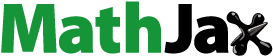
ABSTRACT
SME default prediction is a long-standing issue in the finance and management literature. Proper estimates of the SME risk of failure can support policymakers in implementing restructuring policies, rating agencies and credit analytics firms in assessing creditworthiness, public and private investors in allocating funds, entrepreneurs in accessing funds, and managers in developing effective strategies. Drawing on the extant management literature, we argue that introducing management- and employee-related variables into SME prediction models can improve their predictive power. To test our hypotheses, we use a unique sample of SMEs and propose a novel and more accurate predictor of SME default, the Omega Score, developed by the Least Absolute Shrinkage and Selection Operator (LASSO). Results were further confirmed through other machine-learning techniques. Beyond traditional financial ratios and payment behavior variables, our findings show that the incorporation of change in management, employee turnover, and mean employee tenure significantly improve the model’s predictive accuracy.
Video Abstract
© 2022 The Author(s). Published with license by Taylor & Francis Group, LLC.
Introduction
During the last two decades, finance and management literature have emphasized the necessity of improving SME default predictions, and scholars have observed that we still lack a universally accepted SME default model (Ciampi et al., Citation2021; Habib et al., Citation2020). Among the motives for developing effective SME default predictors, scholars have recognized great potential in reducing lending errors, supporting policymakers in restructuring policy implementation, credit analytics firms in assessing creditworthiness, and involving public and private investors in allocating funds, entrepreneurs in accessing funds, and managers in developing effective strategies (Altman et al., Citation2017). Thus, the implementation of efficient default predictors now lies at the heart of day-to-day banking and rating agencies’ decision-making processes on credit facilities and issuers’ creditworthiness. Furthermore, in recent times, governments have incentivized banks to conduct in-house monitoring of the creditworthiness of clients, many of which are SMEs (Altman & Sabato, Citation2007; Ciampi, Citation2015).
In light of the recognized salience behind effective default risk assessment, the International Financial Corporation (IFC),Footnote1 a member of the World Bank Group, is carrying out a global survey on SME rating agencies to make recommendations for optimal structuring of the rating industry for SMEs in emerging markets. The Inter-American Development Bank (IDB), with the cooperation of the Ibero-American Federation of Stock Exchanges (FIAB), and the assistance of one of this article’s authors and the firm Wiserfunding, Ltd,Footnote2 is also conducting a study on how capital markets can be structured for the specific purpose of providing alternative financing options, in addition to traditional bank loans, for Latin American SMEs. However, SME default predictors are typically less accurate than those regarding large corporations (Hernández-Linares et al., Citation2020).
The extant literature on SME default prediction has investigated how financial indicators and payment behavior variables exert significant influence on default likelihood (Altman & Sabato, Citation2007; Behr & Güttler, Citation2007; Ciampi & Gordini, Citation2012; Ciampi et al., Citation2021; Norden & Weber, Citation2010). Drawing on existing studies in the management literature, we contend that management- and employee-related variables can play an important role in determining accurate SME default prediction. Both these groups of variables, we believe, embed information that is not completely capturable by financial indicators and payment behavior variables. Management boards formulate the strategic direction and structure of a firm (Bermiss & Murmann, Citation2015), and their characteristics and structure exert significant influence on firms’ medium- and long-term viability (Coff, Citation1997). Similarly, employee retention, turnover, accumulated knowledge, and other employee-related characteristics, widely affect several organizational-level variables (Campbell et al., Citation2012a).
To test our hypotheses, we adopt a unique sample of 2,040 SMEs from Croatia. The results of our analysis derive from LASSO techniques and other machine-learning techniques. We examine 87 variables related to financial indicators, 6 variables related to payment behavior, 33 variables related to managers, 21 variables related to employees, and 17 variables that serve as control variables. Importantly, compared to traditional modeling, our study uses a different, early event to classify a SME as defaulted. A SME is classified as defaulted when the firm’s bank account is blocked for 30 or 60 days, since the SME (that is, the debtor) fails to pay debts to the creditors (that is, to the suppliers, banks, government). This “blocking of bank accounts” is an earlier distress-event than a legal bankruptcy or most defaults.
In line with prior studies, our analysis corroborates the importance of both financial figures and payment behavior variables. Also consistent with our hypotheses, the management- and employee-related variables further improve the SME default predictor. We call this new SME default predictor the “Omega Score”.Footnote3 Specifically, the Omega Score includes traditional financial ratios, payment behavior variables and also change in management, “firing ratio,” and mean employee tenure. Compared with existing predictors (for example, the Z''-Score), in a quantification exercise, we show that the Omega Score is capable of improving the correct classification of defaulted debts by 15.6 million euros in our sample, and this leads to a number of theoretical and practical implications which are discussed.
Theoretical background and research hypotheses
Financial indicators, payment behavior variables, and SME default risk
In 1968, Edward I. Altman implemented the first multivariate default formula, the so-called Z-Score, with the aim of predicting large corporation defaults based on a series of corporate income and balance sheet figures. Later, the formula was refined (Altman et al., Citation1977; Altman et al., Citation1995) and then also applied to non-listed firms (that is, the Z''-Score; among others; see Altman et al., Citation2017). Overall, the Z-Score and its early derivations were based on the idea that selected financial figures directly affect default predictions.
However, default prediction models solely relying on information gathered from financial statements lead to an incomplete picture about the firm prospects. Thus, a step forward was made in default prediction models by the integration of financial ratios with payment behavior variables. The importance of prior payment behavior variables highlights the fact that SME default is often a gradual process, with creditor promises being broken prior to the actual default (Altman et al., Citation2010; Norden & Weber, Citation2010; Stevenson & Pond, Citation2016). For example, Norden and Weber (Citation2010) observed that credit line usage and cash flows in a borrower’s checking accounts can provide banks with further information on default risks. In particular, the authors noted that incorporating information on account activity in default models substantially improved their predictions, and they argued that these improvements are especially useful for monitoring SMEs. Over the years, various scholars have recognized the importance of financial figures and payment behavior variables in predicting SME default (Altman & Sabato, Citation2007; Ciampi, Citation2015; Giannozzi et al., Citation2013). In line, the extant literature has shown that integrating payment behavior variables with financial information significantly increases default prediction accuracy compared with default models that adopt financial indicators alone (Back, Citation2005; Laitinen, Citation1999; Turetsky & McEwen, Citation2001; Wilson et al., Citation2000). When financial institutions obtain information about a firm’s recent payment behavior, they can base their solvency assessments not only on financial statement analysis (often available only after various months after the end of the financial year), but also on this more recent information. These arguments and empirical relationships hold for large corporations and they find empirical support as well in the study of SMEs (Ciampi & Gordini, Citation2012). Thus, based on prior literature, we advance the following hypothesis:
Hypothesis 1: The incorporation of payment behavior variables improves SME default risk models above those based solely on financial indicators.
Management-related variables provide additional information on SME default risk
Extant research has revealed how management boards can affect a firm’s performance and viability through their individual actions and collective behaviors (for example, Luo et al., Citation2014; Souder et al., Citation2012). Management boards are entailed to formulate the strategic direction and structure of a firm, convey organizational values to the other people inside the organization, and enhance the motivation of the employees. Moreover, their knowledge is highly centered on high-order routines that govern resource allocation and strategic alignment processes (Bermiss & Murmann, Citation2015; Gioia & Thomas, Citation1996). Thus, while the accumulated knowledge, experience, and capabilities of managers can lead firms to achieve and sustain a competitive advantage, the loss of these managers and the human capital embedded within them can seriously threaten a firm’s medium- and long-term viability (Coff, Citation1997). These assertions are corroborated by several studies showing how the management boards and human capital embedded within them are fundamental for determining a firm’s profitability (Hitt et al., Citation2001) and survival likelihood (Pennings et al., Citation1998). In line with this, departing managers take with them knowledge and capabilities that may barely be present elsewhere inside the organization, thereby opening up expertise gaps that need to be filled.
