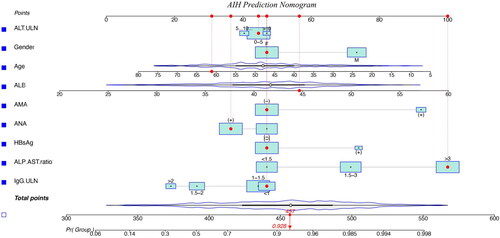
The prediction model was internally evaluated by means of bootstrapping [Citation21]. In internal validation, the ROC curve () was constructed that demonstrates good discrimination with AUROC of 0.834 (95% CI: 0.776–0.891). This means that the model is correct 83.4% of the time in diagnosing AIH among patients with liver diseases. Internal validation revealed that a nomogram cut-off value of 0.153 probability would give a 79.1% specificity and 78.9% sensitivity of having AIH, with a 42.1% positive predictive value and 95.1% negative predictive value. In external validation, the ROC curve () was constructed that demonstrates good discrimination with AUROC of 0.835 (95% CI: 0.752–0.919). The external validation revealed that a nomogram cut-off value of 0.128 probability would give a 75.0% specificity and 84.1% sensitivity of having AIH, with a 71.2% positive predictive value and 86.5% negative predictive value. Therefore, a nomogram risk score of <0.153 would be reasonable to exclude AIH.
Figure 3. The receiver operating characteristic curve estimating the accuracy of the autoimmune hepatitis predictive model. AUROC, area under the receiver operating characteristics curve.
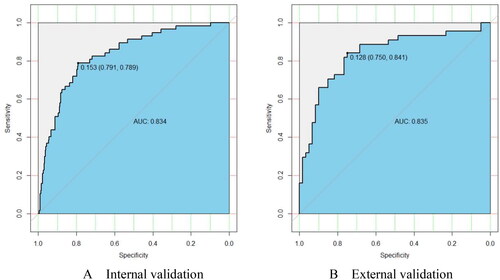
By utilizing this nomogram with the above cut-off values, more than 50% of patients can theoretically avoid liver biopsy. In addition, the calibration curve () shows that there is a good consistency between predicted and observed probabilities in both internal () and external () verification.
Validation of the simplified criteria
The clinical diagnosis based on pathology served as the gold standard of diagnosis for AIH. The sensitivity and specificity of the simplified criteria for probable AIH (≥6) are 54.5% and 80.8%, and 28.3% and 89.7% for definite AIH (≥7), respectively. The predictability of the simplified criteria is 76.4% for probable AIH 79.8% for definite AIH. The positive predictive value of the simplified criteria is 35% for probable AIH and 34.6% for definite AIH. The negative predictive value is 90.2% for probable AIH and 86.7% for definite AIH ().
Table 4. Diagnostic parameters of the simplified criteria for diagnosis of autoimmune hepatitis.
In the reports of Hennes et al. AIH–PBC overlap syndromes were considered as AIH in the calculation of the sensitivity and specificity of both criteria while only AIH was considered in this study. Meanwhile, emperipolesis was found in only four patients with AIH in this retrospective study, but in fact, the incidence of emperipolesis in AIH was much higher [Citation22], which may lead to low simplified scores in parts of patients. Emperipolesis is difficult to be found in histopathology, so in most studies, the incidence of emperipolesis is very low. The results of a multicenter study in the UK [Citation23] showed that emperipolesis was found in only 0.4% of AIH patients. In view of these, the diagnostic efficacy of the simplified criteria in this study is slightly lower than others [Citation4,Citation5].
Discussion
The pathogenesis of AIH has not yet been elucidated, and the clinical manifestations lack specificity [Citation24,Citation25]. Currently, there are no clinically specific biomarkers to reliably diagnose AIH, an invasive liver biopsy is the only reliable way to identify the degree of liver damage and the diagnosis [Citation26]. However, liver puncture has certain technical requirements for doctors that has not yet been popularized in hospitals even tertiary centers [Citation27]. Even if the liver puncture technique can be realized, the interpretation of pathology sections is so difficult [Citation28]. Pathologists are required to be proficient in clinical knowledge, the combination of the two can make a more accurate diagnosis [Citation29]. Only a few clinical pathologists have reached the stage to diagnose AIH. Many patients with AIH were delayed in treatment due to misdiagnosis and missed diagnosis, resulting in the progress of the disease [Citation25]. Parts of AIH patients have progressed to the stage of liver cirrhosis or even liver failure at diagnosis [Citation23]. Early diagnosis and treatment are so meaningful to prevent the progression of the disease and prolong the life of the patients [Citation30,Citation31]. However, it is very difficult to popularize liver biopsy in a short time which is an invasive procedure that may be accompanied by complications including infection, pain, and bleeding. So, we would establish a noninvasive prediction model by common clinical and laboratory indexes, which can minimize invasive operations possibly.
