Abstract
In medical education, the use of flipped classrooms is on the increase. The complex, interdependent active- and social learning activities often built into the ‘in-class’ phase of this new approach may, however, cause cognitive overload, impeding learning. Computer-supported collaborative learning could play a role in reducing such cognitive load. The current study investigated how four constituent factors of ‘in-class’ scenarios for ‘flipped’ classrooms affect the intrinsic, germane and extraneous cognitive load for students. After participating in a computer-supported ‘in-class’ scenario for a radiology training course that combined face-to-face individual activities, small-group work and class-wide activities, undergraduate medical students (n = 135) completed a questionnaire rating the cognitive load perceived. Computer support comprised a program for radiology assessment and a learning dashboard that captured and visualized the assessment results. Total intrinsic load, germane load, and extraneous load (EL) were calculated from the results obtained. The ‘high’ intrinsic load, ‘high’ germane load, and ‘low’ EL indicate that the computer-supported ‘in-class’ scenario was cognitively challenging but that sufficient mental effort could be spent on task performance and learning. We attribute the successful management of the cognitive load imposed by this complex in-class scenario to the specially designed learning dashboard.
Introduction
In medical education, as in other education domains, the ‘flipped’ or ‘inverted’ classroom has gained in popularity as a teaching format (Tolks et al. Citation2016; Chen et al. Citation2018; Lin and Hwang Citation2019). The labels ‘flipping’ and ‘inversion’ refer to the key characteristic of this instructional approach: foundational knowledge traditionally transmitted in-class by lectures is now acquired outside the classroom by preparatory self-study. Elaboration and application of this basic knowledge traditionally done ‘out-of-class’ by homework is now addressed through assignments in the classroom.
Practice points
Flipped classroom research should focus more on the ‘in-class’ phase of this pedagogical approach.
Efficient instruments measuring the cognitive load imposed by ‘in-class’ scenarios of flipped classroom courses on learners are needed to guarantee the effectiveness of the instruction in this phase.
Computer support, especially via learning dashboards, could play an important role in the management of the cognitive load of complex ‘in-class’ flipped classroom scenarios.
Although the ‘flipping’ of traditional lectures can take many forms, in practice and researchers define the ‘flipped’ classroom in various ways. In Abeysekera’s definition (Abeysekera and Dawson Citation2015), which can be taken as standard, the ‘flipped’ classroom is seen as a set of pedagogical approaches that:
move most information-transmission teaching out of class;
use class time for learning activities that are active and social; and
require students to complete pre- and/or post-class activities to fully benefit from in-class work.
Thus, the ‘flipped’ classroom approach is an educational method that sets out to promote student-centered, self-directive, and active learning (Awidi and Paynter Citation2019). In general, such learning will often call into play higher order cognitive functions that are more cognitively demanding than the cognitive functions addressed by more passive traditional teaching formats. The active-learning (application, problem solving) and social-learning (discussion) activities of ‘flipped’ classroom courses are usually organized in the ‘in-class’ phase of a course. These ‘in-class’ activities are often interdependent and arranged in complex scenarios. It is likely that such complex ‘in-class’ scenarios will impose a heavy information-processing load on students.
As Miller (Citation1956) postulated more than half a century ago, the capacity of our working memory is very limited and can process no more than approximately 5–9 units of information at any particular moment. Proceeding from this starting point, Sweller (Citation2010) developed the cognitive load theory (CLT), which suggests that during learning our working memory is subject to different types of load and that overloading working memory impedes learning. In his view, the unchangeable core of a problem or complexity of a task for a given learner imposes the intrinsic load (IL). It is therefore influenced by prior knowledge and experience. The extraneous load (EL) is the additional cognitive load caused by aspects of an assignment that make a task more difficult but do not lead to learning. It is imposed by factors such as ineffective instructional techniques and distractions issuing from the learning environment. Finally, the germane load (GL) is also an additional load, but one that helps learning by activating cognitive processes that produce mental schemas in long-term memory.
