ABSTRACT
Archaeological data is always incomplete, frequently unreliable, often replete with unknown unknowns, but we nevertheless make the best of what we have and use it to build our theories and extrapolations about past events. Is there any reason to think that digital data alter this already complicated relationship with archaeological data? How does the shift to an infinitely more flexible, fluid digital medium change the character of our data and our use of it? The introduction of Big Data is frequently said to herald a new epistemological paradigm, but what are the implications of this for archaeology? As we are increasingly subject to algorithmic agency, how can we best manage this new data regime? This paper seeks to unpick the nature of digital data and its use within a Big Data environment as a prerequisite to rational and appropriate digital data analysis in archaeology, and proposes a means towards developing a more reflexive, contextual approach to Big Data.
Introduction
We are increasingly accustomed to technologically derived views of the world and beyond which would be entirely unknown to anyone living barely a generation ago: from images taken by rovers on Mars to those from landers on asteroids. Similar technologically privileged views constructed from satellite imagery, drone photography, laser scanners and the like are becoming increasingly fundamental to archaeological fieldwork and interpretation. However, this paper seeks to turn this technological gaze inwards and consider the nature of the data we capture through these and other devices, and the implications for archaeological practice. This has become even more important with the rise of Big Data. Characterized as a revolution, a new gold rush, and a new scientific paradigm, archaeologists are increasingly caught up in this whirlwind of opportunity and challenge. On the one hand enthusiastically embraced as transformative, on the other as a socio-technical imaginary predicated on a belief in the pre-eminence of large datasets, archaeology has seen relatively limited enquiry into the phenomenon of Big Data, especially when compared with the growing number of large-scale synthetic analyses undertaken within archaeology under the auspices of Big Data. What are the implications for archaeology of the interrelated concepts of datafication (an emphasis on quantification and automated data generation), dataism (a belief in the accuracy, completeness, and reliability of data), and data centrism (trust in data solutionism and the objectivity of its outcomes), which characterize Big Data in the wider world?
A New Data Paradigm?
In 2012 an article for the New York Times welcomed us to the “Age of Big Data,” a time of data abundance, data-driven prediction and discovery, and the associated development of tools for decision-making (Lohr Citation2012). The same year Forbes Magazine described Big Data as a revolution in our economic and cultural history as big as the first and second Industrial Revolutions (Peters Citation2012). This had previously been declared the “end of theory” (Anderson Citation2008), with the sheer volume of data leading to a reliance on computational methods in a new data-intensive approach to science. As a result:
scientific discovery is not accomplished solely through the well-defined, rigorous process of hypothesis testing. The vast volumes of data, the complex and hard-to-discover relationships, the intense and shifting types of collaboration between disciplines, and new types of real-time publishing are adding pattern and rule discovery to the scientific method (Abbott Citation2009, 114).
Archaeology has not remained aloof from this. For instance, Kristiansen (Citation2014) sought to define a Third Science Revolution in archaeology in part linked to Big Data, and Löwenborg (Citation2018, 48) pointed to the expectations raised by the accumulation of digital data, representing a step towards a new paradigm in archaeology. Kintigh and colleagues saw the grand challenges for future archaeology as predicated on an explosive growth in access to large quantities of data (Kintigh et al. Citation2014, 19), and Gattiglia (Citation2017, 34) and Cooper and Green (Citation2016, 272) have written about the potential transformation of archaeological practice associated with Big Data. Buccellati (Citation2017, 175) has pointed to how archaeologists readily accept vast masses of non-contiguous data, “expecting the hidden connectivity to emerge as we tickle the individual pieces” and the consequent power of what he describes as the unlimited potential for interlacing hierarchies of data fragments at a multiplicity of different levels. Elsewhere, Cunningham and MacEachern (Citation2016, 630) have argued that archaeology aspires to become “big science,” based on (among other things) investment in large-scale projects, the use of advanced and expensive analytical techniques, and the increasing use of Big Data.
The centrality of data to archaeological knowledge has always been the case, but the burden and expectations placed upon data are subtly shifted in a Big Data paradigm. A growing perception of large datasets as resources to be algorithmically mined can be accompanied by a presumption that the data are relatively straightforward and unproblematic, with any problems of reliability or quality overcome by virtue of their quantity. This enables multiple datasets captured under differing conditions and for different purposes to be mashed together into larger, if not big, data. In the process, the conceptual appreciation and understanding of the constitution of archaeological data gained over years of theoretical and practical debate can seem to be set aside in the pursuit of the kind of grand, high-impact factor syntheses that Cunningham and MacEachern warn about (Citation2016, 631). However, as Leonelli notes, debates surrounding Big Data, data-centric research, and data infrastructures have reignited interest in the characterization of data and their transformation into knowledge (Leonelli Citation2015, 810).
In archaeology, for example, Buccellati has argued that the archaeological record is what he calls primordially atomic, since our data are encountered as fragments rather than whole: “they emerge from the soil as disaggregated atoms and are reconstructed through the overall integrity of a proper digital discourse” (Citation2017, 233), and consequently “We do not fragment an observed whole, nor do we impose an analytical fragmentation” (Citation2017, 234). This underlines an ambiguity which frequently exists, in which archaeological data can seem to be both ‘given’ (atomic fragments) and ‘made’ (reconstructed). Archaeologists such as Glyn Daniel, Stuart Piggott, and Christopher Hawkes, for example, considered data as ‘givens,’ crucially distinct from interpretation (e.g., Trigger Citation1998, 3), and as a result the primary way of improving the archaeological knowledge base was through the accumulation of more data and the development of improved techniques for interpreting those data (Trigger Citation1998, 22). In such a light, data are effectively seen as raw materials which, when brought together within a specific context or set of relations, become information which in turn builds into knowledge, in the classic data-information-knowledge-wisdom (DIKW) pyramid (). Seeing data in this light, as raw, or ‘given,’ as something that exists independent of the archaeologist—‘out there,’ waiting to be discovered—is not uncontested from a perspective which sees all data as generated at the point of discovery or recognition, reliant on prior knowledge and experience, and its capture essentially a creative act (e.g., Huggett Citation2015, 15–19). From this standpoint, data are a consequence of cultural and taphonomic processes, emerging as the outcome of the application of knowledge and information in a reversal of the DIKW model, and not raw in any sense. Instead, based on their experience, research objectives etc., the data creator articulates their knowledge to identify and categorize information, and that information is atomized within a digital environment to create data (). Data in these terms are therefore theory-laden, process-laden, and purpose-laden, and not raw in any sense. However, as data have become increasingly perceived as a resource to be mined within a Big Data context, its treatment has arguably reverted to the earlier perception of data as unprocessed, unworked, typically acquired using rigorous scientific methods, distinct from the subjectivities that generated them and independent of the relations and contexts that gave rise to them.
