Abstract
Natural amenities and the aesthetic value of agricultural landscapes are important territorial assets for improving rural tourism and the quality of the living environment. To identify which characteristics shape the visual quality of a landscape, a stated-preference survey was conducted (N = 200) using photorealistic landscape visualisations of four different landscape attributes (point green elements, linear green elements, crop diversity and presence of livestock). We estimated respondents’ preferences for landscape attributes, examined the extent of agreement among respondents and identified socio-economic factors influencing their responses. Results revealed that point elements had the highest general preference. About 70% of respondents preferred diverse and highly structured landscapes, while about 30% of respondents had opposing preferences. Preferences were also found to be dependent on the individual’s sociocultural background, such as their level of education, gender or age. These results can help to improve the multi-objective targeting of policies by including an aesthetic value perspective.
1. Introduction
Agricultural landscapes are known to offer various and substantial benefits to society through the provision of ecosystem services (ES) (Zhang, Ricketts, Kremen, Carney, & Swinton, Citation2007). The interest in ES has increased over the past years, with the focus mostly on (semi-)natural ecosystems, and has often neglected the particularities of agricultural landscapes. Intuitively, the main purpose of the agricultural landscape is to deliver provisioning services such as food, fibre and fuel. However, these areas can also offer cultural ecosystem services (CES) by providing recreational, aesthetic and spiritual benefits (Millennium Ecosystem Assessment, Citation2005). These intangible dimensions of ES are rarely considered (Chan et al., Citation2012), and their valuation remains difficult. An evaluation of these services that will enable policy makers to trade off monetary and non-monetary values in a rational manner is required (TEEB, Citation2010). Though there are numerous discussions and suggestions on how to include a greater variety of social aspects into the ES approach (e.g. Bieling & Plieninger, Citation2013; Chan et al., Citation2012; Szücs, Anders, & Bürger-Arndt, Citation2015), so far, no commonly accepted framework for assessing CES has been developed (Chan et al., Citation2012).
One dimension of CES is the aesthetic value of landscapes. In a study by Plieninger, Dijks, Oteros-Rozas, and Bieling (Citation2013), aesthetic values were reported to be perceived as the most important CES, and aesthetic appreciation was found to be the strongest motivator for interest in environmental topics (Erickson, Ryan, & De Young, Citation2002). However, which visual characteristics of the landscape determine landscape aesthetics is not known (Arnberger & Eder, Citation2011a; Ode, Fry, Tveit, Messager, & Miller, Citation2009). Therefore, we intended with this empirical study to investigate the link between landscape characteristics (hereafter attributes) and people’s perception.
Several theories investigate explanatory factors for the individual perception and preference of landscapes. The evolution theory, for example, argues that based on a common evolutionary background, there is a common preference or aversion for landscape features based on innate, biological reasons; see, for example, the prospect-refuge theory (Appleton, Citation1975) or the information processing theory (Kaplan & Kaplan, Citation1989). Other theories highlight that landscape perception and preferences are shaped by learned behaviour and people’s cultural background (Zube, Sell, & Taylor, Citation1982). It is most likely that both evolutionary and cultural background shape landscape preferences (Arnberger & Eder, Citation2011a; Tveit, Ode, & Fry, Citation2006).
An alternative approach explaining environmental preferences was developed by Tveit et al. (Citation2006) and suggests a range of visual indicators that are based on landscape aesthetic theory and can be used to assess the visual character of landscapes. They propose nine visual concepts; of which, complexity represents a key concept of visual quality and emerges as one important factor in the explanation of landscape preferences (Kaplan & Kaplan, Citation1989; Ode & Miller, Citation2011). Complexity indicators, which refer to the diversity and richness of landscape elements, are described by the density of landscape elements and attributes (number of landscape elements) and the perceived degree of landscape complexity. Following Tveit et al. (Citation2006), we applied the concept of complexity to assess the dimension of diversity using landscape attributes such as linear features, point features and land cover.
Economic valuation of non-marketed goods can help identify which landscape attributes foster the cultural function of agricultural landscapes (van Berkel & Verburg, Citation2014; Koetse, Brouwer, & van Beukering, Citation2015). One of the most commonly used methods is the analysis of stated preferences, also referred to as choice experiment. This method is rooted in traditional microeconomics theories of consumer behaviour, marketing and preference theory and is used to estimate attribute utilities based on an individual’s response to combinations of multiple decision attributes (Louviere, Hensher, & Swait, Citation2000).
Several studies applied visual approaches to evaluate landscape preferences. Some have employed visual stimuli to illustrate attributes or scenarios (Dachary-Bernard & Rambonilaza, Citation2012; Grammatikopoulou, Pouta, Salmiovirta, & Soini, Citation2012), and others have used photographs of landscape elements or entire landscapes (Dramstad, Tveit, Fjellstad, & Fry, Citation2006). In other cases, photorealistic montages of aerial pictures or landscape images (Arnberger & Eder, Citation2011a; van Berkel & Verburg, Citation2014) were used. The digitally calibrated image method (Orland, Daniel, & Haider, Citation1994) as presented in Arnberger and Eder (Citation2011a) is particularly suitable for landscape preference analyses because all image content is under control, and all randomness that could occur is excluded.