A bottleneck for learning with a ‘flipped’ classroom approach might therefore be the ‘in-class’ phase with its complex learning scenarios for which our limited information processing capacity does not suffice. While the ‘out-of-class’ self-study phase is often highlighted as a weak link in the ‘flipped’ classroom approach (Lin and Hwang Citation2019), weaknesses of the ‘in-class’ phase are given much less attention. Despite increased awareness within the educational community that the cognitive load of instruction influences the effectiveness of learning considerably (Van Merriënboer and Sweller Citation2010; Young et al. Citation2014), a review of the current literature on ‘flipped’ classroom courses (Abeysekera and Dawson Citation2015; Chen et al. Citation2018; Lin and Hwang Citation2019) reveals a lack of research on how this educational method affects the cognitive load for learners during the ‘in-class’ phase and how computer support might be used to alleviate the problem of cognitive overload.
The current empirical study aimed to fill the above-mentioned gap in our knowledge by investigating what effect a computer-supported ‘in-class’ scenario of a ‘flipped’ classroom course has on the different types of cognitive load for the learner. It investigated how constituents of the ‘in-class’ scenario integrating individual activities, small group work, and class-wide activities affect the intrinsic, extraneous, and germane cognitive load for students. Learning tasks, teachers, learning technology, and physical environment were defined as the constituent factors of the ‘in-class’ scenario.
The factor ‘learning technology’ included the assessment program ‘VQuest’ that combined 2D and 3D radiologic images with different question types (Vincken et al. Citation2020) and a learning dashboard, an application that captures and visualizes traces of learning activities, in order to promote awareness, reflection, and sense-making. This ‘PRISMA’ learning dashboard was an application developed in-house to support the face-to-face small-group discussions based on results of previously executed ‘Vquest’assessments. Several interaction techniques such as filter, select, and reconfigure-functions were built into the learning dashboard to focus a group on issues considered to be of interest and to support them in tracking and comparing individual responses (de Leng and Pawelka Citation2020).
The present study did not address the ‘out-of-class’ phase of the ‘flipped’ classroom course in which the students acquired foundational knowledge by individual self-study, because the current literature already shows quite adequately how good design of self-study materials (Mayer and Moreno Citation2003; Carpenter et al. Citation2012; Brame Citation2016; Rana et al. Citation2017) can reduce cognitive load during an ‘out-of-class’phase such as this.
Methods
Setting
Undergraduate medical students at the University of Münster take mandatory radiology courses on different body regions such as the chest, abdomen or neural system. These courses are traditionally offered as a series of lectures ending with a small group seminar. In an endeavor to make radiology education more active, standardized and aligned with modern radiologic practice (for instance, digital stack viewing of volumetric CT or MRI images), the educational institute together with the radiology department planned a pilot project to transform this traditional teaching format into a ‘flipped’ classroom format. In April 2018, third-year medical undergraduate students took part in mandatory ‘flipped’ classroom course on chest imaging, the ‘in-class’ phase of which was a single full day event, a so-called ‘radiology day’. In this six to seven hour event, a full cohort of students participated in multi-layered activities scheduled in a large open computer landscape. In an ‘out-of-class’ phase, not covered in this study, students had to prepare themselves with online self-study material.
Learning goals of the course were to acquire: (a) basic skills in systematic viewing of chest images and (b) basic skills in recognizing and describing image patterns of chest images. The ‘in-class’ activities were based on an educational scenario described in a previous study. The current course upscaled this scenario and involved seven groups of 16–21 students. Each student had a computer and each group was supervised by a radiologist. During the ‘radiology day’, students had to work on five clinical cases with several radiologic images (2D X-rays and volumetric CT-images of the chest). The procedure for each clinical case was as follows:
First, students were given 25–30 minutes to elaborate individually on a case using the assessment program ‘VQuest’ ().
Individual interpretations were discussed in succession for [a further] 25–30 minutes within small groups. Each small group was supervised by a radiologist and supported by the ‘PRISMA’ learning dashboard. The learning dashboard presented the responses of all the individual students to the whole group in a structured and anonymous way on a large screen ().
Finally, one radiologist led a 10-minute plenary discussion on disorder/disease-pairs (for instance, ‘pneumonia’ and ‘atelectasis’) that can produce a similar radiologic image pattern (‘consolidation’) and wrapped up the discussion for all seven groups together ().