Figure 1. An archaeological variant of the classic Data-Information-Knowledge-Wisdom pyramid illustrating the distinction between data as ‘things given’ and data as ‘things made.’
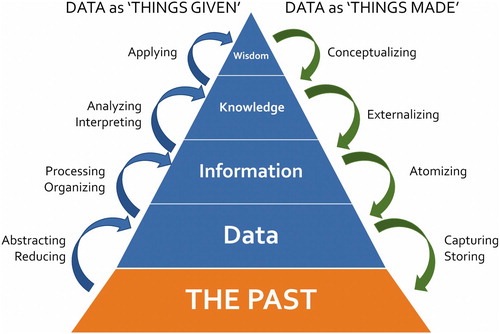
This classic distinction between data as ‘things given’ or ‘things made’ leads to multiple perspectives on data, on what data do and do not represent and consequently how data may be employed most appropriately. For example, in a survey of 45 Information Scientists from 16 countries there were almost as many definitions of data as there were respondents, and while many overlapped to some degree, some were mutually contradictory (Zins Citation2007). More recently, a discussion of archaeological digital data highlighted six definitions of data as a guide to what data can be (Marwick and Pilaar Birch Citation2018, 126 and table 2). An understanding of digital data (and its gaps and absences [Wylie Citation2017, 204]) becomes all the more important when we are told that we are moving into an age of data-centric, data-driven analysis or data-led thinking, in which data takes pre-eminence over theory. Clarity about the nature of digital data and consequently an understanding of its capabilities and limitations is necessary to offset against the enthusiastic determinism of Big Data prophets.
The Digital Data Gaze
Beer has recently emphasized that
we cannot just concern ourselves with the outcomes of data and their analytics … we need to understand those outcomes by exploring how data are seen in the first place … we need to understand the emergence of the data gaze in order to fully understand the consequences of how that gaze is then exercised (Beer Citation2019, 6).
A view of the past fifty years or so of digital archaeology demonstrates changing perspectives on the nature of digital data, even if there has been relatively little debate during that time. For example, in a series of retrospectives Lock has written about the changing relationship of the digital with data through time (e.g., Lock Citation1995; Citation2003, 1–13; Citation2009, 76–78). His model of the development of archaeological computing situated alongside developments in archaeological theory has data embedded within it and he argues that the mediation between data and theory is reliant on their digital representation and manipulation (Lock Citation2003, 9; and see Lock Citation1995, 14ff). As the model by Beale and Reilly (Citation2017: ) also suggests, this parallels a change in the terminology describing computer use in archaeology, from quantitative methods all the way through to digital practice, moving from what Lock describes as data minimizing to data maximizing approaches. In this he contrasts on one hand the data-minimal numerical matrices and flat file databases and the need for theory-driven deductive methods enforced by data-poor digital models, and on the other the richness of multidimensional multi-media data which encourage data-driven analyses (Lock Citation1995, 15–16; Lock Citation2003, 9–12). What this would suggest is that the present circumstance of ‘big’ digital data appearing to drive forward archaeological analysis and interpretation is in fact part of an ongoing development within archaeology rather than revolutionary shift.
But the idea that digital data in the 21st century is simply ‘more of the same’ sits uncomfortably with its description as the “new oil” (Economist Citation2017), the “new gold rush” or that we suffer from “data overload,” a “data deluge,” a “data flood” (e.g., Pink et al. Citation2018, 4)—all terms which encourage a sense of crisis and, at the same time, ground-breaking opportunities. In this kind of environment, digital data are assigned transformative agency yet at the same time assumed to be broadly neutral, transparent, self-evident, and fundamental. Turning the data gaze inwards and examining the nature of digital data is therefore critical as the basis for appreciating its real, rather than imagined, potential.
Digital Data Affordances
Digital data come with a set of affordances: potentialities that facilitate and encourage as well as constrain their application and use (e.g., Majchrzak and Markus Citation2013). Considering technology in terms of affordances can provide a compromise between overly deterministic and social constructivist views of technology (e.g., Nagy and Neff Citation2015, 2). Hence, for example, digitalization is often seen as providing greater flexibility through its separation of function and form, content from medium, in the way it can break down boundaries between data, encourages and supports dynamic and collaborative use, and provides greater scope for re-combining data and generating new datasets. Kaufmann and Jeandesboz (Citation2017, 316–319) suggest a range of digital affordances, many of which directly relate to our use of and relationship with data. These include the malleability and flexibility of digital devices, their storage capabilities, their searchability, their connectivity, their computability, their interactive nature, and their creation and organization of data. All of these—and more—in combination make for an unarguably attractive environment for data production, manipulation, consumption, and knowledge creation. However, at the same time, it can insulate us from the data though access to increasing quantities of data and their apparent quality, usability, and flexibility. For example, Smith has looked at how the consequences of the use of digital devices and data may be to obscure rather than reveal and may prioritize what he calls “data-based gratification” (Citation2018, 2). Following boyd and Crawford (Citation2012, 663), he points to the way that digital data sets can appear to come equipped with an aura of truth, objectivity, and accuracy (Smith Citation2018, 3) and warns of the risk when we
learn to treat and utilise data in parochial and instrumental ways, as simply ‘means to ends’ … rather than as vital artefacts that also agentively construct and structure social experiences and environments (Smith Citation2018, 7).
Data and (Im)materiality
For example, much is made of the immateriality of digital data relative to the materiality of analog records. The significance of digitalization, the inexorable shift from atoms (the material world) to bits (the digital world), was claimed to be irrevocable, unstoppable, and exponential (e.g., Negroponte Citation1995, 4–5). Digital data are seen to offer both potential (flexibility for processing, for transfer, for communication) and risk (data fragility and loss). Does this change the nature of data in the process? The atomization entailed in taking material things and making them digital is inevitably a form of reductionism: we only capture elements that we recognize as being of interest and at the same time we simplify as we abstract from the real world. But is this different to the completion of a traditional context recording sheet, for example? And is the inscription of the data onto the disk substrate or flipping bits in silicon so different to our pencil marks filling out the boxes on the recording sheet? For example, pre-digital technologies such as the punch cards used in the 1890 US Census share many of the characteristics of digital (Armstrong Citation2019). As Strasser and Edwards point out, we talk about digital data in terms of “compiling,” “collecting,” and “assembling” them, “as if they were shells on the beach or a drawer full of Lego pieces” (Citation2017, 330), and in some respects our digital data are little different to material objects which become data by being brought into a collection. Digital data collections are held within defined structures, infrastructures, and architectures in terms of software and hardware: this can be seen as a kind of materiality lodged in silicon (e.g., Drucker Citation2001) but at least provides a kind of proxy materiality which can encourage a view of data as acquiring power through their apparent malleability, portability, and fluidity. This emphasizes that while digital data may be considered immaterial through their decoupling from physical objects, they are nevertheless dependent on physical devices for their re-materialization (e.g., Blanchette Citation2011).
