ABSTRACT
Aims
To investigate the association between gastrointestinal parasites (GIP) and animal behaviour in dairy calves under New Zealand pastoral conditions, using animal-mounted, accelerometer-based sensors.
Methods
Thirty-six, 5–6-month-old, Friesian-Jersey, heifer calves fitted with animal activity sensors to track behaviour were randomly allocated to one of two treatment groups. Half the animals were challenged with an oral dose of 20,000 larvae of Ostertagia ostertagi and Cooperia oncophera once a week for 3 weeks and half were unchallenged. Five weeks after the last dose, seven infected and nine uninfected animals were treated with an oral anthelmintic (AHC) and data collected for a further week. Accelerometer data were classified into minutes per day eating, ruminating, in moderate–high activity or in low activity. Live weight and faecal egg counts (FEC) were recorded weekly over the study period. All animals co-grazed a newly sown pasture not previously grazed by ruminants and were moved every week to fresh grazing. Treatment status was blinded to those managing the animals which were otherwise treated identically.
Results
Complete behavioural records were available from 30/36 calves, (13 challenged and 17 unchallenged). Before treatment with AHC, FEC increased in infected and un-treated calves over the study, while uninfected animals maintained a near zero FEC. There was no difference in live weight gain between the two groups over the study period. Bayesian, multinomial regression predicted differences in animal behaviour between infected and uninfected animals that were not treated with AHC over the 7 weeks following initial infection. Parasitised calves not treated with AHC were less active and spent up to 6 (95% highest density interval (HDI) = 1–11) minutes/day less in low level activity and up to 15 (95% HDI = 7–20) minutes/day less in moderate to high level activity. They ruminated up to 9 (95% HDI = 2–15) minutes/day more and ate up to 10 (95% HDI = 2–19) minutes/day more than control calves that were not treated with AHC. The effect of AHC on time spent in each behaviour differed between infected and uninfected calves and increased the coefficient of dispersion of the behavioural data.
Conclusions and clinical relevance
Small differences in animal behaviour can be measured in calves with GIP. However, to use this to target treatment, further validation studies are required to confirm the accuracy of behavioural classification and understand the complex drivers of animal behaviour in a dynamic and variable pasture-parasite–host environment.
Introduction
Gastrointestinal parasites (GIP) remain an important and limiting impediment to growth within ruminant systems (Ward Citation2006). Traditionally, control of parasites has consisted of regular and repeated treatment of youngstock with anthelmintic (AHC) (Ploeger et al. Citation2000). Within New Zealand’s pastoral dairy system this has contributed to the development of resistance to AHC (Sutherland and Leathwick Citation2011) and to the highest reported rate of AHC resistance amongst cattle parasites in the world (Mason and McKay Citation2006).
The impact of GIP is not uniform within a population of young calves (Kenyon et al. Citation2009; O’Shaughnessy et al. Citation2015) and reducing the number of animals treated reduces the selective pressure for the development of resistance (Jackson et al. Citation2017). This has led to the search for markers of worm burden or measures of animal performance responsive to changes in worm burden, so that targeted selective treatment can be delivered (van Wyk et al. Citation2006).
However, current measures to quantify parasite burden such as faecal egg count (FEC), detection of parasite DNA in faecal material, markers of abomasal inflammation, and antibody response to parasitism lack sensitivity, specificity or both (Eysker and Ploeger Citation2000). Conversely, while measures used to assess animal performance such as live weight gain (LWG) can be used to reduce the amount of AHC used, these methods also lack sensitivity and specificity for identifying animals that are affected by parasitism (Höglund et al. Citation2009, Citation2013; Bates et al. Citation2022).
Observational studies suggest that one of the effects of GIP is a change in animal behaviour (Kyriazakis et al. Citation1998; Berk et al. Citation2016; Forbes Citation2021). In sheep (Hutchings et al. Citation2000) and cattle (Forbes et al. Citation2007), grazing time was observed to be reduced in parasitised animals. Recent advances in the use of accelerometers to continuously record animal behaviour have been used to quantify the effect of disease on animal behaviour, often in combination with geospatial positioning data, remote weighing or measurement of feed intake (González et al. Citation2008, Citation2015; Fan et al. Citation2022).
The accuracy of behavioural classification using this technology has been estimated at around 90% in adult cattle (Schirmann et al. Citation2009; Stygar et al. Citation2021; Chang et al. Citation2022) and an ear-mounted accelerometer designed for detecting behavioural patterns in cattle has been validated in sheep for the detection of behavioural changes induced by an experimental acute health challenge (Fan et al. Citation2023). This approach avoids the time and labour constraints inherent in direct observation and allows continuous monitoring 24 hours of the day for weeks at a time and can characterise behaviour into objective and consistent categories. There are also a growing number of studies where accelerometers have been used to record the potential effect of GIP on animal behaviour. However, the results are not always consistent (Högberg et al. Citation2019, Citation2021), vary between host species (Ikurior et al. Citation2020), and can be subtle, with an increase in bite rate despite a net decrease in intake reported in parasitised sheep (Yoshihara et al. Citation2022). This may reflect more complex patterns of behaviour emerging as part of the animals overall homeostatic response to parasitism (Kyriazakis et al. Citation1998; Burgunder et al. Citation2018).
The current study was designed to investigate the association between GIP and these estimations of animal behaviour in a group of cross-bred dairy heifer calves under New Zealand pastoral conditions, using animal monitoring sensors with behaviour classified into minutes per day spent eating, ruminating, moderately to highly active or in low activity. Our hypothesis was that animals infected with GIP would show differences in these characteristics compared to co-grazing, uninfected animals.
Materials and methods
This study was carried out under the approval of the Lincoln University Animal Ethics Committee, approval number LUAEC 2019-42. The study lasted for a total of 10 weeks from 2 September to 10 November 2020 and consisted of 2 weeks before infection (weeks −1 and −2), 2 weeks over which calves allocated to the infection group were infected (weeks 1 and 2), a further 5 weeks of monitoring with weekly FEC for both infected and control calves (weeks 3–7), before all calves were treated with AHC and monitored for 1 more week (week 8). A diagrammatic summary of the time course of study events is given in .
Figure 1. Timeline of the experimental protocol used to investigate the association between infection with gastrointestinal parasites and animal behaviour measured as minutes per day spent eating, ruminating, and at different levels of activity in dairy heifer calves under pastoral grazing management in New Zealand.
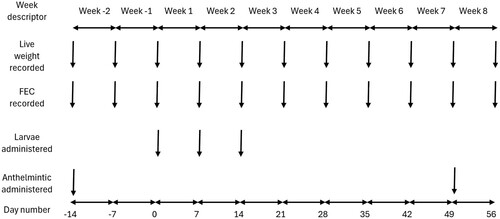
A convenience sample of 36, 5–6-month-old, autumn (March) born, Friesian-Jersey, crossbred calves was recruited from the Lincoln University dairy herd (Lincoln University Dairy Farm, Lincoln University, Canterbury, NZ) for this study. All calves were born at pasture and managed using standard New Zealand dairy calf rearing protocols (Cuttance et al. Citation2017; Bates et al. Citation2019). This will include exposure to pasture previously grazed by dairy calves and cows during the previous season. Prior to recruitment, all calves had been confirmed negative to persistent infection with bovine viral diarrhoea via a negative antigen tissue test (McDougall Citation2021) and were vaccinated twice against leptospirosis and a range of clostridial diseases using two doses of a polyvalent vaccine, 4 weeks apart (Ultravac 7in1; Zoetis New Zealand Ltd, Auckland, NZ).
