Abstract
A digital twin is aimed to be a virtual model designed to accurately replicate a physical system in the digital world. This is why digital twins have become quite popular in recent years in both rail and road domain applications, and some researchers and engineers have started considering the term as a buzz word that attracts increased interest in their research. In some cases, this leads to misunderstandings regarding the digital twin concept, especially if the discussion relates to digital twins built on physics-based models. This paper’s detailed review has been performed to address this issue considering the application of vehicle system dynamics theories as a basis for development of digital twins and the implementation process in digital twin studies. The results show that most works are focusing on digital twin developments at the ‘top level of the pyramid’ and more comprehensive research and investigation studies are needed in terms of the application of vehicle system dynamics in design and construction of digital twins. This paper adopts and summarises the possible directions for the development of a future framework in terms of disciplines relevant to vehicle system dynamics and presents a forecasting roadmap on future development strategies of digital twins.
1. Introduction
The Fourth Industrial Revolution, which is commonly abbreviated as Industry 4.0 or I4.0 and is considered to have commenced in 2011 on the basis of a German government initiative to integrate information and communication technologies in industrial production, introduced some changes by means of the application of cyber-physical systems and joining together of the newly developed digital and the existing advanced technologies and disciplines in one multidomain and multi-physics field that eliminates the lines between physical and digital worlds in transportation. One of the key deliverables of Industry 4.0 is the introduction of new conceptual terminologies in the cross-disciplinary engineering and research world for the digital transformation of design and lifecycle evaluation of products that are commonly known as physical objects. One such concept that is strictly related to the topic of this paper is called the ‘digital twin’. Obviously, not all definitions of digital twin are the same. A lot of questions exist on what we should call a digital twin in vehicle system dynamics and how it varies from simulation modelling. Before going further in this definition, it is necessary to clarify how it is defined in other technology areas in order to present a proper picture of the definition relevant to vehicle system dynamics. As a result of the presence of many uncertainties in this area as well as the current high demand for the development of vehicle designs and their wider applications in the digital world of the Industry 4.0 revolution, this paper is focused on an overview of the definitions, the architecture and simulation aspects, and the challenges in practical applications, hence its aim is to try to formulate the answers for the following questions that have been raised by the vehicle system dynamics community in recent years:
What is the definition of a ‘digital twin’ in terms of its application from a vehicle system dynamics point of view?
What is the difference between an advanced model and a digital twin?
How is vehicle system dynamics able to be applied so as to be fit for purpose in digital twin technologies?
What options are available for a digital twin for vehicle systems to be constructed and what is an appropriate integration approach?
What simulation approaches should be used?
What options are available for a digital twin to be simulated?
What validation process should be used for developing a digital twin?
What are the gaps in our knowledge regarding applications of digital twins and how can they be compensated?
How can we better manage the big digital twin unknowns in vehicle-related studies?
2. Definitions of digital twin
There are plenty of definitions to be found in the publications and commonly those definitions are not well-systemised. Therefore, the best way to present the definitions is to start from the first introduction of the definition of ‘digital twin’, and then to go along the historical transformation of the original definition to what we can observe at the present time.
Some evidence exists that sends us back to 2002 when the original digital twin concept was presented by Michael Grieves at the University of Michigan to industry participants to introduce the idea of a Product Lifecycle Management Center [Citation1]. However, at that time the definition of ‘digital twin’ had not been formulated and it was merely descriptive [Citation1]. It is commonly indicated that the first definition of the digital twin was formulated by researchers in NASA and published in [Citation2]:
A digital twin is an integrated multi-physics, multi-scale, probabilistic simulation of a vehicle or system that uses the best available physical models, sensor updates, fleet history, etc., to mirror the life of its flying twin. The digital twin is ultra-realistic and may consider one or more important and interdependent vehicle systems, including propulsion/energy storage, avionics, life support, vehicle structure, thermal management/TPS, etc. In addition to the backbone of high-fidelity physical models, the digital twin integrates sensor data from the vehicle’s on-board integrated vehicle health management (IVHM) system, maintenance history, and all available historical/fleet data obtained using data mining and text mining. The systems on board the digital twin are also capable of mitigating damage or degradation by recommending changes in mission profile to increase both the life span and the probability of mission success.
As is possible to see from the NASA definition, it is fully related to the aerospace engineering field, and it cannot be applicable in that form to all industries. As a result, the transformation of the original definition started to be quickly progressed after the commencement of the Industry 4.0 revolution. However, there are many other definitions that have been described over time relating to the idea or concept of digital twins. In publication [Citation1], it is written ‘the idea of digital twin is to be able to design, test, manufacture, and use the virtual version of the system’. Meanwhile, others assume that the concept should be built considering that ‘the digital twin is a collection of all digital artifacts that accumulate during product development linked with all data that is generated during product use’ [Citation3]. We have gathered many definitions from publications and patents, which were further reviewed based on the digital twin’s purpose, application area and key points in [Citation4]. From the point of a virtual replica for Industry 4.0 in [Citation5], an experimentable digital twin was defined as ‘a digital twin is a one-to-one virtual replica of a ‘technical asset’’. At the same time, considering the point of dynamic representation for fault diagnostics, it is defined in [Citation6] as ‘Digital twin represents physical entities with their functions, behaviors, and rules dynamically’. In terms of vehicle dynamics, the definitions referring to modelling and simulation are a subject of particular interest. For example, Luo et al. [Citation7] define a digital twin as ‘a multi-domain and ultrahigh fidelity digital model integrating different subjects such as mechanical, electrical, hydraulic, and control subjects’, while Wang et al. [Citation8] state that a ‘Digital twin can be regarded as a paradigm by means of which selected online measurements are dynamically assimilated into the simulation world, with the running simulation model guiding the real world adaptively in reverse’.
Considering aspects of rail vehicle design and dynamics, the general definition was also summarised in [Citation9] based on the definition given in [Citation10–12] and should include four important parts:
‘a model of the object built based on an integrated multiphysics, multiscale, probabilistic simulation approach,
an evolving set of data relating to the object,
a means of dynamically updating or adjusting the model in accordance with the data,
mirror and predict activities/performance over the life of its corresponding physical twin’.
However, at the same time, vehicle digital twin definitions related to different application fields can significantly vary. For example, as published in [Citation10], a digital twin for autonomous vehicles (AV) should satisfy the following four criteria:
‘an accurate and updatable model of a real-world driving environment, including other vehicles and pedestrians and some description of weather and other atmospheric and environmental effects,
models that simulate the response of the sensors deployed on the vehicle based on a careful choice of data from tests of these sensors,
a model of the vehicle response to driving commands (e.g. steering changes, braking, etc.) that takes road surface conditions into account,
and the AV control algorithms’.
However, if the digital twin definition is given for connected and automated vehicles, the focus should be put more on their characterisation as described in [Citation13]. In that article, the digital twins can be characterised using the following dimensions: ‘the digital model, the physical system, the communication between them, the big data that is created from the process, and the services that can be deployed to add value’.
This leads us to the understanding that not all practitioners and researchers use the same definitions for digital twins in their work and that the existing terminologies are quite different for each type of vehicle, and it might bring some new ideas into the digital twin technologies. A similar opinion can also be found in [Citation10] where the authors also referred to use the simplistic definition provided in [Citation14]: ‘A Digital Twin is an executable virtual model of a physical thing or system’, where the physical thing can be anything from a manufactured object, like a car or rail vehicle. This also confirms that the definition of a digital twin is not settled and each definition has its own norms [Citation15].
This discussion indicates that there is considerable scope for further investigations of the physical phenomena in the definition of digital twins as well as vehicle dynamics models for digital twins which could support further development of a definition of the digital twin for its application in vehicle design and vehicle dynamics practice. Therefore, this gap should be filled in order to provide an acceptable terminology which can be used in both rail and road domains for the deployment of the models during the design and operational phases of a vehicle’s life cycle.
3. Advanced model vs simulation modelling vs digital twin
The computer simulation concept was originally proposed in 1979 by the Society for Computer Simulation Technical Committee on Model Credibility [Citation16]. It contains three major cross-connected components knows as Reality, Conceptual Model and Computerised Model. The term ‘Reality’ in modern terminology can be defined as a physical system. The term ‘Conceptual Model’ can be defined by two modern terms of ‘system specification’ (also called ‘technical specification’) and ‘model’. The term ‘Computerized Model’ means ‘an operational computer program which implements a conceptual model’ [Citation16] or ‘an exact replica of the Conceptual Model’ [Citation17]. In order to align these definitions with modern language, the terminology can be re-formulated into two definitions that can be applicable for a simulation process used in vehicle system dynamics. In this case, the model can be defined as published in [Citation18]:
A model is conceived as any physical, mathematical, or logical representation of a system, entity, phenomenon, or process.
The case of a computerised model can be considered
as a set of instructions, i.e. a model needs some agent capable of actually activating and obeying the instructions and generating behaviour. We call such an agent a simulator. Thus, a simulator is any computation system (such as a single processor, a processor network, the human mind, or more abstractly an algorithm) capable of executing a model to generate its behaviour. [Citation18]
Considering early computerised multibody dynamics modelling trends applicable for vehicle dynamics and published in the 1980s and 1990s [Citation19,Citation20], it is possible to observe a clear transition from the common definition for ‘simulator’, which is most often used in the field of computer engineering, to the more specific definition of ‘simulation’ used in vehicle dynamics. As summarised in [Citation21]:
Simulation in vehicle system dynamics has its historical origin in the analysis of the purely mechanical behaviour using mechanical multibody system models.
It is clear that definitions associated with purely mechanical system models used for design remained very simplistic [Citation22] and they cannot replicate complex engineering systems that may contain flexible bodies, mechatronic components, etc. This leads us to the idea that advanced vehicle systems should be denoted as mechatronic vehicles and they should be studied with advanced modelling techniques. Some ideas on how this can be organised have been published by Kortüm and Vaculín [Citation23]. The interaction of vehicle dynamics and other disciplines are handled conveniently by the application of co-simulation techniques that can work in online and real-time simulation modes [Citation24–26]. As a result, it should be able to handle multidisciplinary problems in the manner shown in Figure . Therefore, it is necessary to have different software products for each discipline and to allow them to talk to each other by means of co-simulation. Arnold et al. [Citation21,Citation24] define a co-simulation process as one in which ‘the subsystems are handled or integrated by different integration methods or solvers and each of these methods or solvers can be or is tailored to the corresponding subsystems’. A further comprehensive definition is presented in [Citation27] as ‘co-simulation exploits the modular structure of coupled problems in all stages of the simulation process beginning with the separate model setup and pre-processing for the individual subsystems in different simulation tools’. All the preceding discussion leads us to the definition of an ‘advanced model’ that can be formulated as:
An advanced vehicle dynamic model is the multidisciplinary software-based built model that provides a capability to build an exact replica of the conceptual vehicle model and, at the same time, it provides an accurate response to control commands and operational conditions.
It is necessary to mention that the accuracy of vehicle dynamics simulation will be dependent on two factors: accurate dynamics representation of the vehicle system and the computational time to produce the simulation results. The latter is quite important for advanced models that work in a real-time digital simulation environment.
However, this definition shows that there are more factors applicable for vehicle design and roadworthiness acceptance tasks with quite limited information available on the specific requirements for product cycle, i.e. the introduction of vehicle operational requirements that are commonly observed in the real world of the vehicle operational life cycle as specified by some normative documents such as standards, best practice guides, etc. As a result, the overall arrangement can be represented by the V-model example of the vehicle design process as shown in Figure which was formulated and delivered following details set out in the publications [Citation28–30].
Figure 2. Examples of V-models for the design of a mechatronic system of a vehicle [Citation31].
![Figure 2. Examples of V-models for the design of a mechatronic system of a vehicle [Citation31].](/cms/asset/882237c7-23bb-458e-af47-bf602df82970/nvsd_a_2188228_f0002_ob.jpg)
The output of the V-model is the prototype that is usually defined as ‘a complex system with several separate interacting subsystems’ [Citation32]. In addition, it is obvious that we have some important systems that can be applied or not applied in a simulation process under the relevant conditions of the specific investigations. One example of such an important system is the physical system that is represented by the block ‘Reality’ as shown in Figure . As a result, there is the question that should be answered – how should we make the advanced model more realistic and introduce more data available from the physical system in the advanced model? We exclude an opinion here that ‘if no model exists satisfying our aim and technical limitation, then no simulation is possible’ [Citation33]. One of the possible answers is to use ‘simulation modelling’. Before starting a discussion on the differences between ‘advanced modelling’ and ‘simulation modelling’ approaches, it is necessary to provide the definition of the second term. As defined in [Citation34], simulation modelling is ‘the process of creating and experimenting with a computerised mathematical model of a physical system’. This also means that a computer simulation model can then be referred to as a computer simulator. On the other hand, it is stated in [Citation35] that ‘simulation models aim to replicate the workings and logic of a real system by using statistical descriptions of the activities involved’. The explanation of the necessity to use simulation modelling is explained in [Citation34] by the following disadvantages of the pure simulations:
‘Simulation cannot give accurate results when the input data are inaccurate.
Simulation cannot provide easy answers to complex problems.
Simulation cannot solve problems by itself’.
As a result, the following extensions are considered necessary for simulation modelling as also mentioned in [Citation34]:
‘Simulation model building can require specialised training.
Simulation modelling and analysis can be costly.
Simulation results involve many statistics’.
Therefore, by itself simulation modelling represents a process that can work with an advanced model to deliver the output measures of performance of the physical system. This statement is confirmed with the definition of the simulation model (SM) published by Durán [Citation36] that states:
The SM is a rich and complex structure that departs in important ways from standard models used in scientific research.
All of the foregoing points related to the descriptions and definitions of the term of ‘simulation modelling’ lead us to the understanding that simulation modelling is a process:
where the physical system is replaced with an advanced model, that can be also called a ‘simulation model’ and it is built with a high level of accuracy to properly describe the physical system;
which is designated to perform experiments in the virtual world to deliver information about the physical system;
which is used to analyse a prototype of a physical system to predict its performance in the real world.