In landscape preference studies, a multitude of attributes have been tested. These include elements, such as the presence of water courses (Arriaza, Cañas-Ortega, Cañas-Madueño, & Ruiz-Aviles, Citation2004; Swanwick, Citation2009), and man-made attributes like farm buildings or cultural buildings (Dachary-Bernard & Rambonilaza, Citation2012), which are more or less independent from agricultural management practice. Other attributes, such as visibility of grazing animals (Grammatikopoulou et al., Citation2012), number of land use types, patches and diversity (Dramstad et al., Citation2006) or hedgerows, tree lines and woodland (van Berkel & Verburg, Citation2014; Rambonilaza & Dachary-Bernard, Citation2007), however, are directly linked to farming practice. van Zanten, Verburg, Koetse and van Beukering (Citation2014) provide a comprehensive meta-analysis of stated-preference studies in this regard.
Several studies have suggested that socio-economic, demographic or cultural characteristics such as age, gender, education or the level of familiarity with the landscape, all influence preferences (Arnberger & Eder, Citation2011a, 2011b; Kaplan & Kaplan, Citation1989; Ode et al., Citation2009; Swanwick, Citation2009). Though these studies investigated characteristics that account for heterogeneity among respondents’ responses, many concluded that their findings were insufficient (Dachary-Bernard & Rambonilaza, Citation2012; Dramstad et al., Citation2006), and further examination of sociocultural characteristics of respondents is necessary to improve understanding of how individual landscape preferences are affected (Swanwick, Citation2009).
Several of the existing landscape preference studies have either been applied to natural amenity-rich landscapes (Ode et al., Citation2009), to forest landscapes (Soliva, Bolliger, & Hunziker, Citation2010), to beaches (Stewart, Larkin, Orland, & Anderson, Citation2003) or to urban green spaces (Arnberger & Eder, Citation2011b; Sevenant & Antrop, Citation2010). However, the aesthetic value of agricultural landscapes is important, as well, and through its structure and composition, it is related to agricultural management practices, that is, cropping pattern, hedges or tree rows.
Our main objective is to assess landscape preferences in an agricultural landscape from the point of view of aesthetics. More specifically, we investigate which landscape attributes enable the agricultural landscape to provide aesthetic values to landscape users, both residents and visitors. Hence, the objective is to empirically explore the relationship between attributes of land use (and its spatial structure) and the aesthetic enjoyment different groups in society derived from it. By using a visual choice experiment approach, we aimed to answer the three following questions: (1) What is the level of preference for the different landscape attributes in the case study region (CSR)? (2) Is there agreement among respondents or substantial preference heterogeneity? (3) Which sociocultural characteristics of respondents determine landscape preferences and explain preference heterogeneity?
2. Material and methods
2.1. Case study region
The CSR Märkische Schweiz, with a total size of 576.4 km², is located in North-east Germany (see Figure ). It encompasses ten municipalities with a total population of about 46,500 (Amt für Statistik Berlin-Brandenburg, Citation2012). The area is located in the vicinity of Berlin, which is important to the region’s socio-economic viability. Many people commute between Berlin and the region. Local tourism, an important economic sector along with agricultural production, is mainly based on day-trip visitors from the city.
Figure 1. Location of the CSR Märkische Schweiz (left) with the Federal State Brandenburg (light red) and the County Märkisch Oderland (red) and land cover (right). Survey locations are marked as stars.
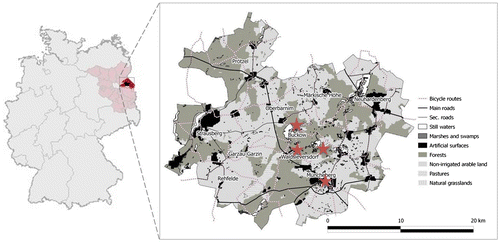
The young moraine landscape is characterised by a mosaic-like, semi-open structure of forests and farmland. Very typical landforms are kettle holes (German: Sölle), which are small ponds that are often surrounded by riverine vegetation. According to the Corine Land Cover (EEA, Citation2007), the share of agricultural area, forests and pastures is about 45, 40 and 5%, respectively. The core part of the CSR is under environmental protection (Nature Park, 205 km²). According to the field block cadastre (MIL, Citation2012), the agricultural landscape is characterised by large farm sizes (average 229 ha per farm holding). Field sizes range from .01 to 353 ha with an average field size of 22 ha (median = 5 ha). This has a strong impact on landscape structures and elements (Ungaro, Zasada, & Piorr, Citation2014).
2.2. Choice experiment design
2.2.1. Selection of landscape attributes
For the reason that this study specifically focuses on the appearance of agricultural landscapes, only those attributes that directly relate to agricultural management were selected for the choice experiment. These landscape elements include green landscape elements like tree rows and hedges near roads and along field margins (1—linear green elements) and individual trees as well as groups of trees located within fields or riparian vegetation around kettle holes (2—point green elements). The diversity of cultivated crops influences the landscape’s appearance because the visual quality of the cropping pattern depends on field size (3—crop diversity). Another attribute that we have included in the preference study concerns the visibility of grazing livestock on grassland (4—livestock).
The four selected landscape attributes of this study were differentiated into three levels (low, medium and high) and two levels (present and not present) for livestock. The intermediate level was considered to represent the current state of landscape attributes in the region. The lowest levels represent the amount of landscape attributes under a future scenario of field enlargement, vanishing landscape elements and intense agriculture. The highest levels represent an abundance of landscape attributes under a future scenario of small-scale practice and sustainable (ecological) extensive farming. Attribute levels and their respective representations are based on their occurrence in the landscape context and derived from extensive land analysis and discussions with local experts and stakeholders from the field of landscape care, environmental science and agriculture. Table provides an overview of the selected landscape attributes and their levels of representation.
