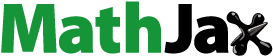
ABSTRACT
This study aims to provide energy-efficient strategies for collections care in the face of financial and environmental challenges. Focusing on paper-based enclosures in storage rooms, the research proposes strategies to enhance energy efficiency by relaxing tight environmental controls. The five-stage process began with a standard test of hygrothermal properties and buffering capacity of the enclosures. In the second stage, a computer model was developed using the first stage results. This model was used in a series of specially designed tests simulating the transient heat, air, and moisture transfer between the room and enclosure to determine an acceptable room environment in which the enclosure conditions could meet collections care standards. The modelling results were used, in the third stage, to develop an AI model that predicted the enclosure conditions using room temperature and relative humidity (RH) inputs. In the final stage, a model for energy consumption of air-conditioning system operations was developed to predict across various control accuracies. Results indicate that the tight maintenance of room RH levels can be relaxed from 47–53% to 33–65%, with a corresponding reduction in energy consumption of approximately 16–18%. This relaxation, while maintaining enclosure conditions in line with conservation standards, was demonstrated through a simulation case at the National Library of Scotland. This study underscores the broader applicability of the developed procedure, asserting its relevance for cultural institutions seeking energy-saving potential through customized enclosure models.
Este estudio tiene como objetivo proporcionar estrategias energéticamente eficientes para el cuidado de las colecciones frente a los desafíos financieros y ambientales. Centrándose en los recintos de papel en las salas de almacenamiento, la investigación propone estrategias para mejorar la eficiencia energética al relajar los estrictos controles ambientales. El proceso de cinco etapas comenzó con una prueba estándar de las propiedades higrotérmicas y la capacidad amortiguadora de los recintos. En la segunda etapa, se desarrolló un modelo informático utilizando los resultados de la primera etapa. Este modelo se utilizó en una serie de pruebas especialmente diseñadas para simular la transferencia transitoria de calor, aire y humedad entre la habitación y el recinto para determinar un entorno ambiental aceptable en el que las condiciones del recinto pudieran cumplir con los estándares de cuidado de las colecciones. Los resultados del modelado se utilizaron, en la tercera etapa, para desarrollar un modelo de IA que predijo las condiciones del recinto utilizando entradas de temperatura ambiente y humedad relativa (RH). En la etapa final, se desarrolló un modelo para el consumo de energía de las operaciones del sistema de aire acondicionado para predecir varias precisiones de control. Los resultados indican que el estricto mantenimiento de los niveles de humedad relativa de la habitación se puede relajar del 47 al 53% al 33 al 65%, con una reducción correspondiente en el consumo de energía de aproximadamente el 16 al 18%. Esta relajación, mantienendo condiciones del recinto de acuerdo con los estándares de conservación, se demostró mediante un caso de simulación en la Biblioteca Nacional de Escocia. Este estudio subraya la aplicabilidad más amplia del procedimiento desarrollado, afirmando su relevancia para las instituciones culturales que buscan potencial de ahorro de energía a través de modelos de cerramiento personalizados.
Introduction
Throughout Europe, numerous heritage collections are housed within historic buildings with significant cultural value (Bülow, Colston, and Watt Citation2002). Preserving these collections, while enhancing operational energy efficiency, poses a considerable challenge. Implementing changes to the construction of existing historic buildings is often a complex undertaking. Alongside this, collections care professionals are challenged to safeguard cultural heritage without risking the natural environment. Passive environmental control emerges as a viable option to effectively mitigate carbon emissions while preserving stored heritage collections (Bohm et al. Citation2001). National Library of Scotland is working to achieve the Scottish Government’s Net Zero strategy 2045 (Net Zero Scotland Citation2019).
