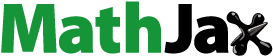
ABSTRACT
Startups rely on alternative forms of financing, such as crowdinvesting, as they usually miss reliable credit ratings and bank loans. We explore the role of geographical distance between investors and startups, and analyze the role of signals and the investor-startup-relationship in investment decisions. Using a unique proprietary dataset from the largest crowdinvesting platform in Mexico, we show that distance between investors and startups negatively affects the investment probability and investment amount. An investor-startup-relationship only diminishes this correlation in specific instances while observable signals do in any case. Evidence is in line with the idea that investors with a close relationship to a startup should be used as fallback options instead of exclusive investors in startups’ early development stage. Furthermore, it underscores that signals are of high importance in modern forms of financing.
The new source of power is not money in the hands of a few, but information in the hands of many.
– John Nasbitt, U.S. author.
Introduction
The rising use of the Internet puts online platforms more and more into the center of attention not only for online sales. The importance of online platforms in the financial sector also constantly increases (OECD, Citation2019). In crowdinvesting, also called equity crowdfunding, platforms enable investors to easily choose from one of multiple equity investments which are offered in a single place. Crowdinvesting platforms lead to a change in investment decisions of private investors as they do not only save time for investors but also increment their access to alternative investment opportunities (Konovalova et al., Citation2020). They ease the access to information in general as well as through features and details, for example, comments or answers on frequently asked questions, which can more easily be made available and disseminated online.
Many studies focus on investment decisions in established investment types, usually conducted without online platforms involved. This research strand consistently shows that the physical distance between investors and investees is negatively correlated with the investment probability and investment amount (Bae et al., Citation2008; Degryse & Ongena, Citation2005; Dejean, Citation2020). In crowdinvesting, most steps in the investment process are arranged online. This fact might suggest that the comparison and evaluation of investment possibilities have become easier in recent years, leading to a lower relevance of the physical distance between the investment parties. However, studies show that also in this comparably new form of financing, investors experience larger information asymmetries with greater physical distance. This informational disadvantage plays a major role in investors’ decision-making in crowdinvesting (Kleinert & Volkmann, Citation2019). A close relationship between investor and investee might decrease information asymmetries, and foster investors’ confidence in startup success so that the investment probability and investment amount increase (Angerer et al., Citation2017; Mollick, Citation2014). Besides information asymmetries reflected through physical distance as a driver of investors’ decision making, social distance might also be proxied by distance. For example, Agrawal et al. (Citation2008) conclude that social aspects are able to substitute the physical distance between investors and investees. In line with the so-called mere-exposure effect, investors might be biased toward physically closer startups since they are exposed more often to the startups or the area the startups are located in and therefore feel, for example, more connected to them. Moreover, objectively observable signals by other investors may decrease uncertainty in decision-making. In turn, these signals may positively influence investment decisions (Courtney et al., Citation2017).Footnote1
In this study, we investigate the link between geographical distance between investors and startups and the investment probability and investment amount in crowdinvesting. Using detailed data on investor and startup characteristics, we conduct logit regressions with 407,497 observations (investment and no investment observations) as well as OLS regressions with fixed effects with 11,054 observations (investment observations only) from the largest Mexican crowdinvesting platform. Thereby, we investigate if distance still plays a role in investment decisions. More specifically, we concentrate on the investment probability and investment amount. We also analyze the moderating effect of the investor-startup-relationship in this context. If any kind of relationship exists, on the one hand, informational disadvantages of investors should decrease as more information is directly transferred from the better informed party, that is, the startup, to the less informed one, that is, the investor. On the other hand, an existing relationship might lead to more trust in the startup through previous encounters and, thus, reduced uncertainty and a feeling of connectedness. Hence, we expect that relationships mitigate the negative effect of distance on the investment size. In the final part of this study, we evaluate the effect of observable signals on investment decisions of future investors in crowdinvesting. We assume that a high number of likes proves to be a signal of confidence by other investors who have already had good experience with the investee or are convinced of a startup’s success in the near future. In turn, we expect potential investors to be motivated to invest more, leading to a higher investment amount.
Our study helps deepening the understanding of existing links between physical distance, personal relationships, and signals in the field of entrepreneurial research. With the given dataset containing information on investors and startups, we provide insights into how rationally observable characteristics influence investment decisions in crowdinvesting, that is, the investment probability and investment amount.
First, we find that a greater distance is associated with a decrease in the investment probability and investment amount. A 10% increase in distance corresponds to a decrease in investment probability by 1.42 pp and the investment amount by 0.39%.
Second, we provide evidence that the investor-startup-relationship correlates with the investment probability as well as the investment amount. A given relationship shows a positive correlation with investment decisions. However, a relationship does not fully counteract the negative role of being farther away from the startup in any case. In addition, this study confirms the finding by, Angerer et al. (Citation2017), that family and friends are frequent investors in startups’ early development stage.
Last, we explore the role of likes, that is, an observable signal, on investment decisions. The investment probability rises with the number of likes received. However, we find a significantly negative correlation of likes on the investment amount, indicating that private investors prefer to hop on projects with an expected positive outcome (indicated by a high number of likes from other investors) with small amounts of money. As the outcome is still uncertain in crowdinvesting, this is in line with the idea of risk diversification. This result shows that investors consider other investors’ opinions in their decision-making process.
Our study relates and contributes to the literature in different ways. First, this study relates to the strand of literature on the effect of geographical distance between investors and startups in investment decisions (Chen et al., Citation2010; Coval & Moskowitz, Citation1999). Furthermore, we add to the literature on the effects of relationships and third-party signals on the link between distance and investment decisions. Second, our study relates to the general literature on crowdinvesting which is typically focused on the U.S. and Europe. Our study expands these results by providing new empirical evidence and insights into the development in Mexico. Mexico, with an expected yearly growth rate of 4.75% in the upcoming years (Statista, Citation2022b), constitutes one of the fastest growing markets for crowdinvesting—to compare, Germany expects a growth rate of 4.49% (Statista, Citation2022a) and the U.S. of 4.07% (Statista, Citation2022c). Third, we use a unique dataset including detailed information on crowdinvestment activities, investor, and startup characteristics. Thus, we provide new insights into investment decisions within this increasingly demanded modern way of financing. We also shed light on the role of startups’ public evaluation (that is, likes) in investment decisions. This topic has become more and more important as the digitalization proceeds with big steps. In turn, online activities in all areas, especially in financial transactions, become of higher focus and a more and more relevant option to consider in investors’ decision-making.
The paper proceeds as follows. We review the literature and develop our hypotheses, and describe the sample, methodology, descriptive statistics, and the research design. Then, we present and discuss our empirical results followed by robustness tests and a description of the limitations of this study. A summary of the main findings concludes.
Literature review and hypotheses development
Unsurprisingly, lacking financial resources restrict startup growth (Beck et al., Citation2006; Levine, Citation2005). In addition, an absent credit and firm history often impedes early stage startups from the access to traditional ways of financing (Carpenter & Petersen, Citation2002). In crowdinvesting, large information asymmetries exist between investors and investees (Kleinert & Volkmann, Citation2019). These information asymmetries between startups as the better and investors as the less informed party play a major role in investors’ decision-making. They stem from market imperfections and result in market inefficiencies (Akerlof, Citation1970; Rosser, Citation2003). Information asymmetries are especially relevant for borrowers in crowdfunding compared to the more traditional ways of financing (Piva & Rossi-Lamastra, Citation2018). Blum and Goldfarb (Citation2006) show that even in the case of purely digital goods sold and used via the Internet, that is, in the absence of trading and other opportunity costs, informational differences seem to play a role in investment decisions. The physical distance between investors’ and startups’ place of domicile may therefore increase information asymmetries between the two parties, for which the information hypothesis, assuming local investors to receive more and timelier information regarding local firms, could lead to more trust about the quality of a startup and lead to higher or more frequent investments in startups located nearby.
And indeed, several researchers find a preference for local investments compared to physically more distant ones (Bae et al., Citation2008; Malloy, Citation2005). Dejean (Citation2020, p. 337) underscores this finding with the explanation of “a regional attachment or a distant relationship with a particular community.” A broad literature confirms this idea in equity and startup investments. First, Coval and Moskowitz (Citation1999) find a preference for local equity investments in the U.S. When investigating the portfolio composition of stocks, investment managers show a strong bias toward local firms. Chen et al. (Citation2010) display the preference toward geographically closer investments in the venture capital market in the U.S. This effect persists even though nonlocal investments outperform local venture capital investments in terms of return. Lutz et al. (Citation2013) confirm this finding for the German venture capital environment. Besides currency and legal frameworks, Niemand et al. (Citation2018) also observe the preference for physically close investments in the equity crowdfunding context. However, they call for further research on this financing method on national level. Hornuf et al. (Citation2022) find a local bias for individual equity crowdfunding investments and investment portfolios. This effect appears to be largest for family, friends, and angel-like investors.