Data and Quantification
Digital data are frequently conceptualized as numeric in form, which means they can be counted and computed (e.g., Kaufmann and Jeandesboz Citation2017, 316). However, numeric data are certainly not exclusively digital: the logarithmic table or the slide rule are equally numeric in terms of their powers of calculation and computation, for example, nor are digital data somehow made neutral through reliance on mathematical processes. Digital data are ultimately stored and processed in binary form, as bits and bytes, and while this remains largely abstract to most users it fundamentally affects aspects of storage and processing, as any programmer experiences as they decide between using an integer or long integer, or a database designer encounters as they select an appropriate field type. At the same time, it is this binary nature that facilitates the transmission of digital data over networks. However, the digital nature of data can disguise an imbalance in information content. As Strasser and Edwards (Citation2017, 336) observe, there is something of a paradox in that a 500-page book and a single scanned photograph may require the same number of bytes of computer memory, yet the book will usually be seen as containing much more information. They suggest:
The fact that many kinds of scientific data, but also so many aspects of our informational lives—from family pictures to favorite music, to epistolary relations—have come to be quantified, and quantified using the same metric, constitutes a historically significant turning point deserving of scholarly attention (Strasser and Edwards Citation2017, 337).
Data and Representation
One clear implication of binary storage is that it consists of an encoded representation of the original data—something that is not easily read by a human. For example, in a database system developed to significantly reduce the storage required by a large archaeological dataset by using binary bit-packing encryption and compression it was estimated that manually decoding a single record would take ten minutes and several sheets of calculations (Huggett Citation1988). Crucially, this required knowledge of the coding algorithm used to be both held external to the system and accessible. Similarly, even simple file formats often contain insufficient information to enable an unambiguous understanding of their meaning. For instance, the Grid file commonly used for raster data in GIS lacks information about which projection was used to make sense of the locational data, what measurement units were used in relation to the grid cell size and the data values, and knowledge of what the data values actually purport to represent. None of this information is included in the digital data itself but (at best) held separately as metadata. As Dourish (Citation2017, 17) has noted, not all these data are equally important: some affect other data, some play a central role in the representation of the data, and some are more critical than others. The issues associated with the reuse of data which has insufficient contextual information, access to codes used in recording systems, etc. have been well-rehearsed elsewhere (e.g., Atici et al. Citation2013; Faniel et al. Citation2013), and of course these are not restricted to digital data—making sense of traditional analog data can be equally problematic. However, the problems may be compounded in a digital context, as data may not only suffer from analog-style problems, but they may also present contextual challenges because of their digital nature: not least requiring a specific software program to retrieve them, for example.
Data and Interpretation
A significant feature of digital data is the way in which it may alter our approach to interpretation. For example, Limp (Citation2016, 350) suggested that traditional field survey data capture is a consequence of observation, followed by interpretation and abstraction, whereas in a digital environment data capture precedes interpretation and abstraction. He suggests that such high-density digital survey leads to a recursive and reflexive engagement with the data, which is clearly beneficial; however, in the process it also changes our relationship with data. Data subtly shifts from something that arises out of our observations and engagement with the physical features to something that is automatically captured absent knowledge and engagement, with limited direct human intervention. In the process, it can be argued that digital data begins to exist the moment it is recorded by the machine and obscures the role of human decisions in its creation (Rendgren Citation2018). Similarly, the use of digital drawing tools ranging from CAD to Structure from Motion photography are increasingly employed as surrogates for traditional field drawing which, among other things, changes the nature of our engagement with the physical remains (Hacıgüzeller Citation2019, 277–278; Morgan and Wright Citation2018, 146–147; Powlesland Citation2016, 32). Furthermore, digital data can constrain and limit subsequent analysis through their structuring and organization which ultimately determine what can and cannot be recorded, and through the set of procedures which shape the retrieval and processing of the data (e.g., Huggett Citation2015, 21–26).
Data and Disintermediation
Digitalization is often associated with disintermediation: the shift from traditional research methods entailing travel, physical access to archives, and face-to-face negotiation, to the technology-based destruction of distance through network connectivity and virtual modes of remote access to data. This can undoubtedly enhance efficiency by removing traditional barriers and constraints, though often not as much as is assumed (e.g., Huggett Citation2000, 13–15). However, in the process it introduces its own new gatekeepers in the shape of the new cyberinfrastructures created to manage the data. These digital infrastructural developments have been largely built and driven by digitally knowledgeable archaeologists, but we are barely beginning to understand the predispositions of these systems (e.g., Svensson Citation2015, 342). For example, it is not just the data that are situated but the data infrastructures themselves are also situated culturally, socially, politically, technologically, and spatially (Svensson Citation2015, 338) and consequently risk the creation of “filter bubbles” which influence certain kinds of data retrieval and use through the design of their search tools and the structuring of their data.
This disintermediation of data is also often associated with a reduction in data friction: for example, digital data are typically seen to be easier to collect, store, rearrange, duplicate, share, and analyze than analog data (e.g., Sepkoski Citation2017, 178). However, it is more the case that the kinds of resistance encountered change with the shift from analog to digital data: the movement of digital data still entails cost, energy and human engagement. Hence,
Every interface between groups and organizations, as well as between machines, represents a point of resistance where data can be garbled, misinterpreted, or lost (Edwards et al. Citation2011, 669),
Data and Amalgamation
Then there is the ease with which digital data can be disembedded or decontextualized, removed from their original locus of discovery and processing and subsequently re-contextualized to enable their reuse in a new setting (e.g., Leonelli Citation2016, 30ff). This process of deconstruction and reconstruction is facilitated in a digital environment, enabling data to be abstracted, remixed, recycled, combining multiple datasets originally separated in space and time (Huggett Citation2018) in ways that would be impossible or at best time-consuming with analog data. While this is not without its challenges, such activities alter our relationship with the data: the arms-length relationship with data encouraged by cyberinfrastructures increases the distance, isolation, even remoteness, of the data consumer from the data producer (Huggett Citation2015). This can create a sense of separation from the data—not so much in terms of the actual data to hand, but in relation to what those data purport to represent. Although the digital analyst is isolated from the object of record in a way that in some respects is no different to the relative isolation experienced through the medium of the printed volume, unlike the printed experience the individual is insulated by the quantity, and apparent quality, usability and flexibility of the digital data. Since much Big Data analysis in archaeology is based upon the amalgamation of ‘small’ data into larger datasets, combining data from multiple sources into massified datasets for analytical purposes, these questions assume greater importance than may have previously been the case. There is a lack of transparency over the manipulation that this typically requires: methods of “data cleansing,” “data integration,” and “data homogenization” are poorly represented in the archaeological literature. This makes it difficult to assess the decisions taken in order to address the different recording conventions, data formats, and data models encountered within the amalgamated datasets and to resolve the host of anomalies within the data themselves. Such problems are only compounded when technical pattern-matching or machine-based aggregation are employed.