The studies reviewed show the potential for energy saving through passive environmental control. The buffering effect of six common storage enclosures (cardboard and plastic polypropylene boxes, mobile rack of shelves, some drawers with tight sealing or non-sealing) is found to be associated with reduced environmental fluctuations inside the enclosures, especially regarding the relative humidity (RH) in an in situ test (Rojas, Cabrera, and Corona Citation2020). The buffering effect, due to the ‘box in box’ configuration, is recommended to relax the tight environmental control in a theoretical study (Perino Citation2018). Relaxing the control band from 48–52% RH to 40–60% RH was predicted to yield a saving of about 40% of annual energy in a museum in a computer modelling study (Ascione et al. Citation2009).
These studies show that there are three separate topics: the buffering effect of the enclosure, the feasibility of relaxing the tight control, and the potential for energy saving, all with the same aim of sustainable collections care. However, there is a lack of integration to combine these three topics. An integration study, from modelling the buffering effect and hygrothermal interaction between macro-environment in the room and micro-environment in the enclosure to predicting energy consumption under various levels of control accuracy, could allow for fine-tuning of control to explore the relationship among control accuracy, micro-environmental conservation quality, and consequent energy consumption.
The enclosures in the National Library of Scotland were folded with one piece of acid-free cardboard, with full lengths overlaid on three sides when closed (). The acid-free cardboard, with a thickness of 1.14 mm, was purposely fabricated in three layers of polypropylene and contains ground calcium carbonate and cotton pulp supplied by (Klug Conservation Citation2023).
In our previous experimental study (Han et al. Citation2024), we measured hygrothermal properties of the cardboard and buffering capacity of the enclosure, with preliminary results supporting the research presented in this paper. Measured properties include thermal diffusivity, specific heat, equilibrium moisture content, water vapour diffusion resistance coefficient, and water vapour diffusion coefficient. They were used in creating a numerical model to simulate transient heat air moisture (HAM) transfer between the micro-environment and macro-environment. Additionally, the tested enclosure was intentionally left empty to measure its actual capacity without any interference from the contents. The results provided validation data for the heat, air and moisture (HAM) model, quantified the buffering capacity of the enclosure, and demonstrating its ability to effectively mitigate the micro-environmental temperature and RH fluctuations. This finding forms the basis for relaxing the macro-environmental control. Specifically, for temperature fluctuations of 5°C within the range of 17–22°C and constant 50% RH over a period of <180 min, the micro-environmental fluctuations were 1–2°C smaller than the macro-environmental ones. Similarly, for humidity fluctuations of 10% RH within 50–60% RH and a fixed temperature of 20°C over a period of <600 min, the micro-environmental fluctuations were 2–8% RH smaller than macro-environmental ones.
Based on the outcomes of our experimental study, this study aims to improve energy efficiency by relaxing the tight macro-environmental controls while the micro-environment complies with conservation standards. There are three objectives. The first is to model the transient (HAM) transfer between the micro-environment and macro-environment, sequentially, to determine an acceptable macro-environment when the micro-environment complies with collections care standards. The second is to develop a real-time prediction model as an agent of the transient HAM simulation. Finally, the third objective is to predict energy consumption at various levels of control accuracy.
Methodology
illustrates a three-stage approach, detailed in an accompanying methodological framework. Leveraging findings from the previous experimental study, it provided hygrothermal properties of cardboard enclosures and buffering capacity test data for HAM model creation and validation, respectively. In the first stage, the HAM model was created by using commercial software, COMSOL Multiphysics. Sequentially, this model was used to determine an acceptable macro-environment for implementing the relaxed control. In the second stage, an artificial neural network (ANN) model was trained using data from the HAM simulation, covering various typical macro-environmental conditions. This model enabled real-time prediction of micro-environment. In the third stage, an energy consumption model was developed using the transfer-function mathematical method to describe the operational characteristics of the air-conditioning system. This model provided energy consumption predictions across various control accuracies.
HAM simulation model
illustrates a 2D cross-section of the enclosure in the simulation model, setting an 80 mm depth in the orthogonal direction. The thickness of cardboard is 1.14 mm. There are two 2 mm gaps. An airflow velocity of 0.2 m/s was set on the external surface of the enclosure, oriented orthogonally. This velocity aligns closely with values typically observed in on-site measurement. Natural flow through the gaps was simulated using an open boundary, with the airflow considered weakly compressive, given that air density primarily depends on temperature. The time-series macro-environmental temperature and RH were used as boundary condition surrounding the enclosure.