Another relevant research stream concerns how specific governance mechanisms (for example, board size and composition, CEO power and experience) influence the ability of firms to survive (Aguilera et al., Citation2008; Dowell et al., Citation2011). For example, Dowell et al. (Citation2011) found that the degree to which governance mechanisms affect a firm’s survival depends on its structural fragility and environmental turbulence. Accordingly, we argue that management-related variables exert strong influence on SMEs, as SMEs are typically more fragile and their limited size confers them with lower financial stability, especially in presence of environmental dynamism (Ferreira de Araújo Lima et al., Citation2020). Moreover, as García and Herrero (Citation2021) pointed out, the composition of the management board and its characteristics are relevant in affecting a firm’s capital structure and likelihood of default. For example, the authors found that gender diversity inside management boards is negatively related to the likelihood of bankruptcy. Similarly, Ciampi (Citation2015) found that corporate governance variables improve SME default prediction. Likewise, management boards with highly qualified and experienced managers enhance the effectiveness of decision-making processes and the organizational readiness to cope with environmental changes (Milliken & Martins, Citation1996). We also emphasize the relevant portions of nonoverlapping information among financial indicators, payment behavior, and management-related variables. In fact, quantitative data that can be extracted from financial indicators and payment behavior variables do not automatically embed information about the relationship between a firm and its management board. Thus, we extend previous studies that have recognized the importance of incorporating corporate governance variables with financial indicators only in developing effective default risk models (Cornée, Citation2017), and we hypothesize that the simultaneous incorporation of financial indicators, payment behavior variables and management-related variables in current default risk models could significantly improve the predictive power of those models. Finally, we consider that, in the context of SMEs, any changes in management boards can lead to sharp changes in the pursued strategic direction and organizational structure because the limited personnel size increases the relative importance of each individual, especially at the top of the organizational hierarchy. Following these lines drawn by past research, we hypothesize:
Hypothesis 2:
The incorporation of management-related variables (for example, management board characteristics, change in management, and management board composition) further improves the SME default risk models that are presently solely based on financial indicators and payment behavior variables.
Employee retention and turnover information improve SME default prediction
Difficulties in hiring and retaining qualified personnel are one of the most cited concerns among top managers interviewed by the Duke CFO Survey (Duke Fuqua School of Business, Citation2022). How to increase employee retention is also a key focal issue addressed by several consulting firms, with the organizations themselves indicating employee turnover as a considerable factor affecting their default risk (Securities and Exchange Commission [SEC], Citation2019). In the extant literature, the relationship between employees and the organizations they work for has been examined from several viewpoints. In detail, scholars have focused on how several factors, including employee mobility, employee turnover, mean employee tenure, and other characteristics, affect the performance and viability of organizations. For example, with respect to employee characteristics, Milliken and Martins (Citation1996) pointed out that diversity in the composition of employee groups affects organizational-level outcomes. In line with this, Zhang (Citation2020), drawing on institutional theory, contended that gender diversity can positively or negatively influence a firm’s performance, depending on the social context in which the firm is embedded.
Previous research has also shown that employee mobility can have positive consequences for a firm in the form of acquiring key employees from competitors (Gardner, Citation2005) and subsequent stimulation of knowledge transfer, innovation, and competitive advantage (Wezel et al., Citation2006). The departures of productive employees can be costly, especially when talent and proprietary knowledge move to rival firms. Accordingly, firms that experience a loss of key employees can suffer from direct and indirect effects associated with this loss. On the one hand, the focal firm can no longer benefit from the important role of these departing key employees. On the other hand, indirect effects connected to this loss might be the advantages obtained by the rival firm that hires the departees. The rival firms can leverage those employees’ experiences to replicate advantageous routines and processes within their firms and more effectively defend against the focal firm’s competitive actions (Aime et al., Citation2010). Not surprisingly, substantial employee turnover increases default risks for source firms, as they experience the loss of strategic human assets (Mawdsley & Somaya, Citation2015).
In a similar vein, scholars have analyzed how employee turnover affects firm performance and viability, as employee turnover is indeed a relevant cost for businesses. When numerous employees leave a focal firm, this might be a symptom of low capability of employee retention. Thus, high employee turnover is associated with negative performance outcomes of the focal firm (Hausknecht & Trevor, Citation2011). Campbell et al. (Citation2012b) observed that the presence of long-tenure employee profiles inside a firm suggests its capability to retain workers and capitalize on the invested value in human assets. Likewise, Li et al. (Citation2021) observed that employee turnover has detrimental effects on a firm’s profitability and growth. Interestingly, data corroborate that this negative association is particularly pronounced for small firms. In fact, in large corporations, knowledge is typically less concentrated among a small number of people, so that employee turnover is less risky for the large firm (Hancock et al., Citation2013; Li et al., Citation2021). Turnover is also associated with future financial performance uncertainty. Nevertheless, the negative effects are offset when turnover is low, confirming that a small turnover rate can be beneficial.
Despite the relevance of this employee-related information for assessing a firm’s risks and viability (particularly for SMEs), employee turnover information is often not incorporated into financial statements or otherwise disclosed by firms. This is related to different types of information with respect to financial indicators, payment behavior data, and management-related variables, but is still highly informative of their future performance (Li et al., Citation2021). Consistent with this idea, we advance our third hypothesis:
Hypothesis 3: The incorporation of employee-related variables (for example, employee retention, turnover, and composition) further improves the SME default risk models presently based solely on financial indicators and payment behavior variables.
Methodology
Sample and setting
We test the proposed hypotheses in the context of Croatia, a European Union member state, in the period 2015–2019. We believe that Croatia from 2015–2019 is a suitable setting to test our hypotheses about SMEs’ payment defaults for several reasons. With respect to the observation period, we limited the gathering of data to the end of 2019, as the period from 2015–2019 represented one in which economic conditions were stable in that country, thereby minimizing the possibility that our results have been compromised by the presence of exogenous shocks. For example, the spread of the COVID-19 pandemic led worldwide countries to provide massive policy support to minimize SME defaults. Dörr et al. (Citation2022) found about 25,000 firms that have not gone bankrupt despite serious financial difficulties. During the COVID-19 pandemic, in Croatia, the number of bankruptcies and account blockages dropped sharply, even though some firms decreased their revenues by 70–80%.
With respect to the context, Croatia is part of the world’s largest marketplace (the EU Single Market), and the World Bank classifies Croatia as a high-income European country.Footnote4 In Croatia, as in other European countries, the SMEs represent about 99% of all firms; the majority are independent, and employ about two-thirds of the workforce (Eurostat, Citation2018). The percentage of bankruptcy declarations in Croatia in the observation period is also similar to that of other European countries (Eurostat, Citation2022). The World Bank indicates that the value added from industryFootnote5 as a percentage of GDP in Croatia is 20%, which is similar to the percentage in developed countries. Financing constraints are prominent in developing countries, but SMEs are also adversely financially constrained in developed countries (Dvouletý et al., Citation2021). Similar enforcement laws for late payments to those in place in Croatia also exist in other European countries (European Commission, Citation2015, Citation2018; European Construction Sector Observatory [ECSO], Citation2020).Footnote6 A legal and economic analysis of late payments by the European Commission (Citation2015, Citation2018) has shown that three of four firms in Europe have experienced late payments in the last three years, with SMEs being disproportionately affected. Croatia has similar payment terms and actual payment durations to those of other European members. In particular, Croatia has a shorter payment duration than in Ireland, France, Italy, Spain, Portugal, or Greece, but longer than in the UK, Germany, or Bulgaria. Moreover, Croatia is very similar to the UK, Spain, France, or Belgium in the share of firms that exercise their right to compensation and/or interest in the event of late payment (European Commission, Citation2015, Citation2018).