As a result, simulation modelling works with data delivered from the real world to formulate testing conditions that will allow making a judgment about where a designed prototype could fail or could operate properly under applied dynamic loads. So, it is quite easy for it to be used during the design stage and the simulation modelling is quite relevant to the output of the V-model, which is designated to deliver the prototype without considering the performance of the existing physical system that can be observed in the operational routine after the design stage is finished and the prototype has been delivered as the final product. This clearly explains why the term ‘digital twin’, defined in Section 2, should be introduced to support service solutions that optimise operations and failure prediction. And as is stated in [Citation37], the digital twins should be built based on multi-domain and multi-level simulation approaches, and ‘these approaches must be embedded in the system engineering and development process and reused in all following lifecycle phases’.
Considering the level of detail, a general comparable structure of data flow for all three simulation technologies (Advanced modelling, Simulation modelling and Digital twin) can be summarised based on the information flow representation idea as published in [Citation9] and presented as shown in Figure . While a simulation box is presented in all three methods and it might be focused on one particular process, a digital twin has one distinguishing feature which is that it can include in itself various simulations (i.e. not only a simulation that focuses on a vehicle dynamics task) in order to analyse and examine its behaviour under multiple possible processes and operational scenarios. If we consider the history timeline, all three simulation technologies have a strong connection with the transition from one development to another as shown in Figure . All these theoretical investigations on the digital twin definitions performed in this section lead us to the tasks for the next sections that require re-considering all recent published research in rail and road domains and re-checking their relevance to digital twin conceptual priorities as shown in Figure .
Figure 3. Conceptual comparison overview of information flow for simulation-based technologies used in vehicle dynamics.
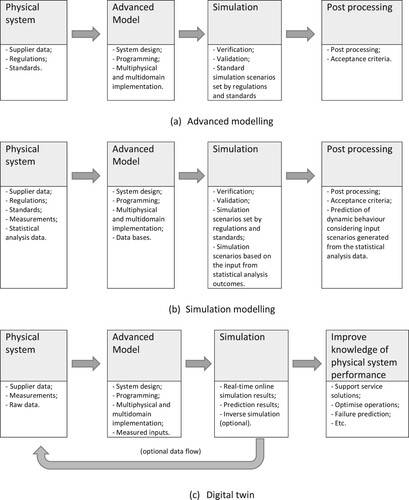
Figure 5. Digital twin development tasks considering the entire lifecycle of the intelligent vehicular systems [Citation38].
![Figure 5. Digital twin development tasks considering the entire lifecycle of the intelligent vehicular systems [Citation38].](/cms/asset/8fe7b672-df3d-4bd5-bae4-792eb60373e8/nvsd_a_2188228_f0005_oc.jpg)
4. Digital twin architecture aspects
As discussed in the previous chapters, definitions of digital twins are as various as the purposes they are intended for. Implementations of digital twins are reported from many different fields of application in recent literature [Citation4,Citation39,Citation40]. However, even within one field of application, ‘current Digital Twin implementations are often application-specific solutions without general architectural concepts and their structures and namings differ, although the basic concepts are quite similar’ [Citation41]. Before highlighting specific applications of digital twins in the rail and road domain in Sections 6 and 7, a generic view on relevant aspects for constructing digital twins in vehicle dynamics applications is provided here, aiming to discuss the ‘basic concepts’ in the above quotation.
A basic scheme of a digital twin is shown in Figure . Following Section 3, the digital twin consists of a set of advanced models and respective data which comprehensively represent the properties and conditions of the physical object and/in its designated environment, and thus allow it to predict its actual and future behaviour through simulation. The digital twin (periodically or continuously) collects and exchanges data and information with existing IT-Systems of the real system, i.e. the ‘physical twin’. Based on these data, the digital twin (dynamically) updates and adjusts its models and parameters in accordance with the real operation conditions. ‘Thus, the Digital Twin extends the concept of model-based system engineering (MBSE) from engineering and manufacturing to the operation and service phases’ [Citation37].
Figure 6. Basic scheme of a digital twin. The digital twin uses information and data from different IT-Systems and makes it available for succeeding phases of the lifecycle of the product [Citation37]. PLM, PDM and SCADA refer to Product Lifecycle Management, Product Data Management, and Supervisory Control and Data Acquisition.
![Figure 6. Basic scheme of a digital twin. The digital twin uses information and data from different IT-Systems and makes it available for succeeding phases of the lifecycle of the product [Citation37]. PLM, PDM and SCADA refer to Product Lifecycle Management, Product Data Management, and Supervisory Control and Data Acquisition.](/cms/asset/15fec4b3-ba25-4776-a60a-78ff257306b7/nvsd_a_2188228_f0006_oc.jpg)
The digital twin evolves with the real system and remains its virtual counterpart across the entire lifecycle. The digital twin is ready-to-use to perform life-cycle-specific simulation tasks and finally closes the loop from operation and service back to the design phase (of a new product or updated revisions of the existing one). Predictive maintenance and fault detection and diagnostics are typical use cases [Citation6,Citation42] since the approach provides an accurate description of the change of the system over time. The model of the digital twin, which is the opposite of a neural network type model, should then be ‘sufficiently physics-based’ [Citation10], such that a change in a model parameter can be correlated to the measurement data. In addition, for issues occurring in later phases of the product, solutions may be established with the aid of the already existing (and verified [Citation79]) simulation models, and by taking the specific historical data of the product into account.
In other digital twin purposes, it is not the long timescale that is of interest, but rather the (real-time) interaction of a system with its environment, or even cross-system interactions of multiple systems within the environment (sometimes referred to as a ‘system of systems’ digital twin [Citation40]). Shao [Citation43] states with respect to smart manufacturing systems that ‘there is no single digital twin that can provide all the solutions for a given manufacturing system, equipment, or process. Different digital twins may be developed with different scope and objectives. In certain cases, multiple digital twins may need to be integrated to cover a larger scope’. Smart manufacturing systems are among the pioneers of Industry 4.0 technologies [Citation44–46], and according to [Citation47], digital twins and Industrial Internet of Things technologies are ‘an integration exercise rather than a development challenge’. Common methods, standards and norms are demanded from the industry to realise the potential of digital twins [Citation15].
For either of the above digital twin scenarios, the aim of the digital twin is to provide solutions for specific objectives and questions which need to be defined in advance. The collection of these objectives then leads to a list of functional requirements (demanded services such as prediction, optimisation and control of future behaviour, adaption of model parameter to actual conditions, etc.) and quality requirements (quality, availability, latency, storage, security of data, etc.). The former requirements are largely decisive for designing the advanced models of the digital twin, or e.g. selecting the applied type of sensor of the physical twin. The latter requirements especially need to be taken care of when designing the architecture of the digital twin system, whereby
a system’s architecture is a representation of a system in which there is a mapping of functionality onto hardware and software components, a mapping of the software architecture onto the hardware architecture, and a concern for the human interaction with these components. That is, system architecture is concerned with the totality of hardware, software, and humans.
Caporuscio et al. [Citation50] discuss architectural concerns for digital twins of organisations. The authors recommend with reference to software engineering that a digital twin architectural framework should include:
‘an Architectural Pattern describing architectural elements and connectors, with constraints on how they can be combined, achieving flexibility;
a Reference Model decomposing functionality into elements (and data between them) that cooperatively satisfy the organization needs;
a Reference Architecture mapping the Reference Model onto system elements and defining the data flows between them’.
Closely following the guidelines from systems and software engineering, the ISO 23247 [Citation51] standard was recently developed for digital twins in the manufacturing industry. Its four parts define ‘a framework to support the creation of digital twins of observable manufacturing elements including personnel, equipment, materials, manufacturing processes, facilities, environment, products, and supporting documents’. Part 2 provides a reference architecture for a digital twin in manufacturing, wherein a digital twin is defined as a ‘fit for purpose digital representation of an observable manufacturing element with synchronization between the element and its digital representation’. The proposed reference architecture is based on the Internet of Things (IoT) reference architecture as defined in ISO/IEC 30141. It specifies a digital twin reference model in terms of four domains: the ‘user domain’, the ‘digital twin domain’, the ‘device communication domain’, and the ‘observable manufacturing domain’, which are further divided into five entities, based on the required tasks and functions of each domain. The entities are further divided into sub – and functional entities, and the resulting ‘Functional view’ of the reference architecture indicates the defined intra-domain and cross-domain interaction patterns as shown in Figure . The ‘Device Communication Entity’ collects data from sensors of the ‘Observable Manufacturing Elements’, i.e. the physical items, and sends data for control and actuation purposes. The ‘Digital Twin Entity’ manages and operates the individual digital twins, performs simulations and analyses based on input data, and processes the results for sharing with the other entities. The ‘User Entity’ provides interfaces to interact with the user, i.e. a human operator or a digital enterprise application such as Enterprise Resource Planning (ERP) systems. The ‘Cross-System Entity’ provides necessary functionalities such as data translation, data assurance, and security support. Parts 1, 3 and 4 additionally provide an ‘Information view’ and an ‘Networking view’ of the reference architecture, defining terminologies, technical requirements and standards for the digital representation and information exchange within the framework.
Figure 7. Functional view of the digital twin reference architecture for manufacturing according to ISO 23247–2 [Citation51]. The digital twin framework comprises four entities and exchanges data with observable manufacturing elements; FE stands for Functional Entity.
![Figure 7. Functional view of the digital twin reference architecture for manufacturing according to ISO 23247–2 [Citation51]. The digital twin framework comprises four entities and exchanges data with observable manufacturing elements; FE stands for Functional Entity.](/cms/asset/b2f52b82-9d2d-4232-8798-e9154fcb0617/nvsd_a_2188228_f0007_ob.jpg)
Several reference frameworks, models and architectures for digital twins are presented in literature, with a different level of detail, and typically tailored for a specific industry and field of application, e.g. [Citation41,Citation52] for industrial energy systems, [Citation53] for the petrochemical industry, [Citation54] for industrial product family design with inclusion of context awareness, [Citation39] from a software engineering perspective, [Citation50] for enterprise organisation, [Citation55,Citation56] for cyber-physical systems, and [Citation57] for connected vehicles.
Therein, authors often use a layered structure to decompose the digital twin system into its essential components and group them in certain layers depending on its functions and interrelations. Steindl et al. [Citation41] present a layer-based generic digital twin architecture for industrial energy systems. Its six layers are aligned with the information technology layers of the ‘Reference Architecture Model Industry 4.0 (RAMI 4.0)’ as defined by the German Electrical and Electronic Manufacturers’ Association to support Industry 4.0 initiatives [Citation58], thus facilitating a common naming and understanding of the proposed architectural structure. The layers read from bottom to top: (i) the ‘Asset Layer’, representing the physical twin, (ii) the ‘Integration Layer’, where both run-time data and engineering data are captured, (iii) the ‘Communication Layer’, which provides necessary protocols for the data exchange with the upper layers, (iv) the ‘Information Layer’, where data is processed, stored, and its access is managed, (v) the ‘Functional Layer’, providing the functional services of the digital twin (here: simulation, monitoring, diagnostics, prediction, control and reconfiguration) as well as a human-machine interface (HMI), and (vi) the ‘Business Layer’, which defines the overall objectives of the digital twin. A ‘Shared Knowledge Base’ acts as a central component inside the Integration Layer, where (dynamic) run-time data gathered from sensors is linked with (static) engineering data (contextual information such as parameter tables, construction drawings, instrumentation diagrams, etc.) to achieve a desired level of semantic expressivity. A proof-of-concept on a laboratory scale is presented in [Citation41] to show the suitability of the framework for handling context information in combination with simulation models, enabling a certain view on the virtual entity of the digital twin. The modelling of context information is based on Semantic Web technologies and reuses existing ontologies (like OWL-S or ML-Schema) to hold contextual information about resources and services in a formal and machine-readable way.
Cyber-physical systems (CPS) ‘represent systems which integrate physical units and processes with computational entities over Internet and allow ubiquitous access of information and services’ [Citation56]. The integration of (multiple) digital twins within a cloud infrastructure is claimed as ‘the true bridge’ between a physical layer and an application layer of cyber-physical systems in [Citation55]. The authors present a digital twin architecture reference model for cloud-based cyber-physical systems. Therein, every physical ‘thing’ is assumed to be accompanied by a representative cyber ‘thing’ hosted in the cloud; interaction among the ‘things’ can occur either through direct ‘ad-hoc’ communication in the physical layer or through ‘peer-to-peer’ communication in the cyber layer of the architecture. Additional layers are proposed to organise communication groups, data exchange, storage and visualisation, and services. An architecture reference model is provided as well, where tasks related to the incorporation, selection and fusion of sensors, models, data and services from different local and cloud-based sources within the CPS are described by using finite state machine, Bayesian networks and fuzzy logic control techniques. The applicability of the framework is demonstrated via a prototype telematics-based driving assistance application for vehicular CPS, which provides location and driving event-specific information or warnings to the driver.
A framework in particular tailored for connecting multiple mobility entities together is presented in [Citation57]. The authors describe their so-called ‘Mobility Digital Twin’ as an ‘Artificial Intelligence (AI)-based data-driven cloud-edge-device framework for mobility services’. The framework basically comprises ‘Human’, ‘Vehicle’, and ‘Traffic’ entities in both the physical space and their associated digital twins in the digital space, as well as the communication between the entities, e.g. by using Internet of Things (IoT) and/or Internet of Vehicles (IoV) technologies [Citation59,Citation60]. A ‘data lake’ is considered in the digital space as ‘a centralized repository that allows structured or unstructured data at any scale to be stored’. The resulting data can be used for micro-services for an individual entity, but also for ‘cooperative control’ or ‘parallel control’ of multiple entities [Citation61]. An example implementation of the framework with cloud-edge computing is discussed in detail to provide an idea of deploying the digital twin concept in the real world, building on Amazon Web Services (AWS) and other (commercial) software tools. The four-layer architecture is shown in Figure .
Figure 8. Cloud-edge example architecture of the ‘Mobility Digital Twin’ in [Citation57].
![Figure 8. Cloud-edge example architecture of the ‘Mobility Digital Twin’ in [Citation57].](/cms/asset/b24057fe-4e0c-4baf-8805-eee4918b86ad/nvsd_a_2188228_f0008_oc.jpg)
A ‘Personalized Adaptive Cruise Control P-ACC’ system is presented in [Citation57] as a case study of the implementation, combining three micro-services: (i) user management and driver type classification of the human digital twin, (ii) cloud-based Advanced Driver-Assistance Systems (ADAS) of the vehicle digital twin, and (iii) traffic flow monitoring and variable speed limit of the traffic digital twin. The capability of the framework was showcased with a real-world test with three drivers, and significantly less takeover events from the drivers were observed for the proposed P-ACC compared to a traditional PID-based ACC-system. As future challenges for digital twins within the transport domain, especially (missing) standardisations for data, HMI, and wireless communications environments are pointed out, as well as open research challenges regarding the generalisation ability of Artificial Intelligence techniques, and security/privacy issues for cloud-edge computing systems were identified.