The HAM model describes the interdependence of heat air and moisture transfer, encompassing heat and moisture balance, heat and air convection balance, and heat and air convection balance. displays the hygrothermal properties of the cardboard, as measured in the preceding study by Han et al. (Citation2024). In this context, to present the equilibrium moisture content (EMC) in modelling, the associated date previously measured within 15–25°C and 33∼75% RH was fitted to obtain a function, .
Table 1. Material properties.
The associated validation data were collected in both tight and relaxed control conditions to ensure that this model was robust enough to reproduce the HAM transfer in this research. Testo 174H temperature and humidity loggers (range: −20 to 70°C, 0–100% RH; accuracy: ±0.5°C, ±3% RH) were used to measure the micro and macro environment. The sampling interval was 15 min for both the tight and relaxed control.
The HAM simulation is highly time-consuming, typically running in hours, so it is not possible to use it in the air conditioning system with a feedback control loop operated on a scale of minutes. It is necessary to create an agent model to predict the micro-environmental temperature and RH in real time. Based on the onsite temperature and RH data collected in tight macro-environmental control, four sets of data were generated to represent four conditions in broadened control bands. Sequentially, they were fed into the HAM model for calculating the micro-environmental data. The micro-environmental and macro-environmental data were respectively used as input and output to train the agent model, ANN.
ANN
The training data is formed of a sequence of features over a set period rather than independent data points. Learning to store effective information within a time sequence is a challenge for common ANNs. Long short-term memory (LSTM) network, a type of sequence-to-sequence ANN, was designed to overcome this challenge (Hochreiter and Schmidhuber Citation1997). The LSTM block effectively manages memory by leveraging the input gate, forget gate, and output gate to control the flow of information, ensuring the capture and retention of relevant data in sequence (Yu et al. Citation2019).
Five sets of data represent the macro and micro environments, spanning from tight control to relaxed control within the qualified condition for preventive conservation. One dataset was from on-site test, representing the tight control condition, while four sets of simulation data was generated by HAM simulation, representing various levels of relaxed control conditions. Together, these five datasets form a comprehensive data package that captures crucial features of transient HAM transfer between micro and macro environments. In this data package, the input of the LSTM was macro-environmental temperature and RH, and the target output was the corresponding micro-environmental temperature and RH. The training dataset was divided into two parts; train set (the first 90% data) and test set (the remaining 10% data) (Arslan and Sekertekin Citation2019; Granata, Di Nunno, and de Marinis Citation2022). Data normalization was used in data processing to avoid distorting differences in the ranges of values (Salem et al. Citation2022). shows the structure and parameters of the LSTM network. The ANN training was run on a common computer equipped with a 1.6 GHz CPU and 16GB RAM.
Table 2. The structure and parameters of the LSTM network.
Energy consumption model
The energy consumption model included three parts: controller, normal HVAC components (cooling coil connected with chiller, electric heater, and electric steam humidifier), and room. This model was developed in MATLAB/Simulink. The controller used a common ON/OFF control method for obtaining quasi-continuous output. Its signal includes a series of pulses produced on a fixed period () and controls the ON time (
) of the pulses for all components (Salsbury Citation2002). The mathematical model is given as Equations (1)–(2).
(1)
(1)
The input to the system is given as
(2)
(2) where
is the percentage of duty ratio,
is the input signal,
is the sample number. Normally, these parameters are tuned by the manufacturer.
The component and room models describe the response characteristics for the HVAC equipment and the room hygrothermal behaviour. All mathematical descriptions use transfer functions that represent a system’s input-output relationship (Afram et al. Citation2018).