Two weeks before the calves were allocated to their treatment groups (Day −14), all calves were treated with an oral AHC at 1 mL/5 kg live weight (LW), delivering 0.2 mg abamectin, 8 mg levamisole, 4.5 mg oxfendazole, 0.1 mg selenium and 0.44 mg cobalt per kg body weight (Matrix Oral for sheep; Boehringer-Ingelheim Animal Health, Auckland, NZ) and fitted with a Monitoring Neck Tag (SCR Engineers Ltd., Netanya, Israel). The neck tags were worn as a neck collar with the accelerometers positioned on the ventral aspect of each calf’s neck to measure inertial and gravitational acceleration in three orthogonal planes. The x-axis corresponding to vertical or up–down movement, the y-axis to backwards and forward movement and the z-axis to right-left movement (González et al. Citation2015).
At this same visit, calves were weighed using a purpose-built weigh system (XR 3000; Tru-Test, Hamilton, NZ), and a faecal sample digitally collected from the rectum into a factory-clean plastic pottle. All calves were then moved to a newly sown pasture (Lolium perenne) on the Ashley Dene research farm (Lincoln University, Lincoln, NZ) that had not previously been grazed, and which was considered free of ruminant nematodes. Pasture allocation was not formally recorded but all calves were grazed together as one group on this pasture (pre-grazing cover of 2,600–3,000 kg DM/ha) with a clean break of fresh pasture offered every day sufficient to allow intakes of 4–5 kg DM pasture per day. Animals were back-fenced from the previous day’s grazing and moved to an entirely fresh area of pasture every week, to minimise any cross contamination of uninfected animals.
Following live weight measurements at Day −14, all 36 calves were allocated hierarchically by LW to one of two treatment groups: half to control and half infected. On Day 0, 14 days after the initial weights and samples had been collected, infected animals were orally dosed with a mixture of Ostertagia ostertagi and Cooperia oncophera to mimic likely real-word larval challenge, and control animals were left uninfected. The larvae were administered using three doses, on Day 0, 7 and 14, of 20,000 L3 larvae of each species, which, assuming a 50% take, would have provided a cumulative challenge of 30,000 of each species in line with guidelines for the efficacy of anthelmintics (VICH Citation1999).
Five weeks from the last dose of larvae, approximately half the animals in each group were randomly selected (using the RAND function from Excel (Microsoft Corporation, Richmond, WA, USA)), for treatment with oral AHC (Matrix Oral for sheep) as described above, with all animals remaining within the experiment for a further week. This gave a 2 × 2 factorial design for the last week with four groups: control and not treated with AHC, control and treated with AHC, infected and not treated with AHC and infected and treated with AHC. At the end of the trial, all calves were treated with the same oral AHC.
Live weights were recorded weekly, and faecal samples taken directly from the rectum of all calves from Day −7, to day 56 for FEC. Animal behaviour was continuously monitored through the Monitoring Neck Tag.
Throughout the experiment, the animals were under the daily care of experienced farm staff and assessed via a veterinary professional. The protocol included a condition that any animal showing clinical signs of parasitism (diarrhoea, lethargy, weight loss) would be removed from the study for individual veterinary treatment and at which point all infected animals would be treated with AHC and the trial would cease.
Faecal egg counts
Faecal sample pottles were transported immediately following collection to the laboratory (Department of Agricultural Sciences, Lincoln University). On arrival, samples were mixed with a small amount of water and left to soak overnight at 5°C before being suspended in a saturated salt solution and the FEC determined from duplicate samples using a modified McMaster technique with a sensitivity of 25 eggs per gram (Ministry of Agriculture Citation1986).
Behaviour data extraction and handling
For each minute of every day, the accelerometer-based collar used a proprietary algorithm to allocate activity to one of seven mutually exclusive categories: ruminating, eating, low, moderate and high activity levels, heavy breathing and other (Bar et al. Citation2019). The collected data were sent wirelessly to a base station for the duration of the experiment and extracted into a. csv file for further statistical analysis.
The accuracy of behavioural classification using this technology has been estimated at around 90% in adult cattle (Schirmann et al. Citation2009; Stygar et al. Citation2021; Chang et al. Citation2022) although the system has not been validated in animals < 6 months of age. This was an off-label use of the technology as the proprietary algorithms are optimised for mature dairy cattle.
Statistical analysis
All analysis was carried out using the statistical programme R v4.2.1 (R Development Core Team; R Foundation for Statistical Computing, Vienna, Austria). The dependent variables (LW, FEC and the minutes of activity per day for each behaviour category) were analysed using Bayesian multivariable mixed models that accounted for the clustering of observations within the dataset and the autocorrelation between repeated measures using the brms package (Bürkner Citation2017). Parameter estimates were expressed in terms of the median and the 95% highest density interval (HDI) of the parameter’s probability distribution (Kruschke Citation2018; Makowski et al. Citation2019). All models were run with four Monte Carlo Markov chains and a total sample size of 16,000, of which 4,000 iterations were used for warm-up and models were assessed in terms of their widely applicable information criterion (WAIC) (Watanabe Citation2010).
Full details of variable selection, model construction and assessment and pre-study power calculations are given in Supplementary Information 1.
Results
After the initial enrolment and allocation to the treatment groups, complete records of behaviour were retrieved for 30/36 calves. The behavioural reporting was completely missing from four calves and had missing values for two calves (data missing from > 50% of readings). These animals were withdrawn from the analysis of behaviour, LW and FEC. Of the 30 animals with complete records, 13 had been allocated to the infected group and 17 to the uninfected group. Subsequently, 7 of the infected and 9 of the uninfected animals were allocated for AHC 7 weeks after the initial infection.
Variable selection
At enrolment the mean body weight was 142.3 (SD 17.7) kg and the mean age was 166 (SD 8.5) days for the 30 calves with complete records. There was no evidence for confounding by weight or age at enrolment with a median difference in weight of −0.8 (95% HDI = −9.8 to 8.1) kg and 1 (95% HDI = −4 to 6) days between infected and control calves. Although both estimated HDI exceeded a margin of ± 5 kg and ± 5 days, respectively, both were centred near the null value. The estimated effect size for weight and age were both small, although with a wide 95% HDI reflecting the small sample size (effect size for weight at enrolment 0.05 (95% HDI = −0.53 to 0.64); for age at enrolment 0.12 (95% HDI = −0.73 to 0.51)). Inclusion of initial weight or age did not improve the WAIC of the models over the period of the study.
Neither soil moisture nor evapotranspiration improved the fit of the models when individually added to the model and so both were excluded. Similarly model fit was not improved by including rainfall or sunshine hours either individually or together and so these variables were excluded, leaving infection status and AHC treatment as the only calf level variables in the models (see Supplementary Information 1 and 2).