Table 1. Description of the representation of landscape attribute levels in the visualisations.
2.2.2. Image visualisation
The four selected landscape attributes with each of the attribute levels were depicted visually. In contrast to other visual landscape preference studies, that have used real photographs, the particular value of developing digitally calibrated images was to control the image content (Orland et al., Citation1994). A basic landscape that was repeated in all of the pictures was designed to include the main characteristics of the region by showing a ground-moraine landscape with a village and forests in the background. Then, the different attribute levels were inserted into this basic design, the low and medium level in the back‐ and middle ground and the high level in the fore‐, middle and background. As a result, 54 photorealistic landscape visualisations combining four different landscape attributes were created and pretested for representativeness with local experts and stakeholders from the field of landscape care, environmental science and agriculture. Figure represents examples of the developed images.
Figure 2. Developed base landscape with (a) all attributes set at level 1; (b) all attributes set at level 2, except for livestock at level 1; (c) linear and point elements set at level 3, crop div. at level 2 and livestock at level 1; and (d) all attributes set at their highest level (3 or 2, respectively).
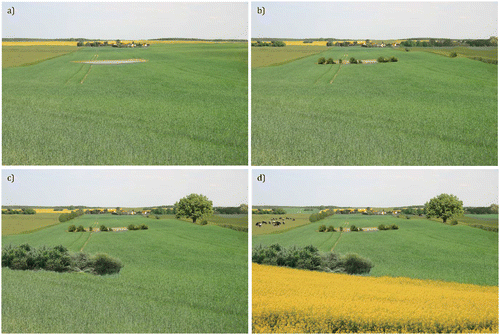
2.2.3. Efficient design
We conducted a pretest (N = 34) to reduce the number of alternatives and choice sets. A Multinomial Logit (MNL) model was estimated using NLogit discrete choice software.Footnote1 The results were used as priors in generating an efficient statistical design using the Ngene software package.Footnote2 The design contains six versions of questionnaires, each with eight choice sets and three landscape images per choice set. The pretest was also applied to ensure visualisation comprehensibility and readability.
2.3. Survey
The main survey was carried out in July 2013 in face-to-face interviews at different spots within the CSR, that is, in the train commuting between Berlin and stations in the Märkische Schweiz, at local festivals, on the beach of a protected lake close to a camping site and in the palace garden in Buckow (see also Figure ). We focused on tourist spots, because at other locations, including towns, the weekly market, or the tourist information, almost no participants could be found due to infrequent visitation. Almost all of the people who were approached and shown the pictures chose to participate in the survey, which highlights the positive effect of the images to trigger interest and enhance participation. In total, 200 people were interviewed.
After respondents were asked if they would participate in a survey about landscape attractiveness (10 min), they were shown a choice set, consisting of three large printed landscape visualisations, all visible at the same time, and were asked which of these three landscape views they preferred from an aesthetic point of view (see Figure ). This was repeated eight times per person. If the respondents were very interested, we provided information on the underlying assumptions or the context of the study after the survey was complete so that respondents were not influenced in their decisions, for example, through giving them a feeling of in-/correct answers.
To obtain variables that explained individual landscape preference heterogeneity, the questionnaire was structured into an initial part that contained the landscape images and a second part that focused on the demographic, sociocultural and attitudinal background of the participant (see Table ).
Table 2. Sociocultural characteristics of respondents.
The origin (visitor/resident), gender and age ranges were evenly distributed. The age of respondents ranged from 6 to 84 years with an average of 46 years (N = 200). In comparison, the average age in the CSR was 45 (Statistische Ämter des Bundes und der Länder, Citation2011). In addition, 44% of respondents had a college or university degree, which is considerably higher than the German average of 14% (Statistisches Bundesamt, Citation2014). A majority of all respondents grew up in the surrounding region (59% in Berlin or Brandenburg). Most of the visitors had been in the region before (82%), many of them more than five times previously (43%).
2.4. Statistical analysis
2.4.1. Preference analysis—Multinomial Logit (MNL) model
A stated-choice approach was used to analyse the trade-off behaviour of visitors and Märkische Schweiz residents. In stated-choice experiments, alternatives are defined as combinations of attributes (Louviere et al., Citation2000). The advantage is that each alternative is evaluated as a whole, and the choices can be modelled as a function of the attributes of the alternatives (McFadden, Citation1974). In a discrete choice experiment, the decision-maker faces a set of alternatives from which the preferred alternative is chosen. The choice made is affected by the observable influences and by unobservable characteristics of the decision-maker. Therefore, following random utility theory, overall utility (Ui) that a person derives from a chosen alternative in a given choice set contains a deterministic component (Vi) and a stochastic component (εi). The overall utility of alternative i is represented as (McFadden, Citation1974):(1)
It is assumed that individuals will choose the alternative that yields the highest utility for them and will choose alternative i over any other alternative j only if the utility Ui is greater than the utility of any alternative Uj.