A 100m2 inner storage room, without any external windows or doors, on an intermediate floor was used for the simulation. This storage room was a typical case representing the library’s strongroom. Historical building performance information was in reference to an ARUP (Citation2017) industrial report. The U values are 0.15, 0.75 W/m2·K for wall and floor. Room loads were calculated based on four typical days of the dataset (hourly temperature and RH fluctuations: about ≤±2∼4°C and ≤±8–21% RH) which represents the feature of four seasons for this storage room in Edinburgh. The control levels were set at ±0.5°C, ±3% RH (tight control), ±2°C, ±10% RH, and ±3°C, ±16% RH (relaxed control).
Results and discussion
illustrates a comparison between measured and simulated microenvironmental temperature and RH. In tight control, absolute errors are <2.5% RH and <0.5°C; in relaxed control, <2% RH and <0.2°C. Simulated trends align closely with measured data, affirming a high accuracy of the HAM model in capturing heat, air, and moisture transfer between micro- and macro-environments.
In addition, as illustrated in , the macro-environmental RH can be relaxed to 33∼65% RH control band with ±16% RH fluctuation over 24 h in the most relaxed condition. Meanwhile, the micro-environmental RH can be maintained in 43–57%RH with <±9% RH fluctuation over 24 h (<±5% RH for 98% of the time). This micro-environment complies with the industrial standards outlined in BS EN 16893 (Citation2018) and the ASHRAE Handbook (Citation2019), which recommend a control band of 40–60% RH with a fluctuation over 24 h of ±10% RH. Therefore, this study suggests there is the potential to relax the currently tight macro-environmental controls. As the conservation sector seeks to develop sustainable environmental parameters, the results of this study demonstrate that it is possible to relax macro-environmental controls without compromising the preventive conservation quality in the micro-environment. However, the theoretical model developed here would need to be adapted and modified to fit the specific nature of different buildings, collections, and storage environments. The crucial aspect here lies in integrating the passive air-conditioning offered by the enclosure with the active air conditioning supplied by the mechanical system, aiming to enhance energy efficiency while maintaining control over the micro-environment.
Table 3. The results of relaxing the environment.
Within the acceptable macro-environment, the ANN can accurately forecast the 24-hour micro-environmental temperature and RH, thereby ensuring the maintenance of a high-quality preventive conservation standard. shows the performance results of the LSTM network. All values of R2 (coefficients of determination) are close to 1 and the values of (mean square error),
(root mean square error),
(mean absolute error) and SD (standard deviation of the errors) are near 0. High precision shows in both training and testing data, which indicates the trained network has a good generalization performance without underfitting (insufficient training to capture data feature) and overfitting (overtraining to capture excessive data feature) problems. Moreover, the temperature errors are smaller than ±0.2°C and ±0.1°C while the RH errors are smaller than ±3% RH and ±2% RH in training and testing data, respectively. In addition, the prediction time was less than 5 s for daily data (96 samples with 15 min interval in 1.6 GHz CPU with 16GB RAM). Therefore, it is an accurate real-time prediction model for relaxing tight macro-environmental control.
Table 4. Performance results of the LSTM network.
Based on the data presented in , relaxing the control accuracy can lead to a decrease in energy consumption. In a 100 m² storage room within a historical building, equipped with a cooling chiller, electric heater, and humidifier, the macro-environmental control accuracy was relaxed from ±0.5°C and ±3% RH to ±2 °C and ±10% RH (relaxed control 1), then to ±3 °C and ±16% RH (relaxed control 2). As a result, energy savings range from 5.4 to 32.3 kWh and 5.8 to 33 kWh, with associated energy-saving ratios of 15.9–18.5% and 17.1–18.9% for these two relaxation levels, respectively. Such degree of energy saving is achievable with the common air-conditioning system operating in Edinburgh's moderate climate. Importantly, this relaxed control strategy is applicable across diverse system configurations, buildings, and climate zones. It unlocks opportunities for implementing highly efficient measures previously restricted by stringent environmental control demands. For instance, it would facilitate the adoption of sensible and latent heat recovery ventilation and the substitution of traditional cooling dehumidification with desiccant wheel.