Tao et al. [Citation62] formulate five ‘dimensions’ to describe the essential ingredients of digital twins: (i) the physical entity, (ii) the virtual entity, consisting of a set of models, (iii) services for both the physical and the virtual entity, (iv) data, and (v) the connection that ties the different parts of the digital twin together. Note, in contrast to Figure , that the physical twin in this case is included in the digital twin framework. From the above considerations, one may deduce that this concept should not be understood as an architectural description; but its ‘dimensions’ are used here to discuss some additional architecture aspects for digital twins from a vehicle system dynamics perspective.
Virtual and physical entity. Modelling and simulation aspects are discussed in full detail in Sections 3 and 5 of this paper. Here, only some additional remarks are given. Models can be white-box (with a physics-based structure), black-box (with a statistical model or neuronal network-based structure) or grey-box (as a combination of the former two) [Citation82]. The benefit of the physical transparency of white-box models are well known, however, when calculation resources or data transmission limitations are of concern, simplified data-driven or reduced order models may be applied within the known operation range of the physical model; James et al. [Citation63] compare physical and data-driven vehicle models for longitudinal dynamics under large-scale real-world driving conditions, with encouraging results for the data-driven models. For a comparative study, Maulik et al. [Citation64] analyse dynamic reduction-based virtual models for digital twins of offroad-vehicles.
‘Data-Augmented Modelling’ refers to the case where available measurement data from the physical twin is used to improve/correct inaccuracies in the solutions/predictions of the digital twin due to limited model fidelity [Citation78]. On the other hand, the digital twin can be used as a ‘virtual sensor’ or ‘soft sensor’ where real measurements are technically not feasible, obviously leading to well-known (state) estimation [Citation65] or condition monitoring applications [Citation66]. Similarly, failures of a physical sensor may be identified and adapted operation strategies applied by relying on digital twins. In a related study, Makke et al. [Citation67] propose a robust IoT-based parking information system, which is based on a Petri Net and adapts automatically to adding or removing sensors and to sensor failures.
Environmental effects are often disregarded (or at least kept constant) in simulation studies, implying that the studied object is in a well-defined operation condition. However, depending on the purpose of the digital twin, (dynamical) environmental effects need to be included in the simulation models. Milošević et al. [Citation68] demonstrate a digital twin framework for model-based operational condition monitoring of railway crossing panels. Sensor temperature was found to be highly correlated with measured sleeper accelerations in the long-term monitoring data and is thus an important parameter to monitor. For road vehicles, digital maps and traffic properties may need to be kept up to date as real-world conditions change [Citation13].
Data. Data in the context of digital twins are often grouped into dynamic and static data [Citation41]. Static data includes CAD data, as-built data, model parameters, etc., but also meta-data such as textual requirements from the initial design phase of a product; for data comprehension and formalisation, ontologies are a well-established approach, see [Citation69] and references therein. Incoming and outcoming dynamic data may be processed in real-time or offline; ‘offline’ in this context means ‘the case where real-time communication is not critical’ [Citation70], and the connection to the physical system happens periodically or just occasion-related.
If a true real-time connection is demanded, special consideration needs to be laid on the infrastructure; Ruba et al. [Citation71] discuss the implementation of a digital twin simulation model for a light vehicle propulsion system on two field programmable gate array (FPGA) as an alternative to a physical test bench. For the offline case, Heber et al. [Citation72] investigate the potential of the blockchain technology in the automotive industry and present a concept to keep track of electric/electronic and software updates over the lifetime of the vehicle. However, the amount of data that can be stored within a blockchain is limited, and the blockchain concept has evolved more toward secure transaction applications with less relevance in engineering [Citation78].
Datasets collected in the digital twin and CPS context may be too large to ‘be perceived, acquired, managed, and processed by traditional IT and software/hardware tools within a tolerable time’, which is used in [Citation73] as a general definition of the term ‘big data’. The authors provide a comprehensive survey on the four phases of big data: data generation, data acquisition, data storage, and data analysis. The survey includes, but is not focused on, technical applications. A literature review of big data, artificial intelligence, and machine learning techniques specifically in the digital twin context is provided by [Citation74]. Machine Learning methods for the purpose of predictive maintenance combining data from off-board and on-board sensors are thoroughly reviewed in [Citation42,Citation75].
Connection. Connection and communication obviously are key aspects of digital twins. A standardisation of protocols, interfaces, gateways, etc. to connect a huge number of heterogeneous components is mentioned as a future challenge for digital twins by many of above authors, e.g. [Citation13,Citation43,Citation40,Citation57]. A review of the current standard landscape of digital twins and Internet of Things (IoT) is provided in [Citation59].
For connected vehicles, Contreras-Castillo et al. [Citation60] discuss the transformation from vehicular ad-hoc networks (VANETs) to Internet of Vehicles (IoV) along with current industry standards. Architectural aspects, current protocols and security requirements are addressed as well, together with recent projects and future research directions. Kiela et al. [Citation76] provide a comprehensive review of vehicle-to-everything (V2X) standards and frameworks for intelligent transport systems (ITS) applications. The two main V2X-technologies, i.e. ‘dedicated short-range communications’ (DSRC) and 4G – and 5G-based ‘cellular vehicle-to-everything’ (C-V2X) are compared, and their core parameters, shortcomings and limitations are discussed.
Relying on the above communication technologies, architectural design alternatives for connected vehicles based on cloud/edge/fog computing are investigated in [Citation77]. The authors provide a comprehensive survey of existing state-of-the-art architectures, which are further classified into ‘computation-aided’ and ‘computation enabled’ architectures. While a vehicle in the former case is intended to share its data (and computation is performed by means of external infrastructure, i.e. cloud/edge/fog servers), in the latter case vehicles also share their computation capabilities within the network.
Services. Digital twin services and applications in rail and road domain are discussed in detail in Sections 6 and 7.
5. Digital twin simulation aspects
The digital twin simulation that considers the application of principle and modelling approaches of vehicle dynamics should be built considering the requirements in [Citation78,Citation79] and it should include the additional components that can be summarised and presented as:
Multidisciplinary (physics-based) model;
Software design and implementation aspects for the model-based design;
Software integration and management;
Cyber-security and risk mitigation;
Verification and validation;
Analysis assumptions and uncertainty quantification;
Data-augmented modelling;
Output visualisation.
Let us go step by step and discuss each component in sub-sections below.
5.1. Multidisciplinary model
The concept design of multidisciplinary vehicle dynamics models relevant to mechatronics tasks with different simulation approaches has been discussed by Kortüm and Valasek [Citation80], and is also relevant for digital twins, especially, the statement that the vehicle model represented in an multibody software package has to be included ‘concurrently’ in the overall system dynamics and it should be realised through the co-simulation process as shown in Figure , where system A and system B are related to different engineering disciplines.
Figure 9. Simulation approaches in vehicle dynamics for different engineering disciplines [Citation80].
![Figure 9. Simulation approaches in vehicle dynamics for different engineering disciplines [Citation80].](/cms/asset/16072d1e-d30a-4961-ae50-14e5e6b2d700/nvsd_a_2188228_f0009_ob.jpg)
The reasons to use coupling of models were published in [Citation81], where it is stated that the simulation models can be divided into sub-system models and they can be easily transformed to the multidisciplinary model terminology relevant to digital twins that we use at the present time:
‘Functionality – control, mechanical, electronic, hydraulic
Geography – company (division) location
Component – protecting property rights
Design – e.g. ride/handling models
Hardware/Software – Software execution to meet real-time constraints and
Hardware in the loop testing
Resolution – model prediction accuracy’.
In addition, as stated in [Citation81], the model simplification for real-time implementations is one of other important issues. It can be also relevant to digital twins considering their architecture aspects and implementation tasks. The general understanding of the usage of the models in different digital twin variants was summarised in [Citation82] and simplistically presented in Figure , however it does not cover all aspects that we observe in vehicle dynamics world and what should be considered in terms of the complexity of whole dynamic systems (e.g. a large number of vehicles in the system, the slower speed computations for asset management and predictive maintenance, etc.). This leads us again to the previous discussion held at the 22nd IAVSD Symposium and presented by Arnold in 2011 [Citation24]: ‘With the increasing complexity of proprietary engineering software tools, the need for combining different programme packages in robust co-simulation environments gains more and more importance’.
Figure 10. Usage of the different digital twin variants, depending on timing and model depth [Citation82].
![Figure 10. Usage of the different digital twin variants, depending on timing and model depth [Citation82].](/cms/asset/4f558228-1ff6-41a4-9069-8625e5e772a9/nvsd_a_2188228_f0010_ob.jpg)
5.2. Software design and implementation aspects for the model-based design
Software design and implementation aspects of digital twins are chosen based on the computational magnitude of the twin and expected deliverables that are set by digital twin-specific requirements. It also requires a proper coupling of software packages (i.e. sub-models) considering ‘complexity and particularly interdisciplinarity of vehicles and their simulation models’ [Citation83]. In addition, as summarised in [Citation24], it is necessary to understand differences in real-time simulation and the simulation of complex multidisciplinary problems. It is understandable that both modelling techniques can be used in digital twins and can have significant effects on their implementation. Digital twins built on real-time simulations are designated to work with the design and driver behaviour tasks. The definition provided in [Citation84] states that ‘real-time simulation is where the virtual world (e.g. multibody model of a passenger car) and the real world (e.g. electronic control units) meet each other’, thus methods for model set-up and time integration in real-time applications are quite strict and well defined.
In terms of the implementation aspects, these can be distinguished in the three standard implementation approaches:
Embedded computer, personal computer, workstation or server;
High performance computing (cluster);
Cloud platform.
While the first two methods are widely used and the deployment mechanism is well-known in the vehicle dynamics society, the cloud platform has only recently started to be under development for the deployment of dynamic models. As stated in [Citation85], ‘the cloud computing allows infrastructure scalability and enables multiple users to utilize the same hardware and software’ that leads to reduction in maintenance, licensing and IT costs. The cloud computing service is a gigantic software virtualisation mechanism that can run multiple instances of software systems and packages, and it can be accessible remotely from different geo-locations through the communication networks that also allow not only to perform computing but also to collect all data from physical systems (e.g. embedded systems, etc.) using IoT + technologies that link the physical world and virtual reality through many modular (layered) cloud platform communication protocols. Both computational and data streams can be operated in the same computerised space.
The main key question in terms of model-based design that should be solved at this stage is how all sub-models of one physical-based model that uses different software packages can be integrated in the time domain to allow a model to perform as expected in real-time or online simulations.
5.3. Software integration and management
Considering that the sub-models should communicate with each other in terms of vehicle dynamics modelling, it is necessary to consider interfaces for performing a co-simulation between different software packages. Arnold et al. [Citation21,Citation24] define a co-simulation approach as a software integration approach that should be equipped with a data exchange algorithm which has discrete synchronisation points with a sampling time of 0.001 s for vehicle system dynamics studies. In the case of rail simulations, we can see a similar statement made by Polach et al. [Citation86] indicating ‘the stiff wheel-rail contact provides high system eigenvalues and therefore make it necessary to run time-domain simulations (by means of numerical integration) with time steps in the order of only 0.1–1 msec’. However, if we consider analysis of sampling times for multidisciplinary tasks [Citation26] as shown in Figure , this leads us to the idea that the sampling time in the case of a digital twin might be dependent on the specific object of the study and the study goals that should be achieved.
Figure 11. Simulation time-step by application [Citation26].
![Figure 11. Simulation time-step by application [Citation26].](/cms/asset/a88cf4b9-e93a-4d20-9916-b149f43bed8d/nvsd_a_2188228_f0011_oc.jpg)
In the published analyses related to vehicle digital twin technologies [Citation71,Citation87–91], there is no analysis on the sampling times included. Considering the common architecture of complex multi-physics systems, the choice of sampling time should be performed at the sub-model level first, and then the main decision should be made at the highest level for software integration with a ‘wrapper’ module that operates the workflow of a digital twin [Citation78]. In Bhatti et al. [Citation87], it was also proposed to separate the models of non-critical sub-frameworks in order ‘to keep the virtual model’s computational complexity low’.
Considering the engineering aspects of the digital twin and that a variety of software packages with their own solvers might be used in the analysis, it is obvious that no unique design of software management exists and the software architecture should be managed with a proper logical flow (‘a series of logical steps in each process’ as specified in [Citation78]) so as to allow capturing not only the required behaviour of a vehicle but also to capture the behaviour of the whole digital twin to deliver sophisticated outcomes for the desired application purposes at reasonable computational costs.
5.4. Cyber-security and risk mitigation
It is clearly understandable that the digital twin is a product that is built as a software solution and any software is a subject for cyber-security threats, thus risk mitigation strategies should be applied. In terms of vehicle dynamics, a special concern should be made considering the development of in-house products to avoid any voluntaries in the coding. Regarding commercial software, the main focus should be put on the deployment aspects. It is necessary to consider that any sensors or network communication points could be an entry point for cyber-security attacks [Citation92], so the vehicle model should have a failure proof mechanism to report any uncertainties to avoid any catastrophic consequences to the cyber-physical system [Citation93]. At the present time, there are no specific requirements formulated for vehicle dynamics models from cyber-security aspects.
5.5. Verification and validation
The verification and validation processes of vehicle models are well known to the vehicle dynamics research community. However, it is not exactly known what is expected for a digital twin’s verification and validation.
Common sense dictates to us that the verification process for a digital twin should include not only algorithm verification and software quality assurance, but it should also require checking the full workflow in a digital twin, i.e. as defined in [Citation78]: ‘checking the interactions of code as a part of a wider software’ and including verification of the software integration and management strategies. The latter task is more specific to be designated for a software engineer rather than a vehicle dynamicist.
The validation process is a quite complex process related to the validation of all sub-models to be sure that they work properly as expected and, at the same time, it requires a validation at the system level. The latter means that a digital twin is validated only at the sub-model level. At this stage, it appears that there are no specific standards on the validation of digital twins, but some ideas in a general sense on implementations in this area can be found in [Citation78,Citation79,Citation90]. However, some preliminary work on the development of respective standards has been started in this direction [Citation94,Citation95].
