Abstract
Optimisation of water and nitrogen use is an effective management tool to conserve resources and reduce environmental pollutions. Response surface methodology (RSM) is defined as a collection of mathematical and statistical methods that are used to develop, to improve or to optimize a product or process. In order to determine optimum levels of water, nitrogen and planting density of canola (Brassica napus L.), a 2-year experiment (2010–2011) was carried out by central composite design as RSM at the research station of Ferdowsi University of Mashhad. The treatments were designed based on low and high levels of irrigation (1500 and 4000 m3 ha−1), nitrogen (0 and 400 kg N ha−1) and density (50 and 150 plant m−2) as independent variables. Furthermore, seed yield, nitrogen losses, nitrogen use efficiency (NUE) and water use efficiency (WUE) were measured as response variables in a full quadratic polynomial model. Optimum levels of irrigation, nitrogen and planting density were suggested to achieve the target range of dependent variables based on three scenarios: economic, environmental and eco-environmental. The results showed that increasing irrigation and fertilizer led to an increase in seed yield and nitrogen losses, whereas increasing canola density resulted in an increase in seed yield but a decrease in nitrogen losses. The optimum levels of water, fertilizer and density based on environmental scenario were 1802 m3 ha−1, 11 kg N ha−1 and 122 plant m−2, respectively. To achieve optimum conditions under the economic scenario, it is necessary to use 3411 m3 water ha−1, 178 kg N ha−1 and 119 plant m−2. Amounts of 2347 m3 water ha−1, 92 kg N ha−1 and 114 plant m−2 were found to be the optimum conditions for the eco-environmental scenario. In general, it seems that resource use based on the eco-environmental scenario may be the most favorable cropping strategy for canola production.
Key words:
INTRODUCTION
Over 80% of the edible oil has been supplied through import in Iran, so extending oil crop cultivation is crucial for self-sufficiency (Safahani-Langerodi et al. Citation2008). Canola (Brussica napus L.) constitutes an important oil crop for the country due to its higher yield compared to other oil crops. Canola seeds contain approximately 40–45% high-quality oil that could be used for human consumption and also as biodiesel (Ariolu et al. Citation2010). Moreover, the remaining seed pulp can be used as animal food (Dogan et al. Citation2011). The cultivation area of canola has expanded rapidly from 135 ha in 1995 to 200,000 ha in 2010 and it has become an important crop in Iran (MAJ Citation2010).
Irrigation is an increasingly important practice for sustaining agriculture in Iran. In most regions of the country, irrigation water supplies are mainly from groundwater sources that are being depleted. About 85% of water used for irrigation comes from non-renewable ground water sources (MAJ Citation2010), and this share is expected to rise and subsequently decrease the ground water level in many parts of Iran. Hence, water used for irrigation from ground water sources has to compete with industry and urban demands (Al-Sulaimi et al. Citation1996). Cultivation of short-duration and less water-demanding crops is important not only to improve the economy but also to utilize the available land and water resources more effectively. Oilseed crops can be grown under a wide range of soil, water and climatic conditions (Al-Jaloud et al. Citation1996). Providing the optimum water supply to crops has a direct impact on crop yield and may also improve fertilizer efficiency (Meinke et al. Citation1997; Banedjschafie et al. Citation2008).
Nitrogen (N) is one of the most important nutrients in crop production systems (Shapiro et al. Citation2003; Maman et al. Citation2003). Excessive use of N in crop production causes a declining trend in nitrogen use efficiency (NUE). It has been reported that not more than 33% of N applied is used by the plant, while the remainder is lost and causes environmental pollution as well as emission of greenhouse gases (Raun and Johnson Citation1999; Shindo et al. Citation2009). Canola requires relatively large amounts of N fertilizer per yield unit compared to other most oil crops (Hocking et al. Citation1997; Singh et al. Citation2006). The yield response of rapeseed to an increasing N rate depends on farm management practices, including irrigation water levels, tillage type, plant density and cultivar (Ozer Citation2003). Increasing the NUE of canola through modifications in farming practices would be beneficial in improving the efficiency of fertilizer use, and the sustainability of canola production systems. In addition, the advantages of optimizing N fertilization on vegetative growth, seed yield and economic profit of canola have been stated in many publications (Cheema et al. Citation2001; Brennan and Bolland Citation2007; Juan et al. Citation2009).
N fertilizer and irrigation water are two of the most important factors in crop production and have been increased considerably over the last few decades for crop production in Iran. The yield of canola responds positively to an increase in the amounts of water and N applied until the optimum level has been reached. The rate of N fertilization for optimizing canola yield can vary with the water level and density. Many authors have studied separately the optimum N fertilization, water irrigation and plant density for canola production in Iran. For example, Gholamhoseini et al. (Citation2008) found that the yield and yield component of winter canola were increased with increasing N levels. Malek-Ahmadi et al. (Citation2009) studied the effect of plant density and N levels on canola seed yield and reported that the highest yield was obtained in 190 plant m2 and 300 kg urea ha−1. The effect of plant density on seed yield and growth of canola varieties was studied by Salehian et al. (Citation2002); Daneshmand et al. (Citation2008) showed that canola yield was improved with associated increasing irrigation water. No research has been conducted simultaneously on N fertilization, water irrigation and plant density for canola production.