The MNL model is the most standard discrete choice model and is specified as (Louviere et al., Citation2000):(2)
In the model, the probability Pi that alternative i is chosen among j alternatives is modelled as the exponent of systematic utility associated with alternative i (eVi) divided by the sum of exponents of systematic utility of all alternatives j (∑eVj), that is, including alternative i. The regression estimates (usually referred to as part-worth utilities) in V are estimated through maximum likelihood analysis. In our MNL model, each attribute level was dummy coded (0 = absent and 1 = present) to test for possible nonlinear relationships between attribute levels and utility, and the minimum levels of all attributes are included as the reference category. Considering the four attributes in this study, the resulting model specification for the deterministic component V associated with alternative j is given by:(3)
with LIV2 as a dummy variable for the presence of livestock, CD2 and CD3 as dummy variables for medium and high level of crop diversity, LE2 and LE3 as dummy variables for a medium and high level of linear elements, PE2 and PE3 as dummy variables for a medium and high level of point elements and β1 to β7 are the utility parameters of the model and are estimated as coefficients. A higher utility parameter or coefficient corresponds to higher utility and, therefore, higher preference for an attribute level.
2.4.2. Analysis of the extent of agreement among respondents—Latent Class Analysis
The extent of agreement among respondents and potential heterogeneity among respondents’ preferences were examined by using a Latent Class Analysis (LCA) modelling approach. The basic assumption of this model is that the observed cases can be assigned to homogeneous preference groups, which are referred to as classes, and that heterogeneity of the respondents can be represented by a finite number of classes (Kamakura & Russell, Citation1989).
In this study, models were estimated without restrictions, which means that all of the parameters could differ across the classes and were not restricted or fixed. Several models with two, three or more classes were estimated, and the model with the minimum Bayesian Information Criterion (BIC) was chosen. A latent class probability was estimated for each class and given the likelihood of belonging to one class. A p-value was also estimated as a criterion for this probability in terms of how plausible the estimation of that probability was. As a result, a model with three classes was assumed plausible for the data in this study.
2.4.3. Influence of sociocultural variables on preferences—model with interactions
We interacted the dummy-coded sociocultural variables (see Table ) with the dummy-coded landscape attributes and again estimate an MNL model. However, including all of the explanatory variables in the model would make it very large (13 person-related categories interacting with seven landscape-attribute levels = 91 interactions) and, therefore, unstable. We therefore excluded those interactions between sociocultural variables and choice attributes that do not have statistically significant coefficients. For this, a stepwise removal of the interactions with the highest p-values was carried out until all interactions were statistically significant at a 10% significance level.
3. Results
3.1. Preference for attributes
The results of the MNL model estimation are summarised in Figure . All utility coefficients show a positive relation between medium/high levels of the considered attributes and the probability of choice. Therefore, the presence of all landscape attributes in the choice experiment was evaluated positively. All coefficients are statistically significant at a 1% level except for medium crop diversity, which was significant at a 10% level.
Figure 4. Multinomial logit model estimations with bars for 95%-confidence interval; higher coefficients correspond to higher preference.
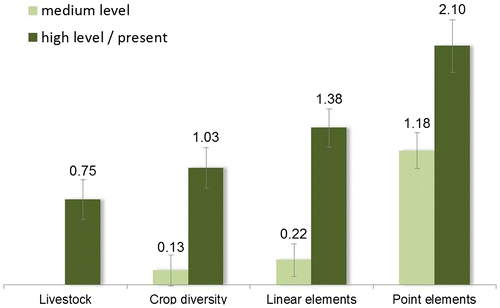
The ranks of the coefficients indicate a strong preference for a high level of point elements, which was by far the most preferred attribute, followed by a high level of linear elements, a medium level of point elements and a high level of crop diversity. Medium levels of linear elements, crop diversity and the presence of livestock are less preferred.
3.2. Analysis of the extent of agreement among respondents
The analysis of the extent of agreement among respondents was carried out using an LCA. The choice of how many classes to include in the model was based on the BIC-value as a statistical criterion, which had a minimum (1.725) for the three-class model. The results are presented in Table . The probability of belonging to one of the three classes is almost evenly distributed with a latent class probability of about 33% per class. The first class of respondents has a strong preference for high crop diversity and a high level of linear elements, which rank first. The second class is characterised by aversion to crop diversity and linear elements. People belonging to this class do not favour a more structured and diverse landscape. Only point elements and livestock are preferred but do not add as much value to the landscape as in the other classes. The third class has strong preferences for high levels of point and linear elements. Accordingly, a majority of about 70% of respondents favour higher attribute levels, and 30% form a class with opposing preferences. Landscape attributes that are preferred across all three classes, and hence reach wide agreement, are point elements and livestock.
Table 3. Results of the Latent Class Analysis with three classes.
3.3. Sociocultural influence on preferences
Some sociocultural variables had a significant influence on at least one of the attribute levels and showed explanatory character. According to the estimation of the model with interactions, those variables are: gender, age, connection to farming, education, value setting, conveyance and activity. Variables that did not have a significant influence on at least one of the attribute levels are: being a visitor or resident, frequency of visiting the landscape, origin (Eastern/Western Germany, urban/rural and lowland/mountainous), or being a member of an association as a proxy for social commitment. The results of the restricted model (after stepwise removal of insignificant interactions) are presented in Table .
Table 4. Results of the restricted model with interactions showing the effects of individual sociocultural background variables on preferences for landscape attributes.
The sociocultural variables have a different degree of influence on the attribute levels. The education variable has the greatest influence on the preference of landscape attributes with significant interactions with all the attribute levels except for livestock. In contrast, connection to agriculture interacts only with one attribute level, namely high level of crop diversity.
