ABSTRACT
In the Sudan Savanna of West Africa, Plinthosols with a petroplinthic or pisoplinthic horizon at ≤ 50 cm from the surface comprise the major soils. Because these horizons limit the rooting volume and water and nutrient storage capacities of the soils, they should be a major cause of decreased crop yield in the Sudan Savanna. However, the local distribution of Plinthosols is not precisely known, and the relationships between soil classes, effective soil depth, and crop yield, which are considered to be closely related to each other on the Plinthosol soils, are not fully understood. To clarify these relationships, we first reassessed the soil toposequence on a slope at the Institute of Environment and Agricultural Research Saria station in Burkina Faso using the current World Reference Base soil classification system. We then determined the relationships between soil classes and sorghum yield and between the effective soil depth and yield. We also assessed whether ground penetrating radar could predict the position of a petroplinthic horizon. We found (1) that Pisoplinthic Petric Plinthosols were found at the upper slope, Petric Plinthosols were found at the middle slope, and Ferric Lixisols were found at the lower to toe slope; (2) that sorghum yield was significantly larger at the Ferric Lixisols, then at the Petric Plinthosols, and lower at the Pisoplinthic Petric Plinthosols; (3) that sorghum yield was proportional to the effective soil depth at which upper boundary of petroplinthic horizon was found (n = 26, R2 = 0.78*** exclusion of waterlogged soil); and (4) that ground penetrating radar could predict the effective soil depth and the position of petroplinthic horizons (n = 4, R2 = 0.99**), suggesting that we could roughly but easily predict sorghum yield and local distribution of Plinthosols having a petroplinthic horizon using GPR. These results may enable us to take more account of the inherent soil conditions when studying soil and water conservation, fertilization methods, and crop breeding, all of which are crucial if sustainable agricultural methods are to be achieved in the Sudan Savanna.
1. Introduction
The Sudan Savanna in West Africa (annual rainfall, 600–900 mm) is a climatic transition zone between the Sahel (annual rainfall, 200–600 mm) to the north and the Guinea Savanna (annual rainfall, 900–1,200 mm) to the south. The Sudan Savanna stretches for ~ 3,300 km from central Senegal and Gambia to northern Nigeria. Its climate is mainly BSh in the Köppen climate classification, i.e., a steppe climate (BS) with a mean annual temperature of 18°C or higher (h). Because of the semi-arid climate, the major crops in the Sudan Savanna are sorghum, pearl millet, cowpea, and groundnut. Maize, root crops, and rice can only be grown in fields rich in soil water (Matlon Citation1987; Callo-Concha et al. Citation2013). According to Callo-Concha et al. (Citation2013), crop production in the Sudan Savanna is limited by low water availability and soil fertility, which are both highly related to the dominant Plinthosol-type soils in this region (EU Citation2013). Plinthosols are defined as soils with a petroplinthic (PP) or pisoplinthic (PX) horizon starting ≤ 50 cm from their surfaces or a plinthic horizon starting ≤ 100 cm from the soil surfaces in the current World Reference Base (WRB) soil classification system (IUSS Working Group WRB Citation2015). Because a plinthic horizon generally develops under forest in a humid climate (unlike the Sudan Savanna) (Sivarajasingham et al. Citation1962; Eswaran et al. Citation1990), Plinthosols with a PP or PX horizon are mostly observed in the Sudan Savanna (EU Citation2013). More specifically, Plinthosols having a PP horizon is dominant in the Sudan Savanna except for Mali (EU Citation2013). Crop production on the Plinthosols is limited since PP and PX horizons reduce soil volume available for root elongation and storage of water and nutrients (Eswaran et al. Citation1990). The FAO report (FAO Citation1983) suggested that a field with an effective soil depth of < 30 cm can be classified as ‘not suitable’ for sorghum production. Lal (Citation1995) reported that crop yield in Africa reduces with decreasing effective soil depth.
To date, soil toposequence in the Sudan Savanna has been described in the old French classification system (Commission de Pédologie et de Cartographie des Sols (CPCS) Citation1967), in the legend for the Soil Map of the World (FAO-UNESCO-ISRIC Citation1988), and in the old WRB system 1998 (FAO, Citation1998) (Zerbo Citation1995; Issaka et al. Citation2004). Consequently, the distribution of Plinthosols is not precisely known, and the relationships between soil classes, effective soil depth, and crop yield, all of which should be closely related to each other, have not been fully evaluated. Junge and Skowronek (Citation2007) noted the need to update soil information in the francophone countries of West Africa where old classification systems are still used, because soil information is crucial for the assessment of soil fertility and decision-making concerning land management and soil conservation (Sanchez et al. Citation1982; Eswaran et al. Citation1997). Junge and Skowronek (Citation2007) also argued that updating soil information facilitates communication between users and scientists of different disciplines and countries.