In order to reduce the resource losses and environmental pollution and achieve appropriate yields, it is necessary to determine optimum levels of resources (such as N and water) and also suitable plant density in agro-ecosystems. On the other hand, there is a complex interaction between the amount of N fertilizer and water with crop density. Due to the wide number of treatment combinations, using conventional multifactor experiments to evaluate of this effect and choosing the best treatment combination is very costly, complicated and time-consuming and associated with considerable experimental error. Hence, the response surface methodology (RSM) has been proposed to determine the influences of individual factors and their interaction. With RSM, the interactions of possible influencing factors on responses can be evaluated with a limited number of experimental runs (Wang et al. Citation2007). So, the objective of this study was to determine the optimal level of N fertilizer, irrigation water and plant density in canola production based on central composite design (CCD) in Iran.
MATERIAL AND METHODS
Response surface methodology (RSM)
In order to achieve an acceptable yield with respect to decreasing environmental pollution, input values should be optimized in accordance to a targeted response. One of the methodologies for obtaining the optimum results is RSM (Kwak Citation2005; Aslan Citation2007). It is essential that the management practice of a farm be economical for achieving the maximum amount of yield and a significant reduction in environmental pollution, saving both resource and production costs. RSM is a statistical method based on the multivariate non-linear model and can be used for optimization of resource use (Zulkali et al. Citation2006; Kalavathy et al. Citation2009). Further, RSM consists of designing experiments to provide adequate and reliable measurements of the response, developing a mathematical model having the best fit to the data obtained from the experimental design, and determining the optimal value of the independent variables that produces a maximum or minimum response (Montgomery Citation2001; Kalavathy et al. Citation2009). It is also useful for studying the interactions of the various parameters affecting the response. RSM examines the responses of several factors by varying them simultaneously with a limited number of experiments. Therefore, RSM is a powerful tool for statistical modelling and optimization of the resources using a lower number of experimental runs planned according to an experimental design (Cojocaru and Zakrzewska-Trznadel Citation2007; Kalavathy et al. Citation2009). The response surface can be expressed as follows:
where y is the response variable and xi is the independent variable.
Experimental layout based on central composite design (CCD)
An effective alternative to the factorial design is the CCD, originally developed by Box and Wilson (Citation1951) and improved upon by Box and Hunter (Citation1957). The CCD gives almost as much information as a three-level factorial with a reduced number of tests (fewer than the full factorial experimental) and arranges the tests with various combinations of independent variables (Obeng et al. Citation2005; Aslan Citation2007). We employed a CCD for three independent variables to design experiments in which the variance of the predicted response, Y, at some points of the independent variable, X, is only a function of the distance from the point to the design center (Clarke and Kempson Citation1997; Kalavathy et al. Citation2009). The number of runs required for the CCD includes the standard 2k factorial with its origin at the center, 2k points fixed axially at a distance from the center to generate the quadratic terms, and replicate runs at the center (r), where k is the number of variables (Aslan Citation2007). Replicates of the test at the center are very important as they provide an independent estimate of the experimental error.
For three variables, the recommended number of tests at the center is six (Box and Hunter Citation1957; Aslan Citation2007). Hence the total number of tests required for the three independent variables is:
Once the desired ranges of values of the variables was designed by Minitab software ver. 14 based on lowest and highest levels of irrigation (1500 and 4000 m3 ha−1), N fertilizer (0 and 400 kg ha−1) and density (50 and 150 plant m−2), they were coded to lie at ± 1 for the factorial points and 0 for the center points (Kalavathy et al. Citation2009). +1, – 1 and 0 are dimensionless coded values of the independent variables which indicate the highest limit, lowest limit and mean of the highest and lowest limits of the variables, respectively. The experimental design matrix resulting from the CCD shown in consists of 20 runs of coded levels expressed as actual values.
Table 1 Coded and actual levels of independent variables
Field experiment and measurements
The experiment was performed in the 2010 and 2011 growing seasons at the research farm of Ferdowsi University of Mashhad, Mashhad, Iran. With regards to the similarity of the achieved results in both years, data was pooled and the pooled data was presented in this paper. The Research Station (36°16´N, 59°36´E) is located at about 985 m above sea level, in the northeast of Iran, having a semiarid climate with hot summers and cool winters. Average temperature and precipitation rate of the Research Station for the 2 years are shown in . The total precipitation (106 mm) during the crop-growing period for 2011 was higher than in the 2010 season (52 mm) and the average temperature (about 21°C) was the same for both years. Before sowing, soil samples were taken from 0–30 cm depth and the physico-chemical characteristics of the field soil were determined ().
Figure 1 Trend of precipitation (mm) and average temperature (°C) for two experimental years in Mashhad, Iran.