However, since the invested amounts are typically relatively small and therefore not much is at stake financially for investors, information asymmetries might not be solely the driver of this phenomenon in crowdfunding. Moreover, in crowdfunding, investors’ participation in the startup is low compared to, for example, venture capitalists. Thus, besides information asymmetries, the so-called mere-exposure effect (Zajonc, Citation1968) could be a further explanation for investors providing money close to their location. The mere-exposure effect is a form of cognitive bias stating that the repeated exposure to a stimulus (in this case, for example, the startup itself or the location the investors live in), changes the way people think and feel about the stimulus itself, even though the stimulus does not change (Bornstein & Craver-Lemley, Citation2022). Through these (multiple) exposures, investors might develop a feeling of liking of that stimuli (Stafford & Grimes, Citation2012) and familiarity to others (Kwan et al., Citation2015). Hence, geographical distance can also be a proxy for cultural or social preferences and, for example, connectedness, and can result in some kind of familiarity between investors, the area, and also the firms located in this geographical area, leading to a higher likelihood or a higher ambition to invest in startups nearby (Lindblom et al., Citation2018). Consequently, we expect:
Hypothesis 1a:
Higher geographical distance between investors and startups negatively correlates with investors’ decision to invest.
Hypothesis 1b:
Higher geographical distance between investors and startups negatively correlates with the investment amount.
In line with the mere exposure effect, Agrawal et al. (Citation2008, p. 259) state that “social proximity […] substitutes spatial proximity,” indicating that social proximity, next to geographical distance, does also play a role in investment decision making. According to the mere-exposure effect, repeated exposures to a stimulus strengthens the attitude regarding this stimulus. Through their repeated interaction, the attitude toward that startup can thus be enhanced (Bornstein & Craver-Lemley, Citation2022) and lead to a positive investment decision compared to a case where the startup is unknown to them. Hence, investors might feel drawn to startups to whom they were exposed in the past, or in other words, those they have already engaged with in the past. Accordingly, based on the mere exposure effect, any gap—be it social or informational—between investors and startups due to geographical distance could be alleviated by previous interactions with the startups.
Empirically, several researchers support the idea that previous interactions or close relationships are associated with a higher investment amount (for example, Angerer et al., Citation2017).Footnote2 Banerji and Reimer (Citation2019) show that the more connections a founder has, the higher the amount of money raised. They state “the social connectedness of founders [to be] the best predictor of funds raised” Banerji and Reimer (Citation2019, p. 46). Mollick (Citation2014) observes similar effects in his exploratory study. Measuring the effect of entrepreneurs’ social network by the number of friends online, he shows that larger personal networks are associated with a higher success probability of crowdfunding projects. Leyden et al. (Citation2014) and Vismara (Citation2016) underscore this idea. They explain the effect by arguing that social ties decrease uncertainty. As several studies have already revealed the positive effect of relationships on the investment probability, we exploit the advantage of this dataset of having detailed information on investors, investments, and startups, and focus on these links and respective interactions to the investment amount.Footnote3 Thus, we hypothesize:
Hypothesis 2:
An existing investor-startup-relationship positively mitigates the role of geographical distance between investors and startups on the investment amount.
Due to, on average, small individual investment amounts in crowdinvesting, the costs of evaluating a startup for crowdinvestors are disproportionately high compared to the amount invested. Following the underlying idea of Bikhchandani et al. (Citation1992) arguing that people use information from others, when this newly gained information outweighs one’s own information, the absence of easily available information in crowdinvesting could result in a fertile field for some kind of herd behavior. Herd behavior can be divided into two aspects. First, persons discount their own information and rely less on it compared to the observed behavior of others. This may indicate the assumption that predecessors are better informed or conducted high cognitive efforts to analyze, for example, the startup. Second, Bandura (Citation1977) already describes that people tend to imitate others meaning that investors engage in herd behavior simply because previous investors did.
For herd behavior to occur, the investors’ previous behavior must be observable for the potential investors. Since we are in an online scenario where no face-to-face communication or direct observation of others is possible, likes are a conceivable way to transfer information between investors. Li and Wu (Citation2013) state that the volume of likes can represent the aggregated approval of a product and can reduce quality uncertainty. Thus, likes act as a positive costless signal for investors’ peers. If new investors think that other investors, in aggregation, have more or even superior information or experiences about the startup represented by the number of likes received, likes as a signal could lead to herd behavior and result in more frequent or even higher investments in said startup. For example, Cornelis et al. (Citation2022) state that the cognitive perception influences potential investors’ funding intention. Moreover, it is not even necessary for a like to be accompanied by an investment, as Bikhchandani et al. (Citation1992) describe that also a similar behavior, in our case investing in the startup, can be triggered by the signal.
Empirically, this notion is confirmed. Signals are able to reduce uncertainty (Ahlers et al., Citation2015). In their empirical studies, Davila et al. (Citation2003) and Courtney et al. (Citation2017) confirm that signals from startups as well as from third parties increase startups’ possibilities for success. Nitani et al. (Citation2019) confirm signals on, for example, firm and owner attributes, to influence the success probability of crowdinvesting campaigns. Block et al. (Citation2018) confirm that signals which are easier to interpret show higher effects on the crowd. Several researchers observe that likes, describing observable “one-click cues” by former users, are a popular signal to use today (Ahlers et al., Citation2015). Likes are common in social media (Sumner et al., Citation2020) and indicate that individuals positively evaluate the content of a post, comment, or picture (Eranti & Lonkila, Citation2015). Thus, they communicate individuals’ “overall positive affect, or sentiment, toward the organization’s message” (Saxton & Waters, Citation2014, p. 287).
While one could argue that likes are only cheap talk and thus not relevant for investment decisions, in recent years, they have received increasing attention by the crowd and have shown higher importance in investors’ decision-making process with the rising use of the Internet (DiPietro et al., Citation2020; Kleinert & Volkmann, Citation2019). Nitani et al. (Citation2019) conclude that crowdfunding investors act rational and give strong weight to observable signals when taking investment decisions. This link is stronger for simple signals and nonexpert private investors (Monti et al., Citation2014). Carr et al. (Citation2018) reveal the importance of the quantity of likes on social media platforms, and Mochon et al. (Citation2017) display that online likes positively influence customer behavior. Thus, since likes are independent from the physical distance, they could mitigate the negative role of physical distance in the decision-making process and we hypothesize:
Hypothesis 3:
The received likes positively mitigate the role of geographical distance between investors and startups on the investment amount.
Data and research design
Data
The dataset for this study comprises data from the largest crowdinvesting platform in Mexico between 2015 and 2019. It includes information on startups and (potential) investors, as well as investors’ individual decision (not) to invest into a particular startup. In contrast to the databases of many former studies, our dataset contains information on the exact investment amount per investor per startup as well as private details, for example, investors’ and startups’ state of domicile. This information enables us to analyze factors influencing the concrete financial support provided while simultaneously looking at, for example, the relationship between startups and investors and other investment-specific characteristics.
Mexico as our country of data provenance represents an ideal environment for crowdinvesting research for several reasons. First, Mexico appears to be a country with very heterogeneous states in terms of the macroeconomic surrounding (see, OECD, Citation2022). Second, similar to blockchain technologies which might reduce problems of inefficient governance and corruption in Mexico (Zbinden & Kondova, Citation2019), crowdinvesting allows money flow between lenders (= investors) and borrowers (= startups). Thus, the financial support provided depends entirely on the online presentation of the startup as there is no previous bilateral communication between investors and startups.
Third, Mexico corresponds to other countries in terms of the development of crowdinvesting in the past couple of years. Since 2018, the transaction volume in Mexico has almost doubled, reaching USD 8.39 million in 2022. Experts of the business data platform “Statista” project the transaction volume in the Mexican crowdinvesting market to further grow by 4.75% per year in the short run (Statista, Citation2022b). This fact underscores the importance of our analyses.
Sample
The dataset includes observations from the platform’s internal management system for which investees are characterized as “startups.” We do not consider observations for which investees are registered as “SMEs,” “revenue-based funding” or “real estate funding” as these categories did not exist throughout the whole sample period. Furthermore, we focus on startups’ successful investment rounds, that is, when the minimum financing goal was reached within the investment period, as it ensures the explanatory power of our results with regard to the investment success. In addition, we do not have investor information from nonsuccessful investment rounds, and thus refrain from including these observations to allow a detailed analysis. We also limit the database to investors and startups located inside Mexico to avoid biased results due to a different macroeconomic environment which might drive our results. We focus on the first investment within each particular bilateral investor-startup-combination. This restriction rests upon the idea that investors learn from former investments in a respective startup (Hornuf & Neuenkirch, Citation2017). Furthermore, keeping these, in our dataset, 26 subsequent investments would not allow additional meaningful analyses due to the low number of observations, and would on top bias our coefficient of the investor-startup-relationship as relationships and knowledge flows in the entrepreneurial market evolve over time (Wadhwa & Kotha, Citation2006).