Ground-penetrating radar (GPR) is used to survey underground soil by emitting electromagnetic waves into the ground and capturing reflected waves that indicate differences in the electrical properties between two soil layers (Davis and Annan Citation1989; Annan Citation2009; Society of Exploration Geophysicists of Japan (SEGJ) Citation2014). According to Doolittle and Butnor (Citation2009) and SEGJ (Citation2014), strong wave reflections are typically produced at soil interfaces that have abrupt boundaries and contrasting soil materials. Doolittle et al. (Citation2005) reported that GPR succeeded in detecting a petroferric contact in an Ultisol, which suggested that GPR can be used to estimate the position of a PP horizon that is an important classification criterion of Plinthosols in the current WRB system (IUSS Working Group WRB Citation2015) and that can highly affect crop yield. If GPR can be used to identify the boundary between an overlying soil and a PP horizon, then it will be a simple tool that can rapidly predict the local distribution of Plinthosols having a PP horizon and crop yield in the Sudan Savanna. Furthermore, GPR measurements may enable us to take more account of the inherent soil conditions (especially productivity) in the research areas such as soil and water conservation, fertilization method, and crop breeding, all of which are crucial for achieving sustainable agriculture in the Sudan Savanna.
In this study, we first reassessed soil toposequences at the Institute of Environment and Agricultural Research (INERA) Saria station in Burkina Faso, Sudan Savanna, using the current WRB system (IUSS Working Group WRB Citation2015). We then studied the relationships between soil classes and sorghum yield and between effective soil depth (defined as the soil thickness overlying the PP horizon) and yield. Finally, we assessed whether GPR could be used to predict the position of a PP horizon.
2. Materials and methods
2.1. Site description
Topographical, soil, crop, and GPR surveys were conducted at the INERA Saria station (N 12°16ʹ, W 2°09ʹ; 300 m above sea level), which is located at the Central Plateau of Burkina Faso, a typical region of the Sudan Savanna. The mean annual rainfall and mean annual temperature is 800 mm and 28°C, respectively, and the climate is classified as BSh in the Köppen climate classification. Rainfall occurs mostly between June and September with almost no rainfall between November and March. The mean annual potential evaporation was between 1,700 and 2,000 mm (Ouattara et al. Citation2006). The major types of trees are Vittelaria paradoxa, Parkia biglobosa, Lannea microcarpa, Adansonia digitate, Tamarindus indica, and Faidherbia albida, the major types of shrubs are Guiera senegalensis and Piliostigma reticulatum, and the major types of herbs are Andropogon gayanus, Cassia tora, Crotalaria retusa, Pennisetum pedicelatum, Schizachyrium exile, and Schoenefeldia gracilis (Guinko Citation1984; Zerbo Citation1995). According to Hottin and Ouedraogo (Citation1975) and Zerbo (Citation1995), geological formations at the station are attributed to the Precambrian; they are more or less metamorphosed. Two major types of rocks are found: (i) intrusive massive granodiorite and tonalite bodies, and (ii) undifferentiated granite and migmatite bodies.
2.2. Topographical survey
A line transect was placed from the river bottom to the plateau (1,200 m in length). Then, the topography on the line transect was assessed using a post-processing kinematic survey with two Global Navigation Satellite System receivers (Promark 100, Ashtech). One receiver served as the base and the other as the rover to collect raw data at an interval of 1 s. The post-processing data analysis was conducted using GNSS Solutions, version 3.8 (Trimble Navigation). The arithmetic mean of vertical error (two-sided 95% confidence interval) was < 0.07 m.
2.3. Soil survey
Soil profiles were first examined with the use of a hand auger (One-Piece Combination Auger, Eijkelkamp; 1.0 m long) at intervals of 50 m on the line transect. Soil texture and color in each horizon were assessed in the field, and the amount of pisoliths on the surface was also recorded. Based on these results, four soil profile groups were identified, and for each profile group, a soil pit was made to obtain a detailed description of the soil and soil samples. Soil profiles in the pits were described according to terms in the FAO report (FAO Citation2006). Soil samples taken from each horizon were subjected to physical and chemical analyses.