FEC during the study
Faecal egg counts were highly dispersed during the study: for the 30 calves with complete records, the range of FEC was 0–2,700 epg with 243/300 (81%) samples having a FEC of 0 epg. The distribution of FEC for infected and uninfected calves that were not treated with AHC during the trial period is shown in .
Figure 2. Weekly faecal egg counts in 30 calves that were fitted with real-time animal behaviour monitors and half of which (black circles) were infected with Ostertagia ostertagi and Cooperia oncophera at three weekly intervals (dashed arrows) and half (grey circles) were left uninfected.
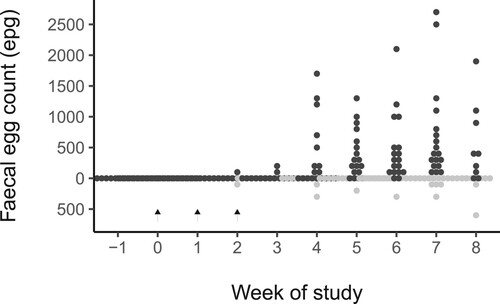
The negative binomial model for FEC predicted that there was no difference between the FEC for infected vs. uninfected calves for Weeks −2 up to and including Week 3. Thereafter, FEC was predicted to be greater for infected calves, with the 95% most likely values for this difference excluding zero for every subsequent week. The greatest predicted difference in FEC was at Week 7 (917 (95% HDI = 0–2,500) epg). A similar effect was seen when looking at the percentage of counts > 50 epg and > 200 epg. For Weeks −2 up to and including week 3, there was no difference between infected and uninfected calves in the percentage of FEC over these thresholds (difference entirely within ± 2.5%). Thereafter, a higher percentage of counts exceeding either threshold was seen in the infected calves with the difference peaking at Week 7 when 71 (95% HDI = 50–90)% and 61 (95% HDI = 35–82)% more samples from infected than uninfected calves, exceeded the threshold of > 50 epg and > 200 epg. After treatment with AHC, for all calves the FEC = 0 and AHC status was not included in the final model predicting FEC.
Live weight during the study
There were 330 individual weights recorded for the 30 calves with an initial mean weight at recruitment of 142.3 (SD 17.70) kg. The model predicted that there was no difference in the weight at the end of the study between infected and control calves, with a mean weight for infected calves at the last weigh visit of 186.6 (95% HDI = 150.71–221.81) kg and for control calves 187.1 (95% HDI = 151.17–221.85) kg. The most likely predicted difference was again small but the 95% HDI exceeded ± 5 kg (0.57 (95% HDI = −6.11 to 7.23) kg). Data was only available for one weigh session after AHC treatment, and the model predicted no effect for AHC treatment on liveweight. AHC status was not included in the final model predicting LW.
Behaviour during the study
The SD of behavioural data from the first week of recording was approximately twice that for every subsequent week of recording and behavioural data from the first week was excluded from the analysis as it was assumed to represent variation reflecting the creation of a new group. Over the remaining 9 weeks of recording, this left 1,890 recordings of the minutes spent per behaviour per day for each calf. The mean time spent eating was 550 (SD 70) minutes/day, for ruminating was 493 (SD 65) minutes/day, for moderate or high levels of activity was 149 (SD 46) minutes/day and for low levels of activity was 249 (SD 67) minutes/day. Infection, AHC status and their interaction were associated with behaviour and were included within the final model.
The minutes spent per day ruminating, eating, moderately or highly active, and at low levels of activity as predicted by the model for infected and uninfected calves that were not treated with AHC (Weeks −1 to 8) is shown in Supplementary Figure 1, and for calves that were treated with AHC (Week 8) in Supplementary Figure 2.
After adjusting for the effects of calf and time, the model predicted differences in behaviour based on both infection and treatment status and their interaction, suggesting that the effect of AHC varied by infection status and the effect of infection differed between untreated and treated calves.
The difference in minutes per day spent in each behaviour due to infection (infected – control) on calves that were untreated (Weeks −1 to 8) and on calves that were treated (Week 8) is shown in . Correspondingly, the difference in minutes per day spent in each behaviour due to AHC treatment (AHC treatment – no treatment) on the behaviour of infected and uninfected calves over the last week of the study (Week 8) is shown in .
Figure 3. Predictions (solid line represents most likely value; shaded area represents the 95% highest density interval) from a multinomial Bayesian mixed model of the effect of infection with Ostertagia ostertagi and Cooperia oncophera at Day 0, 7 and 14 (vertical dashed arrows) on the minutes per day spent eating (a), ruminating (b), moderately or highly active (c) or at low levels of activity (d) in dairy calves that had not been treated with anthelmintic (light grey shading) and that had been treated with anthelmintic (dark grey shading) at Day 49 (solid black arrow).
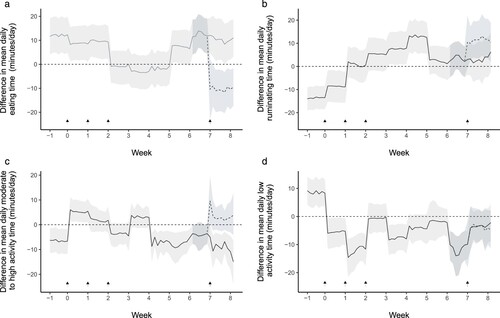
Figure 4. Predictions (solid line represents most likely value; shaded area represents the 95% highest density interval) from a multinomial Bayesian mixed model of the effect of treatment with anthelmintic at Day 49 (black arrow) on the minutes per day spent eating (a), ruminating (b), moderately or highly active (c) or at low levels of activity (d) for dairy calves that had been infected orally with Ostertagia ostertagi and Cooperia oncophera (dark grey shading) or had not been infected (light grey shading).
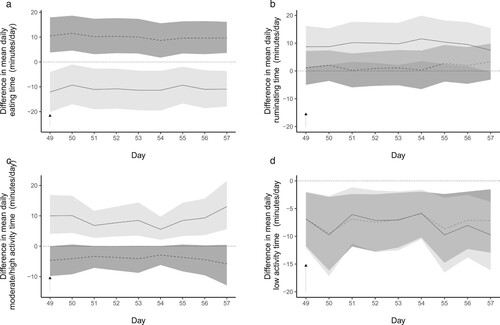
Eating time
The response in eating time to infection varied during the period of follow up and with whether the calves had been treated with AHC. In calves untreated with AHC, before infection, calves allocated to the infection group spent 9 (95% HDI = 2–16) minutes more per day eating than uninfected calves. Adjusting for this difference, the model predicted that infected calves continued to eat more than uninfected calves during the period of administration of the L3 larvae. However, after the last dose of larvae (end of Week 2) eating time decreased for infected calves so that there was effectively no difference in eating behaviour between infected and uninfected calves for 3 weeks (mean difference −2 (95% HDI = −9 to 4) minutes/day). Eating time then increased for infected calves so that 8 weeks after infection, infected calves were eating 10 (95% HDI = 2–19) minutes more per day than uninfected calves. However, in calves treated with AHC, infection decreased the time spent eating (mean difference −11 (95% HDI = −3 to −18) minutes/day).