5.6. Analysis assumptions and uncertainty quantification
While the science of vehicle dynamics has well-established approaches to handle analysis assumptions and uncertainty quantification in the models, the digital twin still has a great number of challenges that should be resolved. The publication analysis presented in [Citation10] shows that the digital twin allows us to estimate what can be measured directly and it gives an uncertainty evaluation for a complex system. The challenge in such cases is to perform analysis using proper assumptions and to quantify the uncertainty in the digital twin. For handling nonlinear and transient models when direct experimental data are unavailable, the best choices involve the use of physics-based laws such as are used in the traditional modelling approaches or using a historical data-driven physics-based approach from a similar complex system where data are approximately known, and the analysis should be updated as new data are available (for example, see [Citation96]).
In the case of uncertainty quantification, it is better to use a variety of methods [Citation78,Citation97,Citation98] that might include probability theories, sensitivity analyses and general toolbox methods, or the predictions are made by other complex models.
5.7. Data-augmented modelling
Data-augmented modelling assumes that special data analysis techniques should be used in order to produce slightly modified copies of already existing data or completely new data from existing data to compensate for the lack of data that cannot be captured from the outputs of physics-based computer models in order to improve predictive capabilities of a digital twin [Citation78]. The implementation of this approach is commonly focused in areas of driving behaviour, semi-autonomous and autonomous control techniques in vehicle studies.
5.8. Output visualisation
The output visualisation is well developed in vehicle dynamics simulators and post-processing analysis tools. However, considering the nature of digital twins, it requires to use different environmental dimensions through the entire life cycle of a system generally as shown in Figure . The output visualisation will be represented by new aspects that have never previously been considered by vehicle dynamicists in the traditional models.
Figure 12. Different dimensions as a subject of consideration in digital twin studies [Citation90].
![Figure 12. Different dimensions as a subject of consideration in digital twin studies [Citation90].](/cms/asset/6391f1b5-718b-445a-9efb-70431ace94f6/nvsd_a_2188228_f0012_oc.jpg)
6. Digital twins in the rail domain
The application of digital twins in the rail domain has been extensively analysed and summarised recently through the analysis of publications related to digital twins in several articles [Citation9,Citation99,Citation100,Citation102,Citation103]. Based on the analysis data, the main areas of digitalisation in rail transport with the usage of rail vehicle dynamics studies are:
Design
Safety analysis
Maintenance
Monitoring
Optimisation.
6.1. Design
The design aspects are generally related to the design of rolling stock and the design of track infrastructure and bridges. The digital twin for the design of a rail vehicle is identified as the outcome of the virtual product development process when ‘the virtual prototype transits towards a digital twin of the specific & unique real-world component or system’ where ‘numerical modelling techniques are necessary to be able to provide high-fidelity assessment of the dynamic behaviour of the vehicle and track interaction’ [Citation99]. The work published in [Citation100] also presents similar ideas as shown in Figure with the additional statement that digital twins of freight rolling stock should ‘correctly assign the service life, overhaul times, and increase operational efficiency, collect information about the real load’ [Citation100]. The transformations in design approaches presented in Figure are possible by means of application of data processing algorithms for ‘Smart Big Data’ [Citation100]. However, neither of these publications discuss how the numerical models should be applied in such a development. It is possible to understand the details provided in [Citation101] considering the level of integration of digital representations for the digital twin design approaches mentioned in [Citation100] as follows:
A digital model is built when the digital representation of the existing or planned physical system is based on manual data flow exchange between the digital object and the physical system, i.e. it does not use automated data exchange between the digital object and physical system.
A digital shadow is built on an automated one-way data flow between the existing or planned physical system and the digital object. However, the second data exchange direction is built on a manual flow data exchange.
A digital twin is built on the concept that automated data flow occurs between the digital object and the physical system in both communication directions.
Figure 13. Difference in approaches to digital freight rolling stock design [Citation101].
![Figure 13. Difference in approaches to digital freight rolling stock design [Citation101].](/cms/asset/486d9507-128d-41c8-854b-54bcb3901b17/nvsd_a_2188228_f0013_ob.jpg)
Another discussion on the implementation of digital twins in locomotive design and the methodology of its application was published in [Citation9]. The V-model approach for locomotive design involving several cycles with many stages of system integration activities during the development process is shown in Figure . While Stages 1 and 2 are well known to vehicle dynamicists, Stage 3 introduces a digital twin in the locomotive design process. As stated in [Citation9], this includes not only full-mechatronic models of the rail vehicles in the train but also a virtual train driver. Stage 3 should be implemented on the virtual simulation platform that is commonly built on a High Performance Computing (HPC) cluster. As a result, the digital twin model is built using a parallel computing and co-simulation scheme that allows software packages for longitudinal train dynamics, vehicle dynamics and track dynamics (where a track model is implemented as a separate model) [Citation104–108] to communicate with each other. The process commonly uses individual independent processor cores on the HPC to simulate each vehicle (implemented in a multibody software package) in the train as it travels over the whole railway route. An example of a typical parallel computing scheme is shown in Figure , where Core 1 to Core n can start their single VSD simulations in parallel [Citation109]. Individual cores then communicate with the longitudinal train dynamics simulation through the co-simulation interface to replicate actual train behaviour by means of the application of digital twin technologies. This commonly requires significant computational resources. Some similar ideas on the computational platform for a railway digital twin tool can be found in [Citation110].
Figure 14. Proposed V-model with several system integration stages for the design of a locomotive product [Citation9].
![Figure 14. Proposed V-model with several system integration stages for the design of a locomotive product [Citation9].](/cms/asset/881cadfa-2c6f-422d-b46c-f79bcb953684/nvsd_a_2188228_f0014_ob.jpg)
Figure 15. Example of parallel computing scheme [Citation109].
![Figure 15. Example of parallel computing scheme [Citation109].](/cms/asset/b762e36c-5e86-4b4e-8a6e-78a3da8e6657/nvsd_a_2188228_f0015_oc.jpg)
6.2. Safety analysis
As stated in [Citation111], the objective of safety analysis is to apply the knowledge of the system safety process in a digital twin to provide design proposals for improving safety in the railway system. There are now increasing applications in this specific area [Citation110] considering that the modernisation or modification of existing rolling stock designs are often required, and it seems quite reasonable that the digital twin techniques can be applicable in such safety analysis exercises.
An example can be found in [Citation112] where the design of a non-standard load sharing wagon consist for transporting rails was assessed by means of the development of a digital twin of an 11-wagon consist. The operational validation was based on the existing Australian rail vehicle roadworthiness acceptance standard AS7509:2017 published by the Rail Industry Safety and Standards Board (RISSB). The design methodology used in this study is shown in Figure (a) along with the modelling approach in 16(b).
Figure 16. Digital twin study for the modification of an 11 wagon consist [Citation112]: (a) V model of the design methodology and (b) modelling methodology.
![Figure 16. Digital twin study for the modification of an 11 wagon consist [Citation112]: (a) V model of the design methodology and (b) modelling methodology.](/cms/asset/832d0fac-f5b9-457e-83e1-13c5a4116d8f/nvsd_a_2188228_f0016_oc.jpg)
6.3. Maintenance
Analysing publications [Citation113–120], it can be easily found that the digital twins are commonly related to three maintenance problems: wheel-rail interface, track components and rolling stock components. Considering that the purpose of this paper is not to discuss maintenance criteria but to instead concentrate on the possible digital twin ideas and architectures, it is possible to see three common trends. The first trend in digital twin maintenance studies can be covered by papers [Citation113–117] and it focuses on the application of advanced simulation models of locomotives, wagons, and track combined with train operational scenarios to calculate long-term rail surface damage using a 1-D longitudinal train simulation and a 3D multibody simulation of multiple vehicles in parallel. Various wheel-rail contact damage models that are used in this specific area allow railway network owners to improve maintenance grinding intervals and impose restrictions on the operational behaviour of trains on the track in order to limit possible track damage. This analysis process assumes that such advanced simulations can ‘be tuned into a digital twin to guide infrastructure managers regarding the condition of rail surface as a function of tonnage passage’ [Citation115]. The methodological framework can be defined as shown in Figure .
Figure 17. Methodological framework as a core for a digital twin in rail surface damage (RSD) study [Citation116].
![Figure 17. Methodological framework as a core for a digital twin in rail surface damage (RSD) study [Citation116].](/cms/asset/f6ae5609-9326-43b5-8050-7e7b0cb2f335/nvsd_a_2188228_f0017_oc.jpg)
The second trend is focused on studies related to wheel and rail material properties that can then be introduced in simulations. As a result, the following statement in [Citation118], which is related to work published in [Citation119] that focuses on the development and application of an in-house software package for the simulation of wheelset stresses, is worthy of close consideration:
Integrated simulations of the deterioration of a complex structure can be done by using data from sensors as inputs to a large physical model. This is part of the idea of a digital twin, which consists of a comprehensive physical and functional description of the component, product or system.
Further development of this idea has been advanced in work [Citation120] that proposes a simulation modelling method to predict rail surface damage based on a locomotive digital twin integrated with calibrated shakedown maps and friction measurements to produce a stress occurrence shakedown heatmap.
The third trend is focused on the usage of a digital twin for prediction of maintenance for the components of rolling stock. For example, the work [Citation121] proposes a complex equipment health management architecture based on digital twin technologies and utilising deep learning algorithms to make maintenance decisions regarding components of the powered bogie of an urban rail transit vehicle. The implementation of the proposed digital twin solution was described with the following steps as:
‘The historical operation data and real-time operation data of complex equipment entity are transmitted to the operation data processing module.
The operation data processing module analyses, processes and refines the data to obtain the change value of twin body parameters.
The parameters obtained from the operation data processing module are used to modify the digital twin of complex equipment.
Run the digital twin and get the corresponding simulation results.
The operation results of the digital twin are transmitted to the prediction and maintenance decision module. Planning maintenance tasks and predicting future health status of complex equipment’.
As it is possible to observe from the review of all three trends, they all require the usage of advanced modelling approaches based on rail vehicle dynamics to achieve their stated goals in predictive maintenance.
6.4. Monitoring
Condition assessment of railway vehicles, track and bridges is one of the critical tasks in railway operational practice. It is clear that better monitoring and analysis of the fatigue of such structures can be carried out with the full implementation of the digital twin concept [Citation122]. There are a limited number of publications in this area with the implementation of vehicle dynamics available in the public domain. In Pillai et al. [Citation123], it is stated that a combined numerical simulation approach based on simplistic multi-body simulation and finite element analysis was developed to obtain the outputs necessary to predict the fault locations and determine sensor placements for a track switch condition monitoring system with the further focus on the development of digital twin models requiring that there is the ‘availability of data for live traffic, of different routes [that] could enable intelligent decisions for supporting condition monitoring and risk-informed predictive maintenance’. This conditional statement leads us to review the work [Citation124] that shows how a monitoring system needs to connect all the elements of available information in one data flow as shown in Figure . The digital twin of the bogie of a high-speed train in [Citation124] was built based on four classical subsystems: ‘a detailed 3D model, FEM models of key parts, a multibody model, and the computation of theoretical characteristic frequencies’. Again, it confirms that the application of vehicle dynamics should be considered in digital twins for precise monitoring and decision making systems.
Figure 18. The flow of information in the monitoring system [Citation124].
![Figure 18. The flow of information in the monitoring system [Citation124].](/cms/asset/f187febf-e73f-46bc-8269-2f08e25d6061/nvsd_a_2188228_f0018_oc.jpg)
6.5. Optimisation
The literature review shows that the optimisation with the usage of digital twins is progressing in two areas:
Energy analysis of train operational scenarios;
Train driving strategies.
Figure 19. Architecture of the multi-locomotive co-simulation approach for locomotive/track damage studies [Citation126].
![Figure 19. Architecture of the multi-locomotive co-simulation approach for locomotive/track damage studies [Citation126].](/cms/asset/cb273365-60c7-491e-9224-9f86af4141eb/nvsd_a_2188228_f0019_oc.jpg)
The optimisation of train driving strategies commonly leads to the development of new intellectual property that is unable to be published in the public domain for commercial reasons. Therefore, the published information is quite limited for detailed analysis of a structure of a digital twin. However, the publication [Citation127] presents the five-dimensional model of a digital twin that was built on simplistic dynamic equations to represent train/vehicle dynamics to support the automatic train operation performance optimisation as shown in Figure .
Figure 20. Framework of digital twin simulation platform [Citation127].
![Figure 20. Framework of digital twin simulation platform [Citation127].](/cms/asset/16042d5e-f237-4720-aed9-066b5a99efcb/nvsd_a_2188228_f0020_oc.jpg)
As a result, there is significant opportunity for new developments in this area, not only in terms of building new simulation platforms, but also in the development of new processing algorithms.
7. Digital twins in the road domain
Digital twin applications in the automotive context have recently been summarised in several review articles and from different points of view, ranging from production [Citation46], predictive maintenance [Citation42], connected and automated vehicles [Citation13,Citation128], driver models [Citation129], to autonomous driving test methods based on digital twins [Citation130]. Bhatti et al. [Citation87] and Piromalis and Kantaros [Citation131] analyse digital twin technologies in the area of smart electric vehicles and from a rather general perspective. The former review is systematically classified in several (sub-)categories as shown in Figure which also highlights the various fields of application where digitals twins have made their way into the automotive domain. Digital twin applications in those fields, which are of most interest from a vehicle system dynamics perspective, are discussed in the following sub-sections.
Figure 21. Various fields of applications of digital twin technology in the automotive industry [Citation87].
![Figure 21. Various fields of applications of digital twin technology in the automotive industry [Citation87].](/cms/asset/a3d94306-8452-4a57-9074-d4ffd96d736a/nvsd_a_2188228_f0021_oc.jpg)
7.1. Predictive maintenance and monitoring
Arena et al. [Citation42] provide a systematic literature review of statistical inference approaches, stochastic methods, and AI techniques for predictive maintenance in the automotive sector. Therein, several approaches for predictive maintenance are analysed: (i) physical model approach, where the process of degradation of a component is directly described by a physical-mathematical model; (ii) knowledge-based approach, where rule-based models aim to simulate the skill and behaviour of experts regarding decision-making; (iii) data-driven approach, where, via the use of statistical, stochastic or traditional machine- and deep learning techniques, a large amount of data and computational power are used to analyse complex systems whose evolution over time is not easily predictable otherwise; (iv) digital twin approach, where the data and models are combined, and the virtual instance of a product is continually updated with its operational data, which ‘opens the way for real-time monitoring of the entire lifecycle of that product’ [Citation42]. However, it remains open what techniques should be used to track the parameter alteration of the physical twin, and to extract the desired information on the performance, maintenance and health status from its digital twin.