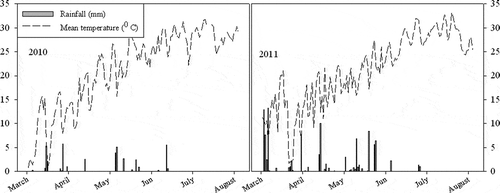
Table 2 Physical and chemical properties of the soil
Canola seeds (Hyola 401, spring cultivar) were hand sown in 3 m × 5 m plots with 50 cm row spacing in six rows on March 1 for both years. As soon as the seeds were sown, furrow irrigation was applied every 10 d. Germination was complete within 5–7 d. The crop was thinned after 3 weeks of emergence at the four-leaf stage to achieve target densities. N fertilizer (urea, 46% N) was split and applied as: one third pre-plant, one third at the beginning of stem elongation and the rest at the beginning of flowering. Irrigation treatments were initiated after plant roots were well established and adjusted by water counter. For 2 years, the farm was totally irrigated 10 times after this period and the amount of water for each interval was calculated based on dividing the total irrigation treatments (4000, 2750 and 1500 m3 ha−1) into 10. Therefore, the amounts of water for each interval were 400, 275 and 150 m3 ha−1 for the irrigation treatments. During the growth season, hand weeding was conducted three times. Five plants were randomly selected from each plot and harvested to determine N concentration by the micro-Keldjahl method (Nelson and Sommers Citation1973) every 14 d. Moreover, at harvesting time, N concentration of seeds, plant residuals and soil (depth of 0–30 cm) were determined, separately. All plants in the central four rows of each plot were hand-harvested to determine biological and seed yield on July 9 and 15 for 2010 and 2011 season growth, respectively.
N losses at the end of the growth season were calculated by Eq. 3:
where Nloss is N losses, Ninitial is the initial soil N content, Nfertilizer is the N fertilizer applied (pure N (N applied × 46%)), Nplant is the amount of plant N at harvesting and Nresidual is the soil N to 30 cm depth after harvesting.
Agronomic N use efficiency (ANUE) and agronomic water use efficiency (WUE) were calculated by Eqs. 4 and 5:
where ys is the seed yield (kg ha−1) and W is the amount of irrigation water (m3 ha−1).
Mathematical modeling
When the response data were obtained from the field experiment, a full quadratic polynomial equation was developed to predict the response as a function of independent variables (Kalavathy et al. Citation2009). The full quadratic polynomial equation as a response model incorporates (Obeng et al. Citation2005; Aslan Citation2007):
Linear terms in each of the variables (x1, x2, … , xn).
Squared terms in each of the variables (x12, x22, … , xn2).
First-order interaction terms for each paired combination (x1x2, x1x3, … , xn-ixn).
The coefficients of the response model (b1, b2, … , bn).
The intercept coefficient (b0).
Thus, for the three variables, the response model is:
where y is the predicted response, bi the linear terms, bii the squared terms, bij the interaction terms and xi and xj represent the coded independent variables. In this study, a full quadratic polynomial equation was obtained using the uncoded independent variables as follows:
The coefficient of the model for the response was estimated using multiple regression analysis technique included in the RSM (Kalavathy et al. Citation2009).
Scenarios
To calculate the optimum levels of water, N fertilizer and canola density as independent variables, we designed three scenarios (economical, environmental and eco-environmental). In each scenario, one of the responses was considered as the main factor to determine the optimum level of treatments. The determining main factor in each scenario was weighted. So, the coefficient of the main factor was 2 times higher than the other factors, which is an indication of a two-fold effect of the factor on the determination of optimum levels compared with the other factors. Grain yield of canola and N losses were considered as the main factors to determine the optimum level of treatments under the economical and environmental scenarios, respectively. In the eco-environmental scenario, the main factor was resource use efficiency (water and N).
RSM evaluation
The adequacy of the model was tested by analysis of variance (ANOVA). In general, the full quadratic polynomial equation was tested to determine the significance of the model and the component of the model (linear, squared, first-order interaction terms). The quality of the fitted model was judged using the determination coefficient (R2). Another criterion used to test differences between predicted and actual data was the 1:1 line. In addition, the lack-of-fit test was used to evaluate the quality of the fitted model. The lack-of-fit calculations are based on a comparison of the residual sum of squares to the sum of squares due to pure error (F-test). The lack-of-fit criterion used in this study was that the significance of lack-of-fit tested with an F-test should be less than 5%.
RESULTS AND DISCUSSION
The variance analysis of the treatments showed that the effect of year on canola traits was insignificant (). Therefore, a variance test was used for equality or homogeneity of variance by Bartlett’s test. The results of Bartlett’s test for each year illustrated the homogeneity of the variances for all traits (p > 0.05). So, the results for the two years were pooled ().
Table 3 Table of analysis of variance (ANOVA) (Mean Square) for the measured traits of canola (Brassica napus L.)