Figure 1. Students elaborating individually on a case on the basis of radiologic images via the assessment program ‘VQuest’.
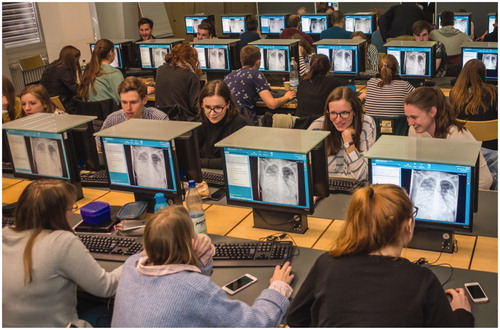
Figure 2. Small groups of about 18 students, supervised by a radiologist, discuss the individual work. The big screen presents the ‘PRISMA’ learning dashboard to the small group in the foreground. In the background is another small group with a radiologist looking at their own learning dashboard.
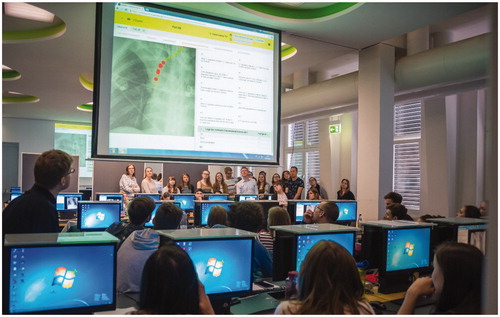
Figure 3. Moderated plenary discussion, involving all seven groups, on disorder/disease-pairs that can produce a similar radiologic image pattern.
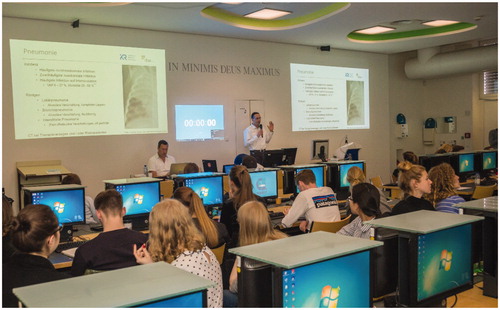
The following constituent factors were identified for the ‘in-class’ phase of the ‘flipped’ classroom course:
Learning task: Individual tasks on systematic viewing/description with small group and class-wide discussions.
Teachers: The radiologists supervising the small groups and the radiologist moderating the class-wide discussions.
Learning technology: The assessment program ‘VQuest’ used for the individual tasks and the learning dashboard ‘PRISMA’ used for the small group discussions.
Physical environment: A large open computer landscape.
Questionnaire
Self-rating instruments have shown promise for accurate and efficient measurement of the three types of cognitive load arising in classroom and training settings (Leppink et al. Citation2013; Sewell et al. Citation2016). We therefore chose to develop a self-rating instrument to measure cognitive load experienced by learners during the ‘in-class’ phase of a ‘flipped’ classroom scenario. We followed the AMEE guide recommendations for drawing up questionnaires for education research (Artino et al. Citation2014). The final version consisted of 18 statements to be responded to on an 11-point Likert scale, in which the anchors were 0 (‘fully disagree’) and 10 (‘fully agree’). The 11-point scale permitted the ordinal data to approximate continuous data, as in prior studies (Leppink et al. Citation2013; Sewell et al. Citation2016). Of the 18 items on the questionnaire, five measured intrinsic load (IL), four germane load (GL) and nine extraneous load (EL) (). Statements for the intrinsic and germane load were formulated in such a way that a high score also indicated a high cognitive load. During pilot testing of the questionnaire with the target learners, it became clear that most statements related to the extraneous load gained clarity when they were formulated in an affirmative way. This meant that for items EL1–EL8 a high score indicated a low extraneous cognitive load. To simplify the direct interpretation of the scores on statements related to extraneous cognitive load together with those on statements applying to the other types of cognitive load, the scores for items EL1–EL8 were inverted so that a higher value reflected a higher cognitive load.
Table 1. Students’ perceptions of the cognitive load of the ‘radiology day’.
