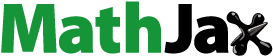
ABSTRACT
It is essential to manage nitrogen (N) fertilization to obtain the appropriate protein contents in wheat grains. In this study, N uptake from the booting stage to flag leaf appearance, and the tiller number at the booting and panicle formation stages was estimated using a handheld active optical sensor of the normalized difference vegetation index (NDVI) under different measurement conditions in Hokkaido, Japan. A stable NDVI was obtained if measured horizontally at 60–120 cm height from the canopy regardless of ambient radiation at different local times . However, the relationship between N uptake and NDVI differed significantly if the NDVI was measured at a 20 cm height (P < 0.01) or at 45° angle to the soil surface (P < 0.001). Regression analysis revealed that N uptake could be fitted by a quadratic curve of NDVI (N uptake = 0.39 + 17.4 × NDVI2; P < 0.001) across the different regions, years, and growth stages if the NDVI was less than 0.75. However, the accuracy was poor if the NDVI was higher than 0.75 probably because the emitted and reflected light was scattered in the canopy owing to increased leaf area and causing saturation of the NDVI. Significant positive correlations were observed between tiller number and NDVI at booting (P < 0.001) and panicle formation (P < 0.001). However, the relationship at the booting stage differed among regions and years. The significant positive correlation between the residuals of the relationship and NDVI of bare soil (P < 0.001) suggested that the NDVI at booting was influenced by soil conditions owing to low green coverage. In conclusion, it is expected the rapid monitoring of N uptake and tiller number of winter wheat using an active optical NDVI sensor will be helpful for simple and rapid decision-making for N fertilization management.
1. Introduction
The normalized difference vegetation index (NDVI) has often been used to estimate dry matter, nitrogen (N) uptake, and grain yield of winter wheat (Moges et al. Citation2004; Diacono, Rubino, and Montemurro Citation2013; Zhang et al. Citation2019). Recently, the size and price of handheld NDVI sensors have decreased and thus use of NDVI sensors has become widespread.
Wheat is an important arable crop in Hokkaido, Japan. Given that 62% of Japanese wheat is produced in Hokkaido (Ministry of Agriculture F and F of J Citation2018), wheat is crucial for Hokkaido agriculture both with regard to food supply and farmers’ income. The purchase price of wheat grains depends on qualities represented as the grade and rank, which are determined by properties such as grain density, proportion of infected grains, and protein content. The protein content of the rank ‘A’ grains must be within 97 to 113 g kg−1. Thus, farmers manage N fertilization so that the protein content of grains is within the standard range of the rank ‘A’. Notably, Hokkaido Fertilizer Recommendations provide guidance on the management of N fertilization to determine additional N fertilization rates (Department of Agriculture Hokkaido Local Government Citation2015; Fueki et al. Citation2015). If the tiller number exceeds 1000 tillers m−2 at the booting stage after snowmelt, additional N fertilizer needs to be applied at the stage of panicle formation in eastern Hokkaido. At the stage of flag leaf appearance, 1) N uptake is estimated from the number of superior (fertile) stems and leaf color (SPAD); and 2) the additional N fertilization rate is calculated from the difference between the required N uptake at harvest calculated from the target yield set by the farmer and the N uptake until the stage of flag leaf appearance estimated by 1). However, counting the number of superior stems and SPAD measurement is complicated and time-consuming, although the prediction accuracy is satisfactory. Thus, it is desirable to develop easier and quicker methods to estimate N uptake using a handheld NDVI sensor also in Hokkaido contributing easy and rapid decision making of additional N fertilization. However, the estimation of N uptake of winter wheat in Hokkaido by NDVI has not been studied yet. The estimation of tiller number by NDVI was studied in Sorachi and Ishikari regions of Hokkaido (Sasaki, Murakami, and Hayashi Citation2014) but has not been studied in Tokachi and Okhotsk regions where typical soil type is different so that the reflectance from soils may influence the accuracy for the estimation of tiller number. Furthermore, studies about the effect of measurement angle and time on NDVI readings are still limited compared with measurement height (Crusiol et al. Citation2017; Nishida et al. Citation2018; Gianquinto et al. Citation2019). The objectives of the present study were (1) to investigate the appropriate measurement conditions for a handheld NDVI sensor and (2) to estimate the N uptake and tiller number of winter wheat from the NDVI in Hokkaido. Also, the estimation accuracy was investigated whether a handheld NDVI sensor could be provided through agricultural extensions in Hokkaido.