Ezhilarasu et al. [Citation132] investigate the role of digital twins in the field of Integrated Vehicle Health Management (IVHM) mainly for aerospace and aircraft vehicles. The authors recommend relying on well-known machine learning and AI techniques from the past decades; the main progress however, besides advancements in sensor technologies and the increased amount of data, lies in the input domain knowledge for digital twins, which can be in the form of rules, ontologies, procedures, and sensor data. Data driven methods for predicting vehicle maintenance have been thoroughly investigated in [Citation75]. Although prior to digital twin applications, some features thereof are found in the two main approaches considered in the thesis, which are:
unsupervised self-learning methods for predictive maintenance based on on-board data with data sent wirelessly to a back-office system for analyses and anomaly detection;
supervised classification of off-board data sources; historical raw data (which are potentially collected for other than maintenance reasons) as well as the repair history data form a large knowledge base that is mined to find patterns, and to match them to subsequent repairs.
Aspects related to data pre-processing, feature selection, self-organising models, and handling unbalanced datasets are covered as well. Notably in these studies, extensive data sets are used which stem from real-world settings with multiple vehicles during their daily operations.
A number of applications of digital twin systems for monitoring, diagnostics, and predictive maintenance for the automotive sector can be found in literature. Algin et al. [Citation133] build a digital twin of vehicle planetary transmissions consisting of five models (structural, kinematic, dynamic, general reliability, and individual diagnostic). The digital twin is used to provide online monitoring of the transmission in operation, and to calculate the lifetime expense based on current data. A data-driven digital twin of a turbocharger of a commercial vehicle is developed in [Citation134] to predict failures based on available CAN parameters, and good agreement to actual failure data and service history are reported. However, less information on chosen methods are available in the paper. A model-driven approach towards health monitoring and predictive maintenance of an automotive braking system is presented in [Citation135]. Multiple modelling formalism are used for the electrical, pneumatic, hydraulic, mechanical, and control-logic based key components to create what the authors refer to as a ‘digital twin’ of the braking system of a conventional passenger vehicle; however, since communication to a physical vehicle/braking system is missing, we rather refer to a multi-physics model in this case. Via respective simulation studies, brake-pad wear rates under normal and abnormal conditions with/without ABS intervention were analysed, and effects related to faulty wheel-speed sensors are discussed. Similarly, Venkatesan et al. [Citation136] derive a data-driven digital twin for health monitoring and prognosis of an electric vehicle motor. While measurement data of an experimental vehicle is used to derive the black-box model of the digital twin, its remaining useful battery life and time to refill the bearing lubricant are calculated by simulation, no comparison to real-life health status of the engine is provided. Scurria et al. [Citation137] create a digital twin of the anchorage of a truck axle from a finite element model using model order reduction; in combination with strain gauges on the original vehicle, the digital twin is used as a virtual sensor for (real-time) monitoring of the strains at hotspot locations that are not accessible otherwise.
Battery technology of (smart) electric vehicles and their potential digital twin applications naturally arouse special attention in recent literature, ranging from engineering design and production to monitoring of state of charge (SOC) and state of health (SOH) to recycling aspects of batteries [Citation138]. An accurate monitoring of SOC and SOH is important for effective health and energy management to ensure good performance and long life of batteries. Suitable methods for estimating these quantities are divided by [Citation138,Citation139] into: (i) model-based approaches (e.g. using the relationship between open circuit voltage and SOC), (ii) filter based approaches (Kalman filter including nonlinear variants, particle filter) and (iii) data-driven approaches (fuzzy logic, neuronal networks, state machines).
Battery management systems (BMS) are an extensive part in the review of [Citation87] on smart electric vehicles. The authors conclude that present battery management technology is ‘far from supplying accurate results in a real-time scenario for wide operating ranges’ and several contributions are discussed where digital twins of batteries are explored to improve the performance and robustness of BMS. Similarly, Ghosh et al. [Citation138] argues that the prediction performance of an individual digital model of a battery can be improved when integrated in a digital twin environment. The authors identify further benefits of digital twins of batteries in four potential aspects as shown in Figure . The work [Citation140] discusses a proof of concept of a cloud-based digital battery twin in an experimental setup for an electric car. Single digital twins for each of the hundreds of lithium-ion cells are combined to a complete model of the battery pack, running in a cloud system. Data from the on-board diagnostic system are sent to the twin via a 4G cell network, and open circuit voltage and internal resistances of the cells are estimated as an indication of the SOC and SOH of the battery. A thorough description of the creation of the digital twin setup and of respective challenges is provided in the paper.
Figure 22. Potential aspects of digital twins of battery management [Citation138].
![Figure 22. Potential aspects of digital twins of battery management [Citation138].](/cms/asset/5c5ae336-4d0b-4658-863f-77f794559128/nvsd_a_2188228_f0022_oc.jpg)
7.2. Connected and automated road vehicles
The state of the art of modelling, control and optimisation for connected and automated vehicles (CAVs), i.e. automated road vehicles ‘that may utilise wireless vehicle-to-everything (V2X) connectivity’, has recently been reviewed in [Citation141] from a vehicle system dynamics perspective, along with aspects related to safety verification, energy efficiency, traffic dynamics, and related future challenges. The review includes vehicle models, motion planning and control techniques for longitudinal, lateral and combined fully autonomous vehicle control of single vehicles. In addition, different aspects of connected vehicles (to each other, to the environment, or to computation power in the cloud) are considered, such as vehicle platooning for improved energy efficiency, or hardware in the loop testing of powertrains via cyber-physical testbeds for improved fidelity, e.g. to evaluate the above control techniques. Traffic scenarios are considered in terms of (string) stability of automated longitudinal control, and connected cruise control and cooperative adaptive cruise control concepts are discussed, where an automated vehicle uses motion signals from surrounding vehicles and/or traffic perturbations from fixed spatial locations via V2X communication, which ‘opens an avenue […] for controlling the large-scale traffic dynamics leading to increased traffic flux and reduced travel time’ [Citation141], which obviously points in the direction of the application of digital twins. Future challenges are summed up as: (i) inclusion of data-augmented models and numerical control design techniques, (ii) verification of those methods as well as of large V2X systems, (iii) reliability and security of the V2X communication, (iv) requirements of the physical and cyber infrastructure for the deployment of connected and automated vehicles on public roads, among others.
Based on the review above, we focus in the following on literature directly related to digital twins, and on aspects that their applications can contribute to CAVs. Since there is already a large number of papers published, we refer to recent literature reviews as well as some additional examples of digital twins in the context of CAV.
The publication [Citation13] reviews the role of digital twins in the context of connected and automated vehicles. The authors provide a general introduction to digital twins, and regarding their definition, they refer to the five-dimensional model of Tao et al. [Citation62] as presented in Section 4, and insist on a permanent communication between physical and digital twins, which is argued to distinguish digital twins from other advanced methodologies related the model-based design or virtual proving grounds, which are often rather based on iteration between the virtual and physical world than on synchronisation. The authors provide a careful review of the digital twin literature with a focus solely on CAVs. The cited papers are grouped into: (i) unmanned autonomous vehicles, or examples from the model-based design that do not strictly fulfil the above definition of digital twins, (ii) automated driving system testing, including large-scale test facilities such as Michigan’s MCity [Citation143], (iii) cyber-physical systems for CAVs that include a model of the human driver and/or the surrounding traffic scenario, typically simulated in the cloud, and (iv) parallel driving, which refers to a concept similar to digital twins, where the physical world, mental world, and artificial world are modelled as three parallel levels, considering interactions among connected vehicles, human drivers, and information [Citation57]. Regarding challenges, the authors add ‘user trust’ to the list above, as well suitable user interfaces, which allow the human user to understand the digital twin performance.
Guo et al. [Citation142] provide a survey on digital twins for the Internet of Vehicles (IoV), which refers to the case where ‘vehicles and traffic systems are modeled as the target objects to form a mapping between virtual spaces (in the server) and physical spaces (on the road)’. A thorough summary of papers is presented, where digital twins are used to facilitate the design, evaluation, and deployment of IoV-based systems. Based thereon, the authors differentiate between a ‘single vehicle with digital twins’ and a ‘traffic system with digital twins’, with the former focusing on the operation of the vehicle itself and the human–vehicle coordination, and the latter focusing on the interaction and synergy among the participants of the traffic system and its environment. Similarly, intra-vehicle and inter-vehicle networks and their architectures are discussed. Intra-vehicle networks operate autonomously within a vehicle and organise the communication between its devices (sensors, controllers, actuators) as well as the communication with and/or the monitoring of the driver. Based on inter-vehicle and V2X communication, the authors outline a digital twin of an entire traffic system, see Figure , which comprises ‘distributed digital twins in edge-cloud collaborative systems, where edge servers store digital twin models of a small area, and a cloud server coordinates them to form an overall one’ [Citation142]. Such a system potentially enables to predict and evaluate the feasibility of certain traffic control strategies. The main services are generally summed up as risk avoidance, itinerary planning, flow control, scheme simulation, and space management.
Figure 23. Illustration of a digital twin of an entire traffic system [Citation142].
![Figure 23. Illustration of a digital twin of an entire traffic system [Citation142].](/cms/asset/a0b1f5f7-2f43-4881-ae3e-3f0dbf56751f/nvsd_a_2188228_f0023_oc.jpg)
Obviously, many technological challenges need to be overcome for realising the above system, ranging, e.g. from machine perception, trajectory planning and decision making in complex traffic scenarios, verification and validation, cyber-security, to the interaction between (automated and human controlled) vehicles, and human-machine interaction [Citation144]. The work [Citation145] presents a working prototype of a real-time cooperative perception system for a digital twin of a traffic environment. The authors introduce their so-called ‘Central System’ architecture, which aims to enable a sharing and integration of information among traffic participants and infrastructure. As a part thereof, the presented ‘Central Perception’ system collects data from both stationary and moving sensors and performs multiple levels of data integration ‘to finally create and visualize a simultaneous, centrally consistent model (digital twin) of all objects of interest in the area covered by the sensors’ field of views’ [Citation145]. The key elements of the systems comprise the calibration of the perception modules (LiDAR, camera, IMU/dGPS), 3D-object detection and tracking, data communication, synchronisation, fusion, and the visualisation in terms of a 3D rendering of the environment combined with the perceived dynamic objects (here: vehicles and pedestrians) by relying on Unity 3D software, see Figure . An overall latency of less than 100 ms was achieved. Thus, the system may serve for future services such as traffic planning, or cloud-assisted and cloud controlled ADAS functions. Readers may also refer to this paper for an overview of similar prototypes realised by other research groups.
Figure 24. Objects sensed by different sensors of the infrastructure (left) and the vehicle (top-right), respectively, and visualisation of the real-time digital twin (bottom-right) [Citation145].
![Figure 24. Objects sensed by different sensors of the infrastructure (left) and the vehicle (top-right), respectively, and visualisation of the real-time digital twin (bottom-right) [Citation145].](/cms/asset/555891ee-f58c-448d-b473-f4b87d88c10d/nvsd_a_2188228_f0024_oc.jpg)
The interaction between automated and human-driven connected vehicles is investigated in [Citation146]. The authors develop a digital twin-assisted cooperative driving system, aiming to allow the connected vehicles to pass non-signalised intersections without stopping. Therefore, an enhanced first-in-first-out slot reservation algorithm is designed, and the planned sequence of crossing vehicles is handed over to a consensus motion control algorithm. Factors such as road grade or acceleration abilities of the individual vehicles are considered, as well as uncertainties regarding the wireless communication in terms of time delays and packet losses. The generated vehicle speed trajectories serve as input for the CAV; for the human-driven connected vehicles, a human–machine interface is developed that uses augmented reality to visualise the reserved slots, assisting the driver to conduct cooperative driving behaviour. The system is tested in a human-in-the-loop simulation environment, and an average of 20% reduction in travel time (and a comparable reduction in estimated fuel consumption) was found compared to traditional signalised intersections. In similar studies of the research group, real-world implementations and the underlying system architecture of digital twins in traffic scenarios are reported as well [Citation57,Citation147].
7.3. Human driver digital twin
Hu et al. [Citation129] review the digitisation of the human driver within intelligent driving and transportation systems. The authors further propose a concept of a ‘driver digital twin’ as an essential element for ‘constructing a harmonious human-centric intelligent driving system that considers the proactivity and sensitivity of the human driver’. It is argued that the individual personalities and preferences of human drivers need to be accounted for (partially or fully) in automated driving systems in order to improve acceptance and trust in intelligent vehicles. Similar to digital twins of machines, there should be a real-time and life-cycle lasting synchronisation between a human driver and its virtual counterpart in order to identify his/her individual characteristics in a timely manner. This potentially allows for an individual adaption of the behaviour of the vehicle autonomous driving functions or assistance systems to the driver’s likings on one hand, and/or to quickly react on occurring anomalies (such as a sudden degradation of the human driver’s capabilities) based on current and historic data on the other hand. An overview of the proposed system is shown in Figure . The authors review the related technologies necessary to ‘measure’ the states of the real driver (i.e. the left upper block in Figure ) by means of vision-based, physiological-based, and driving-based sensors; in addition, representative applications of personalised ADAS systems and human-machine interfaces are summarised from literature. However, it remains unclear what modelling techniques should actually be used to model the digital twin of the driver. Further recent reviews on driver monitoring and personalisation techniques for ADAS can be found in [Citation148,Citation149].
Figure 25. Overview of the digital twin system of a human driver, including the real driver, a multimodal interface and possible applications for intelligent driving systems [Citation129].