The model predicted that the effect of AHC on eating time is not the same in calves that have been infected as in uninfected calves ((a)). In uninfected calves, AHC treatment increased the mean time spent eating in Week 8 (mean increase 10 (95% HDI = 3–17) minutes/day) but decreased the mean time spent eating for infected calves (mean decrease −11 (95% HDI = −3 to −19) minutes/day). This meant that AHC treated and infected calves spent a mean of 21 (95% HDI 11–31) minutes/day less time eating compared to AHC treated and uninfected calves.
Rumination time
In calves that were untreated with AHC, infection appeared to increase rumination time with the difference between infected and uninfected calves changing over the study. Before infection, calves allocated to the infection group were ruminating 9 (95% HDI = 2–15) minutes less per day than uninfected calves. Adjusting for this difference, the model predicted that ruminating time progressively increased for infected calves over Weeks 3 and 4, with infected calves ruminating 8 (95% HDI = 1–15) minutes/day and 13 (95% HDI = 7–20) minutes/day, respectively, more than uninfected calves. For the last 3 weeks of the study, although it was more likely that infected calves continued to ruminate more than uninfected calves, the probability that this difference was > 0 was < 95% (mean probability 66%) with infected calves ruminating a mean of 3 (95% HDI = −4 to 10) minutes more per day than uninfected calves. However, if the calves had been treated with AHC, the effect of infection was a more pronounced increase in rumination time (mean difference 11 (95% HDI = 5–18) minutes/day).
The model again predicts that the effect of AHC on ruminating time is not the same for infected calves as uninfected calves, although the certainty that this difference is > greater than 0 is < 95% ((b)).
In uninfected calves, AHC treatment made virtually no difference to the time spent ruminating, with a mean increase after AHC treatment of 2 (95% HDI = −5 to 8) minutes/day. For infected calves, AHC treatment increased mean ruminating time (mean increase 10 (95% HDI = 2–18) minutes/day). This meant that after AHC treatment, infected calves spent a mean of 8 (95% HDI −2 to 11) minutes/day more ruminating compared to AHC treated and uninfected calves.
Time spent moderately or highly active
In calves that were untreated with AHC, infection appeared to cause a short-term increase in activity over the period of infection. Before infection, calves allocated to the treatment group were moderately or highly active 7 (95% HDI = 1–10) minutes less per day than uninfected calves. Adjusting for this difference, the model predicted a short-term increase in activity in infected calves over the period when worm larvae were being administered (week 1, infected calves were active for 5 (95% HDI = 2–10) minutes/day more than uninfected calves). However, after this (Week 2 onwards), activity in infected calves decreased and there was effectively no difference in activity between infected and uninfected calves for Weeks 2–4 when the mean difference was 1 (95% HDI = −4 to 5) minute/day. For the last 4 weeks of the study, it was more likely that infected calves continued to be less active than uninfected calves, with a mean difference of 8 (95% HDI = 2–12) minutes less per day active for infected calves compared to uninfected. However, in calves treated with AHC, infection increased the time spent moderately/highly active (mean difference 4 (95% HDI = −2 to 12) minutes/day).
The model predicts differences in the response to AHC in calves that are infected compared to those that are not. In uninfected calves, AHC treatment reduced the active time, with a mean decrease in activity after AHC treatment of −4 (95% HDI = −1 to −9) minutes/day. For infected calves, activity increased after AHC treatment (mean increase 8 (95% HDI = 3–16) minutes/day). Overall, after AHC treatment, infected calves spent a mean of 12 (95% HDI = 4–23) minutes/day more in activity compared to AHC treated and uninfected calves ((c)).
Time spent at low levels of activity
In calves that were untreated with AHC, infection was associated with a reduction compared to uninfected calves in the time spent at low levels of activity. Before infection, calves allocated to the treatment group showed low levels of activity for 9 (95% HDI = 4–12) minutes more per day than calves allocated to the uninfected group. Adjusting for this difference, the model predicted that infection reduced the time spent at low levels of activity and this reduction persisted throughout the period of follow up with a mean difference after infection of 6 (95% HDI = 1–11) minutes less per day at low levels of activity for infected calves compared to uninfected calves. In calves treated with AHC, infection also decreased the time spent in low levels of activity (mean difference = −7 (95% HDI = −10 to 3) minutes/day).
For the time spent in low level activity, the model predicts that AHC treatment leads to less time spent at low levels of activity in both infected and uninfected calves. For uninfected calves, the mean decrease was −7 (95% HDI = −2 to −14) minutes/day and for infected calves, there was a mean decrease of −8 (95% HDI = −2 to −15) minutes/day. There was no meaningful difference in this effect of AHC on the time spent at low levels of activity, with infected calves spending 0 (95% HDI = −9 to 7) minutes/day less at low levels of activity after AHC treatment than uninfected calves that had been treated with AHC.
A summary of the effects in Week 8 of infection and AHC and demonstration of their mutual interaction is given in Supplementary Figure 3.
Correlation of behaviours
The intraclass correlation coefficient for all four behavioural variables within each of the model’s grouping variables were consistently low (median 6 (min 1, max 22)%) indicating little clustering of behaviour within the model’s groups (Supplementary Table 1). Correlations between the non-referent behaviours amongst calves were included within the final model as this resulted in a WAIC closer to zero (Vehtari et al. Citation2017). Calves that spent more time eating were also more likely to be calves that ruminated more (correlation coefficient 0.8 (95% HDI = 0.61–0.92) but calves that were moderately to highly active were only moderately more likely to ruminate or eat more (correlation coefficient 0.5 (95% HDI = 0.19–0.73) and 0.3 (95% HDI = −0.06 to 0.30), respectively). Similarly, for days when eating was more likely, ruminating was also more likely (correlation coefficient 0.9 (95% HDI = 0.74–0.91)), but there was only a low level of correlation between eating or rumination and moderate to high levels of activity (correlation coefficient 0.2 (95% HDI = −0.09 to 0.40) and 0.2 (95% HDI = −0.08 to 0.44), respectively).
The correlation for behaviours between the weeks of the study and for behaviours between named days of the week were low, with point values ranging between −0.2 to 0.3 and in all cases centred within a 95% HDI spanning the null value. This suggests that even though the precision of the estimate was low, the most likely value indicated only low levels of correlation.
Variability in behaviours
The effect of infection on the variability in behaviour as expressed by the coefficient of dispersion (COD) was small and variable both between behaviours and with the AHC status of the calves, with the greatest change seen in activity, where infection increased the median COD by 3 (95% CI = 2.9–3.1)%.
Correspondingly, treatment with AHC resulted in small increases in the median COD of all behaviours, with the population 95% CI of the median difference in COD between animals treated with AHC and those not treated with AHC excluding zero in both infected and uninfected calves and for all behaviours (). However, only for eating behaviour did the increase in the dispersion of the data following AHC treatment exceed 4% in both infected (median increase in COD = 4 (95% CI = 0.39–4.3)%) and uninfected calves (median increase in COD = 4 (95% CI = 4.2–4.4)%).