Table 4 Bartlett’s test for equal variance of the measured traits of canola (Brassica napus L.) versus year
Fitted model
Details of the ANOVA for the full quadratic polynomial model and components of this equation (linear, squared and first-order interaction) for the response variables (seed yield, N losses, NUE and WUE) are summarized in . The results of ANOVA showed that the full quadratic polynomial equation and components of the equation were significant based on F test (P < 0.01) for all of the variables except the first-order interaction term for WUE variable (). The polynomial models for the response variables gave insignificant lack-of-fit indicating that the data of experimental were satisfactorily explained (). Determination coefficient of dependent variables included seed yield, N losses, NUE and WUE were 96.1, 99.5, 97.6 and 98.2% respectively, which implied that a high proportion of the variability for these variables was explained by the fitted model (). There was a close correspondence between observed and estimated values of the all response variables based on 1:1 lines ().
Figure 2 Comparison of estimated and observed value of seed yield, nitrogen (N) losses, nitrogen use efficiency (NUE) and water use efficiency (WUE) by 1:1 line.
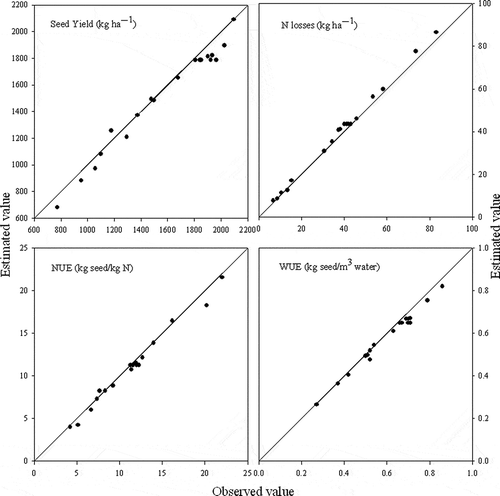
Table 5 Analysis of variance (ANOVA) (Mean Square) table for the responses and variables
Seed yield
The highest seed yield was obtained by application of 4000 m3 water ha−1 and 400 kg N ha−1 in density of 150 plant m−2 and the lowest seed yield was observed at the lowest level of all treatments (1500 m3 ha−1 water, 0 kg N ha−1 and 50 plant m−2) by 2095 and 744 kg ha−1, respectively (). The effects of response levels on seed yield as fitted curves by the full quadratic polynomial equation are shown in . The results revealed that the seed yield was increased with increase in applied N fertilizer under all levels of canola density (). This was expected since fertilizer N applied can provide a better plant growth and development (Ozer Citation2003). In all levels of N fertilizer, increasing of canola density caused to increase in seed yield (). It has been found that the slope of seed yield increased by an increase in density was higher under high levels of N fertilizer than under low levels, because the plant growth was improved in high amount of N fertilizer and resulted in high seed yield. Therefore, the effect of density on yield improvement could be increased at high levels of N fertilizer which clearly suggest the importance of N for higher seed production in canola. Under all levels of density, seed yield was increased by increasing of irrigation and the same results were gained in all amounts of irrigation by an increase in canola density (). The results showed that increasing of density led to higher yield at a high level of irrigation compared to a low level of irrigation (). Thus, the lowest seed yield was obtained with the lowest amounts of irrigation and density. Bañuelos et al. (2002) reported that increasing irrigation resulted in an increase in biomass and seed yield of canola. The evaluation of the response surface of irrigation-N fertilizer on seed yield showed the same trend of irrigation-density and N fertilizer-density (). Seed yield was increased by increasing irrigation and N fertilizer. By increasing N fertilizer at a high level of irrigation, the yield increase trend had a sharper slope compared with at a low level of irrigation. It seems that at low amounts of irrigation, application of higher amounts of N fertilizer had a small effect on canola growth due to low absorption of N under water limitation. However, the absorption of N was improved under non-limitation of water, which resulted in higher seed yield at high levels of irrigation. The effects of different regimes of irrigation and N fertilizer on canola yield showed that application of 200 kg N ha−1 caused an increase in yield, and further application of N higher than 200 kg N ha−1 showed no effect (Kamkar et al. Citation2011). On the other hand, our results illustrated that canola yield was improved until about 350 kg N ha−1, whereas the rate of 160 kg N ha−1 was reported as the optimum N amount for the Anatolia region by Ozer (Citation2003). He found that N rate recommendations are location-specific and affected by environmental conditions, and cultivar, residual soil N, rate and amount of N mineralized from soil organic sources, and individual crop needs are all effective to optimize N fertilizer recommendations.
Figure 3 The yield response to in dependent variable of nitrogen (N) fertilizer, irrigation water and canola (Brassica napus L.) density.