All sociocultural variables included in the restricted model show a positive effect on the considered attributes and the probability of choice except for gender and age, for which negative coefficients can be observed for single attribute levels. Females have significantly higher preferences for livestock and for a medium level of crop diversity and less preference for high levels of linear and point elements than men. Older respondents favoured livestock more than young respondents. A high level of crop diversity is preferred more by people with closer connections to farming and people voting for either the Green or Christian Democratic Party (conservative).
4. Discussion
4.1. Landscape attribute preferences
A Multinomial Logit (MNL) model was used to analyse the preferences for landscape attributes, revealing that a diverse and structured landscape with all of the attributes at a high level was the most preferred. This was expected and substantiated previous research findings. There is evidence in various regional contexts that linear elements and crop diversity contribute to landscape attractiveness (van Berkel & Verburg, Citation2014; Dramstad et al., Citation2006; Rambonilaza & Dachary-Bernard, Citation2007). This is confirmed to some extent by our study as they are ranked second and third. However, our findings clearly indicate the main importance of point elements for the visual quality of the landscape, which ranked highest. Point elements, such as the vegetation around kettle holes or the single tree within the fields, outvalue other attributes and require priority in visual-preference-oriented landscape management. This finding, although it might be region-specific, is important to acknowledge for the reason that this attribute was rarely investigated before. A meta-analysis by van Zanten et al. (Citation2014) identified six studies only (out of 66) that addressed point elements at all. Future studies should investigate the contribution of point elements to the landscape attractiveness more extensively.
Preferences for grazing livestock proved to be low in our study in contrast to the findings in other contexts (e.g. Grammatikopoulou et al., Citation2012; van Zanten et al., Citation2016), where it was one of the most preferred attributes. Though grazing cattle represent a traditional feature of the agricultural landscape, they are increasingly kept in stables and are therefore less evident now than in the past, which, in turn, influences the appearance of landscape that people are familiar with.
Preference patterns for different levels of the same attribute are particularly interesting. Though the additional contribution to utility of the medium levels (the status quo situation) for the crop diversity and linear elements attributes is rather marginal, the added value of the highest levels is much more pronounced. This is consistent with the findings of Rambonilaza and Dachary-Bernard (Citation2007) who showed a significant positive effect for the highest level of linear elements only, which was hedgerows in their case. They highlighted the need for highest element density to make a substantial contribution to landscape attractiveness.
In contrast, for point elements, the medium level already provides a substantial contribution to people’s landscape appreciation, which suggests high sensitivity to any disappearance of those elements, which may occur through the intensification of agricultural management practices. The results support the argument that landscape structures and element-richness increase the attractiveness of the region as a whole.
4.2. Homogeneity and heterogeneity of landscape preferences
Groups of respondents were distinguished by applying an LCA. LCA is a tool to study the extent of agreement among respondents; metrics with similar purposes exist in landscape research, such as the inter-rater reliability statistics (e.g. de la Fuente de Val, Atauri, & de Lucio, Citation2006; Palmer, Citation2000). LCA models have not been applied frequently in visual landscape preference studies (e.g. Arnberger & Eder, Citation2011a; Grammatikopoulou et al., Citation2012). Our analysis has identified three nearly equally sized classes of respondents who were characterised by specific landscape preferences. Wide agreement was found for the two landscape attributes point elements and livestock that are preferred across all three classes.
Two groups representing about 70% of respondents have a similar structure of preference and showed clear preference patterns for crop diversity, point elements and linear elements, while one group encompassing nearly one-third of all respondents displayed no effect or even negative associations with medium and high attribute levels—apparently preferring ‘cleared-out’ landscapes with very low degrees of complexity. Arnberger and Eder (Citation2011a) and Grammatikopoulou et al. (Citation2012) also found this phenomenon, which was less pronounced, with only 9% and 21% of respondents being in favour of lower attribute levels, respectively. The two aspects of the landscape that will cause respondents to fall into groups with distinctly different opinions are linear elements such as hedgerows and alleys, as well as crop diversity. However, landscape preference patterns are variable between individuals and societal groups, implying heterogeneity of opinions about the ideal aesthetic landscape, which has also been shown in previous LCA studies of agricultural landscapes (e.g. Arnberger & Eder, Citation2011a; Grammatikopoulou et al., Citation2012; Sevenant & Antrop, Citation2010). This finding reconfirms that user perspective should be acknowledged when attempting to provide natural amenities and CES as part of a landscape management approach. It should be considered that by implementing the average preference, some groups may be overlooked in terms of what they prefer.
4.3. Influence of sociocultural characteristics
Respondent variables were found to have significant influences on landscape preferences, primarily education, gender and age. Individual attributes such as political opinion, preferred outdoor activity and means of transport were found to be significantly influential. The analysis suggested an increased (positive) sensitivity among specific respondent groups, particularly women, university graduates and people with a pronounced environmental value setting, who showed an increased preference for an element-rich and diverse landscape. It has been argued, for instance, that women more frequently connect to nature concerns and the concept of sustainability (Kleinhückelkotten & Neitzke, Citation2011). In contrast, familiarity with the landscape (Soini, Vaarala, & Pouta, Citation2012) and a relationship to agriculture (Howley, Citation2011) or the place of origin (Adevi & Grahn, Citation2012) were not confirmed to have a relevant effect on preference. The absence of distinct preference patterns between urban (visitors) and rural (inhabitants) users of the landscape was unexpected. Van den Berg and Koole (Citation2006) presumed landscape preference differences between rural and urban residents as a result of the socio-economic composition of both groups and their different direct experience with the managed, local landscape. One could argue that no difference was found because many urban dwellers have rural origins and reveal preferences accordingly. Otherwise, the marginal differences between visitors and residents can be traced back to the common preferences found because structured and diverse landscapes are highly appreciated by tourists and valued as an integral part of the regional identity by residents.