2. Materials and methods
2.1. Site description
The study was conducted mainly at the Central Agricultural Experiment Station (43°3′N, 141°45′E), Tokachi Agricultural Experiment Station (42°53′N, 143°5′E), and Kitami Agricultural Experiment Station (43°45′N, 143°43′E) of the Hokkaido Research Organization, Japan. In addition, experiments were performed in farmers’ fields in the Sorachi (five fields), Tokachi (nine fields), and Okhotsk (four fields) regions, respectively (, ). Winter wheat (Triticum aestivum L.) ‘Kitahonami’, which is currently the most widely planted cultivar in Hokkaido, was cultivated in all fields.
Table 1. Site characteristics and fertilization patterns in this study
2.2. Test 1: Measurement conditions of NDVI
To compare different measurement conditions of the NDVI, experimental plots with different sowing dates (14 September and 5 October 2016), different sowing densities (140 and 255 grains m−2), and different N fertilizer regimes (0-0-0, 4-0-0, 4-4-0, 4-4-6, 4–10-0, 4-0-10, where x-y-z represents x g N m−2 at sowing, y g N m−2 at the booting stage, and z g N m−2 at the panicle formation stage, respectively) were established at the Tokachi Agricultural Experiment Station in 2016/17. Here, the NDVI was measured using an active optical sensor (GreenSeeker® handheld crop sensor, Trimble Navigation Ltd, Sunnyvale, CA, USA) at different heights (20, 60, 90, 100, and 120 cm above the top of the canopy) and different angles (horizontal, 45° along the row, and 45° orthogonal to the row) at the stage of flag leaf appearance on 23 May 2017. Aboveground wheat plants were sampled soon after the measurement of the NDVI. All samples were dried at 75°C for more than 48 h and the dry weight of each sample was measured. The dried samples were milled and digested using the Kjeldahl method. The N concentration of the digested samples was measured using an automated flow injection analyzer (FIAstar 5000 Analyzer, FOSS, Hillerød, Denmark). In addition, the NDVI was measured during the daytime (15:50) and nighttime (19:10) on 15 May 2018, to examine the effect of ambient sunlight on NDVI measurement.
2.3. Test 2: Estimation of N uptake and tiller number using NDVI
The different additional N fertilization rates were established based on randomized blocks design with three replications at each of the Central, Tokachi, and Kitami Agricultural Experiment Stations and in the farmers’ fields (). Nitrogen uptake, tiller number, and the NDVI were measured in these fields at the stages of booting (mainly in early April after snowmelt), panicle formation (early May), and flag leaf appearance (end of May), respectively. The results obtained in 2013/14, 2014/15, 2015/16, 2016/17, and 2017/18 at the Agricultural Experiment Stations were used for the calibration dataset and the other experimental data were used for validation.
2.4. Statistical analysis
To analyze the effect of the measurement height and angle on the relationship between N uptake and NDVI2 for Test 1, analysis of covariance (ANCOVA) and Tukey’s multiple comparison test were conducted to examine whether the measurement height and angle significantly influenced the intercept and slope of the relationship between N uptake and NDVI2. In addition, ANCOVA and Tukey’s multiple comparisons test was applied to test whether the region, year, and growth stage significantly influenced the intercept (single effect) and slope (interaction with the covariate) of the relationship between N uptake and NDVI2 for Test 2. Similarly, ANCOVA and Tukey’s multiple comparison test were used to assess whether the region and year significantly influenced the intercept and slope of the relationship between tiller number and NDVI. The explanatory variables could easily be significant when the sample size was large. Therefore, the effect size (ω2) was calculated to determine whether the explanatory variables had a substantial influence using the following equation (Kirk Citation1996):
where SSvar is the sum of squares of the explanatory variable, d.f. is the degrees of freedom, MSerr is the mean sum of squares of the residuals, and SStot is the total sum of squares. The effect of the explanatory variable is small if ω2 is less than 0.06 (Kirk Citation1996). All statistical analyses were performed using R software version 3.6.1 (R Core Team Citation2019).