Table 5. Daily energy consumption in tight and relaxed control.
In addition, gradients of energy-saving potential range from 3.6 to 21.5 kWh/°C and 0.8 to 4.6 kWh/%RH between tight control and relaxed control 1, and from 2.3 to13.2 kWh/°C and 0.4 to 2.5 kWh/%RH between tight control and relaxed control 2. It is worth noting that the energy-saving potential significantly diminishes when the control accuracy is relaxed beyond ±2 °C and ±10% RH (relaxed control 1), which is attributed to building performance and ambient fluctuations in this simulation case. Hourly ambient fluctuations typically range from ≤±2 °C and ±21% RH in spring to ≤±4 °C and ±8% RH in winter, hovering around ±2 °C and ±10% RH per hour on average. Although relaxed control 2 allows for setting of ±3 °C and ±16% RH, the macro-environmental fluctuations were generally more stable than the set values. Compared to this simulation case, greater potential for implementing the relaxing control lies in conditions characterized poor-performance buildings and large ambient fluctuations. However, when employing the relaxing control in specific scenarios, it needs tuning with passive micro-environmental control inside the enclosure with active macro-environmental control in the storage room.
Conclusions
In this paper, the hygrothermal property of the enclosure was tested for HAM model development and the temperature and RH in the micro-environment and macro-environment were collected for model validation. The model can reproduce the HAM transfer between micro-environment and macro-environment accurately. Based on the HAM simulation, an acceptable macro-environment was determined when the micro-environment complies with collections care standards. The macro-environment can be relaxed to 33–65% RH control band with ±16% RH fluctuation in 24 h in the most relaxed condition. The LSTM network was proposed to achieve real-time predictions by training input and output data from the simulation. In addition, the energy consumption model simulated the system operation in various levels of control accuracy and proved a reduction in energy consumption by approximately 16% to 18%. This promising reduction highlights the potential for relaxing control to achieve energy savings.
There are three significant benefits from this research: the acceptable macro-environment provides an opportunity to ease mechanical stress in air conditioning plant; the LSTM real-time prediction allows for micro-environmental monitoring without deploying extra sensors; and this relaxed control scheme can be used for other libraries and museums in other climate regions or using different kinds of enclosure in their storage rooms.
Furthermore, the approach is not limited to libraries. It could be applied in other institutions to develop bespoke energy-saving programmes suitable for different circumstances.
However, two limitations exist in this study. First, the buffering capacity assessment focused solely on newly made enclosures, overlooking potential changes over their lifespan. Investigating the effect in association with enclosure buffering performance degradation would be pertinent and insightful. Second, the study analysed individual boxes in isolation, neglecting the potential impact of a fully boxed storeroom. Future research could explore the buffering effect in such environments. They could be resolved in future studies.
Disclosure statement
No potential conflict of interest was reported by the author(s).
Additional information
Funding
References
- Afram, A., A. S. Fung, F. Janabi-Sharifi, and K. Raahemifar. 2018. “Development and Performance Comparison of Low-Order Black-Box Models for a Residential HVAC System.” Journal of Building Engineering 15: 137–155. https://doi.org/10.1016/j.jobe.2017.11.021.
- Arslan, N., and A. Sekertekin. 2019. “Application of Long Short-Term Memory Neural Network Model for the Reconstruction of MODIS Land Surface Temperature Images.” Journal of Atmospheric and Solar-Terrestrial Physics 194: 105100. https://doi.org/10.1016/j.jastp.2019.105100.
- ARUP. 2017. Museums and Galleries (U-Value Guide). Accessed November 2017. https://museumdevelopmentnorthwest.files.wordpress.com/2012/06/arup-u-values-guide_single-pg_issue-21-march-2017dble-pg-spread1-final-copy.pdf.
- Ascione, F., L. Bellia, A. Capozzoli, and F. Minichiello. 2009. “Energy Saving Strategies in Air-Conditioning for Museums.” Applied Thermal Engineering 29 (4): 676–686. https://doi.org/10.1016/j.applthermaleng.2008.03.040.