4.4. Methodological considerations and limitations
This visual-preference study was conducted as a discrete choice experiment with digitally calibrated images of an agricultural landscape that was used to assess the landscape preferences of visitors and residents. Visual choice methods using real photographs often lack accuracy in their interpretation because of the difficulties of representing the attributes at different levels within the image (Ode et al., Citation2009). The application of digitally calibrated images allows a high degree of control (constancy and change) of what is actually shown in the picture, improving the reliability of results.
However, even in digitally created images, the type and size of the landscape-attribute representation remains critical to the preference choice, and both need to be taken into consideration when interpreting the results. For instance, other elements in the image that are not subject to the study may affect the appearance of attributes of interest, which can disturb the results. The method is also limited by not considering the quality of the visual representation of landscape attributes, such as the appearance of the deciduous tree or the yellow rape field. A coniferous tree, a field colour with less contrast or a hedgerow that is flowering may have elicited different responses. Additionally, results observed might be caused by insufficient differences in the attribute visualisations, especially for medium levels. It should be further elaborated how visualisation and the reliability of results in visual choice experiments can be improved.
Furthermore, it is noteworthy that landscape preferences are place-specific and vary across different regions (e.g. van Zanten et al., Citation2016). They are also dependent on other territorial assets of the particular area that are either naturally given (such as topography or water courses) or are not subject to agricultural management (forests and built-up structures). Also, the attractiveness of a landscape is not just dependent on the scenery, since many people may appreciate it for the tranquillity, open space or fresh air (Swanwick, Citation2009), for example.
4.5. Relevance for policy and planning
Both agricultural production and rural tourism traditionally play an important economic role in the CSR with its heterogeneous and natural amenity-rich landscape. However, modernisation and ownership consolidation has led to the scale enlargement of agricultural fields and the intensification of agricultural practices (Tietz, Forstner, & Weingarten, Citation2013), which is a main factor behind vanishing landscape structures and elements (Ungaro et al., Citation2014). According to the findings of this study, field enlargement and the linked clearance of landscape elements would negatively affect the aesthetic value of the landscape. As a result, the conservation of existing hedges and tree rows and landscape management efforts for other attributes such as trees and kettle hole vegetation is needed. Priority could be given to attributes on which most people agree, in our case point elements and the presence of livestock, as there is only one landscape to be planned. The methodology used here can help to facilitate regional discussions about future land-use changes and landscape development and support prioritisation of management efforts.
Agri-environmental measures play a role in sustaining and restoring ecosystem services as a local asset formation, and thus, the socio-economic situation as a whole requires more attention in rural development policy. New social and environmental landscapes take shape, a process that has been coined as ‘social landscape of agro-ecological improvement’ (Marsden & Smith, Citation2005). Taking aesthetic values into account, this CES-oriented landscape management approach specifically applies to the design, targeting and evaluation of measures. Organic management measures and ecological focus areas of the European agri-environmental policy support already an opportunity to provide landscape features like buffer strips or afforested areas and improve the crop diversity in a non-targeted way.
5. Conclusion
A stated-choice experiment that used digitally calibrated images was carried out among 200 residents and visitors to analyse the preferences of four different landscape attributes. A general preference for high attribute levels was determined, with the highest general preference for green point and linear elements. Crop diversity and the presence of grazing livestock were found to be less important for the visual landscape value.
We identified three homogenous groups with a distinct preference pattern. While two of the groups had a general preference for medium and high levels of some of the attributes, nearly 30% of all of the respondents preferred low attribute levels instead. Regarding the sociocultural characteristics of respondents, we observed that education, gender and age specifically influenced preferences. Remarkably, no significant differences could be found between residents and visitors of the region.
These results give a first indication how local landscape management from an aesthetic value perspective could be used for the identification of priority landscape measures and areas, and hence support multi-objective targeting of policies.
Funding
This work was supported by the European Commission.
Disclosure statement
No potential conflict of interest was reported by the authors.
Notes
References
- Adevi, A.A., & Grahn, P. (2012). Preferences for Landscapes: A matter of cultural determinants or innate reflexes that point to our evolutionary background? Landscape Research, 37(1), 27–49. doi:10.1080/01426397.2011.576884
- Amt für Statistik Berlin-Brandenburg. (2012). Bevölkerung im Land Brandenburg nach amtsfreien Gemeinden, Ämtern und Gemeinden 31.Dezember 2012 (XLS-file; 83 KB) [Population in the state of Brandenburg after independent municipalities, offices and municipalities 31 December 2012].
- Appleton, J. (1975). The experience of landscapes. New York, NY: Wiley.