We leverage six unique census datasets that include information on all SMEs in the period from 2015 to 2019. A firm with fewer than 250 employees is defined as an SME,Footnote7 and default is predicted for the following year (that is, t + 1).Footnote8 After constructing variables, about 53,000 non-defaulted SME-year observations were found in the analyzed period and 1,020 unique defaulted SMEs. Consistent with the existing literature (Barboza et al., Citation2017), we performed a random selection of the same per-year number of non-defaulted SMEs (1,020 SMEs) from the large pool of 53,000 non-defaulted cases. Thus, the final sample consists of 2,040 SMEs.Footnote9 Training and test samples are split, based on the reporting years, to simulate a robust “real-time default” prediction exercise. The training sample is composed of years 2015 and 2016, while the test sample is composed of years 2017 and 2018.
Measures
Dependent variables
In this study, we employ two dependent variables to capture an SME default event. The blockage date, either 30 or 60 days after a payment violation, was used to build our SME default indicators and to create a timely history of creditor payment defaults. An SME bank account gets blocked when it breaks promises to the creditors – in other words, when a debtor fails to repay debts to the creditors (for example, supplier, bank, government) on the due date. Specifically, we classify an SME as “defaulted” when its bank account is blocked for (i) 30 or (ii) 60 days since the SME failed to pay debts to its creditors. Thus, we construct two dummy variables that set the value as 1 if an SME failed to pay debts to its creditors within 30 and 60 days and 0 otherwise. These two definitions of SME default differentiate our study from the majority of the literature, which is focused on predicting the start of a bankruptcy procedureFootnote10 or the default on bank loans.Footnote11 Thus, our SME default definition includes defaults to bank loans, as well as default payments to suppliers and taxes, and the default is defined earlier than the Basel III criteria. The use of this default definition implies that the model is an earlier indicator of financial difficulties in bank–firm relationships and in commercial transactions and tax credits from the government perspective. This enables additional time for a rescue plan, for suppliers to readjust their strategies, or for banks to estimate earlier their risk exposures (see, Srhoj et al., Citation2022).
Independent variables
This study uses four groups of independent variables: financial indicators, payment behavior variables, management-related variables, and employee-related variables. With regard to the first group, this study adopts administrative data on SMEs’ financial information for the period 2014–2019 from FINA.Footnote12 In detail, we gathered 87 different variables related to figures from balance sheets, income statements, interest rate risk exposure, liquidity, and financial leverage (Altman et al., Citation2017; Giannozzi et al., Citation2013).
The second group of variables concerns payment behavior (that is, indicators of debtors being late in payments to creditors). In total, six variables were gathered, including the number of times a firm has a bank account blocked and the duration of the bank account blockage.
The third group of variables concerns management-related information (that is, information about the management and owners in the SME). In total, 33 variables were gathered in this group. For example, management board gender composition, manager age, management’s previous and current experience, or changes in management).
The fourth group of variables concerns employee-related information. In total, 21 variables were gathered in this group. For example, the mean employee tenure, the firing and hiring ratio (defined as the number of fired or hired employees in total number of employees), the share of work contracts (full and part time), or the share of higher educated employees. The full list of independent variables (divided by groups) and detailed measurement descriptions are reported in the Appendix.
Potential control variables
This study also uses controls for the eight following categories of variables (divided by groups): internationalization, innovation, relational capital/public contract and political connections, firm size, age, industry, region, and year. In total, we considered 17 variables as potential controls. We included a set of firm demographics, such as exports, export and import intensity, firm age, investments in R&D, intangible assets, sector, and region. In addition, we included variables related to the relational capital/public contract and political connections. Public funds can provide a cushion for SMEs to avoid default, which is why we include five variables capturing whether owner or CEO are politically connected or are from a family of politicians (Srhoj & Dragojević, Citation2022), whether the SME is a donator to a political party, and whether they have ongoing public procurement contracts. The full list of control variables and detailed measurement descriptions are reported in the Appendix.
Statistical method
In total, we included a list of 164 predictor variables. We selected the most important predictors by employing least absolute shrinkage and selection operator (LASSO), a method that performs regularization and variable selection (Tibshirani, Citation1996). LASSO is considered the state-of-the-art method for variable selection, and it was found to outperform stepwise logistic regression (Tong et al., Citation2016) or elastic net and adaptive LASSO (Fan et al., Citation2015). Zou and Hastie (Citation2005) suggest that an elastic net can outperform LASSO when the number of potential covariates is larger than the sample size. In our setting, the sample size was more than 10 times larger than the number of independent variables, and although some variables were correlated, the firm-level literature finds that the elastic net does not outperform LASSO in variable selection (Paraschiv et al., Citation2021; Tian et al., Citation2015) or in prediction of firm growth (Coad & Srhoj, Citation2020). We employ the logit LASSO, which selects only those variables with the highest predictive power of SME default.
The probability of SME default, given the independent covariates is estimated as follows:
where are independent variables for the ith firm, including the list of variables explained earlier. The regulation works by adding the penalty to the log-likelihood function:
Subject to .
In this equation, is a tuning parameter that controls the sparsity of the estimator. We follow the guidelines on working with LASSO, as developed by Coad and Srhoj (Citation2020). To run the logit LASSO, we used the function “rlassologit” (in R package “hdm”; Chernozhukov et al., Citation2016).
LASSO was fed gradually with different sets of variables. First, we used the full number of observations, but only with the financial variables, to capture ratios and growth in sales, assets, and so on. Other groups of variables were then added to assess whether these were important for improving prediction. For the selected variables, variable correlations were analyzed and, when the models selected highly correlated variables, those variables whose univariate AIC had a greater value were included (Table SM2). In addition, variance inflation factors (VIFs) were analyzed for all the independent variables in the logit model. The final variables selected with LASSO were used in two ways. First, a logit model was conducted, and prediction performance metrics were documented. Second, a discriminant analysis was conducted with the final set of selected variables to construct a new Omega Score variable. Discriminant analysis was done in R using the “MASS” package and its “lda” and “partimat” functions.
Based on observed SME defaults and predicted SME defaults, in the out-of-sample and out-of-time test sample, our study reports model performance metrics calculated in R with the “caret” package and function “confusionMatrix” (Kuhn et al., Citation2020). In the Supplementary Material (Tables SM3–5, SM7–8), each of the 19-performance metrics are reported. In the “model race,” we placed the strongest emphasis on comparing models based on the area under the curve (AUC), followed by the accuracy and the specificity. As suggested by Paraschiv et al. (Citation2021), we interpreted AUC ∈ [0.7, 0.8) as acceptable, AUC ∈ [0.8, 0.9) as excellent, and AUC ≥ 0.9 as outstanding.
We compare the models by also designing our initial benchmark model based on the seminal Z-Score obtained by the multidiscriminatory analysis (MDA; Altman, Citation1968). This analysis was originally used for corporate bankruptcy prediction but was later refined and applied also to non-listed firms (that is, Z''-Score, among others; see Altman et al. (Citation2017)). The latest version of Altman’s Z''-Score, with four financial ratio variables, was used to construct an SME default benchmark model. However, the Z''-Score model was recalibrated to obtain weights that better fit the analyzed data. This benchmark recalibrated model was then used to calculate the Z''-Score. The benchmark model has the SME default as a dependent variable and a single independent variable – the Z''-Score.