3. Results
3.1. Test 1: Measurement conditions of NDVI at flag leaf appearance
The effect of measurement height on the relationship between N uptake and NDVI2 at the stage of flag leaf appearance is shown in and . N uptake was significantly fitted by NDVI2 at all measurement heights (P < 0.001; ). All R2 values were higher than 0.70, which suggested that N uptake could be estimated by the NDVI at all measurement heights. The highest R2 value was obtained at heights of 60, 90, and 120 cm. The ANCOVA results showed that the interaction between measurement height and NDVI2 (i.e. the effect of measurement height on the regression slope where x axis as NDVI2 and y axis as N uptake) was not significant but the single effect (i.e. the effect of measurement height on the intercept) was significant (P < 0.001, ). Therefore, the ANCOVA was performed again excluding the interaction. The single effect of measurement height was still significant (). The results of multiple comparisons revealed that the intercept was significantly lower at the height of 20 cm than at the other measurement heights (P < 0.05, ). On the other hand, the intercepts among the other measurement heights (60–120 cm) were not significantly different from each other.
Table 2. Summary of ANCOVA for N uptake = a + b× NDVI2 at the different measurement heights and angles where a and b are the intercept and slope, respectively
Figure 2. Relationship between nitrogen (N) uptake and normalized difference vegetation index (NDVI) at different measurement heights in the flag leaf period. Solid lines represent the fitted curve. Asterisks beside the coefficient of determination (R2) indicate the P-value of the regression equation (*** P < 0.001). Different letters beside the intercept value indicate a significant difference among the measurement heights (P < 0.05)
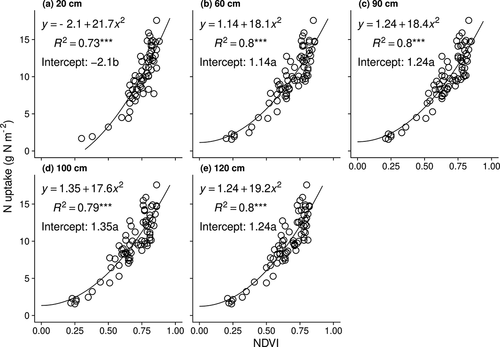
The effect of measurement angle on the relationship between N uptake and NDVI2 is shown in and . Similarly, N uptake was significantly fitted by NDVI2 at all measurement angles (P < 0.001; ). All R2 values were higher than 0.70 and the highest R2 value was obtained at the horizontal measurement. The ANCOVA results indicated that the interaction between measurement angle and NDVI2 (i.e. the effect of measurement angle on the regression slope where x axis as NDVI2 and y axis as N uptake) was not significant but the single effect (i.e. the effect of measurement angle on the intercept) was significant (). Therefore, the ANCOVA was performed again excluding the interaction. The single effect of measurement angle was still significant (). The intercept was significantly lower at the 45° angle (both along and orthogonal to the row) than at the horizontal measurement ().
Figure 3. Relationship between nitrogen (N) uptake and normalized difference vegetation index (NDVI) at different measurement angles in the flag leaf period. Solid lines represent the fitted curve. Asterisks beside the coefficient of determination (R2) indicate the P-value of the regression equation (*** P < 0.001). Different letters beside the intercept value indicate a significant difference among the measurement angles (P < 0.05)
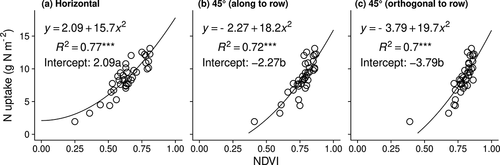
The NDVIs measured at different local times on the same date did not differ significantly ().