- Arnberger, A., & Eder, R. (2011a). Exploring the heterogeneity of rural landscape preferences: An image-based latent class approach. Landscape Research, 36(1), 19–40. doi:10.1080/01426397.2010.536204
- Arnberger, A., & Eder, R. (2011b). The influence of age on recreational trail preferences of urban green-space visitors: A discrete choice experiment with digitally calibrated images. Journal of Environmental Planning and Management, 54(7), 891–908. doi:10.1080/09640568.2010.539875
- Arriaza, M., Cañas-Ortega, J. F., Cañas-Madueño, J. A., & Ruiz-Aviles, P. (2004). Assessing the visual quality of rural landscapes. Landscape and Urban Planning, 69(1), 115–125. doi:10.1016/j.landurbplan.2003.10.029
- van Berkel, D. B., & Verburg, P. H. (2014). Spatial quantification and valuation of cultural ecosystem services in an agricultural landscape. Ecological Indicators, 37(PART A), 163–174. doi:10.1016/j.ecolind.2012.06.025
- Bieling, C., & Plieninger, T. (2013). Recording manifestations of cultural ecosystem services in the landscape. Landscape Research, 38(5), 649–667. doi:10.1080/01426397.2012.691469
- Chan, K. M. A., Guerry, A. D., Balvanera, P., Klain, S., Satterfield, T., Basurto, X., … Tam, J. (2012). Where are cultural and social in ecosystem services? A Framework for Constructive Engagement. BioScience, 62(8), 744–756. doi:10.1525/bio.2012.62.8.7
- Dachary-Bernard, J., & Rambonilaza, T. (2012). Choice experiment, multiple programmes contingent valuation and landscape preferences: How can we support the land use decision making process? Land Use Policy, 29(4), 846–854. doi:10.1016/j.landusepol.2012.01.002
- Dramstad, W. E., Tveit, M. S., Fjellstad, W. J., & Fry, G. L. A. (2006). Relationships between visual landscape preferences and map-based indicators of landscape structure. Landscape and Urban Planning, 78(4), 465–474. doi:10.1016/j.landurbplan.2005.12.006
- EEA. (2007). CLC2006 technical guidelines. Copenhagen:Author.
- Erickson, D. L., Ryan, R. L., & De Young, R. (2002). Woodlots in the rural landscape: Landowner motivations and management attitudes in a Michigan (USA) case study. Landscape and Urban Planning, 58(2–4), 101–112. doi:10.1016/S0169-2046(01)00213-4
- de la Fuente de Val, G., Atauri, J. A., & de Lucio, J. V. (2006). Relationship between landscape visual attributes and spatial pattern indices: A test study in Mediterranean-climate landscapes. Landscape and Urban Planning, 77(4), 393–407. doi:10.1016/j.landurbplan.2005.05.003
- Grammatikopoulou, I., Pouta, E., Salmiovirta, M., & Soini, K. (2012). Heterogeneous preferences for agricultural landscape improvements in southern Finland. Landscape and Urban Planning, 107(2), 181–191. doi:10.1016/j.landurbplan.2012.06.001
- Howley, P. (2011). Landscape aesthetics: Assessing the general publics’ preferences towards rural landscapes. Ecological Economics, 72, 161–169. doi:10.1016/j.ecolecon.2011.09.026
- Kamakura, W. A., & Russell, G. J. (1989). A probabilistic choice model for market segmentation and elasticity structure. Journal of Marketing Research, 26, 379–390.10.2307/3172759
- Kaplan, R., & Kaplan, S. (1989). The experience of nature: A psychological perspective. Cambridge: Cambridge University Press.
- Kleinhückelkotten, S., & Neitzke, H. (2011). Nature awareness study. Hanover. Retrieved from http://www.bfn.de/fileadmin/MDB/documents/themen/gesellschaft/Naturbewusstsein_2011/2011_Nature-Awareness-Study_bf.pdf
- Koetse, M. J., Brouwer, R., & van Beukering, P. J. H. (2015). Economic valuation methods for ecosystem services. In J. A. Bouma & P. J. H. van Beukering (Eds.), Ecosystem services: From concept to practice (pp. 108–131). Cambridge: Cambridge University Press.10.1017/CBO9781107477612
- Louviere, J. J., Hensher, D. A., & Swait, J. D. (2000). Stated choice methods: Analysis and applications. Cambridge; University Press. doi:10.1002/jae.701
- Marsden, T., & Smith, E. (2005). Ecological entrepreneurship: Sustainable development in local communities through quality food production and local branding. Geoforum, 36(4), 440–451. doi:10.1016/j.geoforum.2004.07.008
- McFadden, D. (1974). Conditional logit analysis of qualitative choice behavior. In P. Zarembka (Ed.), Frontiers in econometrics (p. 105:142). New York, NY: Academic Press. Retrieved from http://elsa.berkeley.edu/pub/reprints/mcfadden/zarembka.pdf
- MIL. (2012). Digitales Feldblockkataster (DFBK) Berlin und Brandenburg. geobroker- Landesvermessung und Geobasisinformation Brandenburg (LGB). Retrieved from http://www.mil.brandenburg.de/cms/detail.php/bb1.c.223513.de
- Millennium Ecosystem Assessment. (2005). Ecosystems and human well-being: Synthesis. Washington, DC: Island Press. doi:10.1196/annals.1439.003
- Ode, A., & Miller, D. (2011). Analysing the relationship between indicators of landscape complexity and preference. Environment and Planning B: Planning and Design, 38(1), 24–40. doi:10.1068/b35084