Data collection and analysis
Subjects completed the paper-based questionnaire anonymously, directly following the ‘radiology day’. In addition to the 18 cognitive load items (), respondents provided the number of their so-called ‘clinical group’, an organizational unit to which about six students are allocated at the beginning of their training and in which they continue to collaborate throughout their further studies. We chose this organizational unit as a basis to populate the seven small groups of the ‘in-class’ activities to prevent students losing too much time in getting acquainted with one another. Retrospectively, this ‘clinical group’ number enabled the researchers to relate anonymous subjects with anonymous teachers and computer landscape locations.
Student responses were manually entered in MS Excel 2013 and the entries were double checked by a second person. Means and standard deviations were calculated per item with MS Excel and then averaged for each set of items to calculate total intrinsic (IL), germane (GL) and extraneous load (EL). To aid interpretation of the scores for cognitive load, we classified the scores on an 11-point scale as follows: 0–2 = very low, 2–4 = low, 4–6 = medium, 6–8 = high, and 8–10 = very high cognitive load.
In addition, a routine on-line ‘end of semester’ program evaluation 2.5 months after the ‘radiology day’ was used to collect student perceptions of the effectiveness of the ‘radiology day’ for achieving its central learning goals: the skill of systematic viewing and the skill of describing image pattern of chest images. For this purpose, two questions were added to which participants had to respond on the regular Likert scale of the online program evaluation. The anchors of this 10-point scale were 1 (‘very instructive’) and 10 (‘not instructive at all’). We also analyzed student responses to standard open questions of the program evaluation, inviting them to comment on or make suggestions for the ‘radiology day’ program. The same constituent factors of the ‘in-class’ phase of the ‘flipped’ classroom scenario that formed the basis of the cognitive load questionnaire were used for the deductive analysis of students’ free text responses, namely: learning tasks, teachers, learning technology, and physical environment.
Results
Of the 143 third-year medical undergraduate students participating in the ‘in-class’ phase of the ‘flipped’ classroom scenario, 135 (94%) completed the questionnaire designed to measure the cognitive load imposed by the learning tasks, teachers, learning technology, and physical environment within the scenario. One hundred and twenty students answered all items of the questionnaire (84%). shows the means and standard deviations of the responses for every item of the questionnaire within the three types of cognitive load. The means for the total IL, GL, and EL are also shown.
In the on-line program evaluation carried out 2.5 months after the ‘radiology day’, 121 students (85%) responded to the statement on systematic viewing skills and 122 students (85%) to the statement on description skills. shows the means and standard deviations of the responses.
Table 2. Students’ perception of the instructional effectiveness of the ‘radiology day’.
Analysis of the students’ free text responses in the on-line program evaluation 2.5 months after the ‘radiology day’ identified some frequently recurring issues. Concerning the constituent factor ‘learning task’, students indicated that the ‘in-class’ activities involved too much content for the allocated time and that they lacked sufficient prior knowledge. For the factor ‘physical environment’, students expressed their concern about the suitability of the large open computer landscape for the multi-layered activities. presents these issues together with a few characteristic feedback comments.
Table 3. Students’ remarks regarding ‘radiology day’.
Discussion and conclusions
The ‘in-class’ phases of ‘flipped’ classroom courses regularly encompass scenarios with multi-layered interdependent active- and social learning activities. Such complex scenarios carry the risk of causing cognitive overload, thereby interfering with task performance and/or learning itself. To shed more light on how complex learning scenarios during the ‘in-class’ phase of ‘flipped’ classroom courses in medical education affect the cognitive load for learners and to establish what role computers can play in reducing that load, we investigated the cognitive load imposed by ‘in-class’ active- and social learning activities during a ‘flipped’ classroom radiology course supported by a learning dashboard. Building on previously validated self-rating instruments for measuring different types of cognitive load, we developed an 18-item questionnaire with which we explored the intrinsic load (IL), germane load (GL) and extraneous load (EL) imposed by four constituent factors within the ‘in-class’ phase: learning tasks, teachers, learning technology, and physical environment.