Finally, we used random forest, an ensemble method that is tree based, and XGBoost, also an ensemble method that boosts trees. Recent studies have documented the superiority of boosting and random forest models compared to logit and LASSO models for classification tasks (Alonso & Carbo, Citation2021). This improvement in prediction comes at a higher cost of interpretability; however, we applied random forest and XGBoost on the full set of variables, together with the newly constructed Omega Score, to identify any potential improvements in prediction performance. These machine-learning models were applied in R using packages “ranger” (Wright et al., Citation2020) and “XGBoost” (Chen et al., Citation2019).Footnote13
Results
The original Z''-Score had AUCs of 0.659 and 0.672 for 60 and 30 days, respectively. We recalibrated the Z''-Score model (benchmark model) and obtained better prediction performance; AUC of 0.70 (60 days) and 0.72 (30 days). Thus, conducting a recalibration gave a gain of Δ AUC = 0.041 and Δ AUC = 0.048 for the Z''-Score model. Using our list of 87 financial indicators, we applied LASSO to identify the most important financial indicators (see Table SM1).Footnote14 Our LASSO models outperformed the benchmark model in the out-of-sample and out-of-time test samples (, column 2). We repeated the LASSO procedure, but added the management- and employee-related variables to analyze whether information from these new groups of variables would further improve SME default predictions. The results in point to improvement of the SME default prediction once management and employee-related variables are added to the LASSO procedure (column 3).
Table 1. Prediction performance metrics.
Our Hypothesis 1 stated that the incorporation of payment behavior variables would further improve the SME default risk models presently based solely on financial indicators. To show support for our Hypothesis 1, we incorporated financial variables and the previous payment behavior variables in the LASSO procedure. (column 4) shows the improvement in the prediction performance compared to both the benchmark model and LASSO model with financial indicators. We showed whether incorporation of management- and employee-related variables further improved the SME default prediction models by adding these variables to data related to financial indicators and previous payment behavior variables. (column 5) shows that prediction is improved compared to the LASSO models when financial and previous payment behavior variables are included (column 4 vs 5).
shows the LASSO selected variables and their individual logit coefficients. Predictors that are negatively associated with SME default are surplus dummy, quick ratio, retained earnings over total assets, equity over total investments, and employee tenure. For example, having a positive surplus is associated with a decrease in the probability of SME default. Predictors that are positively associated with SME default are days of debtor and client change, personnel costs over value added, previous payment default, change in management and firing ratio. An increase in the number of previous payment defaults, a higher ratio of fires, or a change in management is associated with a higher probability of SME default (compare, ).Footnote15 These results provide further support for our hypotheses.
Table 2. LASSO selected variables and SME default prediction: logit model.
Based on the LASSO selected variables, we applied MDA to develop the new univariate Omega Score. In a methodological sense, our study goes “back” again, as it uses the method from Altman (Citation1968) but on the newly selected LASSO variables. The main reason for using the MDA is the increased interpretability and usability for analysts who do not have data for many firms or data science competencies. Thus, the benefit is an easy-to-use applicability of a formula, while the potential cost comes at somewhat lower prediction performance. Two versions of the Omega Score formula are proposed, one for the regular SME default (60 days) and one for the early-warning SME default prediction (30 days). More details on the Omega Score formula are provided in the discussion. Upon developing the Omega Score formula with MDA, and calculating the Omega Score for each SME in the test sample, k-Means clustering was applied to group Omega Score into three groups: (1) Alpha – indicating a healthy SME, (2) Beta – indicating an SME that is in-between, but surviving, and (3) Gamma – indicating an SME heading to default.
Jitter plots of Omega Score categories, Omega Scores, and SME defaults in the test sample are provided in Supplementary Material (Figures SM2 and SM2) and give visual indications of how Omega Score groups are acceptable or even excellent predictors of SME defaults. We also show bar plots of defaulted and non-defaulted SMEs across Omega Score groups, demonstrating that the Alpha group has the lowest probability of defaulting, while the Gamma group has the highest probability of default (Figure SM4). reports the numbers of outcomes (SME default) across Omega Score groups and the cumulative incidence of SME defaults. For example, the cumulative incidence of SME defaults within the Alpha group is 15.6%, while the cumulative incidence among the Gamma group is 92.2%. These percentages can be used to calculate risk ratios. For example, using the Alpha group as reference:
Table 3. Omega Score Groups and two SME default definitions: the test sample.
Categorization in the Beta or Gamma groups by Omega Score is associated with a 4.23 and 5.90 times higher risk of SME default compared to the SMEs in the Alpha group.
Next, we calculated the prediction performance metrics of the Omega Score and its groups, compared to the benchmark model. Comparing the benchmark model to two models, one model using categorical Omega Score groups (with Alpha, Beta, and Gamma levels) and the other model using only the Omega Score, shows substantial prediction improvement (compare, , Z'' Score, Omega Score Groups, Omega Score).
Table 4. Prediction performance metrics: Omega vs Z’’-Score.
A simple logit model with only Omega Score as an independent variable shows excellent AUC at 87.2.Footnote16 The Omega Score and Omega groups show similar prediction performance metrics. Comparing Omega Score to Z''-Score, a significant improvement is observed (Δ AUC = 0.168, Δ accuracy = 0.128, Δ sensitivity = 0.107, and Δ specificity = 0.156).
Both random forest and XGBoost improved the prediction performance metrics compared to the model using only the Omega Score (). The improvement was greater for the XGBoost than for the random forest. In particular, the Omega Score showed excellent performance (AUC = 87.2), while XGBoost model improved prediction to outstanding performance (AUC = 90.3). shows which variables are the most important in the XGBoost model. Not surprisingly, the Omega Score is by far the most important individual variable, followed by the duration of the previous payment default, the mean employee tenure, the quick ratio, and interest costs over turnover.Footnote17
Table 5. Prediction performance metrics: Z’’, Omega Score and machine learning models.
In sum, SME default prediction performance metrics were improved as financial indicators were enriched with payment behavior variables, as well as management and employee-related variables. These results corroborate our hypotheses. We incorporated these results into the Omega Score formula presented in the discussion.
Robustness checks
Our results show the management and employee-related variables selected by the LASSO. We tested the robustness of our findings by applying stepwise logistic regression. Results (in Table SM6) show the number of previous creditor payment defaults is selected again, as are the firing ratio and employee tenure. The change in management is selected for the 60 day model, and not in the 30 day model, as in LASSO. In addition, employee age is selected in the 30 day model, but not in the 60 day model. Stepwise-selected financial indicators share many similarities to LASSO, including surplus dummy, quick ratio, days of debtors’ and clients’ change, retained earnings over total assets, and personnel costs over value added. The only new financial indicator is the inventories change. In the Supplementary Material (Tables SM7–8), we show similar but slightly lower prediction performance metrics by stepwise logitsFootnote18 compared to LASSO. In general, both the LASSO and stepwise selected similar variables, and importantly, both methods indicate that firing ratio, employee tenure, and change in management have a significant role in determining SME defaults.Footnote19 As a robustness check, we also investigated whether United States Federal Reserve Board’s recommended variables used in the Comprehensive Capital Analysis and Review (CCAR) and Dodd–Frank Act Stress Tests (DFAST) contribute to the SME default prediction. The results in the Supplementary Material (Table SM9) show that macro variables do not significantly improve the model, and the relevance of other variables from the LASSO selected model remain stable compared to the previous model without macro variables. Finally, to increase the reliability of our results, we adopted alternative SME definitions (based on firm assets and turnover). Again, the results were consistent with previous analysis.