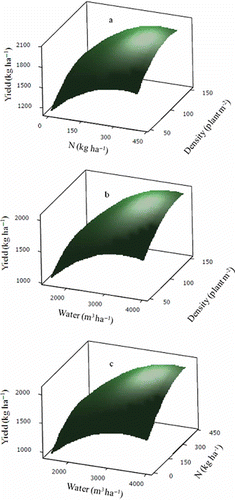
Table 6 Central composite design (CCD) and response results for the study of three experimental variables in coded units
N losses
Application of 4000 m3 ha−1 water and 400 kg N ha−1 with density of 50 plant m−2 had the highest N losses, and the lowest amount was obtained by the application of 1500 m3 water and 0 kg N ha−1 to 150 plant m−2 of canola (). Decreasing irrigation and N fertilizer and increasing canola density caused a decline in N losses. The negative effect of irrigation on N losses was highly observed by increasing irrigation which caused higher losses of N at all levels of canola density. Decreasing canola density was seen to reduce the N losses under all levels of irrigation (). High density of canola may have led to higher absorption of N and reduction of N losses due to increasing contact of the surface of roots with the soil, in comparison with low density. The application of a high amount of irrigation was associated with high available water for leaching of N that caused an increase in N losses under high irrigation. In addition, higher N losses were found under high irrigation at low density compared to high density (). The lowest and highest N losses as affected by both independent variables of irrigation-N fertilizer were related to low irrigation in high density and high irrigation in low density of canola, respectively (). N losses increased from 6.69 kg N ha−1 to 83.09 kg N ha−1 when N fertilizer was increased from 0 to 400 kg ha−1 under all different canola densities (), because high application of N fertilizer led to high available N for leaching which result in high N losses. It seems that the N losses at 0 kg N ha−1 were caused by mineralization of the initial N and loss of part of the available N. In other words, the origin of the N lost in the absence of N fertilizer was the soil initial N. The high amount of N fertilizer led to low recovery of N due to losses through denitrification, ammonia volatilization, runoff and especially leaching, and inefficient utilization by maize (Zea maize L.) crops was reported as an important constraint to N fertilizer use among farmers in the sub-region (Akintoye et al. Citation1999). It should be considered that the N losses came from not only the N fertilizer but also the initial soil N. Low application of N fertilizer at a high density of canola had minimum losses of N based on N fertilizer-density interaction (). Based on the effects of N fertilizer irrigation on N losses, it can be concluded that an increase in irrigation with a low level of N fertilizer may have led to a small increase in N losses, whereas further increase was found at a high level of N fertilizer in the same conditions ().
Nitrogen use efficiency (NUE)
Amounts of NUE ranged from about 4.02 to 22.03. Application of 4000 m−3 ha−1 water and 400 kg N ha−1 with a density of 50 plant m−2 provided the lowest NUE (). It seems that a high plant density of canola improves NUE by increasing biomass which led to reducing N losses due to high absorption of N by developed roots. NUE was reduced by increasing N fertilizer in all densities of canola (). Increasing canola density led to improvment of NUE under different amounts of N fertilizer. Maximum NUE was achieved by the application of no fertilizer at the highest density, and the minimum value was observed at the lowest density and high application of N as affected by N fertilizer-density interaction (). Increasing N fertilizer, especially at low levels, caused a reduction in NUE under all densities of canola, and the lowest NUE was seen with a higher application of N fertilizer. Because further increases in yield diminish with further increases in the amount of fertilizer N used, the efficiency of N utilization decreases as yield increases (Akintoye et al. Citation1999). It is well known that NUE decreases with the increment of N availability (Chamorro et al. Citation2002; Svečnjak and Rengel Citation2006). Evaluating the effects of density irrigation on NUE illustrated that NUE was improved by increasing irrigation at all canola densities. This improvement was higher at low levels of irrigation than at high levels (). Maximum and minimum values of NUE in relation to the density-irrigation effect were gained at high and low levels of density and irrigation, respectively (). Simultaneous effects of irrigation and N fertilizer on NUE showed that enhancing NUE was found by increasing irrigation and decrease in N fertilizer (). Improvement of NUE was associated with lower N utilization and higher grain yield. A study of N fertilizer effects on canola by Hocking et al. (Citation1997) illustrated that increasing N fertilizer from 0 to 150 kg N ha−1 caused a reduction of NUE.
Water use efficiency (WUE)
The highest WUE was obtained in the response surface of 1500 m3 ha−1 water, 400 kg N ha−1 and 150 plants m−2 and 4000 m3 ha−1 water, and the lowest amount of N (0 kg ha−1) and canola density (50 plants m−2) showed the lowest WUE (). Since the WUE is gained by ratio of yield and water consumption (Mandal et al. Citation2006), it seems that increasing the N level and plant density caused improvement in WUE through enhancing canola yield. An increase in both independent variables including N and plant density led to improvement of WUE (). Evaluation of the response surface revealed that the WUE was raised with associated increasing canola density in all N levels. Also, under all of the canola densities, an increase in N level improved WUE (). At the low levels of N (ranging for 0–200 kg ha−1), WUE was enhanced with a sharp slope as affected by increasing N under all densities, whereas an increase in N levels did not have a positive effect on WUE from 300 kg N ha−1 to the highest amount at all densities. The surface response of irrigation water and canola density illustrated that WUE declined remarkably with an increase in irrigation levels (). So, the highest WUE was achieved at the lowest irrigation level under all of the densities. On the other hand, at all irrigation levels, WUE was increased as effected by an increase in canola densities (). Dogan et al. (Citation2011) found that the highest irrigation water use efficiency was achieved at the lowest level of irrigation water, and this trait was decreased by increasing irrigation levels. They reported that the WUE obtained in the different treatments indicated a strong positive relationship between crop yield and irrigation. Assessing the response surface of irrigation water and N illustrated that the WUE was ameliorated as affected by increasing N levels and decreasing water levels (). An increase in the N level led to WUE improvement at all irrigation levels. At all N levels, WUE was reduced by increasing irrigation levels. Hence, the highest WUE was obtained at the highest level of N and the lowest irrigation level ().