After these selection decisions, we create all possible investor-startup-combinations. This procedure results in our initial database with 618,120 investor-startup-combinations (= observations) from 6,120 investors and 101 startups from the years 2015 to 2019. We subsequently drop observations with illogical values in any of our variables of interest. Exemplarily, this involves observations with objectively wrong information on investors’ date of birth (for example, from investors characterized as born in the year 1887). This step decreases our sample by 5,454 observations (that is, 54 investors). Furthermore, we do not include observations for which information on dates does not logically fit our analyses. Exemplarily, this restriction relates to observations for which a startup was funded before an investor profile was created virtually, which logically prevents a possible investment. This excludes 205,169 investor-startup combinations from the sample. Overall, our final dataset for Hypothesis 1a in which we investigate the factors influencing the investment probability contains 407,497 observations. For the subsequent analyses of the investment amount (Hypothesis 1b, Hypothesis 2, and Hypothesis 3), we only include those observations where an investment has taken place. This results in a final sample of 11,054 individual investor-startup combinations.
displays the distribution of investors in Mexico. Investors appear widely spread throughout the country. Still, Mexico City (CMX), the capital city of Mexico, encompasses by far the highest number with approximately one third of all investors in the dataset. It is followed by the State of Mexico (MEX) with approximately half as many investors residing in this state (990).Footnote4 displays the distribution of startups throughout the Mexican states. We find a huge proportion of startups (61 of 101 startups) to be located in Mexico City. The other 50 startups in our dataset are distributed throughout the other states. All values are reported in of the Appendix.
Variables
Investment dummy
In Hypothesis 1a, we investigate the role of distance between investors and startups on the investment probability. To measure the investment probability in crowdinvesting, we employ a dummy variable as dependent variable for whether an investment has taken place ( = 1) or not ( = 0). An “investment” is characterized through the investor’s decision to financially support a startup via the crowdinvesting platform, that is, clicking “invest now,” deciding on the investment amount, and entering all personal information on the platform.
Investment amount
In Hypothesis 1b, Hypothesis 2, and Hypothesis 3, we investigate factors influencing the investment amount per investor per startup. The investment amount is measured in Mexican pesos, enabling us to immediately observe the real effects of the explanatory variables. We use the natural logarithm to deal with the heterogeneous distribution of the amount invested and to deal with outliers to secure the normality and homoscedasticity in the distribution.
Distance
The geographical distance may capture a myriad of factors that might be associated with the investment decision. On the one hand, for investors located nearby, the access to information is easier as they can, for example, talk to the investee’s employees, customers, or competitors. Furthermore, investors can get information on firm’s current development through the local media, or might even have personal connections to the investee’s management team (Bae et al., Citation2008; Coval & Moskowitz, Citation1999). On the other hand, geographical distance may reflect social distance. Dejean (Citation2020, p. 337) speaks of “a regional attachment or a distant relationship with a particular community,” showing that distance is not solely a proxy for information asymmetries, but also (social) connectedness and attitude toward, for example, a region are reflected in the geographical distance. Empirically, we use the variable Distance in logarithmic terms.Footnote5
We measure the physical distance between one specific startup and one specific investor using the midpoints of the startup’s and the investor’s state, rounded to full kilometers. This procedure is based on the nonavailability of data regarding the exact place of residence of the investors. However, due to the large number of Mexican states (32) and the large size of Mexico (1,973,000 km2), this proxy shows widely varying values.
Investor-startup-relationship
We also include the relationship between investors and startups into our regression analyses. Studies show that closer relationships, on average, lead to higher investment amounts (Angerer et al., Citation2017). Within the registration process on the crowdinvesting platform, investors must characterize their relationship to the startup into one of five groups: Customer, event, family/friends, maker network, or stranger. For the purpose of this study, we create a dummy variable which takes the value of one if the investor and startup have any kind of relationship, being it as family/friends, as customers or knowing each other from an event or maker network. In contrast, this variable takes the value of zero if investors denote themselves as strangers to the startup.
Likes
Within the analysis of Hypothesis 3, we take a closer look at the moderating role of the startups’ likes received on the platform on the link between distance and investment decisions. On the platform, registered users can “like” a startup if they support a startup’s idea. The current number of likes per startup is displayed on the platform as one characteristic feature on the list of potential investees presented. For each startup, we know the number of likes received at the time the data was extracted from the database. Likes received represent an observable signal to the less informed party, i.e., (potential) investors (Block et al., Citation2018). They might be seen as “a sense of community […] who share a similar interest […] that may result in increased positive attitudes towards the brand” (Coursaris et al., Citation2016, p. 3547). We include this variable into the analyses using the natural logarithm to account for smaller effects of numerically equal changes for a larger compared to a smaller number of likes.
Control variables
As control variable, we first include the financing goal within a predefined crowdinvesting period set by startups into our analysis. The financing goal describes the minimum amount of financial resources, measured in Mexican pesos, which a startup strives to get within the respective investment period. Including this variable, we follow several researchers (Ahlers et al., Citation2015; Kleinert et al., Citation2020; Vismara, Citation2016). The presentation of a startup’s financing target might correlate with investors’ behavior with regard to providing financial support or not, and regarding size. We include this variable using the natural logarithm.Footnote6
In addition, we account for three investor-specific characteristics within our analyses. We include a dummy for investors’ gender. It takes a value of one for male and a value of zero for female investors. Including this variable accounts for the lower risk aversion of men compared to women (Rieger et al., Citation2015). In turn, it is of high relevance in the comparatively risky investment form of crowdinvesting. We also include investors’ age grouped by decades into the regression. It results in seven dummy variables for investors under or equal to 30, between 31 and 40, between 41 and 50, between 51 and 60, between 61 and 70, between 71 and 80, and above 80 years of age. Thereby, we account for different stages of life that come with different financial needs and, thus, different savings or investment attitudes. The age group of investors under or equal to 30 serves as our reference group within the analyses and the interpretation of the results. Moreover, we employ the natural logarithm of investors’ time on the crowdinvesting platform in days to account for the possibility to invest in other startups listed on the crowdinvesting platform.Footnote7 We measure this experience as the time between the day of investors’ registration on the crowdinvesting platform until the day when the investment by the investor is registered in the platform’s system (= investment date). We assume that the change in investors’ experience becomes lower with a longer personal history on the platform.
Descriptive statistics
displays the summary statistics on observation level for all variables. The average distance between investor and startup amounts to 451.66 km (and 355.26 km for the smaller sample in Hypothesis 1b, Hypothesis 2, and Hypothesis 3).Footnote8 Considering the comparatively high standard deviation, our proxy for information asymmetries between investors and startups is very heterogeneously distributed. After creating all investor-startup combinations, we find an investment in approximately 3% of our observations. The average investment amount which startups receive amounts to 16,126.53 MXN (equals approximately 800 USD) with individual investments ranging from 160 to 1,490,000 MXN. The number of likes received by one startup ranges from 21 to 4,194 likes with an average amount of 1,081 likes. In 72% of all observations, investors and startups show a relationship if an investment takes place.
Table 1. Descriptive statistics.
Looking at the control variables, 75% of the investors in our sample are equal or under 39 years of age. Furthermore, 84% are male. This is in line with previous studies on the typical characteristics of crowdfunding investors (Hervé et al., Citation2019). The average investor in our sample has been registered on the platform for 195 days (that is, approximately 0.5 years) before investing in a particular startup. The financing goal by startups ranges from 109,800 to 12,100,000 MXN (equals approximately 5,500 to 605,000 USD). of the Appendix displays detailed descriptive statistics for all variables.
Demir (Citation2009) and Berre and Le Pendeven (Citation2021) state that the general macroeconomic situation matters for investment decisions in crowdinvesting. Unreported results show that we find a significant effect of most macroeconomic variables within the startups’ states of domicile whereas the macroeconomic situation in the investors’ states does not seem to matter as much in investment decisions. However, these findings lead us to include fixed effects for both investor and startup states into our subsequent analyses to capture all cross-sectional and time-invariant characteristics on both sides.
Our observation period covers the years 2015 to 2019. The duration of startups’ individual investment period ranges between 41 and 243 days with a mean of 126 days. exemplarily displays the distribution of five investment periods of different startups throughout the year 2015. We see that investment periods are differently long and can terminate within a calendar year or continue beyond. We split all individual investment periods of our sample (from the years 2015 to 2019) in quintiles, that is, five equivalently long time frames, to shed light on the investment timing. As shown in , we calculate the absolute number of investments (N) per quintile as well as the average investment amount per investor per startup (mean). We find a large number of crowdinvestors investing shortly before the end of the investment period. On average, the investment amount decreases in the mid of an investment period. Both findings suggest including fixed effects for investment timing into our further analyses to avoid biased results.
Table 2. Distribution of investments per startup investment period.