![Figure 25. Overview of the digital twin system of a human driver, including the real driver, a multimodal interface and possible applications for intelligent driving systems [Citation129].](/cms/asset/78a7250d-d05b-4805-8073-7907c357bc95/nvsd_a_2188228_f0025_oc.jpg)
In a recent study, Best [Citation150] presents a model which realises a real-time characterisation of driver steering behaviour based on a single-track vehicle model and an unscented Kalman filter. In the introduction to the paper, an overview on current driver models, the correlation between respective model parameters and driver awareness and skill and driving style/behaviour identification is provided. Referring to a frequent limitation to emergency manoeuvres, the author remarks: ‘The gold standard would be to deduce driver skill from normal driving in advance of an emergency, and also to take note of the variations in awareness and skill which most drivers experience over time – often within single journeys’. In experiments on a motion simulator and the public road, the presented model proved ‘simple but effective’ in robustly and accurately replicating the individual steering behaviour of five test drivers on open single carriageway roads in real-time, based on the known road geometry and (CAN-bus) data of steering angle and vehicle forward speed. Although not directly referred to as a digital twin by the author, the proposed model might be a well-suited ingredient for an approach as described in the previous paragraph.
A decidedly termed digital twin approach for a behavioural model of drivers is discussed in [Citation151]. The authors propose a framework for human-driven connected vehicles, where driver intention prediction is performed within each vehicle, and the information is then shared with the neighbouring vehicles in real time to estimate the risk of a future collision, and to provide a warning to the drivers, if necessary. The modelling of the human decision making and the traffic context is based on Decision Tree and k-Nearest Neighbour machine learning techniques, and Markov Decision Process in discrete time, respectively, including the vehicle dynamics as a separate block box. The ability of the approach to prevent collisions is demonstrated for a highway driving scenario in a virtual driving simulation environment.
7.4. Vehicle testing
Various strategies for testing, evaluation and validation of advanced driver assistance system (ADAS) and automated driving systems based on simulation and/or field operational tests have been proposed in literature, see examples in [Citation152,Citation153]; recently, digital twin applications are discussed for this purpose as well [Citation154–157]. Digital twins of entire testing environments of a proving ground with the purpose of testing and validation of automated vehicle systems are presented in [Citation158,Citation159], along with overviews of current testing frameworks for automated vehicle systems and related state of the art methods used by the automotive industry. Before referring to the latter in more detail, we first mention several concepts which build on a reduced level of integration of physical and virtual objects and data.
Zhou et al. [Citation160] present a framework for virtual testing of ADAS, where environmental conditions obtained in the real world (in terms of road and other traffic participants) are reproduced within a simulation environment. Since this process of data import and scenario setup is performed automatically, the framework may be considered a ‘digital shadow’ according to [Citation101]. Similarly, an approach based on a one-way data flow from physical to virtual space for testing of software decisions is reported in [Citation161]. In the course of the so-called ‘shadow testing’, a software application is running in the background of a real vehicle’s system during everyday use, receiving sensor data but not taking any control action; instead, the decisions made by the software application are compared to those of the human driver (or to older software versions) and evaluated accordingly.
Other strategies for an evaluation of ADAS build on a fusion of real-world and virtual data, i.e. concepts where ‘measured and simulated environmental aspects are augmented and aligned in order to test ADAS on both worlds’ [Citation152]. The survey quotes several approaches, in which recorded video sequences from real test drives are augmented with synthetic (simulated) camera views, e.g. to evaluate the environmental perception capabilities of the system under test. The fused data may be used as input to the ADAS function of either the simulation model or of a real vehicle [Citation162].
A framework which allows for an arbitrary partitioning of virtual and physical elements of a test scenario is presented in [Citation159]. The so-called Scenario-in-the-Loop concept builds on a digital twin of the entire testing environment, comprising the vehicle under test, the V2X communication, infrastructure elements (e.g. traffic lights, road lighting), movable targets (e.g. pedestrian, bicycle), and (remotely controlled) real vehicles, which may be combined to create complete traffic scenarios. In addition, and due to the parallel and simultaneous course of such a scenario in physical and virtual space, real-world and simulated data can be exchanged freely among the above elements and the central control system of the testing environment. This enables to determine individually, which part of the tested scenario is executed in reality, and which part is performed by simulation. Thus, ‘the main advantage is that the concept can be applied incrementally, starting from full simulation and piece-by-piece replacing the virtual elements to real elements until the optimum level, while running the same test sequence within the same environment for a specific traffic scenario’ [Citation159]. Figure shows the architecture of the framework, and the main building blocks and the underlying technologies with respect to localisation, communication, interfacing, and visualisation are discussed in detail within the paper. Finally, a proof-of-concept demonstration is reported of two different scenarios including an unexpected pedestrian crossing and a car-following situation. Both scenarios were performed with either a virtual or a real target object, and the same reaction of the vehicle under test could be observed in both cases.
Figure 26. Architecture of the Scenario-in-the-Loop validation methodology in the form of a digital twin of a testing environment of a proving ground [Citation159].
![Figure 26. Architecture of the Scenario-in-the-Loop validation methodology in the form of a digital twin of a testing environment of a proving ground [Citation159].](/cms/asset/c4d213e0-4d68-413c-ae3e-6be3bbb0d9fd/nvsd_a_2188228_f0026_oc.jpg)
In addition to active driving systems, optimised passive safety elements such as crash cushions and road safety barriers may contribute to improved road safety outcomes as well, and modern numerical methods are required to capture effects of large deformations and nonlinear friction processes during impact simulations. An approach for combined modelling of vehicle and barrier is discussed in [Citation163], and the author refers to the simulation model as a ‘digital twin’, motivated by results from validation analyses; however, no (real-time) connection between physical and virtual space is mentioned in the paper.
7.5. Miscellaneous
The road is an essential element of the vehicle–environment system for both the physical and the virtual twin. High-definition maps contain static information on many crucial aspects of a roadway, e.g. geometric data (curvature, slope, width of a road/lane, etc.) and roadside data (traffic regulations, obstacles, etc.) with an accuracy of a few centimetres [Citation164]; to dynamically account for the actual road conditions (i.e. those closely ahead of a vehicle), cloud/edge computing is proposed in [Citation165] to include data from preceding vehicles (or specifically designed roadside units [Citation166]) to create a digital twin of a road.
Furthermore, Kaliske et al. [Citation167] envision a digital twin of the entire road-tyre-vehicle system for future mobility, which ‘will allow for more precise forecasts of road status and durability, optimization of maintenance intervals, as well as optimization of the loading of the road’. Physics-based and data-based modelling strategies for tyre, road and the interaction among them are discussed. The physics-based models comprise finite element models of tyre and pavement that take their temperature-dependent behaviour as well as a potential thermomechanical coupling into account. Time homogenisation is proposed to account for the long-term behaviour, as well as different methods to deal with uncertainties in the available data. Contributions of smart tyres and road sensors to monitor tyre-road friction properties have recently been reviewed in [Citation168,Citation169].
Finally, several studies can be found in literature where the term digital twin is used for a (multi-physics) simulation model rather than for a system featuring a virtual-physical connection: Naets et al. [Citation170] aim for a combined identification of vehicle suspension parameters and road profile; Fietkau et al. [Citation171] describe the process of an entirely virtual powertrain development, incorporating all relevant phases, components and simulation disciplines of the development process; Rahate et al. [Citation172] derive a model for an electric three-wheeler vehicle, taking basic mechanical, thermal and electric properties into account in order to optimise the driving range with regard to realistic driving cycles; Foldager et al. [Citation173] discuss the steps necessary to develop a digital twin of an autonomous agricultural vehicle.
8. Discussion and conclusions
The digital twin development process can be explained by a simplistic statement that computers are now much faster and computational capabilities are significantly increased. If we consider the Modelling, Simulation, Information Technology and Processing Technology Area Strategic Roadmap (TASR) developed by NASA that was presented in [Citation2] and the recent developments made in vehicle system dynamics, it is possible to expect the following scenarios in the developments in four core areas of computing, modelling, simulation, and processing as shown in Figure . The analysis performed in this paper as well as the presented roadmap shows that the development of digital twin simulations and architectures is continually progressing, and this is expected to continue if there is ongoing progress in the development of computing, simulation and information technology to accelerate the performance of big data processing that should allow more precise governing of vehicle dynamics system behaviour in its operational environment.
Applications in both rail and road can potentially be extensive in addressing operation, energy, safety and maintenance objectives. Again, in both rail and road applications much depend on the availability and resolution of the infrastructure data. Furthermore, digital twins require a process of making the models specific to each real-world system modelled, so detailed validation and accounting of variations in operational conditions is required. Importantly, this differs from the typical historical application of modelling and simulation which has had either a focus on an ‘average model’ for the fleet, or a focus on worst case conditions.
In rail, advanced modelling techniques have allowed whole trip and whole network operational longitudinal simulations and detailed analyses. Where the rail networks are new and digital survey data exists, all the features are present for digital twin applications. It should also be remembered that the data resolution required for operational studies can usually be achieved using rolling stock and track design data and current speed limit information. Taking the digital twin to the further step of providing rail vehicle dynamics requires up to date wear and degradation data, in regard to both track surface condition and vehicle condition. Data at this level of detail still presents considerable difficulty due to the resolution and volume required. It is expected then that detailed vehicle dynamics digital twins will be offline applications for many applications, whilst digital twins of whole network operation will be easily realised in train track systems with modern survey and rolling stock information.
In road, advanced modelling techniques are progressing more quickly in comparison with rail studies due to the easier opportunities to the transition of testing outcomes into digital twins and this gives quite promising outcomes for the development of new adaptive and interpretable ways of transition of vehicle physics-based models into digital twins and its further potential application in the rail domain.
Summarising all of the above, it is possible to say that this paper has only provided some directions to assemble the theory for the development of digital twin techniques considering multidisciplinary approaches. The existence of a great number of uncertainties allows concluding that the presented and reviewed digital twin building concepts and predictions should be considered as a wish list, and it is still not clear which one of implementation ideas/variants could come true and represents the right way at the end of this exciting journey in digital twin technologies.
Acknowledgements
The editing contribution of Mr. Tim McSweeney (Adjunct Research Fellow, Centre for Railway Engineering) is gratefully acknowledged.
Disclosure statement
No potential conflict of interest was reported by the author(s).
References
- Grieves M, Vickers J. Digital twin: mitigating unpredictable, undesirable emergent behavior in complex systems. In: FJ Kahlen, S Flumerfelt, A Alves, editor. Transdisciplinary perspectives on complex systems. Cham: Springer-Verlag; 2017. p. 85–113.
- Shafto M, et al. Modeling, simulation, information technology & processing roadmap. Washington (DC): National Aeronautics and Space Administration; 2012. Available from: https://www.nasa.gov/sites/default/files/501321main_TA11-ID_rev4_NRC-wTASR.pdf.
- Haag S, Anderl R. Digital twin – proof of concept. Manuf Lett. 2018;15:64–66.
- Liu M, Fang S, Dong H, et al. Review of digital twin about concepts, technologies, and industrial applications. J Manuf Syst. 2021;58(Part B):346–361.
- Schluse M, Priggemeyer M, Atorf L, et al. Experimentable digital twins—streamlining simulation-based systems engineering for industry 4.0. IEEE Trans Ind Inf. 2018;14(4):1722–1731.
- Xu Y, Sun Y, Liu X, et al. A digital-twin-assisted fault diagnosis using deep transfer learning. IEEE Access. 2019;7:19990–19999.
- Luo W, Hu T, Zhang C, et al. Digital twin for CNC machine tool: modeling and using strategy. J Ambient Intell Humaniz Comput. 2019;10:1129–1140.
- Wang P, Yang M, Peng Y, et al. Sensor control in anti-submarine warfare—a digital twin and random finite sets based approach. Entropy. 2019;21(8):767.
- Spiryagin M, Wu Q, Polach O, et al. Problems, assumptions and solutions in locomotive design, traction and operational studies. Railw Eng Sci. 2022. DOI: 10.1007/s40534-021-00263-w.
- Wright L, Davidson S. How to tell the difference between a model and a digital twin. Adv Model Simul Eng Sci. 2020;7(13):1–13.
- West T, Blackburn M. Is digital thread/digital twin affordable? A systemic assessment of the cost of DoD’s latest manhattan project. Procedia Comput Sci. 2017;114:47–56.
- Defense Acquisition University. Digital twin. DAU Glossary of Defense Acquisition Acronyms and Terms. [cited 2022 May 25]. Available from: https://www.dau.edu/glossary/Pages/Glossary.aspx#!both|D|27349.
- Schwarz C, Wang Z. The role of digital twins in connected and automated vehicles. IEEE Intell Transp Syst Mag. 2022. DOI: 10.1109/MITS.2021.3129524
- Hughes A. Forging the digital twin in discrete manufacturing: a vision for unity in the virtual and real worlds. LNS Research’ 2018.
- Tao F, Qi Q. Make more digital twins. Nature. 2019;573:490–491.
- Computer Simulation technical Committee on Model Credibility. Terminology for model credibility, reports of SCS technical committees. Simulation. 1979;32(3):103–104.
- Cameron WD. Terminology for model credibility, technical notes and comments. Simulation. 1980;35(6):206–207.
- Zeigler BP, Muzy A, Kofman E. Chapter 1 - Introduction to systems modeling concepts. In: Zeigler BP, Muzy A, Kofman E, editor. Theory of modeling and simulation: discrete event and iterative system computational foundations. 3rd ed. London: Academic Press; 2019. p. 3–25.
- Kortüm W. Introduction to system dynamics of ground vehicles. Veh Syst Dyn. 1987;16(S1):1–36.
- Jaschinski A. Application of MEDYNA in vehicle technology. Veh Syst Dyn. 1987;16(S1):189–214.
- Arnold M, Carrarini A, Heckman A, et al. Simulation techniques for multidisciplinary problems in vehicle system dynamics. In: M Valášek, editor. Computational mechanics in vehicle system dynamics, supplement to vehicle system dynamics, Vol. 40. London: Taylor & Francis; 2004. p. 17–36.
- Kortüm W, Schiehlen W, Hoffmann M. Progress in integrated system analysis and design software for controlled vehicles. Veh Syst Dyn. 1994;23(S1):274–296.
- Kortüm W, Vaculín O. Is multibody simulation software suitable for mechatronic systems? Proceedings of the 5th World Congress on Computational Mechanics, Vienna, Austria; 2012 Jul 7–12.
- Arnold M, Burgermeister B, Führer C, et al. Numerical methods in vehicle system dynamics: state of the art and current developments. Veh Syst Dyn. 2011;49(7):1159–1207.
- Spiryagin M, Cole C, Sun YQ, et al. Design and simulation of rail vehicles, ground vehicle engineering series. Boca Raton (FL): CRC Press; 2014.
- Belanger J, Venne P, Paquin JN. The what, where and why of real-time simulation, OPAL-RT Technologies, October, 2010, p. 37–49. [cited 2022 June 29]. Available from: https://blob.opal-rt.com/medias/L00161_0436.pdf.