Discussion
This study highlights the importance of incorporating management- and employee-related indicators to extant models based on financial indicators and payment behavior variables for improving SME default predictions. In particular, by drawing on extant management literature, we build on the theoretical relevance of these two new groups of variables and unfold the ways in which they can further improve the predictive power of existing SME default models. Our hypotheses find empirical support, and we incorporate our findings in a new, more accurate, easy-to-use score, the Omega Score. The Omega Score takes the following general form:
Consistent with our analysis, the Omega Score specific formula for the regular 60-day version takes the following form:
Regular
Regular
Omega Score Groups = Alpha (Omega Score ≤ 0.007)
–Healthy SME
Beta (0.007 < Omega Score ≤ 1.626)
–Moderate–risk SME
Gamma (Omega Score ≥ 1.626)
–High–risk SME
Note that the Omega Score Groups reported represent the three alternative classifications of a focal SME, according to its estimated Omega Score. Conversely, the Omega Score specific formula for the early warning (30 days) takes the following form:
Early warning
Early warning
Omega Score Groups = Alpha (Omega Score ≤ 0.564)
–Healthy SME
Beta (0.564 < Omega Score ≤ 0.931)
–Moderate-risk SME
Gamma (Omega Score ≥ 0.931)
–High-risk SME
Next, we quantify the amount of debt in defaulted SMEs that have been correctly classified as defaulted with the Omega Score compared to the benchmark. To do so, we first identify defaulted SMEs and then we look at which defaulted SMEs were misclassified as non-defaulted by the Omega Score and the benchmark model. Second, among defaulted SMEs, we quantify the credit exposure. shows the details on the sum of three types of defaulted debts in period t (bank, suppliers, and government defaulted debtFootnote20).
Table 6. Quantifying the improved SME default prediction; monetary values (in million euros).
The total debt of defaulted SMEs in the out-of-sample and out-of-time test sample is 274 million euros. shows the benchmark model (that is, the Z''-Score) wrongly predicts defaulted SMEs, whose total debt is 82.5 million euros. In other words, defaulted SMEs with total debts of 191.5 million euros are correctly predicted by the benchmark model. The largest amount of defaulted debts are toward the banks, followed by suppliers, while the lowest amount of defaulted debt is toward the government (cf. ). The Omega Score improves the prediction of defaulted SMEs (Δ correctly predicting defaulted SMEs = 46 (172–126)). Compared to the Z''-Score, the Omega Score improves the correct classification of defaulted debt for the additional amount of 15.6 million euros (15.6/82.5 = 18.9% improvement).
The additional 15.6 million euros defaulted debts in the correctly identified SMEs could result in unrecovered loans to banks, suppliers, and the government, assuming they enter the bankruptcy procedure (Srhoj et al., Citation2022). Below, we discuss the main theoretical and practical implications yielded by this study.
Theoretical implications
The present study leads to three main theoretical implications. First, it shows that management- and employee-related variables entail a set of information that is not fully overlapping with financial indicators and payment behavior variables in the assessment of SME defaults. In fact, although the extant management literature corroborates their salience when assessing firm performance and viability (Souder et al., Citation2012), our knowledge of how those two groups of variables behave in presence of more investigated groups of variables (financial indicators and payment behavior variables) is limited (Ciampi, Citation2015). In this study, we show that management- and employee-related variables remain significant in determining SME defaults, even in the presence of much more intensively studied indicators coming from financial statements, creditworthiness analysis, and a large number of potential controls.
As Dowell et al. (Citation2011) pointed out, governance research would also have highly benefited from more studies linking governance variables to firm outcomes. In fact, boards have critical roles in formulating and carrying out strategic decisions that will largely affect the survival chances of firms. Thus, a second strength of this study is that it connects these dots, offering theoretical and empirical support in the intimate connection between management-related variables and survival chances, particularly by linking management-related variables to SME default predictions. Similarly, the Omega Score connects employee-related information to SME default risks. In general, we claim that this study, compared to extant literature, takes a step forward in highlighting the effective role of human capital for preventing (or accelerating) the failure of SMEs. These linkages between information coming from individual-level (management/employees) and firm-level domains bridge micro- and macro-management research domains (Aguinis et al., Citation2011).
Third, the present study sheds new light on the SME risk management literature (for reviews, see Ciampi et al., Citation2021; Ferreira de Araújo Lima et al., Citation2020) by pointing out novel insights regarding which factors most affect their default risk. The risk management literature aims at reducing and/or managing the potential risks that firms can cope with. These risks are often more pronounced for SMEs, as these firms are often more structurally fragile and less financially stable than large corporations. Thus, the development of a more accurate predictor of SME defaults directly contributes to the financial debate about SMEs coping with structural weaknesses in obtaining loans and managing their credit risks.
Practical implications
In the light of the introduced Omega Score and the quantification of its economic value, the present study has various practical implications for a number of business actors, such as creditors, entrepreneurs, and managers of SMEs, as well as potential investors, policymakers, and rating agencies who assess the SME default risks. For example, banks and rating agencies can benefit from this study by gaining some insights and added information for their internal rating models for SMEs. Among several nonfinancial variables tested in this study, our study highlights the most relevant ones that a bank could use in its ongoing credit monitoring.
We suggest modifying the banks’ internal rating models by increasing the role of qualitative variables related to the human capital of employees and management. Management- and employee-related information can increase the accuracy of traditional models based on financial indicators and payment behavior variables. In addition, the methodological contribution related to the mix of LASSO, MDA, and other machine-learning techniques given in the study could be considered by banks in the review process of their internal rating models. Similarly, small entrepreneurs can benefit from this study by using the Omega Score to estimate the default risk of new SME customers and to monitor the ongoing relationship with existing customers and key suppliers. An early identification of financial difficulties of customers allows the creditors to reduce the credit exposure to high-risk customers (or to limit commercial relationships) by reducing the payment terms or by asking for immediate payment on delivery. A credit-scoring procedure able to identify financial difficulties earlier can assist in preventing a contagion phenomenon in the supply chain, thereby generating positive effects for all stakeholders in the economy. Likewise, policymakers can benefit from this study since they can improve probabilistic estimates (for example, on a monthly, or quarterly basis) for the expected tax revenues collected from taxes on income and profits, social security contributions, taxes levied on goods and services, and payroll taxes.
In other words, according to the definition of SME default applied in this study, the Omega Score can easily be used to predict the default in tax credits of SMEs. The model can be used to screen the default risk of SMEs and, therefore, to set up procedures for preventing SME default and appropriate rescue plans. As existing studies show (Cultrera, Citation2020), acting at early stages of SME financial difficulties with preventive procedures can generate positive effects for all stakeholders in the firm and the economy, while increasing the probability for the SME to solve the crisis and remain active. The Omega Score and the suggested procedure for developing an SME default model can constitute a useful tool for early identification of SMEs with a high risk of facing serious financial difficulties.