Figure 5. The effect of anthelmintic (AHC) in uninfected calves (a), the effect of AHC in infected calves (b), the effect of infection in calves without AHC (c) and the effect of infection in calves with AHC (d) on the coefficient of dispersion for the time/day spent eating, ruminating, moderately to highly active and at low levels of activity of dairy calves. Error bars mark the 95% CI for the estimated population median. Infected calves were treated with three oral doses of Ostertagia ostertagi and Cooperia oncophera larvae at Day 0, 7 and 14. AHC treatment was randomly assigned to 7/13 infected and 9/17 uninfected calves at Day 49.
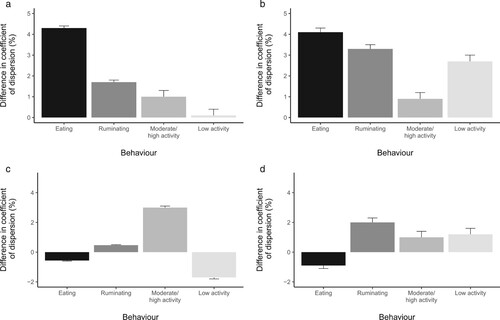
Discussion
This study quantifies the effect of experimental challenge with O. ostertagi and C. oncophera L3 larvae and AHC treatment on the time calves at pasture spent eating, ruminating, at low levels of activity and at moderate/high levels of activity. Our results suggest there are small but measurable differences in behaviour as a result of worm challenge and AHC treatment. Changes in behaviour from AHC treatment depend on the infection status of the calves and may be larger than behavioural changes resulting from infection.
Based on the clear difference in FEC between the groups, we believe the infectious challenge used in this study was successful in establishing a patent infection in the infected group. Towards the end of the study a few control calves had a low FEC, but a sizeable difference in the FEC remained between the groups. No cattle or sheep had been grazed on the pasture, and although it is possible that a small number of larvae were present at the outset, both control and infected groups would have been equally likely to ingest these larvae so producing equivalent levels of natural infection.
Despite FEC in the infected group similar to those associated with a reduction in LWG in previous studies (Merlin et al. Citation2017; Bates et al. Citation2022; Shephard et al. Citation2022), after 8 weeks of follow-up there was no impact of parasitism on LWG in this study, and the LWG in this trial was comparable to the target weight gain for New Zealand heifer replacements (Roche et al. Citation2015). This may reflect a level of nutrition sufficient to overcome the impact of parasitism on LW (Gibson Citation1963; Neves et al. Citation2020). The pathology associated with GIP infection may be attributed to mucosal damage from late larval and adult worms (Fox Citation1997). It is thought to occur within a few days of exposure to infective larvae (Murray et al. Citation1970; Simpson Citation2000) but its progression depends on a complex and evolving interaction between host and parasite (Fox Citation1997; Claerebout and Vercruysse Citation2000). In the absence of post-mortem or serological data on gastrointestinal pathology, we cannot be confident there was a pathological impact on the animal from the patent infection. It had been intended to measure IgA specific to O. ostertagi in serum to demonstrate the extent of the immune response to infection. However, following the outbreak of Mycoplasma bovis in New Zealand in 2017, restrictions placed on the Lincoln University Dairy herd prevented retention and testing serum from animals sharing the same herd identifier which, unfortunately, meant that no samples from the trial calves could be assessed for IgA.
The lack of a difference in LWG suggests that intake was either similar between groups, or that infected animals were able to compensate energy partitioning in some way. A reduction in feed intake is normally associated with GIP and has been identified as part of the host’s homeostatic response to infection (Kyriazakis et al. Citation1998). In a study using first season grazing calves with a similar level of O. ostertagi challenge to the present study, Berk et al. (Citation2016) reported a maximum reduction in intake 25 days after challenge. However, the correlation between grazing time and feed intake is relatively weak (Beauchemin Citation2018; Edwards et al. Citation2024) and although the calves in the current study initially reduced eating time for 3 weeks after infection which then increased to their baseline eating time ((a)), the lack of validation of the sensors in animals < 6 months of age and visual confirmation of the behavioural categorisation mean that this may not have been accompanied by a reduction in intake. Given the lack of LWG difference, if intake reduction occurred, it was probably short-lived. Anorexia in response to parasitism is not seen in partially immune or resistant animals (Kyriazakis et al. Citation1994) but given the relatively slow rate of development of immunity to Ostertagia spp. infection in cattle (Grønvold et al. Citation1992) it is unlikely this would have been a factor in the present study.
Rumination is often seen as a subcategory of feeding behaviour (Fan et al. Citation2022) and might have been expected to mirror the changes in eating behaviour rather than the steady increase after GIP infection seen in the current study. Decreases in rumination have been recorded in adult cows with metritis (Stangaferro et al. Citation2016a), lameness (King et al. Citation2018) and mastitis (Stangaferro et al. Citation2016b) but in the present study the increase in parasitised calves may have been a compensatory change to the decrease in eating time or a result of a change of dietary preference in parasitised animals (Forbes Citation2021).
In calves challenged at turn out with O. ostertagi and C. oncophera L3 larvae, Högberg et al. (Citation2019) reported a decrease in motion for infected calves 46–58 days after challenge, similar to the decrease in activity seen in infected calves during the latter stages of the current study. However, for a comparable period of follow-up to the current study, Högberg et al. (Citation2019) found no difference in lying duration nor the number of lying bouts in challenged calves, whereas we observed a decrease in low activity time in infected calves. In a second study, Högberg et al. (Citation2021) reported an increase in mean daily lying time during the first 40 days after challenge in parasitised calves compared to controls and an increase in activity but only from Day 62 after exposure, a period of follow up exceeding the present study. In both these trials, LW was less (mean 589 (SD 26) g/day and mean 282 (SD 13) g/day, respectively) compared to the current study (mean 689 (SD 21) g/day), consistent with behavioural changes depending on the impact infection has – not least on LWG.
By comparison, during natural challenge in sheep, Ikurior et al. (Citation2020) reported a negative impact of parasitism on activity in heavier animals 42–46 days after first exposure, similar to the decrease in activity seen in parasitised animals 28 days after artificial challenge in the present study. There was no evidence for an interaction between weight and parasitism on behaviour within the present study and this may reflect differences caused by the greater LWG and the lack of observable difference in LWG between infected and uninfected calves.
Differences in the methodology used between studies to record and quantify movement further complicate comparisons. For example, “lying behaviour” recorded in Högberg et al. (Citation2019, Citation2021) is not directly comparable to “low activity time” in the current trial as the latter indicates time spent idle, resting or expressing limited activity, rather than whether the animal is lying down or not. Separately, Ungar et al. (Citation2018) suggested leg movements associated with the onset or termination of lying bouts may be misclassified by pedometer systems as leg movement associated with movement when compared with accelerometer systems. Further, as we have not measured factors such as bite rate and size, we cannot be certain the changes in time spent grazing are synonymous with changes in intake (González et al. Citation2018).