Figure 4. Comparison of normalized difference vegetation index (NDVI) measured between the daytime and the evening. The solid line represents the linear regression and the dashed line represents the 1:1 line. Asterisks beside the coefficient of determination (R2) indicate the P-value of the regression equation (*** P < 0.001)
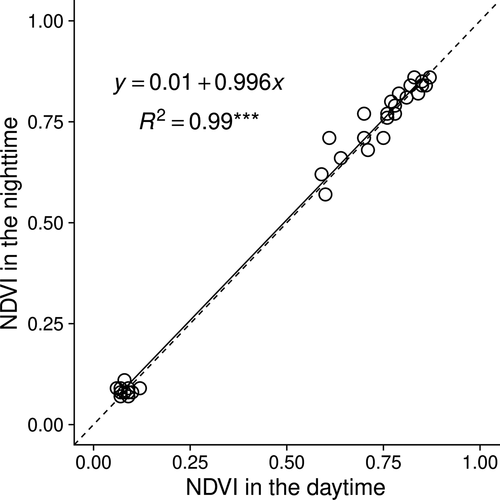
3.2. Test 2: Estimation of N uptake and tiller number using NDVI
Based on the results of Test 1, the NDVI was measured horizontally at heights of 90–100 cm above the canopy in the various experimental fields and the results are shown in and . N uptake was significantly fitted by NDVI2 without first-order term if the NDVI was lower than 0.75 ()). However, N uptake varied considerably in a similar NDVI if the NDVI was higher than 0.75. This relationship was also observed with the validation dataset ()). The root-mean-square error (RMSE) of the fitted model was 1.3 g N m−2 for the calibration and 2.0 g N m−2 for the validation () if the NDVI was lower than 0.75. The N uptake was also significantly fitted for calibration dataset by exponential equation and quadratic equation including first-order term of NDVI. However, the Radj2 was the highest and Akaike information criterion (AIC; Akaike Citation1987) was the lowest for the quadratic equation without first-order term ().
Table 3. Model comparison of fitting curves for N uptake by NDVI for calibration dataset if NDVI < 0.75. Radj2, AIC, and RMSE represent adjusted determination of coefficient, Akaike information criterion, and root-mean-square error (g N m−2), respectively. Higher Radj2, lower AIC, and lower RMSE represents better model
Table 4. Summary of ANCOVA for N uptake = a + b× NDVI2 at the different regions, years, and growth stages where a and b are the intercept and slope, respectively
Figure 5. Relationship between nitrogen (N) uptake and normalized difference vegetation index (NDVI) in different regions and years from the booting stage to flag leaf appearance. (a) calibration and (b) validation dataset. The solid and dotted lines represent the fitted curve and its 95% prediction interval. The vertical dashed line represents the NDVI of 0.75
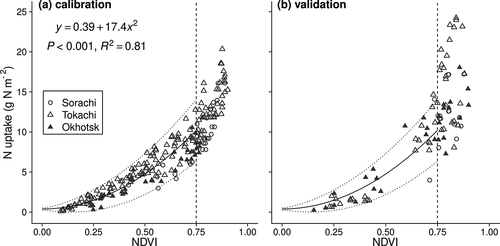
To test the effect of region, year, and growth stage on the relationship between N uptake and NDVI2, an ANCOVA was performed (). The ANCOVA results revealed that the single effect of region (P < 0.001), year (P < 0.001), and growth stage (P < 0.01) was significant, and the interaction between region and NDVI2 was also significant (P < 0.001). The significance of single effects of region, year, and growth stage was also obtained when the ANCOVA was performed again without interactions. However, the effect size (ω2) was less than 0.06 for all regions, years, and growth stages, which suggested that the effects of region, year, and growth stage on the relationship between NDVI2 and N uptake were extremely weak.
The effects of region and year on the relationship between tiller number and NDVI are shown in and . The tiller number was significantly fitted by NDVI at both stages of booting and panicle formation (). However, the tiller number at the booting stage varied considerably compared with that at panicle formation, resulting in lower accuracy at the booting stage (R2 = 0.68, RMSE = 512 tillers m−2) than that achieved at panicle formation (R2 = 0.84, RMSE = 318 tillers m−2). The ANCOVA results showed that the single effects of region and year were significant and their interactions with NDVI were also significant at the booting stage (). In addition, the effect size (ω2) was higher than 0.06 for the single effect of region and year and for the interaction between year and NDVI. Therefore, ANCOVA was not performed again excluding the interactions. The tiller number was higher in Tokachi in 2016 and 2017 than that in the other regions and years in the same NDVI (). In contrast, the ANCOVA results for tiller number at the panicle formation stage showed that the effect size (ω2) was less than 0.06 across regions and years, although the effects were significant (). The low effect sizes were also obtained when the ANCOVA was performed again without the interactions. Thus, with regard to the panicle formation stage, the effects of region and year on the relationship between the tiller number and NDVI were extremely small.
