Abstract
Understorey vegetation is a critical component of biodiversity and an essential habitat component for many wildlife species. However, compared to overstorey, information about understorey vegetation distribution is scant, available mainly over small areas or through imprecise large area maps from tedious and time-consuming field surveys. A practical approach to classifying understorey vegetation from remote sensing data is needed for more accurate habitat analyses and biodiversity estimates. As a case study, we mapped the spatial distribution of understorey bamboo in Wolong Nature Reserve (south-western China) using remote sensing data from a leaf-on or growing season. Training on a limited set of ground data and using widely available Landsat TM data as input, a nonlinear artificial neural network achieved a classification accuracy of 80% despite the presence of co-occurring mid-storey and understorey vegetation. These results suggest that the influences of understorey vegetation on remote sensing data are available to practical approaches to classifying understorey vegetation. The success here to map bamboo distribution has important implications for giant panda conservation and provides a good foundation for developing methods to map the spatial distributions of other understorey plant species.
Abstract
Representative schematic of an artificial neural network. The arrows represent a feed-forward process of transforming input data, such as remote sensing imagery, to an output space (e.g. bamboo existence/absence). Networks are trained through a priori knowledge of output and input relations (ground data and corresponding remote sensing pixel values) and a reiterative back-propagation of training errors to update the hidden layer weights.
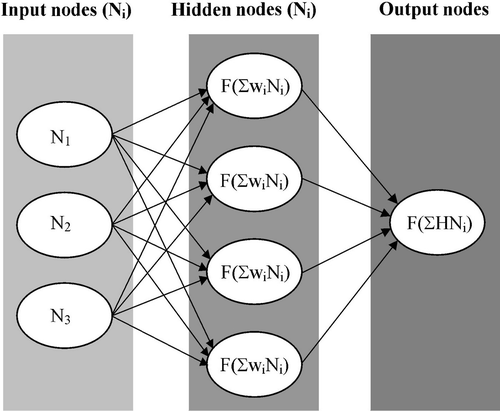
Acknowledgments
We thank the Wolong Nature Reserve Administration, especially Zhang Hemin, for their kind assistance and support of our fieldwork. In addition, we thank Daniel Rutledge and James Brown (Department of Fisheries & Wildlife, Michigan State University) for their technical support. We gratefully acknowledge financial support from the National Aeronautics and Space Administration (NASA Earth Science Fellowship Award), the National Science Foundation (CAREER Award and Biocomplexity Grant), National Institute of Child Health and Human Development (R01 HD39789), American Association for Advancement of Sciences/The John D. and Katherine F. MacArthur Foundation, Michigan State University (All-University Research Initiation Grant, Global Competence Fund, Institute for International Agriculture), the National Natural Science Foundation of China, the Chinese Academy of Sciences, and China Bridges International (North America). We would also like to thank Dr Arthur Roberts and two anonymous reviewers for their constructive comments and suggestions.