- Ode, A., Fry, G., Tveit, M. S., Messager, P., & Miller, D. (2009). Indicators of perceived naturalness as drivers of landscape preference. Journal of Environmental Management, 90(1), 375–83. doi:10.1016/j.jenvman.2007.10.013
- Orland, B., Daniel, T. C., & Haider, W. (1994). Calibrated images: Landscape visualizations to meet rigorous experimental design specification. In Proceedings of Decision Support 2001—Resource Technology 94. American Society for Photogrammetry and Remote Sensing (pp. 919–926), Washington, DC
- Palmer, J. F. (2000). Reliability of rating visible landscape qualities. Landscape Journal, 19(1–2), 166–178. doi:10.3368/lj.19.1-2.166
- Plieninger, T., Dijks, S., Oteros-Rozas, E., & Bieling, C. (2013). Assessing, mapping, and quantifying cultural ecosystem services at community level. Land Use Policy, 33, 118–129. doi:10.1016/j.landusepol.2012.12.013
- Rambonilaza, M., & Dachary-Bernard, J. (2007). Land-use planning and public preferences: What can we learn from choice experiment method? Landscape and Urban Planning, 83(4), 318–326. doi:10.1016/j.landurbplan.2007.05.013
- Sevenant, M., & Antrop, M. (2010). The use of latent classes to identify individual differences in the importance of landscape dimensions for aesthetic preference. Land Use Policy, 27(3), 827–842. doi:10.1016/j.landusepol.2009.11.002
- Soini, K., Vaarala, H., & Pouta, E. (2012). Residents’ sense of place and landscape perceptions at the rural–urban interface. Landscape and Urban Planning, 104(1), 124–134. doi:10.1016/j.landurbplan.2011.10.002
- Soliva, R., Bolliger, J., & Hunziker, M. (2010). Differences in preferences towards potential future landscapes in the swiss alps. Landscape Research, 35(6), 671–696. doi:10.1080/01426397.2010.519436
- Statistische Ämter des Bundes und der Länder. (2011). Statistik lokal—Daten für die Gemeinden, kreisfreien Städte und Kreise Deutschlands [Data for municipalities, independent cities and counties of Germany], [DVD]. Wiesbaden: Statistisches Bundesamt.
- Statistisches Bundesamt. (2014). Bevölkerung nach Bildungsabschluss in Deutschland. Retrieved from https://www.destatis.de/DE/ZahlenFakten/GesellschaftStaat/BildungForschungKultur/Bildungsstand/Tabellen/Bildungsabschluss.html
- Stewart, W., Larkin, K., Orland, B., & Anderson, D. (2003). Boater preferences for beach characteristics downstream from Glen Canyon Dam. Arizona. Journal of Environmental Management, 69(2), 201–211. doi:10.1016/j.jenvman.2003.08.001
- Swanwick, C. (2009). Society’s attitudes to and preferences for land and landscape. Land Use Policy, 26, S62–S75. doi:10.1016/j.landusepol.2009.08.025
- Szücs, L., Anders, U., & Bürger-Arndt, R. (2015). Assessment and illustration of cultural ecosystem services at the local scale—A retrospective trend analysis. Ecological Indicators, 50, 120–134. doi:10.1016/j.ecolind.2014.09.015
- TEEB. (2010). The economics of ecosystems and biodiversity: Mainstreaming the economics of nature: A synthesis of the approach, conclusions and recommendations of TEEB. Environment. Retrieved from http://www.teebweb.org/InformationMaterial/TEEBReports/tabid/1278/Default.aspx
- Tietz, A., Forstner, B., & Weingarten, P. (2013). Non-agricultural and supra-regional investors on the German agricultural land market: An empirical analysis of their significance and impacts. German Journal of Agricultural Economics, 62(2), 86–98.
- Tveit, M., Ode, Å., & Fry, G. (2006). Key concepts in a framework for analysing visual landscape character. Landscape Research, 31(3), 229–255. doi:10.1080/01426390600783269
- Ungaro, F., Zasada, I., & Piorr, A. (2014). Mapping landscape services, spatial synergies and trade-offs. A case study using variogram models and geostatistical simulations in an agrarian landscape in North-East Germany. Ecological Indicators, 46, 367–378. doi:10.1016/j.ecolind.2014.06.039
- Van den Berg, A. E., & Koole, S. L. (2006). New wilderness in the Netherlands: An investigation of visual preferences for nature development landscapes. Landscape and Urban Planning, 78(4), 362–372. doi:10.1016/j.landurbplan.2005.11.006
- van Zanten, B. T., Verburg, P. H., Koetse, M. J., & van Beukering, P. J. H. (2014). Preferences for European agrarian landscapes: A meta-analysis of case studies. Landscape and Urban Planning, 132, 89–101. doi:10.1016/j.landurbplan.2014.08.012
- van Zanten, B. T., Zasada, I., Koetse, M. J., Ungaro, F., Häfner, K., & Verburg, P. H. (2016). A comparative approach to assess the contribution of landscape features to aesthetic and recreational values in agricultural landscapes. Ecosystem Services, 17, 87–98. doi:10.1016/j.ecoser.2015.11.011
- Zhang, W., Ricketts, T. H., Kremen, C., Carney, K., & Swinton, S. M. (2007). Ecosystem services and dis-services to agriculture. Ecological Economics, 64(2), 253–260. doi:10.1016/j.ecolecon.2007.02.024
- Zube, E. H., Sell, J. L., & Taylor, J. G. (1982). Landscape perception: Research, application and theory. Landscape Planning, 9(1), 1–33. doi:10.1016/0304-3924(82)90009-0