Research design
Geographical distance in investment (size) decisions
Hypothesis 1a and Hypothesis 1b focus on the geographical distance between startups and (potential) investors. We expect that a greater distance between investors and startups negatively correlates with investors’ decision to invest (Hypothesis 1a) as well as the investment amount (Hypothesis 1b). With these ideas, we follow several researchers in the field of finance and startup investments (Coval & Moskowitz, Citation1999; Lutz et al., Citation2013; Niemand et al., Citation2018). For analyzing this relationship, we employ a logit regression followed by an OLS regression. We specify the logit regression as follows:
where, in EquationEquation (1)
(1)
(1) measures if an investor i invests in startup s. In turn, we can derive the probability for startup investments via crowdinvesting given certain criteria.
is measured as the physical distance in kilometers between investor i and startup s. We also include fixed effects for startup states k (
and investor states l (
to control for characteristics in the macroeconomic environment. Including fixed effects on a more fine-grained level, for example, the individual investor or startup level, would absorb the role of some explanatory variables (especially the moderating variables Relationship and Likes mentioned below) and cost heterogeneity, which is why we refrain from including these fixed effects in the model (Papke & Wooldridge, Citation2023). Moreover, we would forfeit approximately a third of our observations in cases where only a single investment has occurred, thus diminishing the regression’s power. Therefore, we specify the OLS regression as follows:
where, describes the investment amount of investor i in startup s in Mexican pesos. We add three investor characteristics to the regression equation to control for systematic differences in these regards.
controls for the time investor i has been registered on the crowdinvesting platform.
takes the value of one for male investors and the value of zero for female investors.
measures the age of investor i using six dummy variables for the different decades j of age. The dummy variables are included for investors between 31 and 40, 41 and 50, 51 and 60, 61 and 70, 71 and 80, and over 80 years to account for biases due to differing individual financial needs. Investors under or equal to 30 years serve as the reference group. Moreover, we control for startups’ financing goal using the variable
. In EquationEquation (1)
(1)
(1) , we do not include these control variables as we cannot calculate, e.g., investors’ age at the time of investment, when no investment has taken place. We also include fixed effects for startup states k (
and investor states l (
to control for characteristics in the macroeconomic environment. In EquationEquation (2)
(2)
(2) , we add fixed effects for investment timing (
and each specific month in our time period (
to control for investment time-specific aspects.
Investor-startup-relationship
The relationship between investors and startups constitutes a crucial factor with regard to the financial support provided. Several researchers find that social connections increase the probability of receiving external funding and, in turn, the chances of startup success (Angerer et al., Citation2017; Mollick, Citation2014). Therefore, we expect in Hypothesis 2 that ”an existing investor-startup relationship positively mitigates the role of geographical distance between investors and startups on the investment amount.” We analyze this by including relationship as a main coefficient and an interaction term between distance and relationship in our model.
Startup likes
We investigate the effects of signals, in this case, likes received on the crowdinvesting platform, on investment decisions. Startup likes are an observable signal by a third party about the perceived quality of a startup. Following Mochon et al. (Citation2017) and Carr et al. (Citation2018), we expect that ”the received likes positively mitigate the role of geographical distance between investors and startups on the investment amount” (Hypothesis 3). Instead of relationship, we now include a main coefficient for the received likes and an interaction term for likes and distance in our model.
Empirical results
The role of geographical distance
Column (1) of displays the regression results of a logit regression investigating the role of distance on the investment probability. Columns (2) and (3) depict the results of an OLS regression for distance and the investment amount. In line with our hypotheses and most studies in the research area of crowdfunding, we find a negative correlation between greater distance and the investment probability (−0.1420, p-value: <0.0001) as well as the investment amount (−0.0389, p-value: 0.0003).
Table 3. The link between distance and investment decisions.
In contrast to researchers arguing that the rising use of the Internet diminishes the importance of geographic distance for investment decisions (Agrawal et al., Citation2015; Hornuf & Neuenkirch, Citation2017), we find that distance still matters in crowdinvesting. A 10% increase in distance, corresponds to a decrease in the investment probability by 1.42 pp and the investment amount by 0.39%.Footnote9 Both results are statistically significant at the 1% level. This result can be explained with two arguments. First, greater distance might lead to higher information asymmetries which result in higher uncertainty for investors. Investors with a larger distance to a startup are neither able to easily observe the startup’s progress nor can they directly gather soft personal information on or by the startup’s management. Therefore, investors further apart have to rely on hard information and informational reports created by the startup and published on the crowdinvesting platform. This hard information does not seem to have the same informative value for investors as soft information transferred with lower distance. The finding might be explained by the riskiness of startup investments which cannot easily be measured by hard facts (Carpenter & Petersen, Citation2002). Second, since the invested amounts are relativley small on average and the cognitive effort might not be as high as for larger investments, investors might (unconsciously) engage in a cognitive bias, leading them to prefer startups located closer to themselves. A more positive attitude, triggered through the mere exposure effect, to the closer area could be transferred to the startups in this area and lead to a feeling of being more connected to them, supporting investors’ desire to invest. However, both explanations seem reasonable and to get more clarity, the use of, for example, investor interviews is needed for more insights.
A longer time being registered on the crowdinvesting platform is significantly negatively correlated with the investment amount. We explain this finding by the idea that more experienced investors show lower risk appetite, are less overconfident and diversify their portfolio more compared to less experienced investors (Goetzmann & Kumar, Citation2008; Morin & Suarez, Citation1983). These more experienced investors might be more cautious due to previous (unsuccessful) crowdinvesting activities and thus prefer to conduct a large number of small investments in different projects. Furthermore, we find that men invest, on average, 21.90% more compared to women.Footnote10 As startup investments, and especially crowdinvesting activities, are usually characterized as a risky investment alternative, this is in line with Rieger et al. (Citation2015) who state that men are less risk-averse than their female counterparts.
In addition, we find that the investment amount is higher if the startup has published a larger financing goal. A 10% increase in the financing goal is associated with a 4.80% increase in the individual investment amount. Moreover, the results show an inverted u-shape for the link between investors’ age and the investment amount. This finding appears reasonable due to different stages in life with different financial bases and financial needs.
The role of the investor-startup-relationship
In the following step, we analyze the link of an existing relation between investors and startups on the actual amount provided by investors. As for the whole study, we focus on the first investment of each bilateral investor-startup-combination. We distinguish between investors who are complete strangers to the startup, and investors who show some kind of relationship to the startup, that is, who are characterized as family/friends, customers, or knowing the startup through an event or maker network in our dataset.
Hypothesis 2 suggests that an existing investor-startup relationship positively mitigates the role of distance between investors and startups on the investment amount. displays the estimation results for Equation (3), depicting the coefficients from an OLS regression with and without the interaction of distance and an investor-startup-relationship. As above, we find a significantly negative coefficient for the distance between investors and startups (−0.0349, p-value: 0.0007). The coefficient is of similar size as in the previous analyses. As expected, we find a positive main coefficient of relationships on the investment amount (0.0956, p-value: 0.0456). Investor-startup combinations with a relationship show an investment amount which is, on average, 10.03% higher compared to those without a relationship.Footnote11 This result seems plausible as one can assume that investors with a relationship to a startup experience lower uncertainty due to previous interactions with the startup. Overall, our findings display that both distance and the relationship between startups and investors matter for the investment amount.
Table 4. The link between distance, the startup-investor-relationship, and investment size.
In contrast to our expectations, the interaction coefficient of distance and relationship appears nonsignificant (−0.0014, p-value: 0.8886). Thus, we cannot say that investor-startup relationships counteract the negative main coefficient of distance. Even though it might seem counterintuitive at first glance, this finding is in line with the idea by Lee and Persson (Citation2016). The authors claim that startups should not solely use funding by family and friends as, in times of trouble, “using family finance as risk capital undermines the preexisting familial insurance arrangement” (p. 2344). Hernandez-Trillo et al. (Citation2005) and Hill et al. (Citation2021) underscore this idea. They show family and friends to serve as a “type of insurance” or “safety net,” respectively, in financially difficult times.
We also find statistically significant results regarding investors’ gender, experience, and all age groups except for the one with people 80+ years of age. The coefficients remain qualitatively similar as in the analyses above.
The role of startup likes
In the next step, we analyze the role of signals on investment decisions via crowdinvesting. As one specific measurable signal, we employ the amount of likes received, which is published on the crowdinvesting website. Block et al. (Citation2018) show that likes influence funding decisions in startups. We assume a higher number of likes to be a positive signal for startups’ future evolution and thus to reduce the negative role of physical distance on investment decisions. Consequently, Hypothesis 3 suggests that “the received likes positively mitigate the role of geographical distance between investors and startups on the investment amount.”
displays the estimation results for Equation (4). In columns (3) and (4), we find negative coefficients for a higher distance between investor and startup, and the investment amount. In contrast to our expectations that more likes lead to a higher investment amount, we find a statistically significant negative main coefficient (−0.0818, p-value: 0.0136). This is surprising at first sight. We explain this finding with the idea that projects with a high number of likes are seen as comparatively safe to be financed in the short run. Therefore, investors hop on to these projects with a small amount of money to benefit from investments by other investors. At the same time, these investors seem to keep risk diversification in mind. Thereby, they exploit the indirect support from other investors and can still split their total investment amount on several projects, reducing their individual risk.
Table 5. The link between distance, startup likes, and investment decisions.