Previously, Szyszka and Kyriazakis (Citation2013), who used a mixture of data collected from pedometers and 24-hour video surveillance, reported no effect on any feeding behaviours from artificial challenge with much greater doses of O. ostertagi (75,000, 150,000 and 300,000 L3 respectively) but the animals with the highest level of challenge lay down less frequently but for a longer period of time and took fewer steps 34–46 days after challenge than the control group. This contrasts with a decrease in all levels of activity for the equivalent period of follow up in the present study. These authors also reported a reduction in LWG for the most heavily parasitised group and suggested the idea of a threshold of infection below which behavioural signs would not be detected.
Although 97.5% of all calves were ≥ 5 months old at enrolment and ≥ 6 months old after the last dose of parasite larvae, the lack of publicly available evidence validating the accelerometers in animals ≤ 6 months old is a limitation of the study. Further, many of the animal monitoring systems that are commercially available in New Zealand have been designed and calibrated to optimise a particular aspect of animal behaviour such as oestrus detection in adult cows and have not been validated in animals < 6 months of age. It cannot be assumed that the accuracy of behavioural classification will be as high in animals < 6 months of age, and it is possible the data we observed are proxies for some other state of behaviour within the animal and do not actually reflect differences in rumination, eating and activity levels. We believe this is unlikely given the age of the enrolled calves and even if the classification of behaviours is inaccurate it will not confound the effect of parasitism, which was our main aim.
The range in effects reported across studies reflects the complexity of behavioural responses to parasitism. Our classifications of behaviour were relatively simplistic and mutually exclusive, and this is a further limitation of the current study. The variable and dynamic parasite-animal-pasture environment and the complex drivers of animal behaviour mean that changes in behaviour from infection and AHC may differ between farms, seasons and groups and result in a complex balance of interacting, compensating and competing responses beyond the scope of this small-scale study. Burgunder et al. (Citation2018) used fractal analysis to report differences in the patterns of activity vs. inactivity between parasitised and control calves. These authors found the temporal pattern of behaviour in sheep varied with their infection status, with parasitised animals showing a less complex pattern than parasitised animals treated with AHC. In the present study, using a simpler analysis limited to examining the COD between days, differences in the variance of behaviours between infected and uninfected animals were evident, with larger differences in the variance of behaviour as the result of AHC treatment in both infected and uninfected calves.
Further examination of the effect of parasitism and AHC on the variance of behaviour is warranted. There are few studies documenting the effect of AHC treatment on calf behaviour. In a study on adult dairy cows and heifers, Forbes et al. (Citation2004) reported that in naturally parasitised animals treated with AHC, there was no effect of AHC on rumination time, but idling time decreased and grazing time increased. These authors also reported positive production, LW and condition score changes to AHC suggesting that the impact of AHC and parasitism may vary with the pathology of infection. In contrast, in our study where there was no detectable effect on LW, AHC treatment of infected calves reduced eating and low activity time and increased ruminating and moderate to high activity time.
This was a small study with a limited period of follow up which was unintentionally constrained by a disease outbreak on a neighbouring property forcing the conclusion of the trial, so the impact of AHC could only be assessed for 7 days following a single treatment. Moreover, lack of effect of infection on LWG suggests a low level of pathology and potentially a different behavioural response to when infection is associated with decreased productivity. We also used a non-replicated trial design, which is a limitation for behaviour studies where the behaviour of animals can potentially influence other animals in the same paddock (Neave et al. Citation2020). Moreover, given the lack of validation for the sensors for animals of this class and the lack of visual appraisal of behaviour in the study, there is inevitably uncertainty over whether the reported differences in behaviour reflect differences in behaviour that actually occurred. Notwithstanding this, the Bayesian approach allowed us to quantify the probability of the outcome, whether or not there was a difference between groups. All model coefficients were robust to changes in priors, suggesting, even with the small dataset, that the differences we observed were driven by the data and the models’ predictions are a reasonable representation of this dataset.
For targeted selective treatment to be effective, animals identified for treatment need to respond positively to AHC in terms of productivity and the impact of AHC treatment on the epidemiology of disease and resistance. Selective treatment by definition reduces the amount of AHC used but it does not follow that animals identified as behaving differently because of parasite infection are the ideal performance or epidemiological targets for treatment. Further work to examine changes in the pattern of behaviour in parasitised and affected and parasitised and treated animals is required to reliably identify animals for targeted selective treatment on the basis of their behaviour.
Supplemental Material
Download PDF (615.7 KB)Acknowledgements
Vetlife NZ gratefully acknowledges the co-funding of this project by the Ministry for Primary Industries' Sustainable Food and Fibre Futures Fund. Vetlife gratefully acknowledges the in-kind contribution of anthelmintic product from Boehringer Ingelheim Animal Health NZ Ltd (Manukau City, NZ), the conducting of multiple FEC by Robin McAnulty of Lincoln University, and the in-kind contribution of labour and time from farm staff at Lincoln University. None of these bodies were involved in the design or analysis of the work.
Disclosure statement
Andrew Bates is the Scientific Editor for the New Zealand Veterinary Journal, and recipient of the financial award from the New Zealand Ministry for Primary Industries that supported this project.
Correction Statement
This article was originally published with errors, which have now been corrected in the online version. Please see Correction (https://doi.org/10.1080/00480169.2024.2362959).