Table 5. Summary of ANCOVA for tiller number = a + b× NDVI at the different regions and years where a and b are the intercept and slope, respectively
Figure 6. Relationship between tiller number and normalized difference vegetation index (NDVI) at the stages of (a and b) booting and (c and d) panicle formation. (a) and (c) show the difference among regions, and (b) and (d) show the difference among years. Solid lines indicate the linear regression line and asterisks beside the coefficient of determination indicate the P-value of the regression equation (*** P < 0.001)
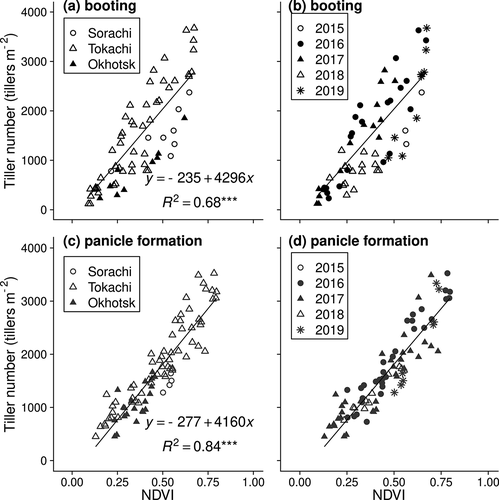
To investigate factors that contribute to regional and interannual differences in the relationship between tiller number and NDVI at the booting stage, regression analysis was performed between the residuals of the relationship (= estimated – measured tiller numbers) and the NDVI of bare soil. The residuals showed a significant positive correlation with the NDVI of bare soil ().
4. Discussion
4.1. Measurement conditions of NDVI
An active optical sensor emits light of two different wavelengths (infrared and red) and calculates the NDVI from the sum and the difference of the two reflectances. The reflectance is inversely proportional to the distance from the target leaves (Holland, Lamb, and Schepers Citation2012). Thus, the reflectance varies considerably with small changes in distance if the sensor is close to the canopy and might result in unstable measurement of the NDVI. In contrast, the reflected light is scattered if the sensor is far from the canopy and the reflection may be below the minimum detection limit. In this study, the sensor was held at 60–120 cm from the canopy and allowed stable measurement of the NDVI (). These results were in agreement with those of previous studies (Martin, López, and Lan Citation2012). Therefore, the NDVI must be measured at 60–120 cm height from the canopy.
The NDVI was increased if the NDVI sensor was inclined (). If inclined, the sensor might receive a greater amount of reflected light from culms as well as from leaves. Thus, the higher NDVI when the sensor was held at 45° angle might result from the inclusion of reflectance from culms.
In contrast to the measurement height and angle, the local time of day did not affect NDVI measurement (). This result indicates that the active optical sensor is robust to changes in ambient radiation because the sensor emits light actively and measures the reflectance. This result suggests that a stable NDVI will be measured under both sunlit and cloudy conditions.
4.2. Estimation of N uptake by NDVI
Region, year, and growth stage significantly affected the intercept of the relationship between N uptake and NDVI2 (). However, their effect sizes (ω2) were small. In the case of the effect of regions on intercept, for example, a priori test shows that the sample size needs to be larger than 158 to obtain ω2 > 0.06 under the condition of significance level (α) = 0.05, statistical power (1 − β) = 0.8 (Cohen Citation1988), the number of treatment = 6, the number of covariate = 1 (only NDVI2), and degree of freedom = 2. In this study, the sample size was 175, which was larger than the required sample size of 158. However, the actual effect size (ω2) of the regions was 0.05 and the actual statistical power (1 − β) was 0.775, which was smaller than the required power of 0.8. Therefore, the effect of regions on intercept substantially produced only minor differences in the relationship between N uptake and NDVI2. The other single effects and their interactions were similar. Therefore, the region, year, and growth stage can be ignored for simplicity.