Limitations and future research avenues
The present study is not free of limitations. One is that this is a single country study, and the observation period is limited to a period in which the economic conditions were stable. This can potentially limit the generalizability of our analysis. Thus, future researchers can move beyond these limitations and test at which extent the Omega Score is generalizable across different countries and time spans. A second limitation is that we do not use structural models since they require the firm’s current market value of assets, which is generally derived from equity market data through an option-based model and other assumptions (volatility of assets over the assessment period, default boundary, expected return on assets, and distribution of assets). Although several ways exist to estimate the market value of a SME (Rikkers & Thibeault, Citation2009), most of them require many assumptions for their application (that is, the average equity multiple of comparable firms, the present value of expected future dividends, and the discounted free cash flow approach). Accordingly, we invite future researchers to adopt structural models to benchmark the Omega Score. Third, in this study, we relied on random sampling. While we believe it could be a valid method to overcome the relatively low frequency of defaults as opposed to non-defaulted firms, future researchers can leverage broader samples of SMEs to create a rating system with several risk classes. In particular, future researchers can construct a transition matrix of credit ratings for the SMEs based on the considered variables and take into account both downgrades and defaults.
Conclusion
This study develops a new and more accurate SME default predictor, the Omega Score, by incorporating management- and employee-related variables into existing models, mostly based on financial indicators and payment behavior variables. We also introduce a timelier definition of SME default, a new combination of methods that helped us considerably improve prediction and provide a number of clear theoretical and practical implications for a variety of stakeholders. However, we are aware that this study gathered data from a single country. In addition, even if the Omega Score proves to be more accurate than existing predictors, we still acknowledge the presence of Type I and Type II errors. Building on this and previous work, we invite future researchers to overcome these limitations and develop increasingly more reliable SME default predictors, considering novel techniques and new groups of variables.
Supplemental Material
Download PDF (873.2 KB)Acknowledgments
A special thanks goes to Marko Rakar, Nikola Kleut and Melko Dragojević for research assistance with data structuring. Previous manuscript version was presented at the International Risk Management Conference in Cagliari from October 1 to October 3, 2021.
Disclosure statement
No potential conflict of interest was reported by the authors.
Supplementary material
Supplemental data for this article is available online at https://doi.org/10.1080/00472778.2022.2135718.
Correction Statement
This article has been corrected with minor changes. These changes do not impact the academic content of the article.
Additional information
Funding
Notes
1 The IFC with the private sector to create opportunities in developing countries to advance financial inclusion and to support job creation. The IFC has commissioned an independent Management Consulting Firm in South Africa, Credit Rating Analytics, to conduct an analysis of the SME credit rating industry within emerging markets.
2 Wiserfunding, Ltd is a prominent credit analytics firm based in London and Mumbai; it provides SME credit risk assessments for global financial institutions and for SMEs themselves.
3 We name our score “Omega” as the final letter of the Greek alphabet. “Omega” is often used to denote the ultimate limit of a set. In contrast to healthy, “Alpha,” firms, Omega firms are “the last” ones, thus more in financial difficulties.
4 World Bank, link (accessed April 29, 2022): https://datatopics.worldbank.org/world-development-indicators/the-world-by-income-and-region.html.
5 ibid.
6 For example, in Spain: Act on Late Payment in Commercial Transactions 69 (3/2004 Law of December 29, 2004); in Ireland: Prompt Payment of Account Act 1997; in France: Law on the Modernisation of the Economy.
7 When constructing financial ratios, SMEs with zero in the denominator had to be discarded. For this reason, SMEs with no employees, capital, assets, or financial debt are not included in the analysis. This also speaks to the nature of SME default analysis. SMEs can exit for nondistressed reasons, such as death of the owners and no children interested in running the business, but this is not of interest in the current analysis.
8 We decided to use a one-year prediction horizon since our default event is much earlier than the bankruptcy event and it represents the start of serious financial difficulties. One-year prediction is also standard in the banking industry and in the majority of the literature (see, for example, Barboza et al., Citation2017). As expected, using longer time horizons for predicting an early default, as in our study, decreases the prediction accuracy.
9 In the Supplementary Material (Table SM10), we show the NACE 1-digit industry distribution of firms, payment defaults, and our sample. Table SM10 shows that our sample is representative of both firm and payment default distributions.
10 To reinforce the idea that our default event is different from the traditional failure/bankruptcy procedure events, we track the access of SMEs to pre/bankruptcy procedures up to two years after their bank accounts are blocked. The percentages of firms that then accessed bankruptcy procedures is 33% (60-day default) and 30% (30-day default).
11 For example, a bank’s internal rating model, based on the Basel III definition of a default as any assets past due more than 90 days.
12 Financial Agency (FINA), link: http://www.fina.hr. Financial information from 2014 is used to construct independent variables (see Appendix).
13 Hyperparameters are tuned automatically.
14 Figure SM1 and Table SM2 show correlation checks and decisions on which variable to keep among the correlated pairs. All models are checked for the VIFs.
15 We run a logit model in which we add firing ratio, employee tenure, and change in management to the reweighted Altman Z’’-Score. The Supplementary Material (Tables SM11–12) shows that three nonfinancial variables are statistically significant and improve prediction performance metrics.
16 This AUC is higher than the LASSO model using financial indicators and management- and employee-related variables (AUC = 82.6) and higher than the LASSO model using financial indicators and creditor-related variables (AUC = 86.0), but a bit lower than a full LASSO model using financial indicators, past-payment behavior, and management- and employee-related variables (AUC = 88.0). We also plot the receiver operating curve with area under the curve for reweighted Altman Z’’-Score and Omega Score in the Supplementary Material Figure SM6.
17 In the Supplementary Material (Figure SM5) we provide a tree example from the boosted tree model with XGBoost for regular SME default definition. In this tree example, the Omega Score is used to make the first split depending on whether its value is below and above 0.548. The high value of ”Gain” for the Omega Score implies that the variable is very important (also shown in Figure SM5), the high value of ”Cover” implies the large number of observations related to the Omega Score. If Omega Score is below that value, second split is done based on whether mean tenure is below or above four years, while if the Omega Score is above the value, second split is done based on whether quick ratio is below or above 0.07. The ”value” in the leaf gives the contribution to the logit, where logit is the sum of the ”value” across all leaves, and as XGBoost is ensemble. The gradient boosted ensemble sums over the predictions of all trees.
18 The selected variables and prediction performance metrics are similar, regardless of whether we randomly shuffle the independent variables and whether we employ backward or forward stepwise selection, or a combination of the two selection procedures.
19 In their attempt to predict housing prices, Mullainathan and Spiess (Citation2017) show LASSO can sometimes select different covariates in different training sets. We therefore also report graphs of our principal component analysis (Figure SM7).
20 For this quantification exercise, we focus only on the regular SME default definition (60 days).
References
- Aguilera, R. V., Filatotchev, I., Gospel, H., & Jackson, G. (2008). An organisational approach to comparative corporate governance: Costs, contingencies, and complementarities. Organization Science, 19(3), 475–492. https://doi.org/10.1287/orsc.1070.0322
- Aguinis, H., Boyd, B., Pierce, C., & Short, J. (2011). Walking new avenues in management research methods and theories: Bridging micro and macro domains. Journal of Management, 37(2), 395–403. https://doi.org/10.1177/0149206310382456
- Aime, F., Johnson, S., Ridge, J. W., & Hill, A. D. (2010). The routine may be stable but the advantage is not: Competitive implications of key employee mobility. Strategic Management Journal, 31(1), 75–87. https://doi.org/10.1002/smj.809
- Alonso, A., & Carbo, J. M. (2021). Understanding the performance of machine learning models to predict credit default: A novel approach for supervisory evaluation. SSRN Electronic Journal. https://doi.org/10.2139/ssrn.3774075
- Altman, E. I. (1968). Financial ratios, discriminant analysis and the prediction of corporate bankruptcy. The Journal of Finance, 23(4), 589–609. https://doi.org/10.1111/j.1540-6261.1968.tb00843.x
- Altman, E. I., Iwanicz-Drozdowska, M., Laitinen, E. K., & Suvas, A. (2017). Financial distress prediction in an international context: A review and empirical analysis of Altman’s Z-Score model. Journal of International Financial Management and Accounting, 28(2), 131–171. https://doi.org/10.1111/jifm.12053
- Altman, E. I., Peck, M., & Hartzell, J. H. (1995). A scoring system for emerging market corporate bonds. Salomon Brothers.