References
- Bar D, Kaim M, Flamenbaum I, Hanochi B, Toaff-Rosenstein RL. Technical note: accelerometer-based recording of heavy breathing in lactating and dry cows as an automated measure of heat load. Journal of Dairy Science 102, 3480–6, 2019. https://doi.org/10.3168/jds.2018-15186
- Bates A, Wells M, Laven RA, Simpson M. Reduction in morbidity and mortality of dairy calves from an injectable trace mineral supplement. Veterinary Record 184, 680, 2019. https://doi.org/10.1136/vr.105082
- Bates AJ, Greer A, McAnulty R, Jackson A. Targeted selective treatment with anthelmintic for New Zealand dairy heifers. Veterinary Parasitology 309, 109757, 2022. https://doi.org/10.1016/j.vetpar.2022.109757
- Beauchemin KA. Current perspectives on eating and rumination activity in dairy cows. Journal of Dairy Science 101, 4762–84, 2018. https://doi.org/10.3168/jds.2017-13706
- Berk Z, Bishop SC, Forbes AB, Kyriazakis I. A simulation model to investigate interactions between first season grazing calves and Ostertagia ostertagi. Veterinary Parasitology 226, 198–209, 2016. https://doi.org/10.1016/j.vetpar.2016.05.001
- Burgunder J, Petrželková KJ, Modrý D, Kato A, MacIntosh AJJ. Fractal measures in activity patterns: do gastrointestinal parasites affect the complexity of sheep behaviour? Applied Animal Behaviour Science 205, 44–53, 2018. https://doi.org/10.1016/j.applanim.2018.05.014
- Bürkner P-C. brms: An R package for Bayesian multilevel models using Stan. Journal of Statistical Software 80, 1–28, 2017. https://doi.org/10.18637/jss.v080.i01
- Chang AZ, Fogarty ES, Moraes LE, García-Guerra A, Swain DL, Trotter MG. Detection of rumination in cattle using an accelerometer ear-tag: a comparison of analytical methods and individual animal and generic models. Computers and Electronics in Agriculture 192, 106595, 2022. https://doi.org/10.1016/j.compag.2021.106595
- Claerebout E, Vercruysse J. The immune response and the evaluation of acquired immunity against gastrointestinal nematodes in cattle: a review. Parasitology 120, 25–42, 2000. https://doi.org/10.1017/S0031182099005776
- Cuttance E, Mason W, McDermott J, Laven R, McDougall S, Phyn C. Calf and replacement heifer mortality from birth until weaning in pasture-based dairy herds in New Zealand. Journal of Dairy Science 100, 8347–57, 2017. https://doi.org/10.3168/jds.2017-12793
- Edwards JP, Qasim M, Bryant RH, Thomas C, Wright-Watson C, Zobel G, Neal MB, Eastwood CR. On-animal sensors may predict paddock level pasture mass in rotationally grazed dairy systems. Computers and Electronics in Agriculture 219, 108779, 2024. https://doi.org/10.1016/j.compag.2024.108779
- Eysker H, Ploeger M. Value of present diagnostic methods for gastrointestinal nematode infections in ruminants. Parasitology 120, S109–S119, 2000. https://doi.org/10.1017/S0031182099005752
- Fan B, Bryant R, Greer A. Behavioral fingerprinting: acceleration sensors for identifying changes in livestock health. J 5, 435–54, 2022. https://doi.org/10.3390/j5040030
- Fan B, Bryant RH, Greer AW. Automatically identifying sickness behavior in grazing lambs with an acceleration sensor. Animals 13, 2086, 2023. https://doi.org/10.3390/ani13132086
- Forbes A. Ruminant behaviour in subclinical parasitic gastroenteritis. Livestock 26, 78–85, 2021. https://doi.org/10.12968/live.2021.26.2.78
- Forbes AB, Huckle CA, Gibb MJ. Impact of eprinomectin on grazing behaviour and performance in dairy cattle with sub-clinical gastrointestinal nematode infections under continuous stocking management. Veterinary Parasitology 125, 353–64, 2004. https://doi.org/10.1016/j.vetpar.2004.07.025
- Forbes AB, Huckle CA, Gibb MJ. Evaluation of the effect of eprinomectin in young dairy heifers sub-clinically infected with gastrointestinal nematodes on grazing behaviour and diet selection. Veterinary Parasitology 150, 321–32, 2007. https://doi.org/10.1016/j.vetpar.2007.09.031
- Fox M. Pathophysiology of infection with gastrointestinal nematodes in domestic ruminants: recent developments. Veterinary Parasitology 72, 285–308, 1997. https://doi.org/10.1016/S0304-4017(97)00102-7
- Gibson TE. The influence of nutrition on the relationships between gastro–intestinal parasites and their hosts. Proceedings of the Nutrition Society 22, 15–20, 1963. https://doi.org/10.1079/PNS19630006
- González LA, Tolkamp BJ, Coffey MP, Ferret A, Kyriazakis I. Changes in feeding behavior as possible indicators for the automatic monitoring of health disorders in dairy cows. Journal of Dairy Science 91, 1017–28, 2008. https://doi.org/10.3168/jds.2007-0530
- González LA, Bishop-Hurley GJ, Handcock RN, Crossman C. Behavioral classification of data from collars containing motion sensors in grazing cattle. Computers and Electronics in Agriculture 110, 91–102, 2015. https://doi.org/10.1016/j.compag.2014.10.018
- González LA, Kyriazakis I, Tedeschi LO. Precision nutrition of ruminants: approaches, challenges and potential gains. Animal 12, s246–s261, 2018. https://doi.org/10.1017/S1751731118002288
- Grønvold J, Nansen P, Gasbarre LC, Christensen CM, Larsen M, Monrad J, Midtgaard N. Development of immunity to Ostertagia ostertagi (Trichostrongylidae: Nematoda) in pastured young cattle. Acta Veterinaria Scandinavica 33, 305–16, 1992. https://doi.org/10.1186/BF03547296
- Högberg N, Lidfors L, Hessle A, Arvidsson Segerkvist K, Herlin A, Höglund J. Effects of nematode parasitism on activity patterns in first-season grazing cattle. Veterinary Parasitology 276, 100011, 2019. https://doi.org/10.1016/j.vpoa.2019.100011
- Högberg N, Hessle A, Lidfors L, Baltrušis P, Claerebout E, Höglund J. Subclinical nematode parasitism affects activity and rumination patterns in first-season grazing cattle. Animal 15, 100237, 2021. https://doi.org/10.1016/j.animal.2021.100237
- Höglund J, Morrison DA, Charlier J, Dimander S-O, Larsson A. Assessing the feasibility of targeted selective treatments for gastrointestinal nematodes in first-season grazing cattle based on mid-season daily weight gains. Veterinary Parasitology 164, 80–8, 2009. https://doi.org/10.1016/j.vetpar.2009.04.016
- Höglund J, Dahlström F, Sollenberg S, Hessle A. Weight gain-based targeted selective treatments (TST) of gastrointestinal nematodes in first-season grazing cattle. Veterinary Parasitology 196, 358–65, 2013. https://doi.org/10.1016/j.vetpar.2013.03.028
- Hutchings MR, Gordon IJ, Robertson E, Kyriazakis I, Jackson F. Effects of parasitic status and level of feeding motivation on the diet selected by sheep grazing grass/clover swards. Journal of Agricultural Science 135, 65–75, 2000. https://doi.org/10.1017/S002185969900790X
- Ikurior SJ, Pomroy WE, Scott I, Corner-Thomas R, Marquetoux N, Leu ST. Gastrointestinal nematode infection affects overall activity in young sheep monitored with tri-axial accelerometers. Veterinary Parasitology 283, 109188, 2020. https://doi.org/10.1016/j.vetpar.2020.109188
- Jackson A, Ellis K, McGoldrick J, Forbes A, Jonsson N, Stear MJ. Targeted anthelmintic treatment of parasitic gastroenteritis in first grazing season dairy calves using daily live weight gain as an indicator. Veterinary Parasitology 244, 85–90, 2017. https://doi.org/10.1016/j.vetpar.2017.07.023