N uptake could be predicted from the NDVI if the NDVI was less than 0.75 (). In previous papers, N uptake was fitted by an exponential equation (Moges et al. Citation2004; Holzapfel et al. Citation2009; Zhang et al. Citation2019). In this study, the best-fitted model was quadratic equation without first-order term followed by the exponential equation, and the quadratic equation including first-order term (). The better estimation accuracy of the exponential equation than the quadratic equation including first-order term was also found in previous papers (Cao et al. Citation2016; Ali, Ibrahim, and Bijay-Singh Citation2019). On the other hand, the quadratic equation without first-order term has not been considered in previous papers. The quadratic equation without first-order term can express both the moderate increase in N uptake when NDVI is low and the steep increase when NDVI is high as compared with the quadratic equation including first-order term. This behavior of the quadratic equation without first-order term is similar to the exponential equation. In fact, RMSE of these two estimation equations was almost similar to each other. Here, the quadratic equation without first-order term was used instead of the exponential equation with an emphasis on practical uses because the RMSE of the quadratic equation without first-order term was slightly better not only for calibration dataset but also for validation dataset compared with the exponential equation.
Farmers typically adjust N fertilization rates 2–3 g N m−2 at the stage of flag leaf appearance. Thus, the estimation accuracy needs to be lower than 2 g N m−2 in practice. The quadratic equation showed that the RMSE was 2.0 g N m−2 for the validation dataset (), indicating that the accuracy of the quadratic estimation was the acceptable upper limit at the least. Therefore, the handheld NDVI sensor can be applied for practical uses as the decision-making of additional N fertilization at the stage of flag leaf appearance.
In contrast with NDVI < 0.75, the NDVI was saturated and estimation of N uptake was difficult if the NDVI was higher than 0.75. This saturation was in agreement with the results of previous studies (Solari et al. Citation2008; Nishida et al. Citation2018; Gianquinto et al. Citation2019; Zhang et al. Citation2019). Zhang et al. (Citation2019) reported that the NDVI was saturated when the N uptake of winter wheat attained about 9 g N m−2. In the present study, the estimated N uptake was 10.2 g N m−2 when the NDVI was 0.75 (). N uptake higher than 9–10 g N m−2 suggests that the leaf area is substantially increased. If the leaf area is sufficiently high, the emitted light from the active sensor and the reflected light from leaves are scattered in the canopy and, as a consequence, insufficient reflected light will be detected by the sensor. Therefore, the NDVI was saturated and did not increase more than 0.75 even though N uptake was higher than 9–10 g N m−2. Based on the above discussion, N uptake can be estimated only when the NDVI is lower than 0.75.
4.3. Estimation of tiller number by NDVI
Tiller number could be estimated from the NDVI at the stage of panicle formation across regions and years () with RMSE of 318 tillers m−2. However, the relationship between tiller number and NDVI at the booting stage varied substantially among regions and years ()) with RMSE of 512 tillers m−2. Tiller number is used to decide whether the additional N fertilizer is applied at the booting stage or not. In eastern Hokkaido, if the tiller number was less than 1,000 tillers m−2, the additional N fertilizer of 2–4 g N m−2 can be applied at the booting stage and the remains need to be applied at the stage of panicle formation. In central Hokkaido, however, the threshold of the tiller number at the booting stage is 800–1,300 tillers m−2; the difference is 500 (= 1,300 − 800) tillers m−2. Therefore, the RMSE of 512 tillers m−2 is not acceptable to estimate tiller number at the booting stage. Regional and interannual differences in the relationship have also been observed in Virginia, USA (Phillips et al. Citation2004). Also, previous papers show that soil type and soil moisture influence the NDVI readings (Nicholson and Farrar Citation1994; Jones et al. Citation2015). At the booting stage, the wheat canopy has not yet covered the soil surface. Thus, reflectance from bare soil might influence the NDVI measured from above the wheat canopy and resulted in a positive correlation between the residuals of tiller number and the NDVI of bare soil (). Therefore, it might not be feasible to estimate the tiller number from the NDVI at the booting stage.