Soil samples were air dried and then passed through a 2-mm sieve. The pH (H2O and 1 M KCl) and electric conductivity (EC) were measured using a soil to solution ratio of 1:5 after shaking for 2 h with a pH electrode (LAQUA F-72, HORIBA) and with a conductivity meter (ES-51, HORIBA), respectively. Total carbon and total nitrogen were determined using the dry combustion method with an elemental analyzer (SUMIGRAPH NC-220F, Sumika Chemical Analysis Service). Exchangeable bases, exchangeable acidity, and cation exchangeable capacity (CEC) were measured basically according to Van Reeuwijk (Citation2002), however, inductively coupled plasma atomic emission spectroscopy (ICP-AES) with a spectrometer (ICPE-9000, SHIMADZU) was used in this study. Effective base saturation (EBS), as defined by the IUSS Working Group WRB (Citation2015), was calculated by dividing exchangeable (Ca2+ + Mg2+ + K+ + Na+) by exchangeable (Ca2+ + Mg2+ + K+ + Na+ + Al3+). Available phosphorus was determined by the Bray-I method (Bray and Kurtz Citation1945; Van Reeuwijk Citation2002) using a Shimadzu UV-1800 spectrophotometer. Particle size distribution was measured as described by Gee and Bauder (Citation1986). After chemical degradation of organic matter by H2O2 and sample dispersion with sodium hexametaphosphate, coarse- and fine-sand fractions (0.2–2 and 0.02–0.2 mm, respectively) were measured by sieving, and silt (0.002–0.02 mm) and clay (< 0.002 mm) fractions were measured with the pipette method. Bulk density was determined by measuring the masses of 100-ml core samples after drying at 105°C. Water retention by the soils was measured using a pressure plate apparatus (Dane and Hopmans Citation2002; DIK-3404, Daiki Rika Kogyo) at pF values between 1.6 and 3.2 and using a dew point potentiometer (Scanlon et al. Citation2002; WP4, Decagon Devices) at pF values between 3.6 and 4.2.
Soil profiles for the aforementioned four groups were classified using the current WRB system (Working Group and Wrb Citation2015), and then the classifications were compared with classifications that had been made previously using the old French system (CPCS Citation1967) and in the legend for the Soil Map of the World (FAO-UNESCO-ISRIC Citation1988) by Zerbo (Citation1995) who investigated soils at the INERA Saria station.
2.4. Crop yield
In the fields where the aforementioned soil pits were dug, sorghum (Sorghum bicolor (L.) Moench, var. Kapelga) was planted by hand in 2015 at a rate of 3.125 pockets m‒2; the intervals between rows and pockets were 80 cm and 40 cm, respectively. To prevent the entry of runoff water from outside the field, fallow (more than 30 m wide) or drainage was made at the two upper sides of each field. The size of each field was ~ 1.0 ha. Because field availability was limited, we did not plant a field for soil profile 2. Before sowing, the fields were plowed using a moldboard (up to 10 cm deep) with animal traction to make soil conditions as uniform as possible. Two weeks after sowing, the number of plants in each pocket was thinned to three. Chemical fertilizer was not applied so that inherent soil productivity would be measured. Weeds were controlled by hand hoeing two to three times per crop season. Sorghum yield was determined using the quadrat sampling method as follows. In each field, 2.4 m by 2.4 m quadrats (5.76 m2) were placed at a density of 1 quadrat per 100 m2 (100 quadrats per 1 ha). Sorghum grown in the 18 pockets within each quadrat was harvested, and the total grain yield in each quadrat was measured after air drying. To examine the relationship between effective soil depth and sorghum grain yield, the thickness of a soil overlying the PP horizons was measured at nine selected quadrats in each field. Note that the depth of a pisolithic layer or PX horizon can be included in the effective soil depth because they contain some fine earth (< 2 mm) and can contribute to crop production.
2.5. GPR survey
A GPR instrument (UtilityScan DF, GSSI) with a dual-frequency digital antenna (300 and 800 MHz) was employed to determine whether GPR could be used to measure the thickness of a soil overlying a PP horizon or effective soil depth. Topsoil near each of the four soil pits was flattened with a hand hoe and rake, and then the soil profiles were surveyed with GPR twice in March, 2016 at the end of the dry season when the soil was extremely dry so that soil moisture would not disturb the radar recordings. Effective soil depth estimated from the recorded section was compared with that determined by the soil survey in the soil pits.