Students rated the extraneous cognitive load (EL) stemming from the teaching techniques and the environment, with an average total score of 2.76 ± 2.25. The germane cognitive load (GL) arising from the student’s use of deliberate strategies to enhance meaningful learning, was rated with an average total score of 6.50 ± 2.16. Finally, the intrinsic cognitive load (IL), related to the complexity of the task and the degree of prior knowledge, received an average total score of 7.46 ± 1.73. Clustering these scores in the ‘load’ categories ‘very low’ [0–2], ‘low’ [2–4], ‘medium’ [4–6], ‘high’ [6–8], and ‘very high’ [8–10], revealed an overall impression that the ‘in-class’ activities of the ‘flipped’ classroom radiology course imposed a ‘low’EL, a ‘high’GL, and a ‘high’IL on students.
According to CLT, cognitive processes that do not contribute to managing tasks or to learning, constitute an EL on our working memory. Owing to the limited capacity of the working memory, this EL uses up already scarce cognitive resources needed to deal with task performance and learning. We therefore interpret the ‘low’ extraneous cognitive load perceived by students during the ‘in-class’ phase of the radiology course investigated as a positive sign. It indicates that it is possible to design multi-layered interdependent activities without losing the critical amount of working memory necessary to deal with inherently complex tasks and at the same time learn from them.
Altogether, the instructional tools chosen here allowed an efficient workflow between the various interdependent activities without imposing any considerable extraneous load (EL4, EL5). In our opinion, the ‘PRISMA’ learning dashboard contributed in several ways to the successful management of cognitive load imposed by this complex in-class scenario. It automatically collated the results of the essentially formative individual assessments and presented them as feedback to the respective groups. Studies on the ‘out-of-class’ phase of flipped classrooms indicate that formative testing with extensive feedback during pre-class preparation stimulates student confidence and motivation (De Kleijn et al. Citation2013). With the learning dashboard, we could apply such an approach in the classroom.
The anonymous and well-structured presentation helped teachers to identify common errors made and discuss possible misconceptions underlying these errors in a constructive way. The learning dashboard also enabled students to reflect on their responses, ask questions, and discuss issues of interest to them. Finally, the interaction functionalities of the dashboard, enabled the teacher to manage and adapt the collated information in real time, so as to decrease EL. The learning dashboard thereby fostered a student-centered approach in which the cognitive load of teaching techniques could be adjusted to prior knowledge and thus promote meaningful learning.
The importance of adjusting teaching to fit individual needs and capabilities so as to optimize cognitive load during learning is also stressed in a recent study on cognitive load imposed during instruction on medical procedures (Sewell et al. Citation2020). Although such training is part of postgraduate education and fully outside a flipped classroom context, it sheds light on how to manage cognitive load during ‘in-class’ flipped classroom teaching. Teachers were interviewed on how they perceived challenges faced by the trainee and their own teaching strategies in relation to cognitive load management, and described employing strategies to match IL to the trainee’s abilities, minimizing EL (e.g. through encouragement or definition of expectations), and optimizing GL (e.g. through schemas or stop and focus techniques). The dashboard applied in our ‘in-class’ scenario supports many of these favorable teaching behaviors.
Applying self-determination theory (Ryan and Deci Citation2000; Van Nuland et al. Citation2012) to our computer-supported ‘in-class’ scenario, the dashboard could be said to make learning manageable (autonomy) and achievable for every participant (competence). Furthermore, the social context of handling issues in a group gives individual students a feeling of being able to cope with learning tasks (competence) and a sense that their efforts to acquire knowledge and skills are valued by their co-workers (relatedness). Competence, autonomy, and relatedness are the three basic cognitive needs identified in self-determination theory as essential for building an intrinsic motivation to learn. Intrinsic motivation can then increase the focus and effort spent on learning and thus potentially lower intrinsic and raise GL (Seufert Citation2018).
One constituent factor of the ‘in-class’ phase, however, detracted from the general picture of a ‘low’ extraneous cognitive load: That was the ‘physical environment’. The large open-plan computer landscape in which the activities took place produced a ‘high’ extraneous cognitive load (EL7). Although the infrastructure of the information technology was adequate for the complex scenario, the large, busy space with little or no physical separation between the groups and their supervisors made it harder for students to concentrate on their own discussions. Moveable sound-absorbing partitions could offer better acoustic and visual privacy in a large, open space computer landscape such as this.