5. Conclusion
An active handheld optical sensor for estimation of the NDVI may be useful for prediction of N uptake by winter wheat at the developmental stages from booting to flag leaf appearance and for estimation of tiller number at the panicle formation stage. The results of this study enable simple and rapid estimation of N uptake and tiller number, and can contribute to the rapid decision-making for N fertilization management of winter wheat. In contrast, if the NDVI is higher than 0.75, the current method (number of superior stems and SPAD) needs to be used to estimate N uptake at the stage of flag leaf appearance.
Acknowledgments
The authors would like to thank the Japan Agricultural Cooperatives, Hokkaido Central Union for funding this work. We are grateful to advisers in the agricultural extension center and to the employees in the local agricultural cooperatives who assisted with the experiments and investigation. Furthermore, we would also like to express our deep gratitude to all the field owners, who readily allowed us to conduct the field experiments and investigation for this work. We thank Robert McKenzie, PhD, from Edanz Group (www.edanzediting.com/ac), for editing a draft of this manuscript.
Disclosure statement
No potential conflict of interest was reported by the authors.
References
- Akaike, H. 1987. “Factor Analysis and AIC.” Psychometrika 52 (3): 317–332. doi:https://doi.org/10.1007/BF02294359.
- Ali, A. M., S. M. Ibrahim, and Bijay-Singh. 2019. “Wheat Grain Yield and Nitrogen Uptake Prediction Using atLeaf and GreenSeeker Portable Optical Sensors at Jointing Growth Stage.” Information Processing in Agriculture 7 (3): 375–383.
- Cao, Q., Y. Miao, J. Shen, W. Yu, F. Yuan, S. Cheng, S. Huang, H. Wang, W. Yang, and F. Liu. 2016. “Improving In-season Estimation of Rice Yield Potential and Responsiveness to Topdressing Nitrogen Application with Crop Circle Active Crop Canopy Sensor.” Precision Agriculture 17 (2): 136–154. doi:https://doi.org/10.1007/s11119-015-9412-y.
- Cohen, J. 1988. Statistical Power Analysis for the Behavioral Sciences. 2nd ed. Hillsdale, New Jersey: Lawrence Erlbaum Associates, Publishers.
- Crusiol, L. G. T., F. C. Carvalho J de, R. N. R. Sibaldelli, W. Neiverth, A. Do Rio, L. C. Ferreira, O. Procópio S de, et al. 2017. “NDVI Variation according to the Time of Measurement, Sampling Size, Positioning of Sensor and Water Regime in Different Soybean Cultivars.” Precision Agriculture 18 (4): 470–490. doi:https://doi.org/10.1007/s11119-016-9465-6.
- Department of Agriculture Hokkaido Local Government 2015: Hokkaido Fertiliser Recommendations 2015.
- Diacono, M., P. Rubino, and F. Montemurro. 2013. “Precision Nitrogen Management of Wheat. A Review.” Agronomy for Sustainable Development 33 (1): 219–241. doi:https://doi.org/10.1007/s13593-012-0111-z.
- Fueki, N., R. Nakamura, A. Sawaguchi, K. Watanobe, T. Suzuki, T. Uchida, and M. Onodera. 2015. “Prediction Of Nitrogen Uptake By Winter Wheat (Triticum aestivum L.) by measurement of superior stem number and leaf color value, for decision-making regarding additional nitrogen fertilization.” Soil Science and Plant Nutrition 61 (5): 769–774. doi:https://doi.org/10.1080/00380768.2015.1021235.
- Gianquinto, G., F. Orsini, G. Pennisi, and S. Bona. 2019. “Sources of Variation in Assessing Canopy Reflectance of Processing Tomato by Means of Multispectral Radiometry.” Sensors (Switzerland) 19 (21): 10–15. doi:https://doi.org/10.3390/s19214730.