With reference to Cassidy (Citation2009), the recorded sections were minimally processed using the dedicated software GPRAssistSe version 1.0, OYO (Radan 7; GSSI also can be used) before interpretation as follows. For each recorded section, a time-zero correction (adjustment of the soil-surface position) was made, and finite impulse response filters were used to remove high- and low-frequency noises with the default settings (vertical low passes of 100 and 200 MHz for the 300 and 800 MHz antennae, respectively; vertical high passes of 800 and 1500 MHz for the 300 and 800 MHz antennae, respectively). An automatic gain function was also applied to each section to improve the visual form. Because strong radar reflections are typically produced by soil interfaces that have abrupt boundaries and separate contrasting soil materials where there is a change in the relative permittivity (Doolittle and Butnor Citation2009; SEGJ Citation2014), a strong radar reflection in each section was considered a boundary between an overlying horizon and a PP horizon. Then, the distance from each soil surface to its boundary was determined by converting the time it took the radar to travel from the surface to the boundary, assuming that the electromagnetic wave velocity in a vacuum is 3.0 × 108 m s‒1 and the relative permittivity of the overlying horizon was 3.0 as reported by Davis and Annan (Citation1989).
2.6. Statistical analysis
Statistical analysis was performed using SPSS Statistics, version 21 (IBM). The significance of difference between grain yields was determined using the Kruskal-Wallis test because the variables did not meet the normality assumption. The relationships between effective soil depth and grain yield, and between predicted and observed effective soil depth, were investigated by simple regression analyses. Significance was always defined as P < 0.05.
3. Results
3.1. Soil toposequence
The results of the topographical survey are shown in the top panel of . Data for 605 m to 650 m from the river bottom could not be collected because the tall trees in the area greatly inhibited data collection. The mean slope between the river bottom and the plateau was 1% and the land was classified as level (FAO Citation2006).
Figure 1. Topography (top panel) and soil profiles (bottom panel) on the line transect.
Soil profiles were surveyed with a 1 m-long hand auger. Soil color was recorded in the moist condition using the Munsell Soil Color Charts.
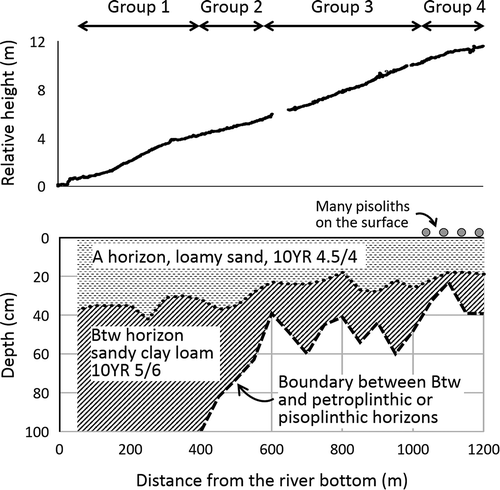
The bottom panel in shows the results of the soil profile survey with the 1 m-long hand auger. Since the auger often broke down petroplinthite which contains abundant pisoliths and dismantled petroplinthite looked like a pisolithic layer, PP and PX horizons could not be distinguished in . A profile of soil at the river bottom could not be made owing to the waterlogged condition of the soil.
According to the amount of pisoliths on the soil surface and the position of the PP/PX horizon, soil profiles were classified into four different groups (). For the group 1 soil, no pisoliths were found on the surface, and the PP/PX horizon was not found within 100 cm of the surface. For the group 2 soil, no pisoliths were found on the surface, and the PP/PX horizon was found within 50 to 100 cm of the surface. For the group 3 soil, a few pisoliths (2%‒5%) were found on the surface, and the PP/PX horizon was found from 30 to 50 cm below the surface. For the group 4 soil, many pisoliths (15%‒40%) were found on the surface, and the PP/PX horizon was found < 40 cm from the surface.
describes the soils for each group, and ‒ present the results of the physical and chemical analyses. Profile 1 soil had argic horizons (Btw, Btwc1, Btwc2) with a CEC of < 24 cmolc kg‒1 clay, an EBS ≥ 50%, and a ferric horizon (Btwc1) starting ≤ 100 cm from the soil surface and therefore was classified as Ferric Lixisols (LX-fr) based on the recent WRB system (IUSS Working Group WRB Citation2015). Profile 2 soil had argic horizons (Btw, Btwc) with a CEC of < 24 cmolc kg‒1 clay, an EBS ≥ 50%, and a ferric horizon (Btwc) starting ≤ 100 cm from the surface; it also had a PP horizon (Bmv) starting within 50 to 100 cm of the surface and therefore was classified as Ferric Petroplinthic Lixisols (LX-pp.fr). Profile 3 soil had a PP horizon (Bmv) starting ≤ 50 cm from the surface and therefore was classified as Petric Plinthosols (PT-pt). Profile 4 soil had PP and PX horizons (Bmv, Ac) starting ≤ 50 cm from the surface and therefore was classified as Pisoplinthic Petric Plinthosols (PT-pt.px). In , iron nodules in mineral concentration (kind is nodule; nature is iron) corresponds to pisoliths and Btwc2 in profile 1, Btwc in profile 3 and Apc and Ac in profile 4 are pisolithic layers with abundant pisoliths. However, most pisolithic layers did not meet a thickness requirement of PX horizon (≥ 15 cm) except for Ac in profile 4.