During the ‘in-class’ phase of a ‘flipped’ classroom course, educators generally strive to involve students in higher order cognitive activities such as the application of previously acquired conceptual and procedural knowledge in solving problems. We would expect more challenging tasks such as these to impose a greater intrinsic cognitive load. Besides being challenging, an effective learning task should be manageable. To reflect and learn from their performance students also need some free working memory to cope with the germane cognitive load. For effective learning, we would expect both the intrinsic and the germane cognitive load to be ‘high’ but not ‘very high’.
We therefore interpret the ‘high’ germane and ‘high’ILs, as revealed by the responses to the cognitive load questionnaire for the ‘in-class’ phase of the radiology course, as a positive sign. The impression received is that the tasks set were cognitively challenging but that at the same time, sufficient mental effort could be spent on learning. This impression is further supported by the students’ responses to questions in the on-line program evaluation of the instructional effectiveness of the ‘flipped’ classroom course.
It is interesting to note, however, that one of the five items making up the total IL, the ‘small group discussions’-task (IL5), was rated considerably lower than the other items. We interpret this relatively low cognitive load of the small group discussions as a negative sign, because such interactions normally require more cognitively demanding activities such as checking and critiquing. It seems that the small groups did not exploit the full potential of the discussions. This impression is reinforced by free text comments in the online program evaluation where students stated that an overfilled schedule with too many tasks left too little time for small group discussions. This is a real loss because interactive student engagement is generally seen as one of the most valuable ingredients for effective learning (Chi Citation2009).
Although the 18-item questionnaire for measuring the cognitive load imposed by constituent factors of the ‘in-class’ phase of a ‘flipped’ classroom course is based on previously validated self-rating instruments for measuring different types of cognitive load, the questionnaire itself did not undergo any exploratory or confirmatory analysis of its construct validity. Although qualitative findings from other sources confirmed the impressions of the cognitive load based on the findings of our questionnaire, more rigorous studies on the validity and reliability of the questionnaire are needed. Future studies could usefully look at learning outcome measures that could not be analyzed in the ‘real-life’ educational setting used by us. On the other hand, the current study can be seen as a first step in the development of instruments that could help to identify areas in complex ‘in-class’ scenarios in which cognitive load is imbalanced or overwhelming. That will then allow us to optimize our teaching in ‘flipped’ classroom courses.
Glossary
A learning dashboard: Is ‘an application that tracks and captures data on user actions and learning activities and visualizes them so as to promote awareness, reflection, sense-making, and impact, for instance by influencing learning behavior. Broadly speaking, there are three types of learning dashboards: those supporting learning during (1) face-to-face lectures, (2) face-to-face group work, and (3) online or blended learning.’ Verbert K, Govaerts S, Duval E, Santos JL, Van Assche F, Parra G, Klerkx J. (2014). Learning dashboards: an overview and future research opportunities. PersUbiquit Comput. 18(6):1499–1514.
Disclosure statement
The authors report no conflicts of interest. The authors alone are responsible for the content and writing of the article.
Additional information
Notes on contributors
Bas de Leng
Bas de Leng, PhD, is an Assistant Professor and Chair of the E-learning Competence Centre of the Medical Faculty Educational Institute (IfAS) at Muenster University (WWU). His research interests lie in the field of Technology-Enhanced Learning, with an emphasis on computer-supported collaborative learning and computer-mediated orchestration of activities in blended learning settings for medical education.
Friedrich Pawelka
Friedrich Pawelka, MSc, is a researcher and software developer, also at the E-learning Competence Centre of the Medical Faculty Educational Institute (IfAS) at Muenster University (WWU). His work focuses on the application of information technology and artificial intelligence to medical education.
References
- Abeysekera L, Dawson P. 2015. Motivation and cognitive load in the flipped classroom: definition, rationale and a call for research. High Educ Res Dev. 34(1):1–14.
- Artino AR, Jr., La Rochelle JS, Dezee KJ, Gehlbach H. 2014. Developing questionnaires for educational research: AMEE Guide No. 87. Med Teach. 36(6):463–474.
- Awidi IT, Paynter M. 2019. The impact of a flipped classroom approach on student learning experience. Comput Educ. 128:269–283.