The interaction coefficient between distance and likes counteracts the negative main coefficients of these variables. With a value of 0.0078, the interaction coefficient is small but statistically significant (p-value: 0.0451). However, it is still of economic significance. For the mean distance in our sample, a 1% increase in the number of likes is associated with an increase in the investment amount of approximately 3.1 pp.Footnote12 As in our previous analyses, the results with regard to investors’ gender, age, and experience are still statistically significant and of similar size as above.
Columns (1) and (2) support our idea of an existing link between likes and investment decisions. We find a substantially larger main coefficient for likes (0.8093, p-value: <0.0001) compared to distance (−0.1457, p-value: <0.0001). Thus, a higher number of likes positively correlate with the investment probability. This indicates that investors rely on other investors’ assessments in their decision-making. This is in line with the literature (Coursaris et al., Citation2016). The positive main coefficient of likes persists when including the interaction between distance and likes. In contrast, the negative main coefficient of distance turns nonsignificant (−0.1137, p-value: 0.2030) which displays that the interaction term captures part of the initial main effect. However, the interaction does not seem to explain sufficiently much of the effect on our dependent variable as the coefficient of this interaction term is also nonsignificant (0.0052, p-value: 0.7262). Summing up, our results suggest a link between physical distance, likes, and investment decisions.
Robustness tests
We first repeat the analyses from above only for observations with investors from outside Mexico City which is by far the largest city of Mexico with approximately 22 million inhabitants.Footnote13 66.25% of our investors are from outside the capital city. Thereby, we account for differences in the general mind-set of investors living in urban versus more rural areas. Bhayani et al. (Citation2019, p. 3775) confirm that “there is considerable difference observed in investment behavior of urban and rural investors, with respect to the choice of investment.” While the authors observe this fact within India, also for American investors it appears to exist (Copeland, Citation2022). Sachan and Chugan (Citation2020) go even further and explain that information processing is more limited for rural investors. Moreover, Chakravarty and Ahsan (Citation2023) find that campaigns from global cities are more likely to be successful and receive more funding. Thus, it is of main importance to further investigate the differences in investment decisions between rural and urban investors, leading us to our first robustness test.
As above, we find negative coefficients for distance in , showing that a higher distance decreases the investment probability as well as the investment amount. In contrast to the results above, we find a different importance of relationships in this sample. Whereas in the case when including all investors from inside and outside Mexico City the main coefficient of the dummy variable for relationship is significantly positive, it turns nonsignificant when reconducting the analyses for investors only from outside Mexico City. Several studies show that people living in rural areas give higher weight to the bonding to family and friends (House et al., Citation1988; Sørensen, Citation2016). We have in mind that it is recommendable for early stage firms to keep family and friends to the greatest extent possible as fallback option when following risky business ideas (Hernandez-Trillo et al., Citation2005). While we find that investors from rural areas seem to be aware of and consider this concept in their decision on the investment amount, investors from urban areas seem to ignore this argument. The main coefficients for likes on the investment probability and investment amount, are similar to above. We again find a significantly positive coefficient for likes on the investment probability and a significantly negative coefficient for the investment amount. The interaction term in column (5) of turns nonsignificant but remains of same size and direction. This finding has to be interpreted with caution but hints toward the idea that likes are more important for urban investors compared to investors living in rural areas.
Table 6. Results excluding observations from investors in Mexico-City.
In the following, we further investigate the role of a higher distance between investors and startups. For this purpose, we exclude investments within the same state.Footnote14 In , we find more negative coefficients for distance compared to the analyses above. This seems reasonable when excluding observations with zero distance between startup and investor. Interestingly, the main coefficient for relationship turns from significantly positive to significantly negative. For investors who are not located in the same state as the investee, having a relationship to the startup negatively correlates with the investment amount. This finding underscores the idea that these investors are aware of the thought that related parties should not be the exclusive supporters of an affiliated startup, but rather be kept as fallback options as far as possible (Hernandez-Trillo et al., Citation2005). In contrast, investors from the same state as the startup, do not seem to keep this idea in mind. The interaction term of distance and relationship changes from nonsignificantly negative to significantly positive. This change seems reasonable for information purposes and uncertainty reduction. If both parties are located in states which are far apart from each other, the information flow is not as easy as in the case of spatial proximity. A relationship could decrease information asymmetries or uncertainty regarding the startup’s quality and allows a smoother information flow. In particular, relationships offset opportunity costs for seeking information (Agrawal et al., Citation2008). The main coefficients for likes are similar as in the main analyses. The coefficient of the interaction term turns again nonsignificant, but remains of the same direction as above.
Instead of including the natural logarithm of the investor-startup-distance into the regression analyses, we now include dummy variables for each quintile as independent variables. The lowest quintile, which serves as reference group in the analysis, contains only zero-distance observations.Footnote15 The highest quintile comprises all investor-startup combinations with a distance of equal to or more than 897 km. Thereby, we strive to look at which distances exactly matter in investment decisions.Footnote16
of the Appendix confirms that a greater distance is associated with a decrease in the investment probability and investment amount. Compared to investor-startup combinations from the lowest-distance quintile, the probability to invest decreases by 1.15 ppFootnote17 and the investment amount decreases by 44.83%Footnote18 for investors with the largest distance to their investees. The results show that there is no threshold above which an increase in distance does not influence the investment decision more negatively anymore. This contradicts Zook (Citation2002) who states that investors do not invest if they cannot drive to the startup. They find this limit in the investor-startup distance to be approximately 100 km. However, we cannot confirm this idea as coefficients become more negative with increasing quintiles. Compared to the main analyses, the coefficients measuring the role of distance appear relatively large. This can be explained by the fact that the dummy variables in this part of the analyses measure the correlation compared to the base group of investor-startup pairs with a very low distance.
Moreover, likes received on a platform and relationship might be endogenous variables. To alleviate this concern, we conduct an instrumental variable-free approach, namely the Gaussian copula method, since this approach offers advantageous features (Hult et al., Citation2018). Following Papies et al. (Citation2017), this approach allows for a statistical test for the existence of endogeneity problems. One key requirement is that the potentially endogenous variable has to pass a nonnormality test (Park & Gupta, Citation2012). We use the Shapiro–Wilk to test for this nonnormality which is in line with previous literature and the most commonly test used by researchers in this regard (Becker et al., Citation2022). Unreported results show that Likes is nonnormally distributed and the Gaussian copula approach can be applied. For Relationship as a binary variable, the distribution is also nonnormal. The inclusion of the Gaussian copula in both regressions leads to a nonsignificant coefficient of the copula term, which indicates that an endogeneity problem should not exist (Papies et al., Citation2017). The main coefficients of the relationship and likes remain of similar size and significance as before, strengthening our main results.
Last, we investigate if the level of social proximity within a relationship matters for investment decisions. We now qualify only those investors as having a relationship to a startup when they are marked as “family/friends” in the platform’s managerial system. Investors knowing the startup as customers, from a fair, or through a maker network are now considered as having no relationship, that is, being strangers to the startup. Crowdinvesting describes a form of financing which startups usually choose in a very early development stage. In this stage, they often use mainly family and friends as financing source (Angerer et al., Citation2017) even though it might not be optimal (Lee & Persson, Citation2016). As displays, we find a negative main coefficient for distance and a positive main coefficient for relationship. While the size and significance of the coefficient of distance stay similar as in the main analyses above, the main coefficient for the relationship strongly increases, indicating that a close relationship correlates with a high individual investment amount.
Possible limitations and implications for future research
Several aspects constitute possible limitations to our analyses and call for future research. There are limitations regarding the dataset. The analyses are based on data stemming from one individual crowdinvesting platform which depicts the largest one in Mexico. This might lead to a self-selection bias with regard to the fact that this specific platform might attract certain participants of the financial sector more than others. In addition, our sample, by construction, only constitutes of investors having access to the Internet and being open-minded regarding innovative investment opportunities. However, the large size of our platform enables us to exploit a large heterogeneity in our variables of interest. Furthermore, we control for investor-specific and startup-specific characteristics.
Furthermore, we know about potential endogeneity within our data. Endogeneity among the observations with regard to the investment probability, the investment amount as well as our independent variables might lead to biased results. Exemplarily, this might be the risk-return profile of a particular startup, the time horizon of startups’ dividend payments, or state-specific regulation of the financial sector. We control for this issue by including startup-state and investor-state fixed effects, as well as time and investment timing fixed effects. In addition, by conducting the Gaussian copula approach, we empirically test that no endogeneity problem is present. The idea of measuring investor experience using investors’ time being registered on the platform might also not be the ideal proxy. We argue that crowdinvesting activities are not comparable to any other equity investment alternative, which leaves space for future research, employing other proxies to measure investor experience. The idea of measuring investor experience using investors’ time being registered on the platform might also not be the ideal proxy. We argue that crowdinvesting activities are not comparable to any other equity investment alternative, which leaves space for future research, employing other proxies to measure investor experience.
One minor argument might be related to Goethner et al. (Citation2021) who show that different factors and signals influence crowdinvestors differently. Hence, they claim that it is difficult to measure the impact of a certain signal on a heterogeneous group of investors. This concern is not of major importance in our study as our database contains in-depth information on investors as well as startups. However, this topic leaves space for future research following Piva and Rossi-Lamastra (Citation2018) who call for deeper analyses with regard to the effect of signals on different types of investors.