First, we screened the variables that may be related to the diagnosis of AIH through the revised scoring system and the simplified criteria. The main contents of the revised descriptive criteria consisted of the following: 7 points of clinical features, 14 points of serological features, 5 points of histopathological manifestations and 3 points of the response to immunosuppressive therapy. The simplified criteria mainly included four parts: serum autoantibodies, IgG, liver histology and absence of viral hepatitis. Then, we determined the factors enrolled to establish the nomogram model by univariate and multivariable logistic regression analysis, including ALT, IgG, ALP/AST, ALB, ANA, AMA, HBsAg, age and gender. The other 30% of the patients from the second hospital of Nanjing served as the internal verification group with AUROC of 0.834 (95% CI: 0.776–0.891), which revealed that a nomogram cut-off value of 0.153 probability would give a 79.1% specificity and 78.9% sensitivity of having AIH. The patients came for consultation served as the external verification group with AUROC of 0.835 (95% CI: 0.752–0.919), showed that a nomogram cut-off value of 0.128 probability would give a 75.0% specificity and 84.1% sensitivity of having AIH.
According to the results of univariate and multivariate analyses, IgG, age, and female gender were identified as risk factors, while ALP/AST, AMA, HBsAg, and ALB were protective factors. In the revised original criteria, female, elevated IgG, ANA(+), ALP/AST <1.5, and negative HBsAg are considered scoring criteria, while ALP/AST > 3, AMA(+), and HBsAg(+) are considered negative criteria. In the simplified criteria, ANA(+), elevated IgG, and negative HBsAg are scoring criteria. Overall, the results of this study are consistent with the clinical characteristics. IgG serves as an important indicator of AIH disease activity and the diagnosis of AIH. The prevalence of AIH is higher in females, with a male-to-female ratio of ∼1:4-5, making female gender an independent risk factor for AIH. ALT and AST reflect the degree of liver cell injury, while ALP often indicates bile stasis. If the ALP/AST ratio is elevated, it suggests predominantly cholestatic injury, and if the ratio decreases, it indicates predominantly hepatocellular injury. AMA (+) is a specific marker for PBC. As for ALB, although it may not have significant clinical significance in AIH, it showed notable statistical differences in the research results. This may be related to globulin (GLO). AIH can often lead to elevated GLO, reflecting immune system activity. The lower the proportion of ALB, the higher the proportion of GLO, indicating a higher likelihood of AIH. However, this hypothesis requires further research for validation.
Compared with the simplified criteria, which give an 80.8% specificity and 54.5% sensitivity for probable AIH and an 89.7% specificity and 28.3% sensitivity for definite AIH, the nomogram model shows certain advantages. Moreover, the simplified criteria need invasive liver biopsy while the prediction model is a non-invasive diagnostic model.
This study has several advantages. First, according to the current literature, this is the first study to develop a nomogram model to predict the risk of AIH in patients with unexplained liver disease [Citation32,Citation33]. Then, the technology of liver puncture and pathological diagnosis in our hospital is mature, and it is in a leading position in China. A large group of over 1000 patients who underwent liver biopsy in our hospital from 2017 to 2019 were included, and their clinical, laboratory and histological data were widely described and scored with simplified criteria. Moreover, after more than two years of follow-up, these patients confirmed the diagnosis of AIH. Few units can finish that due to the extensive liver biopsies. In addition, the multicenter study is more reliable. We have established an external verification cohort through consultation with patients. And there are only nine indicators which all common clinical indicators included in the model. Finally, the internal and external verification of this non-invasive prediction model shows good diagnostic ability, which has a great advantage over the simplified criteria that requires invasive liver biopsy.
However, this study does have some limitations. We only observed 2017 to 2019 of patients, and then we will continue to include patients in the past three years to expand the observation cohort, which can further optimize the model. In this retrospective cohort, AIH accounts for only about 16% of the patients we included, which will reduce the predictability and positive predictive value of the prediction model. This study is aimed at Chinese patients, so it is not clear whether this nomogram model is applicable to other races. Future studies need to assess the suitability of this model in a more diverse patient population.
