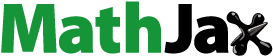
The authors state, “This book was written for students of the health sciences and serves as an introduction to the study of biostatistics – the use of numbers and numerical techniques to extract information from data and facts, and to then use this information to communicate scientific results.”
They go on to add, “Some say that statistics is the study of variability and uncertainty. We believe there is truth to this adage and have used it as a guide to divide the book into three parts covering the basic principles of VIP: (1) variability, (3) inference, and (2) probability.”
Part I: Variability Chapters 2–4
“Descriptive statistics, the topic of Chapter 2, are methods for organizing and summarizing a set of measurements.” Chapter 3 (Rates and Standardization) deals exclusively with measurements that assume only two values. Chapter 4 introduces the classical life table, one of the most important numericalsummary techniques available in the health sciences. “Life tables are used by public health professionals to characterize thewell-being of a population, and by insurance companies to predict how long individuals will live. In this chapter, the study of mortality begun in Chapter 3 is extended to incorporate the actual time to death for each individual, resulting in a more refined analysis.”
“Together, Chapters 2 through 4 demonstrate that the extraction of information from a collection of measurements is not precluded by the variability among those measurements.”
Part II: Probability Chapters 5–8
“Although it is not practical in its pure form, its basic principles – which we investigate in Chapter 5 – can be applied to provide a means of quantifying uncertainty. An important application of probability theory arises in medical screening and diagnostic testing, as we see in Chapter 6.”
Chapters 7 has brief descriptions of well-known probability distributions and Chapter 8 discuses sampling and inference distributions with emphasis on the Central Limit Theorem.
Part III: Chapters 9–22 Inference
“On the basis of the sample, we draw conclusions about the entire population, including the part of the population we did not measure – those not in the sample,” the authors offer. The reviewer likes to add that inference is based on a sample and statistical theory.
The list of topics organized into chapters is somewhat standard and since there are quite a few the treatment tends to be brief and to the point. In the list below key topics in the chapters are identified, but these are not exhaustive.
Confidence Intervals: one-sided, two-sided, student’s t
Hypothesis Testing: one-sided, two-sided, errors, power, sample size estimation
Comparison of Two Means: paired, independent, sample size estimation
ANOVA: One-way, multiple comparison procedures (Bonferroni only; no HSD)
Nonparametric Methods: Sign test, Wilcoxon, Kruskal-Wallace, advantages and disadvantages
Inference on Proportions: normal approximation, exact methods, Wilson interval, two proportions
Contingency Tables: Chi-square test, McNemar’s test, odds ratio, Berkson’s fallacy
Correlation: Scatter plot, Pearson, Spearman rank correlation coefficient
Simple Linear Regression: the model, inferences, model evaluation
Multiple Linear Regression: Least Squares, inferences on coefficients, indicator variables, model selection and evaluation
Logistic Regression: the dichotomous response model, indicator variables, Simpson’s paradox
Survival Analysis: Life table method, product limit (Kaplan-Meier) method, log-rank test, Cox proportional hazards model
Sampling Theory: SRS, systematic, stratified, cluster, ratio estimator, two-stage cluster sampling, sources of bias
Study Design: Randomized studies and observational studies.
The reviewer found Chapters 2 (Rates and Standardization) and 3 (Life Tables) to be of special interest since they are not completely standard additions to statistics texts. The authors do an excellent job of motivating this material. Useful graphs and their interpretation are generously sprinkled within these chapters. Life tables as a predictor of longevity along with mean and median survival are described. The superimposed life table curves for first century Rome, London, and Breslau are quite interesting (over vastly different time periods) and well illustrate age specific mortality rates and their interpretation.
The reviewer will use the Chapter 19 (Logistic Regression) to illustrates the authors’ methods.
“There are many situations, however, in which the response of interest is dichotomous rather than continuous. Examples of variables that assume only two possible values are disease status (disease is either present or absent) … In general, the value 1 is used to represent a “success,” or the outcome we are most interested in, and 0 represents a “failure.” The mean of the dichotomous random variable Y, designated p, is the proportion of times that Y takes the value 1. Equivalently,
Just as we estimate the mean value of the response when Y is continuous, we would like to be able to estimate the probability p associated with a dichotomous response for various values of an explanatory variable. To do this, we use a technique known as logistic regression.”
The authors offer the following example: “Among marathon runners, hyponatremia – defined as a decrease in blood sodium concentration to a value less than or equal to 135 millimoles per liter – can cause life-threatening illness and, in extreme cases, death. In a sample of 488 adults who completed the Boston Marathon and who are considered to be representative of the larger population of runners who complete marathons, 62 were diagnosed with hyponatremia.” That is, 12.7% of runners suffered from hyponatremia. “We might suspect there are certain factors which affect the likelihood that a particular individual will develop hyponatremia. If we could classify a runner according to these characteristics, it might be possible to calculate a more informative estimate of their probability of developing hyponatremia.” One possibility is that weight gain (hydration) might be explanatory. The authors explain why the linear regression model is inadequate (the predicted response p might be outside the interval [0,1]). “We might try to solve this problem by fitting the model p = exp(β+β1x). This equation guarantees that the estimate of p is positive. We would soon realize, however, that this model is also unsuitable. Although the term exp(β0+β1x) cannot produce a negative estimate of p, it can result in a value that is greater than 1.”
“To accommodate this additional constraint, we consider a model of the form
The expression on the right, called a logistic function, is a nonnegative, S-shaped, monotonically increasing function which can be used to model a probability that cannot yield a value that is either negative or greater than 1. Consequently, it restricts the estimated value of p to the required range.”
From this the authors point out that the log odds are a linear function of x (in the example weight gain) and that the linear model coefficients are estimated using maximum likelihood (without going into details) and that the estimated probability of hyponatremia, p-hat, can be found using antilogarithms of each side of the fitted logistic equation. They top this with a graph of estimated p versus x (weight gain).
The remainder of the chapter introduces indicator variables (also seen in Chapter 18 Multiple Linear Regression) and uses that idea to build a logistic multiple regression model with two predictors (ultimately dealing with interaction also).
Simpson’s paradox (defined by the authors as: “Simpson’s paradox occurs when the magnitude or direction of the relationship between two variables is influenced by the presence of a third factor”) is also discussed in this chapter in the context of logistic regression and odds ratios.
This book is a useful reference on standard biostatistics topics suited for those with limited mathematical and statistical experience and can certainly be used (and has) as text for persons in programs in the health sciences.
Wludyka and Associates, Jacksonville, FL