Conclusion
In this study, we compile detailed information on investor and startup characteristics to reveal the factors influencing investment decisions in crowdinvesting. Specifically, we look at the effect of distance between investors and startups on the investment probability and investment amount. We investigate the moderating role of relationships and signals in this context. Recent studies show that the physical distance correlates with a lower investment probability and a lower investment amount (Bae et al., Citation2008; Dejean, Citation2020). In crowdinvesting, information costs are comparatively high as individual investments are usually small and little contact between investors and startups precedes the investment. In those cases where investor and startup already have some kind of relationship (for example, through connections as family or friends, through meetings at a fair, and so on), uncertainty decreases, which might lead investors to support startups in a different way (Angerer et al., Citation2017; Mollick, Citation2014). Furthermore, objectively observable signals which a startup has received on a platform, influence investors’ decision-making (Courtney et al., Citation2017).
Our sample consists of 407,497 investor-startup combinations including 11,054 investments. We use data from the largest Mexican crowdinvesting platform and investigate the characteristics associated with investors’ decision-making regarding their distance to the startup, relationships, and signals. First, we find that distance correlates negatively with the investment probability and the investment amount. A 10% increase in distance is associated with a decrease in the investment probability by 1.42 pp and the investment amount by 0.39%. In contrast, lower investor experience, male gender, and a higher financing goal correlate positively with the individual investment amount.
Second, Investors with a relationship to the startup, on average, invest more than those not having any kind of connection to the investee. However, relationships are not able to counteract the negative main role of higher distance in any case. While rural investors seem to be aware of the idea of startups keeping people with a relationship, especially family and friends, as fallback options for the case of a negative development of the startup (Hernandez-Trillo et al., Citation2005; Lee & Persson, Citation2016), investors from urban areas do not seem to consider this idea. In the final part of this paper, we measure signals through likes on the crowdinvesting platform. We observe that signals positively correlate with the investment probability but have a significantly negative role on the investment amount. This is in line with the idea that private investors care about the opinion from other investors but also consider the concept of risk diversification.
Investigating the role of physical distance, relationships and signals on investment decisions is of main importance for researchers, politicians as well as all participants of the financial sector. Crowdinvesting constitutes a more and more important investment alternative for nonprofessional investors. With an expected increase in market transactions of 4–5% in the upcoming years in the U.S., Mexico, and Germany, crowdinvesting is currently experiencing a boom in its evolution. A few years ago, policymakers identified crowdfunding as a possibility to overcome geographic boundaries (Guenther et al., Citation2018). Given the currently rising inflation rates and still low interest rates on traditional investment opportunities, traditional equity investments and also nontraditional investment opportunities, for example, crowdinvesting, has become an almost inevitable approach for private investors to think about when deciding their investment alternatives. As equity investments occupy a more and more relevant part of the financial market, understanding its functionality and the factors influencing its development is of high interest for all participants of the financial system.
In addition, it is widely known that family and friends serve as first financial support in the very early stage of startups’ existence (Angerer et al., Citation2017). However, in order to receive sufficient funding for future development, other financing sources should be taken into account (Lee & Persson, Citation2016). Understanding the interplay between social and geographical distance and the investor-startup-relationship is thus of main importance for startups. Based on our findings, startups should be mindful of geographical factors that may influence their audience’s behavior. It appears advantageous for startups to establish a presence in the minds of investors. Regular exposure to a startup can positively shape investors’ perceptions and potentially increase their willingness to invest. If startups aim to expand and attract funding from investors in distant locations with differing cultural or social norms, employing an offensive advertising strategy in these regions could effectively introduce the startup and foster a favorable impression. This approach also helps mitigate information gaps by educating potential investors about the startup. Tailored advertising strategies, readily accessible through platforms like social media, are predestined for this purpose. Furthermore, startups that cultivate a sense of community may experience accelerated growth. When investors feel a strong connection to the brand and its supporters, demonstrated through engagement on social media or website interactions, it may attract new investors influenced by existing investors’ behavior. Consequently, among other factors, the presentation of the startup itself, which is multidimensional (as seen in Ahsan et al., Citation2018), emerges as a crucial factor. However, further analyses and insights from disciplines such as marketing, psychology, and social science are required to fully understand the mechanics of this sense of “belonging.”
Acknowledgments
We thank Amelie Wulff for her excellent research assistance. Moreover, we thank Victoria Bozhenko, Werner Neus, Jan Riepe, and the participants of the BFGA conference 2022 and the internal research seminar for their valuable comments and insights.
Disclosure statement
The authors have received proprietary data from a commercial platform as indicated in the paper. Beyond that, there is no connection to the data provider.
Notes
1 Sah and Stiglitz (Citation1986, p. 716) subsume such phenomena under “the architecture of an economic system [which] affects the errors made by individuals within the system, as well as how these errors are aggregated.” Ultimately, these interdependencies describe Bayesian arguments.
2 In studies analyzing relationships in investment decisions, a “relationship” may also describe the situation of having already invested in a startup (e.g., Sorenson and Stuart Citation2001). We define the term “relationship” for this study in our section on Variables.
3 The same argument holds for Hypothesis 3 when investigating the role of likes.
4 The State of Mexico describes the surrounding area of Mexico City. The capital cities of Jalisco and Nuevo Léon are Guadalajara and Monterrey, which are the second and third largest city in Mexico, respectively. Thus, this finding is not surprising.
5 We add one to the investor-startup-distance of all observations. Thereby, we keep investor-startup-combinations from the same state. We proceed similar for all other variables which are measured using the natural logarithm (investment amount, financing goal, investor experience, and startup likes).
6 One could also imagine taking the already collected proportion of the financing goal as control variable into the analysis. However, we miss information on the overall investment amount by all investors in each particular point in time. In addition, we cannot sum up individual investments to get this amount as we only include first investments per bilateral investor-startup-combination into our analysis.
7 This study concentrates on the first bilateral investor-startup interaction. Thus, investors cannot collect experience from former investments into the same startup.
8 For understandability purposes, we describe absolute numbers in the text instead of the natural logarithms which are depicted in .
9 Calculation of the investment probability: Marginal effects as displayed in Derivation A1 of the Appendix.
10 Calculation: ( −1) * 100.
11 Calculation: ( −1) * 100.
12 Calculation: 0.0818 − (0.0818 − 0.0078 * 3.9733).
13 The second and third largest cities of Mexico, that is, Guadalajara (Jalisco) and Monterrey (Nuevo Léon), show a population of approximately one quarter of the population of Mexico City each.
14 Unreported evidence shows that 87.63% of these zero-distance-observations describe investments within Mexico City. This equals 95.04% of the observations in our larger sample for the analysis of Hypothesis 1a.
15 It is alike in the smaller sample in Hypothesis 1b, Hypothesis 2, and Hypothesis 3 with 3,387 observations in the lowest quintile.
16 of the Appendix displays further details on the descriptive statistics.
17 Calculation: Marginal effects as displayed in Derivation A1 of the Appendix.
18 Calculation: ( −1) * 100.
References
- Agrawal, A., Catalini, C., & Goldfarb, A. (2015). Crowdfunding: Geography, social networks, and the timing of investment decisions. Journal of Economics & Management Strategy, 24(2), 253–274. https://doi.org/10.1111/jems.12093
- Agrawal, A., Kapur, D., & McHale, J. (2008). How do spatial and social proximity influence knowledge flows? Evidence from patent data. Journal of Urban Economics, 64(2), 258–269. https://doi.org/10.1016/j.jue.2008.01.003
- Ahlers, G. K. C., Cumming, D., Günther, C., & Schweizer, D. (2015). Signaling in equity crowdfunding. Entrepreneurship Theory and Practice, 39(4), 955–980. https://doi.org/10.1111/etap.12157
- Ahsan, M., Cornelis, E. F. I., & Baker, A. (2018). Understanding backers’ interactions with crowdfunding campaigns. Journal of Research in Marketing and Entrepreneurship, 20(2), 252–272. https://doi.org/10.1108/JRME-12-2016-0053
- Akerlof, G. A. (1970). The market for “lemons”: Quality uncertainty and the market mechanism. Quarterly Journal of Economics, 84(3), 488–500. https://doi.org/10.2307/1879431
- Angerer, M., Brem, A., Kraus, S., & Peter, A. (2017). Start-up funding via equity crowdfunding in germany – a qualitative analysis of success factors. Journal of Entrepreneurial Finance, 19(1), 1–34. https://www.econstor.eu/handle/10419/197535
- Bae, K.-H., Stulz, R. M., & Tan, H. (2008). Do local analysts know more? A cross-country study of the performance of local analysts and foreign analysts. Journal of Financial Economics, 88(3), 581–606. https://doi.org/10.1016/j.jfineco.2007.02.004
- Bandura, A. (1977). Social learning theory. Prentice-Hall (Prentice-Hall series in social learning theory).