Resource optimization
The optimum level of irrigation, N and canola density for achieving the targeted amount of responses based on three scenarios—economic, environmental and eco-environmental—is shown in . Seed yield was considered the main variable to determine the optimum level of recourse in the economic scenario. The results showed that application of 3411 m3 ha−1 water and 178 kg N ha−1 in density of 119 plant m−2 provided 2011 kg seed, 44 kg N ha−1 as losses, 14 kg seed kg−1 N for NUE and 0.59 kg seed m−3 water for WUE (). With respect to applied N fertilizer (178 kg N ha−1) to achieve the highest yield in the economic scenario, it could be concluded that application of N fertilizer at more than 200 kg ha−1 has no significant effect on yield enhancement. Subsequently, surplus N was lost by leaching and emission which resulted in environmental pollution. The determining variable to make a decision for optimizing resources was N loss reduction under the environmental scenario. In this scenario, the main target was decreasing environmental pollution with little attention to economic yield. The values of independent variables suggested for the environmental scenario were 1802 m3 water, 11 kg N ha−1 and 122 plant m−2. Based on the proposed values of the independent variables, the response variables (seed yield, N losses, NUE and WUE) were estimated to be 1080 kg seed ha−1, 10 kg N ha−1, 15 kg seed kg−1 N and 0.67 kg seed m−3, respectively (). To obtain the optimum levels of the independent variables in the eco-environmental scenario, NUE and WUE were the main variables, so the eco-environmental scenario was strongly affected by NUE and WUE. The optimum amount of irrigation, fertilizer and density was achieved by 2347 m3 ha−1 water, 92 kg N ha−1 and 114 canola m−2 in the eco-environmental scenario based on the fitted model (), and these values of resources led to gains of 1633 kg seed ha−1, 29 kg N ha−1, 18 kg seed kg−1 N and 0.76 kg seed m−3 water for seed yield, N losses, NUE and WUE, respectively (). The amounts of N losses in the environmental scenario were 77% and 65% smaller than economic and eco-environmental scenarios, respectively.
Table 7 Optimizing of independent variables and obtained values for response variables
In essence, with respect to the eco-environmental scenario which considers both seed yield (as an economic index) and N losses (as an environmental index), using resources (water and N fertilizer) and density based on this scenario can be suggested as an appropriate approach for achieving a desirable yield with associated reduction of environmental pollution.
CONCLUSION
Our results show that to maximize seed yield of canola, all amounts of independent variables (N, water and crop density) must be kept at the highest tested levels. On the other hand, to minimize N losses, the amount of N fertilizer and irrigation must be applied at the lowest tested levels while the density must be kept at the highest level. The highest level of the investigated treatments resulted in the highest canola seed yield, but N loss was high under this condition. Therefore, using resource use efficiency such as NUE and WUE as a criterion could ensure desirable yield and quality, and utilize the resources effectively, which has a marked effect on environmental health. For the economic scenario, the optimum values of water, fertilizer and density were 3411 m3 water ha−1, 178 kg N ha−1 and 119 plant m−2, respectively, and the optimum amount of the variables was obtained at 1802 m3 water ha−1, 11 kg N ha−1 and 122 plant m−2, respectively, under the environmental scenario. In the trial area, the farmers commonly apply more than 250 kg N ha−1 and 3000 m3 ha−1 water for canola production which is associated with high losses. Therefore, our results indicated that the application of 2347 m3 water ha−1 and 92 kg N ha−1 based on the eco-environmental scenario can be optimized for resource use, reduce environmental hazards and produce acceptable canola yield.
ACKNOWLEDGMENTS
The authors acknowledge the financial support of the project by Ferdowsi University of Mashhad, Iran.