- Kenyon F, Greer AW, Coles GC, Cringoli G, Papadopoulos E, Cabaret J, Berrag B, Varady M, van Wyk JA, Thomas E, et al. The role of targeted selective treatments in the development of refugia-based approaches to the control of gastrointestinal nematodes of small ruminants. Veterinary Parasitology 164, 3–11, 2009. https://doi.org/10.1016/j.vetpar.2009.04.015
- King MTM, LeBlanc SJ, Pajor EA, Wright TC, DeVries TJ. Behavior and productivity of cows milked in automated systems before diagnosis of health disorders in early lactation. Journal of Dairy Science 101, 4343–56, 2018. https://doi.org/10.3168/jds.2017-13686
- Kruschke JK. Rejecting or accepting parameter values in Bayesian estimation. Advances in Methods and Practices in Psychological Science 1, 270, 2018. https://doi.org/10.1177/2515245918771304
- Kyriazakis I, Oldham JD, Coop RL, Jackson F. The effect of subclinical intestinal nematode infection on the diet selection of growing sheep. British Journal of Nutrition 72, 665–77, 1994. https://doi.org/10.1079/BJN19940070
- Kyriazakis I, Tolkamp B.J., Hutchings MR. Towards a functional explanation for the occurrence of anorexia during parasitic infections. Animal Behaviour 56, 265–74, 1998. https://doi.org/10.1006/anbe.1998.0761
- Makowski D, Ben-Shachar M, Lüdecke D. Bayestestr: describing effects and their uncertainty, existence and significance within the Bayesian framework. Journal of Open Source Software 4, 1541, 2019. https://doi.org/10.21105/joss.01541
- Mason P, McKay C. Field studies investigating anthelmintic resistance in young cattle on five farms in New Zealand. New Zealand Veterinary Journal 54, 318–22, 2006. https://doi.org/10.1080/00480169.2006.36717
- McDougall S. Effect of calf age on bovine viral diarrhea virus tests. Journal of Veterinary Diagnostic Investigation 33, 528–37, 2021. https://doi.org/10.1177/1040638721998821
- Merlin A, Chauvin A, Lehebel A, Brisseau N, Froger S, Bareille N, Chartier C. End-season daily weight gains as rationale for targeted selective treatment against gastrointestinal nematodes in highly exposed first-grazing season cattle. Preventive Veterinary Medicine 138, 104–12, 2017. https://doi.org/10.1016/j.prevetmed.2017.01.011
- *Ministry of Agriculture. Manual of Veterinary Parasitological Laboratory Techniques. His Majesty’s Stationery Office, London, UK, 1986
- Murray M, Jennings F, Armour J. Bovine ostertagiasis: structure, function and mode of differentiation of the bovine gastric mucosa and kinetics of the worm loss. Research in Veterinary Science 11, 417–34, 1970. https://doi.org/10.1016/S0034-5288(18)34269-3
- Neave HW, Costa JHC, Weary DM, von Keyserlingk MAG. Long-term consistency of personality traits of cattle. Royal Society of Open Science 7, 191849, 2020. https://doi.org/10.1590/s1984-29612020006
- Neves JH, Carvalho N, Amarante AFT. Gastrointestinal nematode infections do not hinder the development of Simmental X Nellore crossbred calves raised with a nutritionally enhanced diet. Revista Brasileira de Parasitologia Veterinária 29, e015819, 2020. https://doi.org/10.1590/s1984-29612020006
- O’Shaughnessy J, Earley B, Mee JF, Doherty ML, Crosson P, Barrett D, de Waal T. Controlling nematodes in dairy calves using targeted selective treatments. Veterinary Parasitology 209, 221–8, 2015. https://doi.org/10.1016/j.vetpar.2015.02.024
- Ploeger HW, Borgsteede FHM, Sol J, Mirck MH, Huyben MWC, Kooyman FNJ, Eysker M. Cross-sectional serological survey on gastrointestinal and lung nematode infections in first and second-year replacement stock in the Netherlands: relation with management practices and use of anthelmintics. Veterinary Parasitology 90, 285–304, 2000. https://doi.org/10.1016/S0304-4017(00)00246-6
- Roche JR, Dennis NA, Macdonald KA, Phyn CVC, Amer PR, White RR, Drackley JK. Growth targets and rearing strategies for replacement heifers in pasture-based systems: a review. Animal Production Science 55, 902–15, 2015. https://doi.org/10.1071/AN14880
- Schirmann K, von Keyserlingk MAG, Weary DM, Veira DM, Heuwieser W. Validation of a system for monitoring rumination in dairy cows. Journal of Dairy Science 92, 6052–5, 2009. https://doi.org/10.3168/jds.2009-2361
- Shephard RW, Hancock AS, Playford M, Oswin S. A systematic review and meta-analysis of impact of strongyle parasitism on growth rates in young cattle. Veterinary Parasitology 309, 109760, 2022. https://doi.org/10.1016/j.vetpar.2022.109760
- Simpson H. Pathophysiology of abomasal parasitism: is the host or parasite responsible? The Veterinary Journal 160, 177–91, 2000. https://doi.org/10.1053/tvjl.2000.0491
- Stangaferro ML, Wijma R, Caixeta LS, Al-Abri MA, Giordano JO. Use of rumination and activity monitoring for the identification of dairy cows with health disorders: part III. Metritis. Journal of Dairy Science 99, 7422–33, 2016a. https://doi.org/10.3168/jds.2016-11352
- Stangaferro ML, Wijma R, Caixeta LS, Al-Abri MA, Giordano JO. Use of rumination and activity monitoring for the identification of dairy cows with health disorders: part II. Mastitis. Journal of Dairy Science 99, 7411–21, 2016b. https://doi.org/10.3168/jds.2016-10908
- Stygar AH, Gómez Y, Berteselli GV, Dalla Costa E, Canali E, Niemi JK, Llonch P, Pastell M. A systematic review on commercially available and validated sensor technologies for welfare assessment of dairy cattle. Frontiers in Veterinary Science 8, 634338, 2021. https://doi.org/10.3389/fvets.2021.634338
- Sutherland I, Leathwick D. Anthelmintic resistance in nematode parasites of cattle: a global issue? Trends in Parasitology 27, 176–81, 2011. https://doi.org/10.1016/j.pt.2010.11.008
- Szyszka O, Kyriazakis I. What is the relationship between level of infection and “sickness behaviour” in cattle? Applied Animal Behaviour Science 147, 1–10, 2013. https://doi.org/10.1016/j.applanim.2013.05.007
- Ungar ED, Nevo Y, Baram H, Arieli A. Evaluation of the IceTag leg sensor and its derivative models to predict behaviour, using beef cattle on rangeland. Journal of Neuroscience Methods 300, 127–37, 2018. https://doi.org/10.1016/j.jneumeth.2017.06.001
- van Wyk JA, Hoste H, Kaplan RM, Besier RB. Targeted selective treatment for worm management – how do we sell rational programs to farmers? Veterinary Parasitology 139, 336–46, 2006. https://doi.org/10.1016/j.vetpar.2006.04.023
- Vehtari A, Gelman A, Gabry J. Practical Bayesian model evaluation using leave-one-out cross-validation and WAIC. Statistical Computing 27, 1413–32, 2017. https://doi.org/10.1007/s11222-016-9696-4
- *VICH. Efficacy of Anthelmintics: Specific Recommendations for Ovines. Veterinary International Cooperation on Harmonisation, Brussels, Belgium, 1999
- Ward C. Mathematical models to assess strategies for the control of gastrointestinal roundworms in cattle: 1. Construction. Veterinary Parasitology 138, 227–37, 2006. https://doi.org/10.1016/j.vetpar.2006.01.054
- Watanabe S. Asymptotic equivalence of Bayes cross validation and widely applicable information criterion in singular learning theory. Journal of Machine Learning Research 11, 3571–94, 2010
- Yoshihara Y, Saiga C, Tamura T, Kinugasa T. Relationships between sheep nematode infection, nutrition, and grazing behavior on improved and semi-natural pastures. Veterinary Animal Science 19, 100278, 2022. https://doi.org/10.1016/j.vas.2022.100278
- *Non-peer-reviewed