Table 1. Summary of morphological properties of the soils from four groups.
Table 2. Physical properties of the soil from each of the four groups.
Table 3. Chemical properties of the soils from four groups.
3.2. Relationships between soil classes and sorghum yield
The annual rainfall in 2015 was 800 mm, the mean at the INERA Saria station. shows the sorghum yields in the fields of groups 1, 3, and 4. (As stated above, the soil of group 2 was not cultivated.) Because the grain yields did not follow normal distributions, they are illustrated by boxplots (). Grain yield was significantly greater for LX-fr, with a moderate-to-large effective soil depth (1,070 kg ha‒1), then for PT-pt, with small effective soil depth (559 kg ha‒1), and lower for PT-pt.px, with a very small effective soil depth (244 kg ha‒1). Although a pisolithic layer or a PX horizon is often considered unproductive, the Apc and Ac horizons at PT-pt.px. contributed, not largely, but surely, to crop production.
3.3. Relationship between effective soil depth and sorghum yield
Effective soil depth in the field was 70–142 cm (mean, 112 cm) for group 1, 33–55 cm (mean, 44 cm) for group 3, and 18–34 cm (mean, 26 cm) for group 4. shows the significant correlation between grain yield (y axis, in Mg ha‒1) and effective soil depth (x axis, in cm). In the field for group 1, the lowest position closest to the river was affected by the waterlogging. When the value for the waterlogged soil was removed, the regression equation was y = 0.0143x ‒ 0.174 (; n = 26, R2 = 0.78***), and when that value was included, y = 0.0122x ‒ 0.0972 (n = 27, R2 = 0.63***).
3.4. Relationship between predicted effective soil depth by GPR and observed depth
The GPR equipment employed in this study (UtilityScan DF, GSSI) has a dual-frequency digital antenna (300 and 800 MHz) and can, therefore, record two types of data. We employed the 800 MHz antenna to predict the effective soil depth ≤ 75 cm from the surface and the 300 MHz antenna to predict the effective soil depth > 75 cm from the surface. shows the strong correlation between predicted effective soil depth by GPR and that determined by the soil survey in the four soil pits (n = 4; y = 1.02x, R2 = 0.99**). The arithmetic mean of error, i.e., the difference between the predicted and observed values, was 2.9 cm. When the effective soil depths ≤ 50 cm and > 50 cm from the surface were predicted using the recorded sections taken with the 800 MHz and 300 MHz antennae, respectively, the slope of the regression equation was 0.963, R2 was 0.95 (though P < 0.05), and the error increased to 5.7 cm. Notably, the sections obtained with the 300 MHz antenna could not be used to predict effective soil depth at ≤ 40 cm below the surface (frequency was too low).
4. Discussion
4.1. Soil toposequence
We found PP horizons (Bmv) in all the four profiles (). Prescott and Pendleton (Citation1952) reported that laterite (now known as petroplinthite) is generally found at level terrains that are similar to our study area (, top panel). Although the processes by which the PP horizons in this study area formed and the origin of the iron that indurates them are not clear (either in situ or allochthonous), the PP horizons contained abundant pisoliths (), which suggests that the petroplinthites are ‘old’ (Sivarajasingham et al. Citation1962).