- Holland, K. H., D. W. Lamb, and J. S. Schepers. 2012. “Radiometry of Proximal Active Optical Sensors (AOS) for Agricultural Sensing.” IEEE Journal of Selected Topics in Applied Earth Observations and Remote Sensing 5 (6): 1793–1802. doi:https://doi.org/10.1109/JSTARS.2012.2198049.
- Holzapfel, C. B., G. P. Lafond, S. A. Brandt, P. R. Bullock, R. B. Irvine, M. J. Morrison, W. E. May, and D. C. James. 2009. “Estimating canola (Brassica napus L.) yield potential using an active optical sensor.” Canadian Journal of Plant Science 89 (6): 1149–1160. doi:https://doi.org/10.4141/CJPS09056.
- Jones, J. R., C. S. Fleming, K. Pavuluri, M. M. Alley, M. S. Reiter, and W. E. Thomason. 2015. “Influence of Soil, Crop Residue, and Sensor Orientations on NDVI Readings.” Precision Agriculture 16 (6): 690–704. doi:https://doi.org/10.1007/s11119-015-9402-0.
- Kirk, R. E. 1996. “Practical Significance: A Concept Whose Time Has Come.” Educational and Psychological Measurement 56 (5): 746–759. doi:https://doi.org/10.1177/0013164496056005002.
- Martin, D. E., J. D. López, and Y. Lan. 2012. “Laboratory Evaluation of the GreenSeekerTM Hand-held Optical Sensor to Variations in Orientation and Height above Canopy.” International Journal of Agricultural and Biological Engineering 5: 43–47.
- Ministry of Agriculture F and F of J. 2018. “Statistical Survey on Crops.”
- Moges, S. M., W. R. Raun, R. W. Mullen, K. W. Freeman, G. V. Johnson, and J. B. Solie. 2004. “Evaluation of Green, Red, and near Infrared Bands for Predicting Winter Wheat Biomass, Nitrogen Uptake, and Final Grain Yield.” Journal of Plant Nutrition 27: 1431–1441.
- Nicholson, S. E., and T. J. Farrar. 1994. “The Influence of Soil Type on the Relationships between NDVI, Rainfall, and Soil Moisture in Semiarid Botswana. I. NDVI Response to Rainfall.” Remote Sensing of Environment 50 (2): 107–120. doi:https://doi.org/10.1016/0034-4257(94)90038-8.
- Nishida, M., M. Namikawa, H. Kudo, Y. Ohdaira, and T. Takahashi. 2018. “Relationship between Nitrogen Status in Rice Plants (Oryza Sativa L.) Of Super-heavy Panicle Type Cultivar “Iwaidawara” and NDVI Readings Temporally Measured at Different Heights by Hand-held Active NDVI Sensor.” Japanese Journal of Soil Science and Plant Nutrition 89: 146–151.
- Phillips, S. B., D. A. Keahey, J. G. Warren, and G. L. Mullins. 2004. “Estimating Winter Wheat Tiller Density Using Spectral Reflectance Sensors for Early-spring, Variable-rate Nitrogen Applications.” Agronomy Journal 89 (3): 591–600. doi:https://doi.org/10.2134/agronj2004.0591.
- R Core Team. 2019. R: A Language and Environment for Statistical Computing. Vienna, Austria: R Foundation for Statistical Computing.
- Sasaki, F., N. Murakami, and S. Hayashi. 2014. “Characteristics and Utilization for Mobile NDVI Sensor.” Japanese Journal of Farm Work Research 49 (4): 155–161. doi:https://doi.org/10.4035/jsfwr.49.155.
- Solari, F., J. Shanahan, R. Ferguson, J. Schepers, and A. Gitelson. 2008. “Active Sensor Reflectance Measurements of Corn Nitrogen Status and Yield Potential.” Agronomy Journal 100 (3): 571–579. doi:https://doi.org/10.2134/agronj2007.0244.
- Zhang, J., X. Liu, Y. Liang, Q. Cao, Y. Tian, Y. Zhu, W. Cao, and X. Liu. 2019. “Using a Portable Active Sensor to Monitor Growth Parameters and Predict Grain Yield of Winter Wheat.” Sensors (Switzerland) 19. doi:https://doi.org/10.3390/s19051108.