Metadata—descriptions of, or information about the data—are often seen as a means of establishing a common context amongst the ambiguities, anomalies, and differences typically experienced across multiple datasets. In the process metadata offers to reduce data friction, although creating metadata can itself be the cause of additional friction through the requirements of its creation and consequent burden on the data providers (e.g., Edwards et al. Citation2011, 673). However, loss of context concerns more than just the technical structuring of the data or typographical errors within it: the data context also entails the individual circumstances of their creation, their recording, and any prior processing and manipulation. This is frequently missing from much archaeological data and the metadata that does exist is very restricted in focus, primarily relating to the needs of discovery (the title of the data and its location, authorship, rights, sources etc.). The use of paradata—provenance and process metadata, focusing on the origins of the data and their derivation along with the methodologies used to generate and manipulate the data—remains largely abstract. When little or no information is provided about these kinds of processes, confidence in the derived data and their subsequent use must be limited at best.
Data Relations
Discussion of digital data affordances such as these highlights that they constrain at the same time as they enable. In certain respects, the affordances of digital data are not so dissimilar to those associated with analog data: their materiality, numerical and informational nature, and representation all have their parallels in an analog environment, and of course, much digital data started their journey as analog in the first place. However, there are significant aspects of digital data which do change the nature of our engagement with data: their near-instant access, volume, and flexibility, not least as understood in Big Data, have transformative potential for the practice of archaeology (e.g., Huggett Citation2018, 101). But it is important to recognize that as the affordances of the digital intervene in and mediate our production, access, and use of data, they have the potential to complicate our relationship with data in ways that may not be helpful to our archaeological practice.
For example, Smith identifies three kinds of data-based relations that arise in a digital environment (Citation2018, 8–11). The first is “fetishization,” when the significance of the data is inflated and assigned a higher level of insight than is warranted by virtue of their digital affordances, or, indeed, those affordances may be largely imaginary and wreathed in mystery. The second is “habituation,” whereby the familiarity, proximity, accessibility, and apparent usability of digital data means that we overlook—or are unaware of—their underlying limitations. The third is “seduction,” in which we are enchanted by our access to digital infrastructures and data flows, using interfaces and tools deliberately designed to encourage and ease our access whilst invisibly shaping it.
The fetishization of digital tools has long been a feature in archaeology, in terms of an emphasis on greater speed, on power, on surface appearance, and on disguise through mystique and lack of transparency, for example (Huggett Citation2004), giving rise to habituation and seduction. Digital data and their associated infrastructures can inadvertently heighten these risks, with interfaces designed to both enable and constrain our use through simultaneously influencing what can be accessed and analyzed and disguising the underlying shortcomings of the data.
To observe that archaeological data are messy, emphasizing their partial, fragmentary, incomplete nature, incorporating embedded interpretations, inconsistent levels of uncertainty and variable expert opinion all mixed together as a set of observations derived across multiple times and numerous places, is not new (e.g., Cooper and Green Citation2016; Gattiglia Citation2015; Holdaway et al. Citation2019). Wylie has written of how archaeological evidence bites back through its “shadowy data,” the “notoriously fragmentary and incomplete nature of the surviving “data imprints” of past lives,” “the paucity and instability of the inferential resources they rely on,” “legible only if they conform to expectations embedded in the scaffolding of preunderstandings that define the subject domain and set the research agenda” (Wylie Citation2017, 204). How we deal with these preunderstandings, with the instability of our digital sources, remains a challenge, and simply applying Big Data approaches does not resolve these problems—if anything, they set them aside or risk covering them up. Studies employing large datasets (if not Big Data) are often unclear over whether methods have been employed to address sampling biases within the data (e.g., Robbins Citation2013, 58), and, for instance, national monument event databases are often poorly understood (Evans Citation2013, 32). For example, national databases use deceptively simple records in to represent highly complex multi-period sites which may have been investigated in various ways at various times, and whose characterization has changed from time to time (Newman Citation2011). Similarly, the complexities of taphonomy in the creation of archaeological features are often poorly represented in our excavation databases, many of which conflate the identification of features with the interpretation of them (Holdaway et al. Citation2019, 876–877). A recent Big Data study employing data to examine aspects of worldwide religion and society (Whitehouse et al. Citation2019) was almost immediately critiqued for aspects of its data collection and manipulation (Slingerland et al. Citation2019) and correcting for these was suggested to reverse the original findings (Beheim et al. Citation2019). Importantly, it was only possible for this continuing debate (e.g., Savage et al. Citation2019) to occur since the data and codes used in the original study were made openly available and underlines both the value of open access and the challenges associated with handling large-scale datasets.
In a Big Data environment it is claimed that messy data is no longer a problem: “It isn’t just that ‘more trumps some’, but that, in fact, sometimes ‘more trumps better’” (Mayer-Schönberger and Cukier Citation2013, 33). Intuitively, the bigger the sample the better the outcome is likely to be. Indeed, in the context of a debate surrounding the analysis of radiocarbon dates, Timpson, Manning, and Shennan (Citation2015, 200–201) suggest that we can set aside concerns over biases in the data since attempting to remove the biases does not necessarily improve the quality and hence reliability of the resulting inferences. They offer three reasons why this might be the case:
Firstly, archaeological data are often frustratingly sparse, and this causes a large sampling error that can easily dwarf the effects of particular biases. Secondly, all data are subject to many different biases. By using the broadest possible inclusion criteria from multiple sources, the Law of Large Numbers predicts that the combination of many different biases will approach a random error. Thirdly, dirty data will have the effect of hiding (adding noise to) any true underlying pattern. This will certainly make it harder to detect what is really going on, but this has the desirable effect of making the null hypothesis harder to reject, thus making the statistical test conservative (Citation2015, 201).
Data-driven Analysis
On its own, a data-centric approach to archaeology employing large datasets and even new tools is not sufficient to lay claim to a paradigm shift or a new scientific revolution in archaeology. Moving beyond data themselves, the key transformation is the way that Big Data and its methods are associated with a shift in theory and methodology: from hypothesis-driven to data-driven analysis. In his famous “end of theory” provocation, Anderson (Citation2008) claimed that data can be analyzed without hypotheses, that algorithms could seek out correlations within large datasets: what Lohr characterizes as “listening to the data” (Citation2015, 104). In this way, Big Data are seen to not need a priori theory, models or hypotheses: instead, they anticipate serendipity, the discovery of pattern where none was previously visible, the revelation of insights derived through access to vast bodies of data. Pour data into a more or less black box of computational analytical tools and stir well in the search for correlations.