The central discovery of this retrospective study revolves around the utilization of a nomogram to anticipate the presence of AIH among patients with unexplained liver conditions. This approach effectively employs routine clinical parameters that are readily accessible to a broad range of medical practitioners, extending beyond larger medical centers to primary healthcare facilities as well. As a result, this model could be seamlessly integrated into the diagnostic practice of primary hospitals. However, it’s important to note that this model should undergo further refinement and validation within a more diverse patient population in the future. If proven robust, this predictive model has the potential to serve as a non-invasive tool for diagnosing AIH, enabling early intervention and treatment. Subsequently, we will create of an online prediction tool that can be easily accessed by the general public.
Disclosure statement
No potential conflict of interest was reported by the author(s).
Additional information
Funding
References
- Liu HL, Yang YF, Xiong QF, et al. Etiological analysis of hepatopathy of unknown etiology in 470 cases undergoing routine liver biopsy examination. Zhonghua Gan Zang Bing Za Zhi. 2019;27:885–889.
- Johnson PJ, McFarlane IG. Meeting report: international autoimmune hepatitis group. Hepatology 1993;18(4):998–1005. doi: 10.1002/hep.1840180435.
- Alvarez F, Berg PA, Bianchi FB, et al. International autoimmune hepatitis group report: review of criteria for diagnosis of autoimmune hepatitis. J Hepatol. 1999;31(5):929–938. doi: 10.1016/s0168-8278(99)80297-9.
- Hennes EM, Zeniya M, Czaja AJ, et al. Simplified criteria for the diagnosis of autoimmune hepatitis. Hepatology 2008;48(1):169–176. doi: 10.1002/hep.22322.
- Qiu D, Wang Q, Wang H, et al. Validation of the simplified criteria for diagnosis of autoimmune hepatitis in Chinese patients. J Hepatol. 2011;54(2):340–347. doi: 10.1016/j.jhep.2010.06.032.
- Mack CL, Adams D, Assis DN, et al. Diagnosis and management of autoimmune hepatitis in adults and children: 2019 practice guidance and guidelines from the American association for the study of liver diseases. Hepatology 2020;72(2):671–722. doi: 10.1002/hep.31065.
- Harrington C, Krishnan S, Mack CL, et al. Noninvasive biomarkers for the diagnosis and management of autoimmune hepatitis. Hepatology 2022;76(6):1862–1879. doi: 10.1002/hep.32591.
- Sripongpun P, Pongpaibul A, Charatcharoenwitthaya P. Value and risk of percutaneous liver biopsy in patients with cirrhosis and clinical suspicion of autoimmune hepatitis. BMJ Open Gastroenterol. 2021;8(1):e000701. doi: 10.1136/bmjgast-2021-000701.
- Caldwell S, Northup PG. Bleeding complication with liver biopsy: is it predictable? Clin Gastroenterol Hepatol. 2010;8(10):826–829. doi: 10.1016/j.cgh.2010.06.010.
- Zhang Z, Wang J, Wang H, et al. Develop and validate a novel online AIHI-nomogram to predict severe liver inflammation in patients with autoimmune hepatitis. Ann Hepatol. 2023;28(6):101134. doi: 10.1016/j.aohep.2023.101134.
- Zhang Z, Wang J, Wang H, et al. An easy-to-use AIHF-nomogram to predict advanced liver fibrosis in patients with autoimmune hepatitis. Front Immunol. 2023;14:1130362. doi: 10.3389/fimmu.2023.1130362.
- Llovet LP, Gratacós-Ginès J, Téllez L, et al. Noninvasive prediction of outcomes in autoimmune hepatitis-related cirrhosis. Hepatol Commun. 2022;6(6):1392–1402. doi: 10.1002/hep4.1889.
- Heus P, Reitsma JB, Collins GS, et al. Transparent reporting of multivariable prediction models in journal and conference abstracts: TRIPOD for abstracts. Ann Intern Med. 2020;173:42–47. doi: 10.7326/M20-0193.
- Snell KIE, Levis B, Damen JAA, et al. Transparent reporting of a multivariable prediction model for individual prognosis or diagnosis (TRIPOD): the TRIPOD statement. BMJ. 2023;381:e073538. doi: 10.1136/bmj.g7594.