- Banerji, D., & Reimer, T. (2019). Startup founders and their LinkedIn connections: Are well-connected entrepreneurs more successful? Computers In Human Behavior, 90, 46–52. https://doi.org/10.1016/j.chb.2018.08.033
- Beck, T., Demirgüç-Kunt, A., & Maksimovic, V. (2006). The influence of financial and legal institutions on firm size. Journal of Banking and Finance, 30(11), 2995–3015. https://doi.org/10.1016/j.jbankfin.2006.05.006
- Becker, J.-M., Proksch, D., & Ringle, C. M. (2022). Revisiting Gaussian copulas to handle endogenous regressors. Journal of the Academy of Marketing Science, 50(1), 46–66. https://doi.org/10.1007/s11747-021-00805-y
- Berre, M., & Le Pendeven, B. (2021). Startups, growth, and the macroeconomic environment: Valuation and business cycles. Working Paper. http://entfin.org/wp-content/uploads/2021/06/startups-growth-and-the-macroeconomic-environment-valuation-and-business-cycles.pdf
- Bhayani, M., Bobade, P., Malkar, V., & Kawale, P. (2019). Disparity in investment behavior of urban and rural individual investors. International Journal of Social Science and Economic Research, 4(5), 3764–3776.
- Bikhchandani, S., Hirshleifer, D., & Welch, I. (1992). A theory of fads, fashion, custom, and cultural change as informational cascades. Journal of Political Economy, 100(5), 992–1026. https://doi.org/10.1086/261849
- Block, J., Hornuf, L., & Moritz, A. (2018). Which updates during an equity crowdfunding campaign increase crowd participation? Small Business Economics, 50(1), 3–27. https://doi.org/10.1007/s11187-017-9876-4
- Blum, B. S., & Goldfarb, A. (2006). Does the internet defy the law of gravity? Journal of International Economics, 70(2), 384–405. https://doi.org/10.1016/j.jinteco.2005.10.002
- Bornstein, R. F., & Craver-Lemley, C. (2022). Mere exposure effect. In R. F. Pohl (Ed.), Cognitive illusions (pp. 241–258). Routledge.
- Carpenter, R., & Petersen, B. (2002). Capital market imperfections, high-tech investment, and new equity financing. The Economic Journal, 112(477), F54–F72. https://doi.org/10.1111/1468-0297.00683
- Carr, C. T., Hayes, R. A., & Sumner, E. M. (2018). Predicting a threshold of perceived facebook post success via likes and reactions: A test of explanatory mechanisms. Communication Research Reports, 35(2), 141–151. https://doi.org/10.1080/08824096.2017.1409618
- Chakravarty, D., & Ahsan, M. (2023). The effect of global city founding location on crowdfunding success and internationalization. Academy of Management Proceedings, 2023(1). https://doi.org/10.5465/AMPROC.2023.13347abstract
- Chen, H., Gompers, P., Kovner, A., & Lerner, J. (2010). Buy local? The geography of venture capital. Journal of Urban Economics, 67(1), 90–102. https://doi.org/10.1016/j.jue.2009.09.013
- Copeland, C. (2022). Understanding the finances of rural vs. urban Americans. EBRI Issue Brief / Employee Benefit Research Institute, 553, 1–16.
- Cornelis, E. F. I., Baker, A. M., & Ahsan, M. (2022). Mitigating a crisis of confidence: The effect of crisis response strategies on reward‐based crowdfunding success. Strategic Entrepreneurship Journal, 16(1), 67–96. https://doi.org/10.1002/sej.1408
- Coursaris, C. K., van Osch, W., & Balogh, B. A. (2016). Do facebook likes lead to shares or sales? exploring the empirical links between social media content, brand equity, purchase intention, and engagement. In 2016 49th Hawaii International Conference on System Sciences (HICSS) (pp. 3546–3555). IEEE.
- Courtney, C., Dutta, S., & Li, Y. (2017). Resolving information asymmetry: Signaling, endorsement, and crowdfunding success. Entrepreneurship Theory and Practice, 41(2), 265–290. https://doi.org/10.1111/etap.12267
- Coval, J. D., & Moskowitz, T. J. (1999). Home bias at home: Local equity preference in domestic portfolios. The Journal of Finance, 54(6), 2045–2073. https://doi.org/10.1111/0022-1082.00181
- Davila, A., Foster, G., & Gupta, M. (2003). Venture capital financing and the growth of startup firms. Journal of Business Venturing, 18(6), 689–708. https://doi.org/10.1016/S0883-9026(02)00127-1
- Degryse, H., & Ongena, S. (2005). Distance, lending relationships, and competition. The Journal of Finance, 60(1), 231–266. https://doi.org/10.1111/j.1540-6261.2005.00729.x
- Dejean, S. (2020). The role of distance and social networks in the geography of crowdfunding: Evidence from France. Regional Studies, 54(3), 329–339. https://doi.org/10.1080/00343404.2019.1619924
- Demir, F. (2009). Macroeconomic uncertainty and private investment in Argentina, Mexico and Turkey. Applied Economics Letters, 16(6), 567–571. https://doi.org/10.1080/13504850701206528
- DiPietro, F., Grilli, L., & Masciarelli, F. (2020). Talking about a revolution? Costly and costless signals and the role of innovativeness in equity crowdfunding. Academy of Management Proceedings, (1), 1–32. https://doi.org/10.1080/00472778.2020.1816435
- Eranti, V., & Lonkila, M. (2015). The social significance of the Facebook Like button. First Monday. https://doi.org/10.5210/fm.v20i6.5505
- Goethner, M., Luettig, S., & Regner, T. (2021). Crowdinvesting in entrepreneurial projects: Disentangling patterns of investor behavior. Small Business Economics, 57(2), 905–926. https://doi.org/10.1007/s11187-020-00332-0
- Goetzmann, W. N., & Kumar, A. (2008). Equity portfolio diversification. Review of Finance, 12(3), 433–463. https://doi.org/10.1093/rof/rfn005
- Guenther, C., Johan, S., & Schweizer, D. (2018). Is the crowd sensitive to distance? How investment decisions differ by investor type. Small Business Economics, 50(2), 289–305. https://doi.org/10.1007/s11187-016-9834-6
- Hernandez-Trillo, F., Pagan, J. A., & Paxton, J. (2005). Start-up capital, microenterprises and technical efficiency in Mexico. Review of Development Economics, 9(3), 434–447. https://doi.org/10.1111/j.1467-9361.2005.00286.x
- Hervé, F., Manthé, E., Sannajust, A., & Schwienbacher, A. (2019). Determinants of individual investment decisions in investment-based crowdfunding. Journal of Business Finance & Accounting, 46(5–6), 762–783. https://doi.org/10.1111/jbfa.12372
- Hill, K., Hirsch, D., & Davis, A. (2021). The role of social support networks in helping low income families through uncertain times. Social Policy & Society, 20(1), 17–32. https://doi.org/10.1017/S1474746420000184
- Hornuf, L., & Neuenkirch, M. (2017). Pricing shares in equity crowdfunding. Small Business Economics, 48(4), 795–811. https://doi.org/10.1007/s11187-016-9807-9
- Hornuf, L., Schmitt, M., & Stenzhorn, E. (2022). The local bias in equity crowdfunding: Behavioral anomaly or rational preference? Journal of Economics & Management Strategy, 31(3), 693–733. https://doi.org/10.1111/jems.12475
- House, J. S., Umberson, D., & Landis, K. R. (1988). Structures and processes of social support. Annual Review of Sociology, 14(1), 293–318. https://doi.org/10.1146/annurev.so.14.080188.001453
- Hult, G., Tomas, M., Hair, J., Proksch, F., Dorian, S., Marko, P., Andreas, R., & Christian, M. (2018). Addressing endogeneity in international marketing applications of partial least squares structural equation modeling. Journal of International Marketing, 26(3), 1–21. https://doi.org/10.1509/jim.17.0151
- Kleinert, S., & Volkmann, C. (2019). Equity crowdfunding and the role of investor discussion boards. Venture Capital, 21(4), 327–352. https://doi.org/10.1080/13691066.2019.1569853
- Kleinert, S., Volkmann, C., & Grünhagen, M. (2020). Third-party signals in equity crowdfunding: The role of prior financing. Small Business Economics, 54(1), 341–365. https://doi.org/10.1007/s11187-018-0125-2
- Konovalova, M. E., Kuzmina, O. Y., & Zhironkin, S. A. (2020). Digital technologies as a factor of expanding the investment opportunities of business entities. In S. I. Ashmarina, M. Vochozka, & V. V. Mantulenko (Eds.), Digital Age: Chances, challenges and future (Vol. 84, pp. 180–188). Springer International Publishing (Lecture Notes in Networks and Systems.