A less extreme archaeological perspective suggests that rather than replacing the hypothesis-driven approach, the data-driven or evidence-based approach still uses models and hypotheses but that these now follow the analysis rather than precede it (Gattiglia Citation2015, 115–116). This appears to be a sensible compromise given that, implicitly or explicitly, we are always working within one theoretical regime or another. However, as highlighted above, theory is not some kind of post-hoc add-on to the data: theories and hypotheses are used to recognize, select, collect and record the data in the first place. A priori archaeological theory always precedes data collection and analysis, and indeed, analysis will be constrained by the theoretical constructs applied during the recognition, categorization, and collection of data. The affordances of digital data—their apparent malleability, flexibility, connectivity, mutability, and computability—can encourage us to lose sight of the way in which they become contaminated with methodological and theoretical bias. As long ago as 1989, Wylie warned that such a strictly data-oriented approach to research presumes that culture-historical reconstructions of the past will be unproblematic if only archaeologists can establish sufficiently complete knowledge of the record (Wylie Citation1989, 3). It is interesting therefore to consider whether we are seeing a resurgence of empiricism—a reversion rather than a new data-centric revolution.
More recently, archaeologists were cautioned about the risk of gathering ever greater amounts of evidence while assuming that it otherwise largely speaks for itself (Bevan Citation2015, 1481). As Bowker has observed,
Just because we have big Data does not mean that the world acts as if there are no categories. And just because we have big (or very big, or massive) data does not mean that our databases are not theoretically structured in ways that enable certain perspectives and disable others (Bowker Citation2014, 1797).
And despite what has been claimed for Big Data, correlation does not imply causation. The correlations we find in archaeology do not explain cultural process because they are several steps removed from human practice: effectively we employ proxy data as a means of accessing the immaterial processes behind the tangible evidence we have to hand (visibility as a proxy for knowledge in GIS, or friction as a proxy for accessibility, artifact density for levels of human activity, radiocarbon plots for prehistoric occupation, tombs as indicators of settlement, material culture traits as proxies for social identity and/or group membership, or trade and exchange, and so on). Even establishing a correlation can be problematic: for example, in the context of human-environment interactions they have been described as epistemologically fragile and logically insufficient (Contreras Citation2016, 11):
the identification of correlation is at once a statement of hope and an admission of defeat. It is a statement of hope in that reportage of climate-culture correlation is driven by a conviction that it should be possible to develop the putative links further, and an admission of defeat in that it remains unclear how those links can be developed (Contreras Citation2016, 9, emphasis in original).
Reimagining Big Data
Talk of a digital revolution, a new data-centered scientific paradigm, can overlook the discontinuities in digital data practice which make a properly critical, reflexive, and considered engagement with our digital data even more important. As Kitchin (Citation2014, 1) has argued, “there is an urgent need for wider critical reflection within the academy on the epistemological implications of the unfolding data revolution,” and he suggests that “a potentially fruitful approach would be the development of a situated, reflexive and contextually nuanced epistemology.” The key question is how this might be achieved by reimagining archaeological Big Data, building on the discussion above.
First, we need to seek ways of taking better account of context of the data that make up Big Data: its circumstances of creation, recording, processing, and manipulation before it ever even becomes Big Data. This is frequently missing: the metadata that does exist is very restricted in focus, and the use of paradata remains largely unrealized for the most part. Studies of archaeological data reuse point to the way in which the absence of data context is circumvented (Atici et al. Citation2013, 667; Faniel et al. Citation2013, 298) yet we generally proceed to analysis in its absence and as a consequence, different analysts come to different conclusions regarding the same data. The solution to this entails the development of a biography of data: detail of its complete lifecycle. How can we best capture and bring this data biography to bear, and incorporate Wylie’s “preunderstandings” into data past and present? Resolving this data contextual problem would have significant implications for data archiving, data reuse, analytical replicability, as well as Big Data analysis all at the same time, so could be justifiably regarded as a fundamental objective for archaeology.
As part of this data biography, we need to develop clearer and more transparent ways of handling and resolving issues in our data. The assumption that simply adding more data will overcome problems of bias and effectively reduce if not remove the influence of data errors as the number of data records increases is not sustainable. As discussed above, some studies show that the role of data quality is if anything more important in Big Data as the impact of poor data quality can increase rather than reduce as dataset size increases (e.g., Woodall et al. Citation2014; Meng Citation2018). How do we go about cleansing our data? How do we deal with missing data? How do we go about integrating different datasets? Holdaway et al. (Citation2019, 874) have emphasized that the implementation of Big Data in archaeology raises issues of database integration, standardization of content, recording, and suitable use of data management systems, so that the integration of data obtained from different sources remains problematic, and the increasing quantity and diversity of data generated makes these issues worse. And what happens to those ‘big’ datasets once the analysis is completed? As Cooper and Green (Citation2016, 298) have asked, what are the politics and etiquettes of connecting, employing, and making archaeological data available on an unprecedented scale?
Secondly, we need to resist the overt empiricism associated with Big Data and the inversion of the traditional hypothesis-data analysis relationship. Rather than data-driven, we need to ensure that our enquiries remain hypothesis-driven. This is not to argue for some idealized primacy of the hypothesis-driven methodology, but to recognize that the data themselves are driven by the theories and the prior knowledge and experience of the analyst, and analysts before them in the case of data reuse. While this may be frequently overlooked, it undermines the presumed purity of the data-driven approach. Further, the shift in relationship between data and interpretation in a digital environment discussed above changes the nature of our engagement with the physical archaeological remains in ways that we need to be appropriately critical about.
Thirdly, we need to consider more closely the range of tools and processes brought to bear on Big Data. Like our data, the decision trees, logistic and linear regression models, association rules, Bayes classifiers, neural networks, and so on all have their own histories, cultural contexts, epistemologies and biases which need to be considered (Mackenzie Citation2015, 431). Some might argue such a depth of understanding is not a prerequisite to making successful use of these tools, but the alternative is a kind of push-button approach (such as we often see with our use of GIS) which leaves us exposed to unanticipated and unrecognized errors. In fact, an appreciation of the background to these tools—their historiography, and the assumptions, limitations, and so on associated with them—is not as difficult as an understanding of precisely how they work in algorithmic terms, and might present a reasonable alternative to this level of deconstruction (Huggett Citation2017: section 8).