As mentioned by Prescott and Pendleton (Citation1952) and by Sivarajasingham et al. (Citation1962), PP horizons were found in subsoils (), although Nahon (Citation1986) noted that iron crusts in western Senegal are generally present at the top of soil profiles. In this study, the thickness of the soil overlying the PP horizon was less in the upper slope and greater in the lower slope (), probably as a result of soil erosion in the upper slope and its redeposition at the lower slope. Above the PP horizons, there were soil layers with abundant (40% to 80% by volume) pisoliths in profiles 1 (Btwc2), 3 (Btwc), and 4 (Apc and Ac) (see ‘mineral concentration’ in ), however a PX horizon could only be considered to exist in profile 4 as its thickness is defined as ≥ 15 cm. Sivarajasingham et al. (Citation1962) noted that pisolithic layers are often observed above PP horizons. The pisolithic layers found in our study might not be a colluvial deposit from an adjacent area at a greater height that once existed but might be developed by disintegration or dismantling of the underlying PP horizons. We suggest this possibility because the sizes and shapes of the pisoliths in the pisolithic layers of profiles 1, 3, and 4 were similar and because the thicknesses of the pisolithic layers did not progressively increase downslope. If the pisoliths above the PP horizons are colluvial deposits, their sizes and shapes should be smaller and rounder in the lower slope than in the upper slope, and the pisolithic layers should be thicker in the lower slope.
A sharp rise in the clay content at each Bt horizon was found for profiles 1, 2, and 3. The clay in the Bt horizons would have been illuvially accumulated from the overlying horizons. However, it is also possible that the A horizons in those three profiles are aeolian deposits from the Sahara Desert and are not related to the development of the Bt horizons. Grove (Citation1958) argued that sand dunes in West Africa extended south to about 800-mm isohyet during the last glacial maximum.
and ‒ show that PT-pt.px mainly formed the upper slope, that PT-pt and LX-pp.fr mainly formed the middle slope, and that LX-fr mainly formed the lower and toe slopes. Comparison of the soil classes in this study with those used in the old French system (CPCS Citation1967) and the legend for the Soil Map of the World (FAO-UNESCO-ISRIC Citation1988) reported by Zerbo (Citation1995) suggests the following: (1) that les sols ferrugineux tropicaux lessivés indurés (IX-123) superficiels (0 to 20 cm) and peu profonds (20 to 40 cm) in the old French system and the Lithic or Eutric Leptosols in the legend for the Soil Map of the World correspond to PT-pt.px in the current WRB system; (2) that some of the IX-123 moyennement profonds (40 to 60 cm) and part of the Haplic LX with a petroferric phase correspond to PT-pt; (3) that the other IX-123 moyennement profonds (40 to 60 cm), some of the IX-123 profonds (> 60 cm), and many of the LX-fr with a petroferric phase correspond to LX-pp.fr; and (4) that the other IX-123 profonds (> 60 cm) and LX-fr correspond to LX-fr. These correlations facilitate the conversion from soil classes in the old classification systems to those in the current WRB system in francophone countries of West Africa.
4.2. Soil productivity
Soil productivity was correlated with soil classes in the current WRB system (), which indicated that each soil class can be a good indicator of its potential productivity in the Sudan Savanna. These results are explained by the relative thicknesses of the soil overlying the PP horizon (i.e., effective soil depth) that are important classification criteria for Plinthosols and Lixisols in the current WRB system and that greatly affect sorghum yield (). When a soil class is LX-fr, LX-pp.fr, PT-pt, or PT-pt.px, then its PP horizon must start, by definition, at ˃100, 50‒100, ≤ 50, and ≤ 50 cm from its surface, respectively, and the effective soil depth will have the same values. The difference in productivity for PT-pt and PT-pt.px was mainly a consequence of the positions of their PP horizons – for PT-pt.px it was shallower than PT-pt ().
Sorghum yield was proportional to the effective soil depth (). This would be because the effective soil depth is highly related to soil volume which governs water holding capacity as well as nutrient supplying capacity. Hurni (Citation1985) also reported a linear relationship between grain yield and soil depth. With this relationship in hand, farmers in the Sudan Savanna should be able to easily understand the soil productivity in their fields by determining the petroplinthite position.
4.3. Potential of GPR for predicting soil productivity and distribution of Plinthosols
shows that GPR can be used to reliably predict effective soil depth. Because effective soil depth is strongly correlated with sorghum yield (), GPR can be employed to expeditiously and two-dimensionally predict soil productivity in the Sudan Savanna, which will enable soil and crop scientists, crop breeders, and other associated researchers to take into account the inherent soil productivity in their studies by using GPR.
In our study, GPR could detect PP horizons, as had been suggested by Doolittle (Citation2005). This indicates that LX-fr, LX-pp.fr, and PT-pt can be distinguished by using GPR. While, GPR could not detect pisolithic layers, probably because pisolithic layers in this study are too thin or contain more fine earth (). However, shows the possibility that PT-pt and PT-pt.px could be distinguished by the effective soil depth, though they are distinguished, in the definition, by the presence or absence of a pisoplinthic horizon. When the effective soil depth is < 33 cm or 33–50 cm, the soil can roughly be considered PT-pt.px or PT-pt, respectively. This result suggests that GPR can help to predict, although not always precisely, the distribution of Plinthosols having a PP horizon in the Sudan Savanna.