- Kwan, L.-Y.-Y., Yap, S., & Chiu, C.-Y. (2015). Mere exposure affects perceived descriptive norms: Implications for personal preferences and trust. Organizational Behavior and Human Decision Processes, 129, 48–58. https://doi.org/10.1016/j.obhdp.2014.12.002
- Lee, S., & Persson, P. (2016). Financing from family and friends. The Review of Financial Studies, 29(9), 2341–2386. https://doi.org/10.1093/rfs/hhw031
- Levine, R. (2005). Finance and growth: Theory and evidence. In P. Aghion & S. N. Durlauf (Eds.), Handbook of economic growth (Vol. 1.1A, pp. 865–934). Elsevier (Handbooks in economics.
- Leyden, D. P., Link, A. N., & Siegel, D. S. (2014). A theoretical analysis of the role of social networks in entrepreneurship. Research Policy, 43(7), 1157–1163. https://doi.org/10.1016/j.respol.2014.04.010
- Li, X., & Wu, L. (2013). Observational learning and social-network word-of-mouth: Evidence from Groupon. SSRN Electronic Journal. https://doi.org/10.2139/ssrn.2264411
- Lindblom, T., Mavruk, T., & Sjögren, S. (2018). East or west, home is best: The birthplace bias of individual investors. Journal of Banking and Finance, 92, 323–339. https://doi.org/10.1016/j.jbankfin.2016.10.002
- Lutz, E., Bender, M., Achleitner, A.-K., & Kaserer, C. (2013). Importance of spatial proximity between venture capital investors and investees in Germany. Journal of Business Research, 66(11), 2346–2354. https://doi.org/10.1016/j.jbusres.2012.04.016
- Malloy, C. J. (2005). The geography of equity analysis. The Journal of Finance, 60(2), 719–755. https://doi.org/10.1111/j.1540-6261.2005.00744.x
- Mochon, D., Johnson, K., Schwartz, J., & Ariely, D. (2017). What are likes worth? A facebook page field experiment. Journal of Marketing Research, 54(2), 306–317. https://doi.org/10.1509/jmr.15.0409
- Mollick, E. (2014). The dynamics of crowdfunding: An exploratory study. Journal of Business Venturing, 29(1), 1–16. https://doi.org/10.1016/j.jbusvent.2013.06.005
- Monti, M., Pelligra, V., Martignon, L., & Berg, N. (2014). Retail investors and financial advisors: New evidence on trust and advice taking heuristics. Journal of Business Research, 67(8), 1749–1757. https://doi.org/10.1016/j.jbusres.2014.02.022
- Morin, R.-A., & Suarez, A. F. (1983). Risk aversion revisited. The Journal of Finance, 38(4), 1201. https://doi.org/10.1111/j.1540-6261.1983.tb02291.x
- Niemand, T., Angerer, M., Thies, F., Kraus, S., & Hebenstreit, R. (2018). Equity crowdfunding across borders: A conjoint experiment. International Journal of Entrepreneurial Behavior and Research, 24(4), 911–932. https://doi.org/10.1108/IJEBR-07-2017-0256
- Nitani, M., Riding, A., & He, B. (2019). On equity crowdfunding: Investor rationality and success factors. Venture Capital, 21(2–3), 243–272. https://doi.org/10.1080/13691066.2018.1468542
- OECD. (2019). An introduction to online platforms and their role in the digital transformation. https://www.oecd.org/innovation/an-introduction-to-online-platforms-and-their-role-in-the-digital-transformation-53e5f593-en.htm
- OECD. (2022). OECD Economic surveys (Mexico, May 2019). https://www.oecd-ilibrary.org/economics/oecd-economic-surveys-mexico-2019_a536d00e-enhttp://www.oecd.org/economy/mexico-economic-snapshot/
- Papies, D., Ebbes, P., & van Heerde, H. J. (2017). Addressing endogeneity in marketing models. In P. S. H. Leeflang, J. E. Wieringa, T. H. A. Bijmolt, & K. H. Pauwels (Eds.), Advanced methods for modeling markets (pp. 581–627). Springer International Publishing (International Series in Quantitative Marketing.
- Papke, L. E., & Wooldridge, J. M. (2023). A simple, robust test for choosing the level of fixed effects in linear panel data models. Empirical Economics, 64(6), 2683–2701. https://doi.org/10.1007/s00181-022-02337-y
- Park, S., & Gupta, S. (2012). Handling endogenous regressors by joint estimation using Copulas. Marketing Science, 31(4), 567–586. https://doi.org/10.1287/mksc.1120.0718
- Piva, E., & Rossi-Lamastra, C. (2018). Human capital signals and entrepreneurs’ success in equity crowdfunding. Small Business Economics, 51(3), 667–686. https://ideas.repec.org/a/kap/sbusec/v51y2018i3d10.1007_s11187-017-9950-y.html.10.1007/s11187-017-9950-y
- Rieger, M. O., Wang, M., & Hens, T. (2015). Risk preferences around the world. Management Science, 61(3), 637–648. https://doi.org/10.1287/mnsc.2013.1869
- Rosser, J. B. (2003). A noble prize for asymmetric information. The economic contributions of george akerlof, michael spence and Joseph Stiglitz. Review of Political Economy, 15(1), 3–21. https://doi.org/10.1080/09538250308445
- Sachan, A., & Chugan, P. K. (2020). Availability bias of urban and rural investors: Relationship study of the Gujarat State of India. Journal of Behavioural Economics, 8(1), 1–6. https://doi.org/10.12691/jbe-8-1-1
- Sah, R. K., & Stiglitz, J. E. (1986). The architecture of economic systems: Hierarchies and polyarchies. The American Economic Review, (76), 716–727. https://www.jstor.org/stable/1806069, checked on 10/18/2023.
- Saxton, G. D., & Waters, R. D. (2014). What do stakeholders like on facebook? Examining public reactions to nonprofit organizations’ informational, promotional, and community-building messages. Journal of Public Relations Research, 26(3), 280–299. https://doi.org/10.1080/1062726X.2014.908721
- Sørensen, J. F. L. (2016). Rural–urban differences in bonding and bridging social capital. Regional Studies, 50(3), 391–410. https://doi.org/10.1080/00343404.2014.918945
- Sorenson, O., & Stuart, T. E. (2001). Syndication networks and the spatial distribution of venture capital investments. The American Journal of Sociology, 106(6), 1546–1588. https://doi.org/10.1086/321301
- Stafford, T., & Grimes, A. (2012). Memory enhances the mere exposure effect. Psychology and Marketing, 29(12), 995–1003. https://doi.org/10.1002/mar.20581
- Statista. (2022a). Crowdinvesting in Germany. https://www.statista.com/outlook/dmo/fintech/alternative-financing/crowdinvesting/germany?currency=usd
- Statista. (2022b). Crowdinvesting in Mexico. https://www.statista.com/outlook/dmo/fintech/alternative-financing/crowdinvesting/mexico
- Statista. (2022c). Crowdinvesting in the United States. https://www.statista.com/outlook/dmo/fintech/alternative-financing/crowdinvesting/united-states?currency=usd
- Sumner, E. M., Hayes, R. A., Carr, C. T., & Wohn, D. Y. (2020). Assessing the cognitive and communicative properties of facebook reactions and likes as lightweight feedback cues. First Monday. https://doi.org/10.5210/fm.v25i2.9621
- Vismara, S. (2016). Equity retention and social network theory in equity crowdfunding. Small Business Economics, 46(4), 579–590. https://doi.org/10.1007/s11187-016-9710-4
- Wadhwa, A., & Kotha, S. (2006). Knowledge creation through external venturing: Evidence from the telecommunications equipment manufacturing industry. Academy of Management Journal, 49(4), 819–835. https://doi.org/10.5465/amj.2006.22083132
- Zajonc, R. B. (1968). Attitudinal effects of mere exposure. Journal of Personality and Social Psychology, 9(2), 1–27. https://doi.org/10.1037/h0025848
- Zbinden, F., & Kondova, G. (2019). Economic development in Mexico and the role of blockchain. Advances in Economics and Business, 7(1), 55–64. https://doi.org/10.13189/aeb.2019.070106
- Zook, M. A. (2002). Grounded capital: Venture financing and the geography of the Internet industry, 1994–2000. Journal of Economic Geography, 2(2), 151–177. https://doi.org/10.1093/jeg/2.2.151
Appendix
Table A1. Local distribution of investors and startups.
Table A2. Detailed descriptive statistics.
Table A3. The link between distance and investment decisions.
Table A4. Results excluding observations with investors and startups from the same state.
Table A5. Results dividing distance into 20%-quantiles.
Table A6. Results only qualifying family as not being strangers to the startup.
Derivations
Derivation A1: Marginal effects
This part displays the mathematical approach on the calculation of marginal effects. As the dependent variable in logit regressions is a dummy variable, one must interpret the coefficients using marginal effects.
As a starting point, we use the general formulation of a logistic regression with being the log-specified regressor of interest and
being a vector of covariates:
Taking the partial derivative with respect to , we obtain
This is approximately equivalent to
Thus, we obtain
The average marginal effect thus reads
Variable definitions
All “Explanatory and Dependent Variables” and the “control variables: investor and investment characteristics” are based on information retrieved from the internal management system of the crowdinvesting platform.
Table A7. Variable definitions.