Finally, we need to develop a more thoughtful, transparent approach to Big Data, which entails being appropriately critical about both our data and our tools. This can be encapsulated in two distinct approaches. First, through seeking to understand Big Data as a site of practice by effectively excavating the digital layers and affordances of data. This can be characterized as a form of cognitive digital archaeology in which the black-boxed digital data and digital tools are effectively deconstructed layer by layer, from their conception, their incorporation in hardware and software, the mediation of software and hardware interfaces, and ultimately their application and use in an archaeological context (Huggett Citation2017). Secondly, through developing alongside this an ethnographic approach to the use of Big Data, seeking to reveal the motivations and actions of the largely hidden creators, developers, programmers, as well as users, of the data and its associated tools (e.g., Huggett Citation2012, 546–548). This entails finding the people within the systems (after Seaver Citation2018, 382) since our use of these tools is mediated by the actions and decisions of those who collected and processed the data and the designers and developers who designed and created the tools themselves, some of whom will be far removed from or effectively hidden from sight within the digital data and devices.
Conclusions
Our archaeological perspectives are increasingly reliant on digital data, much of it now directly derived through digital technologies. While we are increasingly aware of the way in which data are required to be organized in specific ways in order to fit the structures imposed by the digital tools we use, we tend to pay less attention to the ways in which those structures and tools subsequently shape what we do with those data, how we understand and represent data, how data are (re)interpreted and (re)produced, and the implications of the shift from analog to digital data. We need to be more cognizant of the possibilities and risks associated with digital data and methodologies, and the consequences that may flow, appreciating that they are not simple, straightforward, or capable of being set aside in the enthusiastic pursuit of data-driven solutions. In short,
Above all, we need new critical approaches to Big Data that begin with deep skepticism of its a priori validity as a naturalized representation of the social world … Rather than invest in Big Data as an all-knowing prognosticator or a shortcut to ground truth, we need to recognize and make plain its complexities and dimensionality as an emerging theory of knowledge (Crawford, Miltner, and Gray Citation2014, 1670).
Acknowledgments
I should like to thank Parker VanValkenburg and Andrew Dufton for the invitation to present a version of this paper in their “Archaeological Vision in the Age of Big Data” symposium at SAA2019 in Albuquerque and to subsequently contribute to the published collection. I also thank Erik Gjesfield and Enrico Crema for their invitation to contribute to the “Big Data in Archaeology: Practicalities and Possibilities” conference at the University of Cambridge where aspects of this paper were first trialed. Finally, I am grateful to the anonymous reviewers and the editors for their constructive and helpful feedback. As ever, any errors and misconceptions remain my own.
Disclosure Statement
The authors declare no potential conflict of interest.
Notes on Contributor
Jeremy Huggett (Ph.D. 1992, North Staffordshire Polytechnic) is a Senior Lecturer in Archaeology at the University of Glasgow, conducting research into the theory and practice of digital archaeology. His research addresses social, political, and philosophical issues of the application of information technologies in archaeology and their effect on our understanding of the past. He blogs at https://introspectivedigitalarchaeology.com/
ORCID
Jeremy Huggett http://orcid.org/0000-0002-7535-9312
References
- Abbott, M. R. 2009. “A New Path for Science?” In The Fourth Paradigm: Data-Intensive Scientific Discovery, edited by T. Hey, S. Tansley and K. Tolle, 111–116. Redmond WA: Microsoft Research.
- Anderson, C. 2008. “The End of Theory: The Data Deluge Makes the Scientific Method Obsolete.” Wired, June 23, 2008. https://www.wired.com/2008/06/pb-theory/.
- Armstrong, D. 2019. “The Social Life of Data Points: Antecedents of Digital Technologies.” Social Studies of Science 49 (1): 102–117. https://doi.org/10.1177/0306312718821726.
- Atici, L., S. W. Kansa, J. Lev-Tov, and E. Kansa. 2013. “Other People’s Data: A Demonstration of the Imperative of Publishing Primary Data.” Journal of Archaeological Method and Theory 20 (4): 663–681. https://doi.org/10.1007/s10816-012-9132-9.
- Beale, G., and P. Reilly. 2017. “Digital Practice as Meaning Making in Archaeology.” Internet Archaeology 44, https://doi.org/10.11141/ia.44.13.
- Beer, D. 2019. The Data Gaze: Capitalism, Power and Perception. London: Sage.
- Beheim, B., Q. Atkinson, J. Bulbulia, W. Gervais, R. Gray, J. Henrich, M. Lang, et al. 2019. “Corrected Analyses Show that Moralizing Gods Precede Complex Societies but Serious Data Concerns Remain.” PsyArXiv, https://doi.org/10.31234/osf.io/jwa2n.
- Bevan, A. 2015. “The Data Deluge.” Antiquity 89 (348): 1473–1484. https://doi.org/10.15184/aqy.2015.102.
- Blanchette, J.-F. 2011. “A Material History of Bits.” Journal of the American Society for Information Science and Technology 62 (6): 1042–1057. https://doi.org/10.1002/asi.21542.
- Bowker, G. 2014. “The Theory/Data Thing.” International Journal of Communication 8: 1795–1799. https://ijoc.org/index.php/ijoc/article/view/2190.
- boyd, d., and K. Crawford. 2012. “Critical Questions for Big Data.” Information, Communication & Society 15 (5): 662–679. https://doi.org/10.1080/1369118X.2012.678878.
- Buccellati, G. 2017. A Critique of Archaeological Reason: Structure, Digital, and Philosophical Aspects of the Excavated Record. Cambridge: Cambridge University Press.
- Contreras, D. 2016. “Correlation Is Not Enough: Building Better Arguments in the Archaeology of Human-Environment Interactions.” In The Archaeology of Human-Environment Interactions: Strategies for Investigating Anthropogenic Landscapes, Dynamic Environments, and Climate Change in the Human Past, edited by D. Contreras, 3–22. New York: Routledge.
- Cooper, A., and C. Green. 2016. “Embracing the Complexities of ‘Big Data’ in Archaeology: The Case of the English Landscape and Identities Project.” Journal of Archaeological Method and Theory 23: 271–304. https://doi.org/10.1007/s10816-015-9240-4.
- Crawford, K., K. Miltner, and M. Gray. 2014. “Critiquing Big Data: Politics, Ethics, Epistemology.” International Journal of Communication 8: 1663–1672. https://ijoc.org/index.php/ijoc/article/view/2167/.
- Cunningham, J., and S. MacEachern. 2016. “Ethnoarchaeology as Slow Science.” World Archaeology 48 (5): 628–641. https://doi.org/10.1080/00438243.2016.1260046.
- Dourish, P. 2017. The Stuff of Bits: An Essay on the Materialities of Information. Cambridge MA: MIT Press.
- Drucker, J. 2001. “Digital Ontologies: The Ideality of Form in/and Code Storage – or – Can Graphesis Challenge Mathesis?” Leonardo 34 (2): 141–145. https://doi.org/10.1162/002409401750184708.
- Economist, The. 2017. “Leader: The World’s Most Valuable Resource is no Longer Oil, but Data.” The Economist, May 6, 2017. https://www.economist.com/leaders/2017/05/06/the-worlds-most-valuable-resource-is-no-longer-oil-but-data.