- Altman, E. I., & Sabato, G. (2007). Modeling credit risk for SMEs: Evidence from the US market. Abacus, 43(2), 332–357. https://doi.org/10.1111/j.1467-6281.2007.00234.x
- Altman, E. I., Sabato, G., & Wilson, N. (2010). The value of non-financial information in SME risk management. The Journal of Credit Risk, 6(2), 1–33. https://doi.org/10.21314/JCR.2010.110
- Altman, E. I., Haldeman, R. G., & Narayanan, P. (1977). ZETATM analysis A new model to identify bankruptcy risk of corporations. Journal of Banking & Finance, 1(1), 29–54. https://doi.org/10.1016/0378-4266(77)90017-6
- Back, P. (2005). Explaining financial difficulties based on previous payment behavior, management background variables and financial ratios. European Accounting Review, 14(4), 839–868. https://doi.org/10.1080/09638180500141339
- Barboza, F., Kimura, H., & Altman, E. I. (2017). Machine learning models and bankruptcy prediction. Expert Systems with Applications, 83, 405–417. https://doi.org/10.1016/j.eswa.2017.04.006
- Behr, P., & Güttler, A. (2007). Credit risk assessment and relationship lending: An empirical analysis of German small and medium‐sized enterprises. Journal of Small Business Management, 45(2), 194–213. https://doi.org/10.1111/j.1540-627X.2007.00209.x
- Bermiss, Y. S., & Murmann, J. P. (2015). Who matters more? The impact of functional background and top executive mobility on firm survival. Strategic Management Journal, 36(11), 1697–1716. https://doi.org/10.1002/smj.2320
- Campbell, B. A., Coff, R., & Kryscynski, D. (2012b). Rethinking sustained competitive advantage from human capital. Academy of Management Review, 37(3), 376–395. https://doi.org/10.5465/amr.2010.0276
- Campbell, B. A., Ganco, M., Franco, A. M., & Agarwal, R. (2012a). Who leaves, where to, and why worry? Employee mobility, entrepreneurship and effects on source firm performance. Strategic Management Journal, 33(1), 65–87. https://doi.org/10.1002/smj.943
- Chen, T., He, T., Benesty, M., Khotilovich, V., Tang, Y., Cho, H., Chen, K., Mitchell, R., Cano, I., Zhou, T., Li, M., Xie, J., Lin, M., Geng, Y., & Li, Y. (2019). Package “xgboost.” In CRAN.
- Chernozhukov, V., Hansen, C., & Spindler, M. (2016). Hdm: High-dimensional metrics. The R Journal, 8(2), 185–199. https://doi.org/10.32614/RJ-2016-040
- Ciampi, F. (2015). Corporate governance characteristics and default prediction modeling for small enterprises. An empirical analysis of Italian firms. Journal of Business Research, 68(5), 1012–1025. https://doi.org/10.1016/j.jbusres.2014.10.003
- Ciampi, F., Giannozzi, A., Marzi, G., & Altman, E. I. (2021). Rethinking SME default prediction: A systematic literature review and future perspectives. Scientometrics, 126(1), 2141–2188. https://doi.org/10.1007/s11192-020-03856-0
- Ciampi, F., & Gordini, N. (2012). Small enterprise default prediction modeling through artificial neural networks: An empirical analysis of Italian small enterprises. Journal of Small Business Management, 51(1), 23–45. https://doi.org/10.1111/j.1540-627X.2012.00376.x
- Coad, A., & Srhoj, S. (2020). Catching gazelles with a lasso: Big data techniques for the prediction of high-growth firms. Small Business Economics, 55(3), 541–565. https://doi.org/10.1007/s11187-019-00203-3
- Coff, R. W. (1997). Human assets and management dilemmas: Coping with hazards on the road to resource-based theory. Academy of Management Review, 22(2), 374–402. https://doi.org/10.2307/259327
- Cornée, S. (2017). The relevance of soft information for predicting small business credit default: Evidence from a social bank. Journal of Small Business Management, 57(3), 699–719. https://doi.org/10.1111/jsbm.12318
- Cultrera, L. (2020). Evaluation of bankruptcy prevention tools: Evidences from COSME programme. Economics Bulletin, 40(2), 978–988.
- Dörr, J. O., Licht, G., & Murmann, S. (2022). Small firms and the COVID-19 insolvency gap. Small Business Economics, 58(2), 887–917. https://doi.org/10.1007/s11187-021-00514-4
- Dowell, G., Shackell, M. B., & Stuart, N. V. (2011). Boards, CEOs, and surviving a financial crisis: Evidence from the internet shakeout. Strategic Management Journal, 32(10), 1025–1045. https://doi.org/10.1002/smj.923
- Duke Fuqua School of Business. (2022). Duke CFO survey 2022. Retrieved May 24, 2022, from https://www.fuqua.duke.edu/duke-fuqua-insights/cfo-survey-q2-2022
- Dvouletý, O., Srhoj, S., & Pantea, S. (2021). Public SME grants and firm performance in European Union: A systematic review of empirical evidence. Small Business Economics, 57(1), 243–263. https://doi.org/10.1007/s11187-019-00306-x
- European Commission. (2015) . Ex-post Evaluation of Late Payment Directive Publications Office, Directorate-General for Internal Market, Industry, Entrepreneurship and SMEs, Lofstrom, F., Rivoire, L., Gallo, C., et al. https://data.europa.eu/doi/10.2873/016503
- European Commission. (2018). Business-to-business transactions: A comparative analysis of legal measures vs. soft law instruments for improving payment behaviour – Final Report https://doi.org/10.2873/500086
- European Construction Sector Observatory. (2020). Late payment in the construction sector. Analytical report. https://www.fiec.eu/application/files/6616/0259/5873/2020_Analytical_Report_Late_Payment_in_Construction.pdf
- Eurostat. (2018). Statistics on small and medium-sized enterprises. https://ec.europa.eu/eurostat/statistics-explained/index.php?title=Statistics_on_small_and_medium-sized_enterprises#Country_by_country_analysis
- Eurostat. (2022). Quarterly registrations of new businesses and declarations of bankruptcies – statistics. https://ec.europa.eu/eurostat/statistics-explained/index.php?title=Quarterly_registrations_of_new_businesses_and_declarations_of_bankruptcies_-_statistics
- Fan, L., Chen, S., Li, Q., & Zhu, Z. (2015). Variable selection and model prediction based on lasso, adaptive lasso and elastic net. In 2015 4th International Conference on Computer Science and Network Technology (ICCSNT) (pp. 579–583).
- Ferreira de Araújo Lima, P., Crema, M., & Verbano, C. (2020). Risk management in SMEs: A systematic literature review and future directions. European Management Journal, 38(1), 78–94. https://doi.org/10.1016/J.EMJ.2019.06.005
- García, C. J., & Herrero, B. (2021). Female directors, capital structure, and financial distress. Journal of Business Research, 136, 592–601. https://doi.org/10.1016/j.jbusres.2021.07.061
- Gardner, T. M. (2005). Interfirm competition for human resources: Evidence from the software industry. Academy of Management Journal, 48(2), 237–256. https://doi.org/10.5465/amj.2005.16928398
- Giannozzi, A., Roggi, O., Altman, E. I., & Sabato, G. (2013). Building SME rating. Is it necessary for lenders to monitoring financial statements of the borrowers? Bancaria-Sezione Forum, n. 10, ISSN 0005-4623.