- MODELISAR. Functional mock-up interface for model exchange and co-simulation. Document version 2.0 Beta 4; 2012 Aug 10.
- Gausemeier J, Moehringer S. New guideline VDI 2206 – a flexible procedure model for the design of mechatronic systems. In: A Folkeson, K Gralen, M Norell, etal, editors. Proceedings of 14th International Conference on Engineering Design (ICED 03), Aug 19–21. Stockholm: The Design Society; 2003. p. 599–608.
- Verein Deutscher Ingenieure. VDI 2206 - entwicklungsmethodik für mechatronische systeme [Design methodology for mechatronic systems]. Berlin: Beuth Verlag; 2004.
- Liu-Henke X, Lückel J, Jäker K-P. Development of an active suspension/tilt system for a mechatronic railway carriage. IFAC Conference on Mechatronic Systems, Darmstadt, Germany, 18-20 September 2000. IFAC Proc. 2000;33(26):283–288.
- Spiryagin M, Bruni S, Bosomworth C, et al. Rail vehicle mechatronics. Boca Raton (FL): CRC Press; 2022.
- Woolfson MM, Pert GJ. An introduction to computer simulation. Oxford: Oxford University Press; 1999.
- Raczynski S. Modeling and simulation: the computer science of illusion. West Sussex: Wiley; 2006.
- Chang CA. Simulation modelling: handbook: a practical approach. Boca Raton (FL): CRC Press; 2014.
- Gibson RR. Chapter 5 - Planning and plant layout. In: DA Snow, editor. Plant engineer's reference book. 2nd ed. Oxford: Butterworth-Heinemann; 2002. p. 5-1–5-18.
- Durán JM. What is a simulation model? Minds Mach. 2020;30:301–323.
- Boschert S, Rosen R. Digital twin—the simulation aspect. In: Hehenberger P, Bradley D, editor. Mechatronic futures. Cham: Springer; 2016. p. 59–73.
- Marte C, Six K. Optimising the railway system by virtualisation. Vol. 4. Virtual Vehicle Magazine. Special Edition: Digital Rail Solutions; 2019, p. 5–7.
- Minerva R, Lee GM, Crespi N. Digital twin in the IoT context: a survey on technical features, scenarios, and architectural models. Proc IEEE. 2020;108(10):1785–1824.
- Segovia M, Garcia-Alfaro J. Design, modeling and implementation of digital twins. Sensors. 2022;22(14):5396.
- Steindl G, Stagl M, Kasper L, et al. Generic digital twin architecture for industrial energy systems. Appl Sci. 2020;10(24):8903.
- Arena F, Collotta M, Luca L, et al. Predictive maintenance in the automotive sector: a literature review. Math Comput Appl. 2022;27(2):21.
- Shao G. Use case scenarios for digital twin implementation based on Iso 23247. Gaithersburg (MD): National Institute of Standards; 2021.
- Grieves M. Digital twin: manufacturing excellence through virtual factory replication. Whitepaper; 2014.
- Lee J, Bagheri B, Kao H-A. A cyber-physical systems architecture for industry 4.0-based manufacturing systems. Manuf Lett. 2015;3:18–23.
- Biesinger F, Weyrich M. The facets of digital twins in production and the automotive industry. 2019 23rd International Conference on Mechatronics Technology (ICMT); 2019 Oct 23–26, 6 p.
- Harper KE, Ganz C, Malakuti S. Digital twin architecture and standards. IIC J Innov. 2019;12:72–83.
- Bass L. Software architecture in practice. 4th ed. (Clements P, Kazman R, editors). Boston (MA): Addison-Wesley; 2021.
- Bauer T, Antonino PO, Kuhn T. Towards architecting digital twin-pervaded systems. 2019 IEEE/ACM 7th International Workshop on Software Engineering for Systems-of-Systems (SESoS) and 13th Workshop on Distributed Software Development, Software Ecosystems and Systems-of-Systems (WDES); 2019 May 28.
- Caporuscio M, Edrisi F, Hallberg M, et al. Architectural concerns for digital twin of the organization 2020. Cham: Springer International Publishing; 2020.
- International Standard ISO 23247-2. Automation systems and integration — digital twin framework for manufacturing — Part 2: reference architecture; 2021.
- Lei Z, Zhou H, Hu W, et al. Toward a Web-based digital twin thermal power plant. IEEE Trans Ind Inf. 2022;18(3):1716–1725.
- Hu S, Wang S, Su N, et al. Digital twin based reference architecture for petrochemical monitoring and fault diagnosis. Oil Gas Sci Technol. 2021;76:9.
- Lim KYH, Zheng P, Chen C-H, et al. A digital twin-enhanced system for engineering product family design and optimization. J Manuf Syst. 2020;57:82–93.
- Alam KM, El Saddik A. C2PS: a digital twin architecture reference model for the cloud-based cyber-physical systems. IEEE Access. 2017;5:2062.
- Josifovska K, Yigitbas E, Engels G. Reference framework for digital twins within cyber-physical systems. 2019 IEEE/ACM 5th International Workshop on Software Engineering for Smart Cyber-Physical Systems (SEsCPS); 2019 May 28.
- Wang Z, Gupta R, Han K, et al. Mobility digital twin: concept, architecture, case study, and future challenges. IEEE Internet Things J. 2022;1:17452–17467.
- Hankel M, Rexroth B. The reference architectural model industries 4.0 (RAMI 4.0). Zvei, Frankfurt, Germany; 2015.
- Jacoby M, Usländer T. Digital twin and internet of things—current standards landscape. Appl Sci. 2020;10(18):6519.
- Contreras-Castillo J, Zeadally S, Guerrero-Ibañez JA. Internet of vehicles: architecture, protocols, and security. IEEE Internet Things J. 2018;5(5):3701–3709.
- Wang FY, Zheng NN, Cao D, et al. Parallel driving in CPSS: a unified approach for transport automation and vehicle intelligence. IEEE/CAA JAutom Sin. 2017;4(4):577–587.
- Tao F, Zhang M, Nee AYC. Chapter 3 - Five-dimension digital twin modeling and its key technologies. In: Tao F, Zhang M, Nee AYC, editors. Digital twin driven smart manufacturing. London, UK: Academic Press; 2019. p. 63–81.
- James SS, Anderson SR, Lio MD. Longitudinal vehicle dynamics: a comparison of physical and data-driven models under large-scale real-world driving conditions. IEEE Access. 2020;8:73714–73729.
- Maulik S, Riordan D, Walsh J. Dynamic reduction-based virtual models for digital twins - a comparative study. Appl Sci. 2022;12:12147154.
- Viehweger M, Vaseur C, van Aalst S, et al. Vehicle state and tyre force estimation: demonstrations and guidelines. Veh Syst Dyn. 2021;59(5):675–702.
- Tsunashima H, Hirose R. Condition monitoring of railway track from car-body vibration using time-frequency analysis. Veh Syst Dyn. 2022;60(4):1170–1187.
- Makke O, Gusikhin O. Robust IoT based parking information system. communications in computer and information science 1475. Cham: Springer International Publishing; 2021, p. 227.
- Milošević MDG, Pålsson BA, Nissen A, et al. Demonstration of a digital twin framework for model-based operational condition monitoring of crossing panels. In: Orlova A, Cole D, editors. Advances in dynamics of vehicles on roads and tracks II. Cham: Springer International Publishing; 2022. p. 95–105.
- Barricelli BR, Casiraghi E, Fogli D. A survey on digital twin: definitions, characteristics, applications, and design implications. IEEE Access. 2019;7:167653–167671.
- Shao G, Helu M. Framework for a digital twin in manufacturing: scope and requirements. Manuf Lett. 2020;24:105–107.
- Ruba M, Nemes RO, Ciornei SM, et al. Digital twin real-time FPGA implementation for light electric vehicle propulsion system using EMR organization. 2019 IEEE Vehicle Power and Propulsion Conference, VPPC; 2019.
- Heber D, Groll M. Towards a digital twin: how the blockchain can foster E/E-traceability in consideration of model-based systems engineering. 21st International Conference on Engineering Design, ICED 2017; Vancouver, BC, Canada, 2017 Aug 21–25.
- Chen M, Mao S, Liu Y. Big data: a survey. Mobile Netw Appl. 2014;19(2):171–209.
- Rathore MM, Shah SA, Shukla D, et al. The role of AI, machine learning, and big data in digital twinning: a systematic literature review, challenges, and opportunities. IEEE Access. 2021;9:32030–32052.
- Prytz R. Machine learning methods for vehicle predictive maintenance using off-board and on-board data [dissertation]. Halmstad University Press; 2014.
- Kiela K, Barzdenas V, Jurgo M, et al. Review of V2X–IoT standards and frameworks for ITS applications. Appl Sci. 2020;10(12):4314.
- Wang H, Liu T, Kim B, et al. Architectural design alternatives based on cloud/edge/fog computing for connected vehicles. IEEE Commun Surv Tutor. 2020;22(4):2349–2377.
- Wagg D, Worden K, Barthorpe R, et al. Digital twins: state-of-the-art future directions for modelling and simulation in engineering dynamics applications. ASCE-ASME J Risk Uncertain Eng Syst B: Mech Eng. 2020;6:030901.
- Worden K, Cross EJ, Barthorpe RJ, et al. On digital twins, mirrors, and virtualizations: frameworks for model verification and validation. ASCE-ASME J Risk Uncertain Eng Syst B: Mech Eng. 2020;6:030902.
- Kortüm W, Valasek M. The 4th IAVSD-Herbertov workshop: modelling and simulation of mechatronic vehicles: tools, standards and industry demands - objectives, issues and summary of results. Veh Syst Dyn. 1999;33(Suppl 1):191–201.
- Veitl A, Gordon T, Van De Sand A, et al. Methodologies for coupling simulation models and codes in mechatronic system analysis and design. Veh Syst Dyn. 1999;33(Suppl 1):231–243.
- Palensky P, Cvetkovic M, Gusain D, et al. Digital twins and their use in future power systems. Digital Twin Sep 1: paper 4; 2021. [cited 2022 August 4]. Available from: https://digitaltwin1.org/articles/1-4.
- Vaculín O, Krüger WR, Valášek M. Overview of coupling of multibody and control engineering tools. Veh Syst Dyn. 2004;41(5):415–429.
- Arnold M, Burgermeister B, Eichberger A. Linearly implicit time integration methods in real-time applications: DAEs and stiff ODEs. Multibody Sys Dyn. 2007;17:99–117.
- Khaled N, Pattel B, Siddiqui A. Digital twin development and deployment on the cloud. London: Academic Press, Elsevier; 2020.
- Polach O, Berg M, Iwnicki S. Chapter 17: simulation of railway vehicle dynamics. In: Iwnicki S, Spiryagin M, Cole C, McSweeney T, editor. Handbook of railway vehicle dynamics - 2nd ed. Boca Raton (FL): CRC Taylor & Francis; 2020.
- Bhatti G, Mohan H, Singh RR. Towards the future of smart electric vehicles: digital twin technology. Renewable Sustainable Energy Rev. 2021;141:110801.
- Wang Z, Liao X, Zhao X, et al. A digital twin paradigm: vehicle-to-cloud based advanced driver assistance systems. 2020 IEEE 91st Vehicular Technology Conference (VTC2020-Spring), 2020, p. 1–6.
- Rassõlkin A, Vaimann T, Kallaste A, et al. Digital twin for propulsion drive of autonomous electric vehicle. 2019 IEEE 60th International Scientific Conference on Power and Electrical Engineering of Riga Technical University (RTUCON); 2019, p. 1–4.
- Schluse M, Rossmann J. From simulation to experimentable digital twins: simulation-based development and operation of complex technical systems. 2016 IEEE International Symposium on Systems Engineering (ISSE); 2016, p. 1–6.
- Jones D, Snider C, Nassehi A, et al. Characterising the digital twin: a systematic literature review. CIRP J Manuf Sci Technol. 2020;29:36–52.
- Al-Rubaye AS, Tsourdos A, Avdelidis NP. Digital twin analysis to promote safety and security in autonomous vehicles. IEEE Commun Mag. 2021;5(1):40–46.
- Lampropoulos G, Siakas K. Enhancing and securing cyber-physical systems and industry 4.0 through digital twins: a critical review. J Softw Eval Process. 2022;2494:1–12.
- IPC-2551. International standard for digital twins; 2020, p. 1–70.
- Flamigni F, Pileggi P, Barrowclough O, et al. First report on standards relevant for digital twins. Change2Twin project: Deliverable D1.2. Version 1.0. Horizon 2020, EU; 16-03-2021, p. 1–75.
- Moya B, Badías A, Alfaro I, et al. Digital twins that learn and correct themselves. Int J Numer Methods Eng. 2022;123:3034–3044.
- Kapteyn M, Knezevic D, Huynh D, et al. Data-driven physics-based digital twins via a library of component-based reduced-order models. Int J Numer Methods Eng. 2022;123:2986–3003.
- Funfschilling C, Perrin G. Uncertainty quantification in vehicle dynamics. Veh Syst Dyn. 2019;57(7):1062–1086.
- Kitanoski F. Digital solutions for railway systems. Vol. 4. Virtual Vehicle Magazine. Special Edition: Digital Rail Solutions; 2019, p. 3–4.
- Shevchenko DV. Methodology for constructing digital twins in railway transport. VNIIZHT Sci J. 2021;80(2):91–99. (in Russian). DOI:10.21780/2223-9731-2021-80-2-91-99.
- Kritzinger W, Karner M, Traar G, et al. Digital twin in manufacturing: a categorical literature review and classification. IFAC-PapersOnLine. 2018;51(11):1016–1022.
- Dirnfeld R. Digital twins in railways: state of the art, opportunities, and guidelines. VT2022. Bachelor Degree Project, Linnaeus University, Faculty of Technology, Department of Computer Science and Media Technology; 2022. Available from: http://www.diva-portal.org/smash/get/diva2:1674046/FULLTEXT01.pdf.
- Pappaterra MJ. A review of literature and public datasets for the application of artificial intelligence in the railway industry [master’s thesis]. Department of Information Technology, Uppsala Universitet; 2022. Available from: https://www.diva-portal.org/smash/get/diva2:1647149/FULLTEXT01.pdf.