- Edwards, P., M. Mayernik, A. Batcheller, G. Bowker, and C. Borgman. 2011. “Science Friction: Data, Metadata, and Collaboration.” Social Studies of Science 41 (5): 667–690. https://doi.org/10.1177/0306312711413314.
- Evans, T. 2013. “Holes in the Archaeological Record? A Comparison of National Event Databases for the Historic Environment in England.” The Historic Environment: Policy & Practice 4 (1): 19–34. https://doi.org/10.1179/1756750513Z.00000000023.
- Faniel, I., E. Kansa, S. Whitcher Kansa, J. Barrera-Gomez, and E. Yakel. 2013. “The Challenges of Digging Data: A Study of Context in Archaeological Data Reuse.” In Proceedings of the 13th ACM/IEEE-CS Joint Conference on Digital Libraries (JCDL ‘13), edited by T. Cole, R. Sanderson, and F. Shipman, 295–304. New York: Association for Computing Machinery. https://doi.org/10.1145/2467696.2467712.
- Gattiglia, G. 2015. “Think Big About Data: Archaeology and the Big Data Challenge.” Archäologische Informationen 38: 113–124.
- Gattiglia, G. 2017. “From Digitization to Datafication. A New Challenge is Approaching Archaeology.” In Il Telescopio Inverso: big Data e Distant Reading Nelle Discipline Umanistiche. AIUCD 2017 Conference, edited by F. Ciotti and G. Crupi, 29–33. Firenze: Associazone per l’Informatica Umanistica e la Cultura Digitale. http://doi.org/10.6092/unibo/amsacta/5885.
- Hacıgüzeller, P. 2019. “Archaeology, Digital Cartography and the Question of Progress: The Case of Çatalhöyük (Turkey).” In Re-Mapping Archaeology: Critical Perspectives, Alternative Mappings, edited by M. Gillings, P. Hacıigüzeller, and G. Lock, 267–280. London: Routledge.
- Hodder, I. 2018. “Big History and a Post-Truth Archaeology.” The SAA Archaeological Record 18 (5): 43–45.
- Holdaway, S., J. Emmitt, R. Phillipps, and S. Masoud-Ansari. 2019. “A Minimalist Approach to Archaeological Data Management Design.” Journal of Archaeological Method and Theory 26 (2): 873–893. https://doi.org/10.1007/s10816-018-9399-6.
- Huggett, J. 1988. “Compacting Anglo-Saxon Cemetery Data.” In CAA87. Computer and Quantitative Methods in Archaeology 1987, edited by C. Ruggles and S. Rahtz, 269–274. Oxford: BAR International Series 393. Available at: https://proceedings.caaconference.org/paper/26_huggett_caa_1987/.
- Huggett, J. 2000. “Computers and Archaeological Culture Change.” In On the Theory and Practice of Archaeological Computing, edited by G. Lock and K. Brown, 5–22. Oxford: Oxford University Committee for Archaeology Monograph 51.
- Huggett, J. 2004. “Archaeology and the New Technological Fetishism.” Archeologia e Calcolatori 15: 81–92. http://www.archcalc.cnr.it/indice/PDF15/05_Hugget.pdf.
- Huggett, J. 2012. “Lost in Information? Ways of Knowing and Modes of Representation in e-Archaeology.” World Archaeology 44 (4): 538–552. https://doi.org/10.1080/00438243.2012.736274.
- Huggett, J. 2015. “Digital Haystacks: Open Data and the Transformation of Archaeological Knowledge.” In Open Source Archaeology: Ethics and Practice, edited by A. Wilson and B. Edwards, 6–29. Berlin: De Gruyter Open. https://doi.org/10.1515/9783110440171-003.
- Huggett, J. 2017. “The Apparatus of Digital Archaeology.” Internet Archaeology 44, https://doi.org/10.11141/ia.44.7.
- Huggett, J. 2018. “Reuse Remix Recycle: Repurposing Archaeological Digital Data.” Advances in Archaeological Practice 6 (2): 93–104. https://doi.org/10.1017/aap.2018.1.
- Kaufmann, M., and J. Jeandesboz. 2017. “Politics and ‘the Digital’: From Singularity to Specificity.” European Journal of Social Theory 20 (3): 309–328. https://doi.org/10.1177/1368431016677976.
- Kintigh, K., J. Altschul, M. Beaudry, R. Drennan, A. Kinzig, T. Kohler, W. Limp, et al. 2014. “Grand Challenges for Archaeology.” American Antiquity 79 (1): 5–24. https://doi.org/10.7183/0002-7316.79.1.5.
- Kitchin, R. 2014. “Big Data, New Epistemologies and Paradigm Shifts.” Big Data & Society 1 (1): 1–12. https://doi.org/10.1177/2053951714528481.
- Kristiansen, K. 2014. “Towards a New Paradigm? The Third Science Revolution and its Possible Consequences in Archaeology.” Current Swedish Archaeology 22: 11–34.
- Leonelli, S. 2015. “What Counts as Scientific Data? A Relational Framework.” Philosophy of Science 82 (5): 810–821. https://doi.org/10.1086/684083.
- Leonelli, S. 2016. Data-centric Biology: A Philosophical Study. Chicago: University of Chicago Press.
- Limp, W. 2016. “Measuring the Face of the Past and Facing the Measurement.” In Digital Methods and Remote Sensing in Archaeology: Archaeology in the Age of Sensing, edited by M. Forte and S. Campana, 349–369. Cham: Springer. DOI 10.1007/978-3-319-40658-9_16.
- Lock, G. 1995. “Archaeological Computing, Archaeological Theory, and Moves Towards Contextualism.” In CAA94. Computer Applications and Quantitative Methods in Archaeology 1994, edited by J. Huggett and N. Ryan, 13–18. Oxford: Tempus Reparatum. Available at: https://proceedings.caaconference.org/paper/02_lock_caa_1994/.
- Lock, G. 2003. Using Computers in Archaeology: Towards Virtual Pasts. London: Routledge.
- Lock, G. 2009. “Archaeological Computing Then and Now: Theory and Practice, Intentions and Tensions.” Archeologia e Calcolatore 20: 75–84. http://www.archcalc.cnr.it/indice/PDF20/7_Lock.pdf.
- Lohr, S. 2012. “Age of Big Data.” New York Times, February 12, 2012. https://www.nytimes.com/2012/02/12/sunday-review/big-datas-impact-in-the-world.html.
- Lohr, S. 2015. Dataism: Inside the Big Data Revolution. London: Oneworld.
- Löwenborg, D. 2018. “Knowledge Production with Data from Archaeological Excavations.” In Archaeology and Archaeological Information in the Digital Society, edited by I. Huvila, 37–53. Abingdon: Routledge.