REFERENCES
- Akintoye HA, Klinga JG, Lucas EO 1999: N-use efficiency of single, double and synthetic maize lines grown at four N levels in three ecological zones of West Africa. Field Crops Res., 60, 189–199. doi:10.1016/S0378-4290(98)00122-1
- Al-Jaloud AA, Hussian G, Karimulla S, Al-Hamidi AH 1996: Effect of irrigation and nitrogen on yield and yield components of two rapeseed cultivars. Agric. Water Manage., 30, 57–68. doi:10.1016/0378-3774(95)01207-9
- Al-Sulaimi J, Viswanathan MN, Naji M, Sumait A 1996: Impact of irrigation on brackish ground water lenses in Northern Kuwait. Agric. Water Manage., 31, 75–90. doi:10.1016/0378-3774(96)01237-1
- Ariolu HH, Kolsarici O, Goksu AT, Gulluoglu L, Arslan M, Caliskan S, Sogut T, Kurt C, Arslanoglu F 2010: Yag bitkileri uretiminin artirilmasi olanaklari. Ziraat Muhendisligi VII. Teknik Kongresi. Bildiriler Kitabi., 17, 361–376.
- Aslan N 2007: Application of response surface methodology and central composite rotatable design for modeling the influence of some operating variables of a Multi-Gravity Separator for coal cleaning. Fuel, 86, 769–776. doi:10.1016/j.fuel.2006.10.020
- Banedjschafie S, Bastani S, Widmoser P, Mengel K 2008: Improvement of water use and N fertilizer efficiency by subsoil irrigation of winter wheat. Europ. J. Agron., 28, 1–7. doi:10.1016/j.eja.2007.03.004
- Bañuelos GS, Bryla DR, Cook CG 2002: Vegetative production of kenaf and canola under irrigation in central California. Ind. Crop Prod., 15, 237–245. doi:10.1016/S0926-6690(01)00119-4
- Box GEP, Hunter JS 1957: Multi-factor experimental designs for exploring response surfaces. Ann. Math. Stat., 28, 195–241. doi:10.1214/aoms/1177707047
- Box GEP, Wilson KB 1951: On the experimental attainment of optimum conditions. J. Roy. Statist. Soc. Ser. B Metho., 13, 1–45.
- Brennan RF, Bolland MDA 2007: Effect of fertiliser phosphorus and nitrogen on the concentrations of oil and protein in grain and the grain yield of canola (Brassica napus L.) grown in south-western Australia. Aust. J. Exp. Agric., 47, 984–991. doi:10.1071/EA06115
- Chamorro AM, Tamagno LN, Bezus R, Sarandón S.J. 2002: Nitrogen accumulation, partition, and nitrogen-use efficiency in canola under different nitrogen availabilities. Commun. Soil Sci. Plant Anal., 33, 493–504. doi:10.1081/CSS-120002759
- Cheema MA, Malik MA, Hussain A, Shah SH, Basra SMA 2001: Effects of time and rate of nitrogen and phosphorus application on the growth and the seed and oil yields of Canola (Brassica napus L). J. Agron. Crop. Sci., 186, 103–110. doi:10.1046/j.1439-037X.2001.00463.x
- Clarke GM, Kempson RE 1997: Introduction to the Design and Analysis of Experiments, Arnold, London. pp334.
- Cojocaru C, Zakrzewska-Trznadel G 2007: Response surface modeling and optimization of copper removal from aqua solutions using polymer assisted ultrafiltration. J. Membrane Sci., 298, 56–70. doi:10.1016/j.memsci.2007.04.001
- Daneshmand AR, Shirani-Rad AH, Nour-Mohamadi G, Gh Z, Daneshian J 2008: Effect of water deficit and different nitrogen rates on yield, yield components and physiological traits of two rapeseeds (Brassica napus L) cultivars. J. Agric. Sci. Natur. Resour., 15, 112–121.
- Dogan E, Copur O, Kahramanb A, Kirnak H, Guldur ME 2011: Supplemental irrigation effect on canola yield components under semiarid climatic conditions. Agric. Water Manag., 98, 1403–1408. doi:10.1016/j.agwat.2011.04.006
- Gholamhoseini M, Aghaalikhani M, Malakouti MJ 2008: Effect of natural Zeolite and nitrogen rates on Canola Forage quality and quantity. J. Sci. Technol. Agric. Nutar. Resour., 12(45), 537–548. (In Persian).
- Hocking PJ, Kirkegaard JA, Angus JF, Gibson AH, Koetz EA 1997: Comparison of canola, Indian mustard and Linola in two contrasting environments I effects of nitrogen fertilizer on dry-matter production, seed yield and seed quality. Field Crop Res., 49, 107–125. doi:10.1016/S0378-4290(96)01063-5
- Juan Z, Jian-Wei1 L, Fang C, Yin-Shui L 2009: Increasing yield and profit of rapeseed under combined fertilization of Nitrogen, Phosphorus, Potassium, and Boron in Yangtze river Basin. Acta Agron Sin., 35, 87–92.