- Gioia, D. A., & Thomas, J. B. (1996). Identity, image, and issue interpretation: Sensemaking during strategic change in academia. Administrative Science Quarterly, 41(3), 370–403. https://doi.org/10.2307/2393936
- Habib, A., Costa, M. D., Huang, H. J., Bhuiyan, M. B. U., & Sun, L. (2020). Determinants and consequences of financial distress: Review of the empirical literature. Accounting & Finance, 60(S1), 1023–1075. https://doi.org/10.1111/ACFI.12400
- Hancock, J., Allen, D. G., Bosco, F., McDaniel, K. R., & Pierce, C. A. (2013). Meta-analytic review of employee turnover as a predictor of firm performance. Journal of Management, 39(3), 573–603. https://doi.org/10.1177/0149206311424943
- Hausknecht, J. P., & Trevor, C. O. (2011). Collective turnover at the group, unit, and organizational levels: Evidence, issues, and implications. Journal of Management, 37(1), 352–388. https://doi.org/10.1177/0149206310383910
- Hernández-Linares, R., Kellermanns, F. W., & López-Fernández, M. C. (2020). Dynamic capabilities and SME performance: The moderating effect of market orientation. Journal of Small Business Management, 59(1), 162–195. https://doi.org/10.1111/jsbm.12474
- Hitt, M. A., Biermant, L., Shimizu, K., & Kochhar, R. (2001). Direct and moderating effects of human capital on strategy and performance in professional service firms: A resource-based perspective. Academy of Management Journal, 44(1), 13–28. https://doi.org/10.2307/3069334
- Kuhn, M., Wing, J., Weston, S., Williams, A., Keefer, C., Engelhardt, A., Cooper, T., Mayer, Z., Kenkel, B., Benesty, M., Lescarbeau, R., Ziem, A., Scrucca, L., Candan, C., Tang, Y., & Hunt, T. (2020). Package “caret. The R Journal. https://topepo.github.io/caret/
- Laitinen, E. K. (1999). Predicting a corporate credit analyst’s risk estimate by logistic and linear models. International Review of Financial Analysis, 8(2), 97–121. https://doi.org/10.1016/S1057-5219(99)00012-5
- Li, Q., Lourie, B., Nekrasov, A., & Shevlin, T. (2021). Employee turnover and firm performance: Large-sample archival evidence. Management Science. Ahead-of-print. https://doi.org/10.1287/mnsc.2021.4199
- Luo, X., Kanuri, V. K., & Andrews, M. (2014). How does CEO tenure matter? The mediating role of firm-employee and firm-customer relationships. Strategic Management Journal, 35(4), 492–511. https://doi.org/10.1002/smj.2112
- Mawdsley, J., & Somaya, D. (2015). Employee mobility and organizational outcomes: An integrative conceptual framework and research agenda. Journal of Management, 42(1), 85–113. https://doi.org/10.1177/0149206315616459
- Milliken, F. J., & Martins, L. (1996). Searching for common threads: Understanding the multiple effects of diversity in organizational groups. Academy of Management Review, 21(2), 402–433. https://doi.org/10.2307/258667
- Mullainathan, S., & Spiess, J. (2017). Machine learning: An applied econometric approach. Journal of Economic Perspectives, 31(2), 87–106. https://doi.org/10.1257/jep.31.2.87
- Norden, L., & Weber, M. (2010). Credit line usage, checking account activity, and default risk of bank borrowers. The Review of Financial Studies, 23(10), 3665–3699. https://doi.org/10.1093/rfs/hhq061
- Paraschiv, F., Schmid, M., & Wahlstrøm, R. R. (2021). Bankruptcy prediction of privately held SMEs using feature selection methods. SSRN Electronic Journal. https://doi.org/10.2139/ssrn.3911490
- Pennings, J. M., Lee, K. M., & van Witteloostuijn, A. (1998). Human capital, social capital, and firm dissolution. Academy of Management Journal, 41(4), 425–440. https://doi.org/10.2307/257082
- Rikkers, F., & Thibeault, A. E. (2009). A structural form default prediction model for SMEs, evidence from the Dutch market. Multinational Finance Journal, 13(3/4), 229–264. https://doi.org/10.17578/13-3/4-4
- Securities and Exchange Commission. (2019). Modernization of Regulation S-K Items 101, 103, and 105. Retrieved May 22, 2022, from https://www.sec.gov/rules/proposed/2019/33-10668.pdf
- Souder, D., Simsek, Z., & Johnson, S. G. (2012). The differing effects of agent and founder CEOs on the firm’s market expansion. Strategic Management Journal, 33(1), 23–41. https://doi.org/10.1002/smj.944
- Srhoj, S., & Dragojević, M. (2022). Public procurement and supplier job creation: Insights from auctions. The Journal of Law, Economics, and Organization.
- Srhoj, S., Kovač, D., Shapiro, J. N., & Filer, R. K. (2022). The impact of delay: Evidence from formal out-of-court restructuring. Journal of Corporate Finance, 102319. https://doi.org/10.1016/j.jcorpfin.2022.102319
- Stevenson, T., & Pond, K. (2016). SME lending decisions - the case of UK and German banks: An international comparison. Studies in Economics and Finance, 33(4), 501–508. https://doi.org/10.1108/SEF-12-2014-0243
- Tian, S., Yu, Y., & Guo, H. (2015). Variable selection and corporate bankruptcy forecasts. Journal of Banking and Finance, 52, 89–100. https://doi.org/10.1016/j.jbankfin.2014.12.003
- Tibshirani, R. (1996). Regression shrinkage and selection via the lasso. Journal of the Royal Statistical Society: Series B, 58(1), 267–288. https://doi.org/10.1111/J.2517-6161.1996.TB02080.X
- Tong, L., Erdmann, C., Daldalian, M., Li, J., & Esposito, T. (2016). Comparison of predictive modeling approaches for 30-day all-cause non-elective readmission risk. BMC Medical Research Methodology, 16(1), 26. https://doi.org/10.1186/s12874-016-0128-0
- Turetsky, H., & McEwen, R. (2001). An empirical investigation of firm longevity: A model of the ex ante predictors of financial distress. Review of Quantitative Finance and Accounting, 16(4), 323–343. https://doi.org/10.1023/A:1011291425075
- Wezel, F. C., Cattani, G., & Pennings, J. M. (2006). Competitive implications of interfirm mobility. Organization Science, 17(6), 691–709. https://doi.org/10.1287/orsc.1060.0219
- Wilson, N., Summers, B., & Hope, R. (2000). Using payment behaviour data for credit risk modelling. International Journal of the Economics of Business, 7(3), 333–346. https://doi.org/10.1080/13571510050197230
- Wright, M. N., Wager, S., & Probst, P. (2020). Package “ranger”. A fast implementation of random forests. CRAN Repository.
- Zhang, L. (2020). An institutional approach to gender diversity and firm performance. Organization Science, 31(2), 439–457. https://doi.org/10.1287/orsc.2019.1297
- Zou, H., & Hastie, T. (2005). Regularization and variable selection via the elastic net. Journal of the Royal Statistical Society: Series B, 67(2), 301–320. https://doi.org/10.1111/j.1467-9868.2005.00503.x