- Mackenzie, A. 2015. “The Production of Prediction: What Does Machine Learning Want?” European Journal of Cultural Studies 18 (4-5): 429–445. https://doi.org/10.1177/1367549415577384.
- Majchrzak, A., and M. Markus. 2013. “Technology Affordances and Constraints Theory (of MIS).” In Encyclopedia of Management Theory, edited by E. Kessler, 832–836. Thousand Oaks, CA: Sage.
- Marwick, B., and S. Pilaar Birch. 2018. “A Standard for the Scholarly Citation of Archaeological Data as an Incentive to Data Sharing.” Advances in Archaeological Practice 6 (2): 123–143. https://doi.org/10.1017/aap.2018.3.
- Mayer-Schönberger, V., and K. Cukier. 2013. Big Data: A Revolution That Will Transform How We Live, Work, and Think. London: John Murray.
- Meng, X.-L. 2018. “Statistical Paradises and Paradoxes in Big Data (I): Law of Large Populations, Big Data Paradox, and the 2016 US Presidential Election.” The Annals of Applied Statistics 12 (2): 685–726. https://doi.org/10.1214/18-AOAS1161SF.
- Morgan, C., and H. Wright. 2018. “Pencils and Pixels: Drawing and Digital Media in Archaeological Field Recording.” Journal of Field Archaeology 43 (2): 136–151. https://doi.org/10.1080/00934690.2018.1428488.
- Nagy, P., and G. Neff. 2015. “Imagined Affordance: Reconstructing a Keyword for Communication Theory.” Social Media + Society 1 (2): 1–9. https://doi.org/10.1177/2056305115603385.
- Negroponte, N. 1995. Being Digital. London: Hodder and Stoughton.
- Newman, M. 2011. “The Database as Material Culture.” Internet Archaeology 29, https://doi.org/10.11141/ia.29.5.
- Peters, B. 2012. “The Age of Big Data.” Forbes, July 12 2012. https://www.forbes.com/sites/bradpeters/2012/07/12/the-age-of-big-data/.
- Pink, S., M. Ruckenstein, R. Willim, and M. Duque. 2018. “Broken Data: Conceptualising Data in an Emerging World.” Big Data & Society 5 (1): 1–13. https://doi.org/10.1177/2053951717753228.
- Powlesland, D. 2016. “3Di – Enhancing the Record, Extending the Returns, 3D Imaging from Free Range Photography and its Application During Excavation.” In The Three Dimensions of Archaeology: Proceedings of the XVII UISPP World Congress, 1-7 September 2014, Burgos, Spain, edited by H. Kamermans, W. de Neef, C. Piccoli, A. Posluschny, and R. Scopigno, 13–32. Oxford: Archaeopress.
- Rendgren, S. 2018. “What Do We Mean By ‘Data’?” idalab blog, June 20, 2018. https://idalab.de/blog/data-science/what-do-we-mean-by-data.
- Robbins, K. 2013. “Balancing the Scales: Exploring the Variable Effects of Collection Bias on Data Collected by the Portable Antiquities Scheme.” Landscapes 14 (1): 54–72. https://doi.org/10.1179/1466203513Z.0000000006.
- Savage, P., H. Whitehouse, P. François, T. Currie, K. Feeney, E. Cioni, R. Purcell, et al. 2019. “Reply to Beheim et al.: Reanalyses Confirm Robustness of Original Analyses.” SocArXiv, https://doi.org/10.31235/osf.io/xjryt.
- Seaver, N. 2018. “What Should an Anthropology of Algorithms Do?” Cultural Anthropology 33 (3): 375–385. https://doi.org/10.14506/ca33.3.04.
- Sepkoski, D. 2017. “The Database Before the Computer?” Osiris 32: 175–201. https://doi.org/10.1086/693991.
- Slingerland, E., M. Monroe, B. Sullivan, R. Walsh, D. Veidlinger, W. Noseworthy, C. Herriott, et al. 2019. “Historians Respond to Whitehouse et al. (2019), ‘Complex Societies Precede Moralizing Gods Throughout World History.’” PsyArXiv, https://doi.org/10.31234/osf.io/2amjz.
- Smith, G. 2018. “Data Doxa: The Affective Consequences of Data Practices.” Big Data & Society 5 (1): 1–15. https://doi.org/10.1177/2053951717751551.
- Strasser, B., and P. Edwards. 2017. “Big Data Is the Answer … But What Is the Question?” Osiris 32: 328–345. https://doi.org/10.1086/694223.
- Succi, S., and P. Coveney. 2019. “Big Data: The End of Scientific Method?” Philosophical Transactions of the Royal Society A: Mathematical, Physical and Engineering Sciences 377: 1–15. https://doi.org/10.1098/rsta.2018.0145.
- Svensson, P. 2015. “The Humanistiscope: Exploring the Situatedness of Humanities Infrastructure.” In Between Humanities and the Digital, edited by P. Svensson and D. Goldberg, 337–353. Cambridge, MA: MIT Press.
- Timpson, A., K. Manning, and S. Shennan. 2015. “Inferential Mistakes in Population Proxies: A Response to Torfing’s ‘Neolithic Population and Summed Probability Distribution of 14C-Dates.’” Journal of Archaeological Science 63: 199–202. https://doi.org/10.1016/j.jas.2015.08.018.
- Trigger, B. 1998. “Archaeology and Epistemology: Dialoguing Across the Darwinian Chasm.” American Journal of Archaeology 102 (1): 1–34. https://doi.org/10.2307/506135.
- Whitehouse, H., P. François, P. Savage, T. Currie, K. Feeney, E. Cioni, R. Purcell, et al. 2019. “Complex Societies Precede Moralizing Gods Throughout World History.” Nature 568: 226–229. https://doi.org/10.1038/s41586-019-1043-4.
- Woodall, P., A. Borek, J. Gao, M. Oberhofer, and A. Koronios. 2014. “An Investigation of How Data Quality is Affected by Dataset Size in the Context of Big Data Analytics.” Proceedings of the 19th International Conference on Information Quality (ICIQ-2014), 24–33. https://ualr.edu/informationquality/iciq-proceedings/iciq-2014/.
- Wylie, A. 1989. “Archaeological Cables and Tacking: The Implications of Practice for Bernstein’s Options Beyond Objectivism and Relativism.” Philosophy of the Social Sciences 19 (1): 1–18. https://doi.org/10.1177/004839318901900101.
- Wylie, A. 2017. “How Archaeological Evidence Bites Back: Strategies for Putting Old Data to Work in New Ways.” Science, Technology, & Human Values 42 (2): 203–225. https://doi.org/10.1177/0162243916671200.
- Zins, C. 2007. “Conceptual Approaches for Defining Data, Information, and Knowledge.” Journal of the American Society for Information Science and Technology 58 (4): 479–493. https://doi.org/10.1002/asi.20508.