5. Conclusions
Topographical, soil, crop, and GPR surveys were conducted at the Sudan Savanna where the major soils are Plinthosols having a PP horizon, and therefore, crop yield is expected to be reduced. We found a correspondence between the soil classes used in the old French classification system and the legend for the Soil Map of the World and those in the current WRB system, which facilitates conversion among soil classes in francophone countries. We found that soil types in the Sudan Savanna defined by the current WRB system can be a simple indicator of soil productivity because crop yield correlated strongly with effective soil depth, which is an important criterion for defining Plinthosols and Lixisols in the current WRB system. We also demonstrated that GPR can be used to predict the effective soil depth and soil productivity and roughly predict the local distribution of Plinthosols having a PP horizon in the Sudan Savanna, which should make it easier for researchers to take into account inherent soil conditions in their research by using GPR.
Acknowledgments
This study was conducted as part of the JIRCAS-INERA collaborative project entitled ‘Development of technologies for sustainable agricultural production in the African Savanna (2011–2015)’ and ‘Development of watershed management model in the Central Plateau, Burkina Faso (from 2016).’We thank Dr. Lamien Niéyidouba, Dr. Korodjouma Ouattara, Dr. Adama Kaboré, and Mr. Simporé Kouka for their support.
Disclosure Statement
Not applicable
References
- Annan AP 2009: Electromagnetic principles of ground penetrating radar.In Ground Penetrating Radar: theory and applications, Jol HMed., pp.1–40. Elsevier Science, Amsterdam.
- Bray RH, Kurtz LT 1945: Determination of total, organic, and available forms of phosphorus in soils. Soil Sci. 59, 39–46. doi:10.1097/00010694-194501000-00006
- Callo-Concha D, Gaiser T, Webber H, Tischbein B, Müller M, Ewert F 2013: Farming in the West African Sudan Savanna: insights in the context of climate change. Afr. J. Agric. Res. 8, 4693–4705. doi:10.5897/AJAR2013.7153
- Cassidy NJ 2009: Ground penetrating radar data processing, modelling and analysis.In Ground Penetrating Radar: theory and applications, Jol HMed., pp.141–176. Elsevier Science, Amsterdam.
- Commission de Pidologie et de Carthographie des Sols (CPCS) 1967: Classification des sols. Ecole Nationale Supérieure d’Agronomie, Grignon. http://library.wur.nl/isric/fulltext/isricu_i33696_001.pdf (February, 2018)
- Dane JH, Hopmans JW 2002: Pressure plate extractor.In Methods of soil analysis: part 4. Physical methods, Dane JH, Topp GCeds., pp.688–690. SSSA, Madison, WI.
- Davis JL, Annan AP 1989: Ground-penetrating radar for high-resolution mapping of soil and rock stratigraphy. Geophys. Prospect. 37, 531–551. doi:10.1111/j.1365-2478.1989.tb02221.x
- Doolittle JA, Butnor JR 2009: Soils, peatlands, and biomonitoring.In Ground Penetrating Radar: theory and applications, Jol HMed., pp.178–202. Elsevier Science, Amsterdam.
- Doolittle JA, Daigle JJ, Kelley JA, Tuttle JW 2005: Using GPR to characterize plinthite and ironstone layers in ultisols. Soil Surv. Horiz. 46, 179–184. doi:10.2136/sh2005.4.0179
- Eswaran H, De Coninck F, Varghese T 1990: Role of plinthite and related forms in soil degradation. In Advances in Soil Science, Lal R, Stewart BAeds., Vol. 11 pp.109–127.Springer, New York. doi: 10.1007/978-1-4612-3322-0_3
- Eswaran H, van den Berg R, Reich E 1997: An assessment of the soil resources of Africa in relation to productivity. Geoderma. 77, 1–18. doi:10.1016/S0016-7061(97)00007-4
- EU 2013: Soil Atlas of Africa, Jones A, Breuning-Madsen H, Brossard M et al. eds., European Commission, Publications Office of the European Union, Luxembourg.
- FAO 1983: Guidelines: Land Evaluation for Rainfed Agriculture. FAO Soils Bulletin, Vol. 52 FAO, Rome.