- ASHRAE. 2019. ASHRAE 2019 Handbook: Heating, Ventilating and Air-Conditioning Applications (SI). With contributions from V. Beltran, C. Grzywacz, J. Linden, M. Lukomski, S. Michalski, J. Taylor, and J. Tetreault, 24.1-24.43. Atlanta: ASHRAE.
- Bohm, C. B., K. Zehnder, H. Domeisen, and A. Arnold. 2001. “Climate Control for the Passive Conservation of the Romanesque Painted Wooden Ceiling in the Church of Zillis (Switzerland).” Studies in Conservation 46 (4): 251–268. https://doi.org/10.1179/sic.2001.46.4.251.
- BSI. 2018. BS EN 16893:2018. Conservation of Cultural Heritage—Specifications for Location, Construction and Modification of Buildings or Rooms Intended for the Storage or Use of Heritage Collections. London: British Standards Institution.
- Bülow, A. E., B. J. Colston, and D. S. Watt. 2002. “Preventive Conservation of Paper-Based Collections Within Historic Buildings.” Studies in Conservation 47 (Supp. 3): 27–31. https://doi.org/10.1179/sic.2002.47.s3.006.
- Granata, F., F. Di Nunno, and G. de Marinis. 2022. “Stacked Machine Learning Algorithms and Bidirectional Long Short-Term Memory Networks for Multi-Step Ahead Streamflow Forecasting: A Comparative Study.” Journal of Hydrology 613A: 128431. https://doi.org/10.1016/j.jhydrol.2022.128431.
- Han, B., X. Li, F. Wang, J. Bon, and I. Symonds. 2024. “The Buffering Effect of a Paper-Based Storage Enclosure Made From Functional Materials for Preventive Conservation.” Indoor and Built Environment 33 (1): 167–182. https://doi.org/10.1177/1420326X23118.
- Hochreiter, S., and J. Schmidhuber. 1997. “Long Short Term Memory.” Neural Computation 9 (8): 1735–1780. https://doi.org/10.1162/neco.1997.9.8.1735.
- Klug Conservation. 2023 “Useful Knowledge No. 1 – Cotton or Chemical Wood Pulp? No. 4 – Fillers, No. 6 Polypropylene – A Material for Long-Term Archiving?” Accessed November 2023. https://www.klug-conservation.com/Useful-knowledge.
- Net Zero Scotland. 2019 About Net Zero. Accessed 14 May 2019. https://www.netzeronation.scot/the-importance-of-net-zero.
- Perino, M. 2018. “Air Tightness and RH Control in Museum Showcases: Concepts and Testing Procedures.” Journal of Cultural Heritage 34: 277–290. https://doi.org/10.1016/j.culher.2018.01.022.
- Rojas, A., F. Cabrera, and A. Corona. 2020. “Temperature and Relative Humidity in a Palaeontological Collection: The Buffering Effect of Microenvironments.” Annales de Paleontologie 106 (4): 104206. https://doi.org/10.1016/j.annpal.2020.102446.
- Salem, H., A. E. Kabeel, E. M. S. El-Said, and O. M. Elzeki. 2022. “Predictive Modelling for Solar Power-Driven Hybrid Desalination System Using Artificial Neural Network Regression with Adam Optimization.” Desalination 522: 115–411. https://doi.org/10.1016/j.desal.2021.115411.
- Salsbury, T. I. 2002. “A New Pulse Modulation Adaptive Controller (PMAC) Applied to HVAC Systems.” Control Engineering Practice 10 (12): 1357–1370. https://doi.org/10.1016/S0967-0661(02)00099-0.
- Yu, Y., X. Si, C. Hu, and J. Zhang. 2019. “A Review of Recurrent Neural Networks: LSTM Cells and Network Architectures.” Neural Computation 31 (7): 1235–1270. https://doi.org/10.1162/neco_a_01199.