- Cole C, Spiryagin M, Wu Q, et al. Modelling, simulation and applications of longitudinal train dynamics. Veh Syst Dyn. 2017;55:1498–1571.
- Spiryagin M, Wolfs P, Cole C, et al. Design and simulation of heavy haul locomotives and trains. Ground vehicle engineering series. Boca Raton (FL): CRC Press; 2017.
- Spiryagin M, Persson I, Wu Q, et al. A co-simulation approach for heavy haul long distance locomotive-track simulation studies. Veh Syst Dyn. 2019;57(9):1363–1380.
- Wu Q, Spiryagin M, Cole C, et al. Parallel computing in railway research. Int J Rail Transp. 2020;8(2):111–134.
- Wu Q, Spiryagin M, Sun Y, et al. Parallel co-simulation of locomotive wheel wear and rolling contact fatigue in a heavy haul train operational environment. Proc Inst Mech Eng Part F J Rail Rapid Transit. 2021;235(2):166–178.
- Spiryagin M, Wu Q, Bosomworth C, et al. Understanding the impact of high traction hybrid locomotive designs on heavy haul train performance. Proceedings of AusRAIL PLUS 2019, delivering growth; creating opportunity; embracing technology, 3-5 Dec 2019, Sydney, NSW, Australia, p. 1–12.
- Uzuka T. Preface. RTRI simulation technologies. Railway Technical research Institute. Ascent 10 (March); 2022, p. 2–5. Available from: https://bunken.rtri.or.jp/doc/fileDown.jsp?RairacID= 0048000073.
- Parbst B, Dolmans E, Rasmussen TF. Digital twin and railway system safety. Project Group 7, Report. Safety by Design, University of Twente; February 2022, p. 1–20. Available from: https://safety.productions/wp-content/uploads/2020/02/Group_07.pdf.
- Sun YQ, Nielsen D, Wu Q, et al. Design and safety analysis of a 11-waggon consist for transporting rails. Aust J Mech Eng. 2022: 1–15. DOI: 10.1080/14484846.2021.2022576
- Nia SH, Stichel S. Multibody simulation as virtual twin to predict the wheel life for iron-ore locomotive wheels. Proceedings of International Heavy Haul Association Conference, IHHA 2019, Narvik, Norway, 2019 Jun 12–14.
- Stichel S, Nia SH, Krishna V, et al. Wheel and rail maintenance planning with support of digital twins. Presentation at William Hay Railroad Engineering Seminar, Railtec, University of Illinois at Urbana-Champaign, 2021 Spring Semester, 16 Apr 2021. Available from: https://railtec.illinois.edu/wp/wp-content/uploads/Stichel_Sebastian_Hay_Seminar_2021_April.pdf.
- Krishna VV, Wu Q, Nia SH, et al. Long freight trains & long-term rail surface damage – a systems perspective. Veh Syst Dyn. 2022: 1–24. DOI: 10.1080/00423114.2022.2085584
- Krishna VV, Wu Q, Nia SH, et al. Long freight trains & long-term rail surface damage: towards digital twins that enable predictive maintenance of track. World Congress of Railway Research, WCRR 2022, Birmingham, UK, 2022 Jun 6–10, p. 1–6.
- Krishna VV. Long freight trains & long-term rail surface damage [doctoral thesis in Engineering Mechanics]. KTH, Stockholm, Sweden; 2022.
- Maglio MM. Influence of wheel thread damage on wheelset and track loading – field tests and numerical simulations. Thesis for the degree of Licentiate, Department of Mechanics and Maritime Sciences, Chalmers University of Technology, Gothenburg, Sweden; 2020.
- Maglio M, Pieringer A, Nielsen J, et al. Wheel–rail impact loads and axle bending stress simulated for generic distributions and shapes of discrete wheel tread damage. J Sound Vib. 2021;502: 116085.
- Bernal E, Spiryagin M, Vollebregt E, et al. Prediction of rail surface damage in locomotive traction operations using laboratory-field measured and calibrated data. Eng Fail Anal. 2022;135:106165. DOI: 10.1016/j.engfailanal.2022.106165.
- Zhang T, Du W, Zhang G, et al. PHM of rail vehicle based on digital twin global reliability and Prognostics and Health Management (PHM-Nanjing), 15–17 Oct 2021, Nanjing, China; 2021, p. 1–5. DOI: 10.1109/PHM-Nanjing52125.2021.9613068.
- Grunert G. Data and evaluation model for the description of the static–dynamic interface between trains and railway bridges. Eng Struct. 2022;262:114335.
- Pillai N, Shih JY, Roberts C. Sensor selection and placement for track switch condition monitoring through validated structural digital twin models of train–track interactions. Eng Proc. 2021;10:49.
- Bustos A, Rubio H, Soriano-Heras E, et al. Methodology for the integration of a high-speed train in maintenance 4.0. J Comput Design Eng. 2021;8(6):1605–1621.
- Spiryagin M, Szanto F, Oldknow K, et al. Advanced modelling and performance evaluation of hydrogen-powered heavy haul locomotive. Proceedings of the 2022 Joint Rail Conference, ASME. Virtual, Online, 2022 Apr 20–21, V001T05A001, p. 1–8.
- Spiryagin M, Persson I, Wu Q, et al. A co-simulation approach for heavy haul long distance locomotive-track simulation studies. Veh Syst Dyn. 2019;57(9):1363–1380.
- Meng Z, Tang T, Wei G, et al. Digital twin based comfort scenario modeling of ATO controlled train. J Phys Conf Ser. 2020;1654:012071.
- Chu W, Wuniri Q, Du X, et al. Cloud control system architectures, technologies and applications on intelligent and connected vehicles: a review. Chin J Mech Eng. 2021;34:139.
- Hu Z, Lou S, Xing Y, et al. Review and perspectives on driver digital twin and its enabling technologies for intelligent vehicles. IEEE Transactions on Intelligent Vehicles; 2022, 24p.
- Liu Y, Zhang K, Li Z. Application of digital twin and parallel system in automated driving testing. Proceedings 2021 IEEE 1st International Conference on Digital Twins and Parallel Intelligence, DTPI; 2021, 4p.
- Piromalis D, Kantaros A. Digital twins in the automotive industry: The road toward physical-digital convergence. Appl Syst Innov. 2022;5:65.
- Ezhilarasu CM, Skaf Z, Jennions IK. Understanding the role of a digital twin in Integrated Vehicle Health Management (IVHM). 2019 IEEE International Conference on Systems, Man and Cybernetics (SMC); 2019 Oct 6–9, 8p.
- Algin V, Ishin M, Paddubka S. Models and approaches in design and diagnostics of vehicles planetary transmissions. IOP Conf Ser: Mater Sci Eng. 2018;393:10.
- Hiwase SD, Jagtap P. Predictive maintenance of automotive component using digital twin model. SAE Technical Paper 2022-28-0075; 2022, 7p.
- Magargle R, Johnson L, Mandloi P, et al. A simulation-based digital twin for model-driven health monitoring and predictive maintenance of an automotive braking system. Proceedings of the 12th International Modelica Conference, Prague, Czech Republic; 2017 May 15–17, p. 35–46.
- Venkatesan S, Manickavasagam K, Tengenkai N, et al. Health monitoring and prognosis of electric vehicle motor using intelligent-digital twin. IET Electr Power Appl. 2019;13(9):1328–1335.
- Scurria L, Risaliti E, Buss D, et al. Executable digital twin - prevent the early failure of a truck anchorage using smart virtual sensors. SAE Technical Paper 2022-01-0767; 2022, 9p.
- Ghosh N, Garg A, Li W, et al. Engineering design of battery module for electric vehicles: comprehensive framework development based on density functional theory, topology optimization, machine learning, multidisciplinary design optimization, and digital twins. J Electrochem Energy Convers Storage. 2022;19:030902-1-19.
- Li B, Bei S. Estimation algorithm research for lithium battery SOC in electric vehicles based on adaptive unscented kalman filter. Neural Comput Appl. 2019;31:8171–8183.
- Merkle L, Pöthig M, Schmid F. Estimate e-golf battery state using diagnostic data and a digital twin. Batteries. 2021;7(15):22.
- Ersal T, Kolmanovsky I, Masoud N, et al. Connected and automated road vehicles: state of the art and future challenges. Veh Syst Dyn. 2020;58(5):672–704.
- Guo J, Bilal M, Qiu Y, et al. Survey on digital twins for internet of vehicles: fundamentals, challenges, and opportunities. Digital Communications and Networks; 2022, 17p.
- Peng H. MCity Abc Test. Whitepaper; 20222. [cited 2022 December]. Available: https://mcity.umich.edu/wpcontent/uploads/2019/01/mcity-whitepaper-ABC-test.pdf.
- Eichberger A, Liu H, Fellendorf M, et al. Advances in automated driving systems. Basel: MDPI - Multidisciplinary Digital Publishing Institute; 2022.
- Tihanyi V, Rövid A, Remeli V, et al. Towards cooperative perception services for ITS: digital twin in the automotive edge cloud. Energies. 2021;14:5930.
- Wang Z, Han K, Tiwari P. Digital twin-assisted cooperative driving at non-signalized intersections. IEEE Trans Intell Veh. 2022;7(2):198–209.
- Liao X, Wang Z, Zhao X, et al. Cooperative ramp merging design and field implementation: a digital twin approach based on vehicle-to-cloud communication. IEEE Trans Intell Transp Syst. 2021;23(5):4490–4500.
- Halin A, Verly JG, Van Droogenbroeck M. Survey and synthesis of state of the art in driver monitoring. Sensors. 2021;21(16):48.
- Hasenjäger M, Heckmann M, Wersing H. A survey of personalization for advanced driver assistance systems. IEEE Trans Intell Veh. 2020;5(2):335–344.
- Best MC. Real-time characterisation of driver steering behaviour. Veh Syst Dyn. 2019;57(1):64–85.
- Chen X, Kang E, Shiraishi S, et al. Digital behavioral twins for safe connected cars. Proceedings of the 21th ACM/IEEE International Conference on Model Driven Engineering Languages and Systems; Copenhagen, Denmark: Association for Computing Machinery; 2018, p. 144–153.
- Stellet JE, Zofka MR, Schumacher J, et al. Testing of advanced driver assistance towards automated driving: a survey and taxonomy on existing approaches and open questions. 2015 IEEE 18th International Conference on Intelligent Transportation Systems; 2015 Sept 15–18, 8p.
- Batsch F, Kanarachos S, Cheah M, et al. A taxonomy of validation strategies to ensure the safe operation of highly automated vehicles. J Intell Transp Syst. 2022;26(1):14–33.
- Hallerbach S, Xia Y, Eberle U, et al. Simulation-based identification of critical scenarios for cooperative and automated vehicles. SAE Int J Connect Autom Veh. 2018;1(2):93–106.
- Brunner P, Denk F, Huber W, et al. Virtual safety performance assessment for automated driving in complex urban traffic scenarios. IEEE Intelligent Transportation Systems Conference (ITSC); October 27–30, 2019, 7p.
- Shadrin SS, Makarova DA, Ivanov AM, et al. Safety assessment of highly automated vehicles using digital twin technology. 2021 Intelligent Technologies and Electronic Devices in Vehicle and Road Transport Complex, TIRVED 2021 - Conference Proceedings; 2021, 5p.
- Xue D, Cheng J, Zhao X, et al. A vehicle-in-the-loop simulation test based digital-twin for intelligent vehicles. 2021 IEEE DASC/PiCom/CBDCom/CyberSciTech Congress; 2021 October 25–28, 5p.
- Yang L, Wang R, Zhao X, et al. CAVTest: a closed connected and automated vehicles test field of chang’an university in China. SAE Int J Connect Autom Veh. 2021;4(4):423–435.
- Szalay Z. Next generation X-in-the-loop validation methodology for automated vehicle systems. IEEE Access. 2021;9:35616–35632.
- Zhou J, Schmied R, Sandalek A, et al. A framework for virtual testing of ADAS. SAE International J Passeng Cars Electron Electr Syst. 2016;9(1):66–73.
- Templeton B. Tesla's “shadow” testing offers a useful advantage on the biggest problem in robocars; 2019. [cited 2022 December]. Available from: https://www.forbes.com/sites/bradtempleton/2019/04/29/teslas-shadow-testing-offers-a-useful-advantage-on-the-biggest-problem-in-robocars/.
- Zofka MR, Kohlhaas R, Schamm T, et al. Semivirtual simulations for the evaluation of vision-based ADAS. 2014 IEEE Intelligent Vehicles Symposium Proceedings; 2014 June 8–11, 6p.
- Demiyanushko I. Modern methods of the digital virtual analysis for design and tests of road vehicle restraint systems. IOP Conf Ser: Mater Sci Eng. 2021;1159:13.
- Liu R, Wang J, Zhang B. High definition map for automated driving: overview and analysis. J Navig. 2020;73(2):324–341.
- Zhang J, Letaief KB. Mobile edge intelligence and computing for the internet of vehicles. Proc IEEE. 2020;108(2):246–261.
- Marai O E, Taleb T, Song L. Roads infrastructure digital twin: a step toward smarter cities realization. IEEE Network. 2021;35(2):136–143.
- Kaliske M, Behnke R, Wollny I. Vision on a digital twin of the road-tire-vehicle system for future mobility. Tire Sci Technol. 2021;49(1):2–18.
- Pomoni M. Exploring smart tires as a tool to assist safe driving and monitor tire-road friction. Vehicles. 2022;4(3):744–765.
- Rasol M, Schmidt F, Ientile S, et al. Progress and monitoring opportunities of skid resistance in road transport: a critical review and road sensors. Remote Sens. 2021;13(18):26.
- Naets F, Geysen J, Desmet W. An approach for combined vertical vehicle model and road profile identification from heterogeneous fleet data. 2019 8th IEEE International Conference on Connected Vehicles and Expo, ICCVE 2019 – Proceedings; 2019, 5p.
- Fietkau P, Kistner B, Munier J. Virtual powertrain development. Proc Inst Mech Eng D: J Automobile Eng. 2020;234(14):3288–33301.
- Rahate SL, Gupta N, Umbarkar Y, et al. Robust approach for development and analysis of electric three wheeler vehicle using digital twin. SAE Technical Paper 2021-26-0323; 2021, 8p.
- Foldager FF, Thule C, Balling O, et al. Towards a digital twin - modelling an agricultural vehicle. Lecture notes in computer science. 12479. Cham: Springer International Publishing; 2021; p. 109–123.