- FAO 1998: World reference base for soil resources. World Soil Resources Report, Vol. 84. FAO, Rome. http://www.fao.org/docrep/W8594E/W8594E00.htm (February, 2018)
- FAO 2006: Guidelines for soil description. FAO, Rome. http://www.fao.org/docrep/019/a0541e/a0541e.pdf (February, 2018)
- FAO-UNESCO-ISRIC 1988: Revised legend of the FAO-UNESCO soil map of the world. World Soil Resources Report, Vol. 60. FAO, Rome. http://www.isric.org/sites/default/files/isric_report_1988_01.pdf (February, 2018)
- Gee GW, Bauder JW 1986: Particle-size analysis. In Methods of soil analysis: part 1. Physical and mineralogical methods, agronomy, Klute Aed., Vol. 9 pp.383−411. ASA-SSSA publisher, Madison, WI.
- Grove AT 1958: The Ancient Erg of Hausaland, and similar formations on the south side of the Sahara. Geogr. J. 124, 526−33. doi:10.2307/1790942
- Guinko S 1984: Végétation De La Haute-Volta, Doctor thesis, University of Bordeaux III, Bordeaux.
- Hottin G, Ouedraogo OF 1975: Notice Explicative De La Carte Géologique a 1/1,000,000 De La République De La Haute-Volta, Direction de la Geologie et des Mines, Ouagadougou.
- Hurni H 1985: Erosion-productivity-conservation systems in Ethiopia.In Proceedings of 4th International Conference on Soil Conservation, pp.654−674. Venezuelan Society of Soil Science, Maracay.
- Issaka RN, Senayah JK, Adjei EO, Oppong J 2004: Soil resources of Saboba-Chereponi district: an assessment for agricultural production. West Afr. J. Appl. Ecol. 5, 93−101. doi:10.4314/wajae.v5i1.45600
- IUSS Working Group WRB 2015: World reference base for soil resources 2014, update 2015. World Soil Resources Report, Vol. 106. FAO, Rome.
- Junge B, Skowronek A 2007: Genesis, properties, classification and assessment of soils in Central Benin. West Africa. Geoderma. 139, 357−370. doi:10.1016/j.geoderma.2007.02.015
- Lal R 1995: Erosion-crop productivity relationships for soils of Africa. Soil Sci. Soc. Am. J. 59, 661−667. doi:10.2136/sssaj1995.03615995005900030004x
- Matlon P 1987: The West African semiarid tropics.In Accelerating food production in Sub-Saharan Africa, Mellor JW, Delgado CL, Blackie MJeds., pp.59−77. Johns Hopkins Univ. Press, Baltimore, MD.
- Nahon DB 1986: Evolution of iron crusts in tropical landscapes.In Rates of chemical weathering of rocks and minerals, Colman SM, Dethier DPeds., pp.169−191. Academic Press, London.
- Ouattara K, Ouattara B, Assa A, Sédogo PM 2006: Long-term effect of ploughing, and organic matter input on soil moisture characteristics of a ferric lixisol in Burkina Faso. Soil Till Res. 88, 217–224. doi:10.1016/j.still.2005.06.003
- Prescott JA, Pendleton RL 1952: Laterite and lateritic soils. Commonwealth Bureau of Soil Science Technical Communication, No. 47, Commonwealth Agricultural Bureau, Farnham Royal, Buckinghamshire.
- Sanchez PA, Couto W, Buol SW 1982: The fertility capability soil classification system: interpretation, application and modification. Geoderma. 27, 283–309. doi:10.1016/0016-7061(82)90019-2
- Scanlon BR, Andraski BJ, Bilskie J 2002: Dew point potentiameter.In Methods of soil analysis: part 4. Physical methods, Dane JH, Topp GCeds., pp.662–665. SSSA, Madison, WI.
- Sivarajasingham S, Alexander LT, Cady JG, Cline MG 1962: Laterite. In Advances in agronomy, Norman AGed., Vol. 14 pp.1–60.Academic Press, New York. doi: 10.1016/S0065-2113(08)60435-6
- Society of Exploration Geophysicists of Japan (SEGJ) 2014: Application manual of geophysical methods to engineering and environmental problems, European Association of Geoscientists and engineers (EAGE) publications, Houten, Utrecht.
- van Reeuwijk LP 2002: Procedures for soil analysis. Technical Paper, No. 9. ISRIC, Wgeningen. http://www.isric.org/sites/default/files/ISRIC_TechPap09.pdf (February, 2018)
- Zerbo 1995: Caractérisation Des Sols Des Stations De Recherches Agricoles De l’INERA, INERA, Ouagadougou.