- Kalavathy HM, Regupathib I, Pillai MG, Miranda LR 2009: Modelling, analysis and optimization of adsorption parameters for H3PO4 activated rubber wood sawdust using response surface methodology (RSM). Colloid Surfaces B: Biointerfaces, 70, 35–45. doi:10.1016/j.colsurfb.2008.12.007
- Kamkar B, Daneshmand AR, Ghooshchi F, Shiranirad AH, Safahani Langeroudi AR 2011: The effects of irrigation regimes and nitrogen rates on some agronomic traits of canola under a semiarid environment. Agric. Water Manage., 98, 1005–1012. doi:10.1016/j.agwat.2011.01.009
- Kwak JS 2005: Application of Taguchi and response surface methodologies for geometric error in surface grinding process. Int. J. Mach. Tool Manuf., 45, 327–334. doi:10.1016/j.ijmachtools.2004.08.007
- MAJ (Ministry of Agriculture of the IR of Iran) Planning and Economics Department, Statistics Bank of Iranian Agriculture 2010: Visited at 24/09/2011
- Malek-Ahmadi H, Alizade H, Majnoon-Hosseini N, Shirani-Rad AH 2009: Effect of plant density and N fertilizer on yield and some morphological traits of winter canola (Brassica napus L). Iranian. J. Field. Crop. Sci., 40, 173–182. (In Persian).
- Maman N, Lyon DJ, Mason SC, Galusha TD, Higgins R 2003: Pearl Millet and Grain Sorghum Yield Response to Water Supply in Nebraska. Agron. J., 95, 1618–1624. doi:10.2134/agronj2003.1618
- Mandal KG, Hati KM, Misra AK, Bandyopadhyay KK 2006: Assessment of irrigation and nutrient effects on growth, yield and water use efficiency of Indian mustard (Brassica juncea) in central India. Agric. Water Manage., 85, 279–286. doi:10.1016/j.agwat.2006.05.004
- Meinke H, Hammer GL, van Keulen H, Rabbinge R, Keating BA 1997: Improving wheat simulation capabilities in Australia from a cropping systems perspective: Water and nitrogen effects on spring wheat in a semi-arid environment. Development in Crop Sci., 25, 99–112. doi:10.1016/S0378-519X(97)80012-8
- Montgomery DC 2001: Design and Analysis of Experiments fifth ed, John Wiley & Sons, New York.
- Nelson DW, Sommers LE 1973: Determination of total nitrogen in plant material. Agron. J., 65, 109–112. doi:10.2134/agronj1973.00021962006500010033x
- Obeng DP, Morrell S, Napier TJN 2005: Application of central composite rotatable design to modelling the effect of some operating variables on the performance of the three-product cyclone. Int. J. Miner. Process., 76, 181–192. doi:10.1016/j.minpro.2005.01.002
- Ozer H 2003: Sowing date and nitrogen rate effects on growth, yield and yield components of two summer rapeseed cultivars. Europ. J. Agron., 19, 453–463. doi:10.1016/S1161-0301(02)00136-3
- Raun WR, Johnson GV 1999: Improving nitrogen use efficiency for cereal production. Agron. J., 91, 357–363. doi:10.2134/agronj1999.00021962009100030001x
- Safahani-Langerodi A, Aynehband A, Zand E, Nour-mohammadi Gh, Baghestani MA, Kamkar B 2008: Evaluation of competitive ability in some canola (Brasica napus) cultivars with wild mustard (Sinapis arvensis) and relationship with canopy structure. J. Agric. Sci. Natur. Resour., 15(2), 211–222.
- Salehian J, Rafiey M, Fathi G, Siadat SA 2002: Effect of plant density on seed yield and growth of colza varieties at Andimeshek conditions In Proceedings of the 7th Iranian Crop Science Congress Karaj, Iran 24–26 August 2002.
- Shapiro CA, Ferguson RB, Ilergen GW, Dobcrmann AR, Wortrnann CS 2003: Fertilizer suggestions for corn. University of Nebraska. NcbGuide 074-174-A. Lincoln, NE. http://www.ianrpubs.unl.edu/sendlt/g174.pdf
- Shindo J, Okamoto K, Kawashima H, Konohira E 2009: Nitrogen flow associated with food production and consumption and its effect on water quality in Japan from 1961 to 2005. Soil Sci. Plant Nutr., 55, 532–545. doi:10.1111/j.1747-0765.2009.00382.x
- Singh B, Rengel Z, Bowden JW 2006: Carbon, nitrogen and sulphur cycling following incorporation of canola residue of different sizes into a nutrient-poor sandy soil. Soil Biol. Biochem., 38, 32–42. doi:10.1016/j.soilbio.2005.03.025
- Svečnjak Z, Rengel Z 2006: Canola cultivars differ in nitrogen utilization efficiency at vegetative stage. Field Crop Res., 97, 221–226. doi:10.1016/j.fcr.2005.10.001
- Wang JP, Chen YZ, Ge XW, Yu HQ 2007: Optimization of coagulation–flocculation process for a paper-recycling wastewater treatment using response surface methodology. Colloids and Surfaces A: Physicochem. Eng. Aspects, 302, 204–210. doi:10.1016/j.colsurfa.2007.02.023
- Zulkali MMD, Ahmad AL, Norulakmal NH 2006: Oryza sativa L husk as heavy metal adsorbent: Optimization with lead as model solution. Biores. Technol., 97, 21–25. doi:10.1016/j.biortech.2005.02.007