- James MT, Pannu N, Hemmelgarn BR, et al. Derivation and external validation of prediction models for advanced chronic kidney disease following acute kidney injury. JAMA 2017;318(18):1787–1797. doi: 10.1001/jama.2017.16326.
- Fenlon C, O’Grady L, Doherty ML, et al. A discussion of calibration techniques for evaluating binary and categorical predictive models. Prev Vet Med. 2018;149:107–114. doi: 10.1016/j.prevetmed.2017.11.018.
- Jacobs JP, Shahian DM, O’Brien SM, et al. Assessing the performance of risk models with discrimination and calibration. Ann Thorac Surg. 2023;115(1):282–286. doi: 10.1016/j.athoracsur.2022.05.032.
- Kwo PY, Cohen SM, Lim JK. ACG clinical guideline: evaluation of abnormal liver chemistries. Am J Gastroenterol. 2017;112(1):18–35. doi: 10.1038/ajg.2016.517.
- Sebode M, Weiler-Normann C, Liwinski T, et al. Autoantibodies in autoimmune liver disease—clinical and diagnostic relevance. Front Immunol. 2018;9:609. doi: 10.3389/fimmu.2018.00609.
- Eng K, Lopez R, Liccardo D, et al. A non-invasive prediction model for non-alcoholic steatohepatitis in paediatric patients with non-alcoholic fatty liver disease. Dig Liver Dis. 2014;46(11):1008–1013. doi: 10.1016/j.dld.2014.07.016.
- Harrell FE, Jr., Califf RM, Pryor DB, et al. Evaluating the yield of medical tests. JAMA 1982;247(18):2543–2546. doi: 10.1001/jama.1982.03320430047030.
- de Boer YS, van Nieuwkerk CM, Witte BI, et al. Assessment of the histopathological key features in autoimmune hepatitis. Histopathology. 2015;66(3):351–362. doi: 10.1111/his.12558.
- Gordon V, Adhikary R, Appleby V, et al. Diagnosis, presentation and initial severity of autoimmune hepatitis (AIH) in patients attending 28 hospitals in the UK. Liver Int. 2018;38(9):1686–1695. doi: 10.1111/liv.13724.
- Sebode M, Hartl J, Vergani D, et al. Autoimmune hepatitis: from current knowledge and clinical practice to future research agenda. Liver Int. 2018;38(1):15–22. doi: 10.1111/liv.13458.
- Mieli-Vergani G, Vergani D, Czaja AJ, et al. Autoimmune hepatitis. Nat Rev Dis Primers. 2018;4:18017. doi: 10.1038/nrdp.2018.17.
- Lenti MV, Rossi CM, Melazzini F, et al. Seronegative autoimmune diseases: a challenging diagnosis. Autoimmun Rev. 2022;21(9):103143. doi: 10.1016/j.autrev.2022.103143.
- Rockey DC, Caldwell SH, Goodman ZD, et al. Liver biopsy. Hepatology. 2009;49(3):1017–1044. doi: 10.1002/hep.22742.
- Mieli-Vergani G, Zen Y, Vergani D. Reassessement of the histological features of autoimmune hepatitis. Liver Int. 2022;42(5):954–956. doi: 10.1111/liv.15219.
- Gatselis NK, Zachou K, Koukoulis GK, et al. Autoimmune hepatitis, one disease with many faces: etiopathogenetic, clinico-laboratory and histological characteristics. World J Gastroenterol. 2015;21(1):60–83. doi: 10.3748/wjg.v21.i1.60.
- Qiu DK. [Importance on the early diagnosis and treatment of autoimmune hepatitis.] Zhonghua Gan Zang Bing Za Zhi. 2012;20:321–323.
- Anastasiou OE, Dogan-Cavus B, Kucukoglu O, et al. Corticosteroid therapy improves the outcome of autoimmune hepatitis-induced acute liver failure. Digestion. 2018;98(2):104–111. doi: 10.1159/000487940.
- Kirstein MM, Metzler F, Geiger E, et al. Prediction of short- and long-term outcome in patients with autoimmune hepatitis. Hepatology. 2015;62(5):1524–1535. doi: 10.1002/hep.27983.
- Biewenga M, Verhelst X, Baven-Pronk M, et al. Development and validation of a prognostic score for long-term transplant-free survival in autoimmune hepatitis type 1. Unit Eur Gastroenterol J. 2021;9(6):662–671. doi: 10.1002/ueg2.12112.