- Brame CJ. 2016. Effective educational videos: principles and guidelines for maximizing student learning from video content. CBE-Life Sci Educ. 15(4):es6.
- Carpenter SK, Cepeda NJ, Rohrer D, Kang SHK, Pashler H. 2012. Using spacing to enhance diverse forms of learning: review of recent research and implications for instruction. Educ Psychol Rev. 24(3):369–378.
- Chen K-S, Monrouxe L, Lu Y-H, Jenq C-C, Chang Y-J, Chang Y-C, Chai PY-C. 2018. Academic outcomes of flipped classroom learning: a meta-analysis. Med Educ. 52(9):910–924.
- Chi MTH.2009. Active–constructive–interactive: a conceptual framework for differentiating learning activities. Top Cogn Sci. 1(1):73–105.
- De Kleijn RAM, Bouwmeester RAM, Ritzen MMJ, Ramaekers SPJ, Van Rijen HVM. 2013. Students’ motives for using online formative assessments when preparing for summative assessments. Med Teach. 35(12):e1644–e1650.
- de Leng B, Pawelka F. 2020. The use of learning dashboards to support complex in-class pedagogical scenarios in medical training: how do they influence students’ cognitive engagement? Res Pract Technol Enhanc Learn. 15(1):14.
- Leppink J, Paas F, Van der Vleuten CPM, Van Gog T, Van Merriënboer JJG. 2013. Development of an instrument for measuring different types of cognitive load. Behav Res Methods. 45(4):1058–1072.
- Lin H-C, Hwang G-J. 2019. Research trends of flipped classroom studies for medical courses: a review of journal publications from 2008 to 2017 based on the technology-enhanced learning model. Interact Learn Environ. 27(8):1–27.
- Mayer RE, Moreno R. 2003. Nine ways to reduce cognitive load in multimedia learning. Educ Psychol. 38(1):43–52.
- Miller GA. 1956. The magical number 7, plus or minus 2- some limits on our capacity for processing information. Psychol Rev. 63(2):81–97.
- Rana J, Besche H, Cockril lB. 2017. Twelve tips for the production of digital chalk-talk videos. Med Teach. 39(6):653–659.
- Ryan R, Deci E. 2000. Self-determination theory and the facilitation of intrinsic motivation, social development, and well-being. Am Psychol. 55(1):68–78.
- Seufert T. 2018. The interplay between self-regulation in learning and cognitive load. Educ Res Rev. 24:116–129.
- Sewell JL, Boscardin CK, Young JQ, Cate O, O'Sullivan PS. 2016. Measuring cognitive load during procedural skills training with colonoscopy as an exemplar. Med Educ. 50(6):682–692.
- Sewell JL, Bowen JL, Cate OT, O'Sullivan PS, Shah B, Boscardin CK. 2020. Learning challenges, teaching strategies, and cognitive load: insights from the experience of seasoned endoscopy teachers. Acad Med. 95(5):794–802.
- Sweller J. 2010. Element interactivity and intrinsic, extraneous, and germane cognitive load. Educ Psychol Rev. 22(2):123–138.
- Tolks D, Schäfer C, Raupach T, Kruse L, Sarikas A, Gerhardt-Szép S, Kllauer G, Lemos M, Fischer MR, Eichner B, et al. 2016. An introduction to the inverted/flipped classroom model in education and advanced training in medicine and in the healthcare professions. GMS J Med Educ. 33(3):46.
- Van Merriënboer JJG, Sweller J. 2010. Cognitive load theory in health professional education: design principles and strategies. Med Educ. 44(1):85–93.
- Van Nuland H, Taris T, Boekaerts M, Martens R. 2012. Testing the hierarchical SDT model: the case of performance-oriented classrooms. Eur J Psychol Educ. 27(4):467–482.
- Vincken KL, Ravesloot CJ, Mol C. 2020. Vquest; [accessed Apr]. http://vquest.nl/
- Young JQ, Van Merrienboer J, Durning S, Ten Cate O. 2014. Cognitive load theory: implications for medical education: AMEE guide No. 86. Med Teach. 36(5):371–384.