Abstract
As an intrinsic property of natural materials, land surface emissivity (LSE) is an important surface parameter and can be derived from the emitted radiance measured from space. Besides radiometric calibration and cloud detection, two main problems need to be resolved to obtain LSE values from space measurements. These problems are often referred to as land surface temperature (LST) and emissivity separation from radiance at ground level and as atmospheric corrections in the literature. To date, many LSE retrieval methods have been proposed with the same goal but different application conditions, advantages, and limitations. The aim of this article is to review these LSE retrieval methods and to provide technical assistance for estimating LSE from space. This article first gives a description of the theoretical basis of LSE measurements and then reviews the published methods. For clarity, we categorize these methods into (1) (semi-)empirical or theoretical methods, (2) multi-channel temperature emissivity separation (TES) methods, and (3) physically based methods (PBMs). This article also discusses the validation methods, which are of importance in verifying the uncertainty and accuracy of retrieved emissivity. Finally, the prospects for further developments are given.
1. Introduction
Land surface emissivity (LSE), as an intrinsic property of natural materials, is often regarded as an indicator of material composition, especially for the silicate minerals, although it varies with viewing angle and surface roughness (Sobrino, Raissouni, and Li Citation2001; Sobrino, Jimenez-Munoz, and Verhoef Citation2005). Therefore, LSE is important not only for studies of soil development and erosion and for estimation of amounts of sparse vegetative cover and changes in this cover, but also for bedrock mapping and resource exploration (Gillespie et al. Citation1998), as well as for the accurate estimates of surface energy budgets (Jin and Liang Citation2006).
Generally speaking, the retrieval of LSE from space is not easy. The direct estimation of LSE from passive satellite measurements is impossible due to the combined effects of the land surface temperature (LST) and LSE or the atmospheric contamination (Li, Petitcolin, and Zhang Citation2000; Jiang, Li, and Nerry Citation2006). In other words, there are at least two problems to be resolved besides the radiometric calibration and cloud detection (Becker and Li Citation1995): (1) a separation of surface emissivity and temperature from radiance at ground level and (2) atmospheric corrections.
First, the problem of underdetermination is troublesome because the problem is mathematically unsolvable even for at-ground radiances. The radiative transfer equation (RTE) shows that the radiance emitted from the surface in the infrared region is a function of its temperature and its emissivity. If the radiance is measured at N wavelengths, there will always be N + 1 unknowns, corresponding to N emissivities at each wavelength and an unknown surface temperature. The solution of a set of underdetermined equations described by measured radiances is the main difficulty in the retrieval of LST and LSE. The coupling of LST and LSE makes the determination of LSE require the simultaneous determination of LST and vice versa (Li and Becker Citation1993; Becker and Li Citation1995; Li, Petitcolin, and Zhang Citation2000).
In addition, atmospheric absorption and emission and surface reflection further complicate the problem. The energy exchange between the land surface and the atmosphere is always mixed in the thermal infrared (TIR) spectral band. On the one hand, the emissivity is not equal to 1 (unity) and causes a reduction of the surface-emitted radiance; on the other hand, it reflects the downwelling of the atmospheric irradiance back into the atmosphere. The anisotropy of reflectivity and emissivity can reduce or increase the total radiance from the surface (Prata Citation1993). Moreover, the surface-leaving radiance is attenuated by the atmosphere before reaching the sensors. Accounting for the compensation of upwelling atmospheric radiance, the atmosphere can also have indeterminate effects on the surface-leaving radiance. Obviously, the combined effect of the non-unity emissivity and the atmosphere increases the complexity of retrieving LST and LSE from the top-of-atmosphere (TOA) measurements (Dash et al. Citation2005). From these points of view, LSE can be considered as a critical variable that must be known a priori to correct surface radiances and retrieve LST. Furthermore, it is the key point for solving the coupling problem of the land surface and the atmosphere.
Attempts to extract the emissivity information from space have been undertaken for several decades, and the remotely sensed data do supply a practicable approach for the investigation of emissivity on a wide spatial and temporal scale. However, because of the inherent physical problems mentioned above, the uncertainty or non-unique solutions appear during the process of LSE retrieval. Fortunately, along with the development of remote sensing, several methods have been proposed for LSE retrieval. The aim of this article is to analyse and compare these LSE retrieval methods and to provide technical assistance when estimating LSE from space in remote sensing. The following sections are organized as follows. In Section 2, some theoretical background is given, including the definition and angular variation of emissivity, which is the basis for retrieving emissivity from space. In Section 3, concrete methods are briefly recalled individually, from empirical methods to physics-based methods. In Section 4, comparison and analysis of different methods are given to assist the selection of methods in various circumstances. In Section 5, a validation method of satellite-derived LSE is proposed to verify the accuracy of the retrieved LSE. Finally, the outlook of this area is given in Section 6.
2. Basic theoretical background
2.1. Definition of emissivity
The emissivity, ϵ, at a given wavelength λ (units, μm) and temperature T (units, K), is defined as the ratio of the radiance emitted by a body at temperature T and the radiance
emitted by a black body at the same temperature T, that is,
It is worth noting that T here is the radiometric temperature, which is also called the skin temperature because it corresponds to the radiation emitted from a depth which is of the order of the penetration depth (Becker and Li Citation1995). For example, the penetration depth is within a few millimetres in the TIR region (Wan Citation1999). Thus, it should be discriminated from other definitions of temperature, e.g. thermodynamic or kinetic temperature (Becker and Li Citation1995; Norman and Becker Citation1995).
Because emissivity varies with wavelength, the channel effective emissivity of a uniform surface at surface temperature T for channel i for a given finite wavelength range from λ1 to λ2 is calculated as (Wan and Dozier Citation1996)
2.1.1. Case of a flat surface
The definition of emissivity mentioned above comes from the homogeneous isothermal surfaces. However, natural surfaces observed from space are usually heterogeneous, especially in the situation of low spatial resolution. The spatial resolution varies from several metres to kilometres, making it hard to find a ‘pure’ and ‘isothermal’ pixel. LST can only be defined without any ambiguity for homogeneous surfaces at thermal equilibrium. Considering the difficulties in relating the radiance of an ensemble of natural media at different temperatures to a black body distribution at the same effective temperature, Norman and Becker (Citation1995) proposed two definitions: the ‘r-emissivity’ and the ‘e-emissivity’ for a flat surface.
1. | r-emissivity For a flat pixel composed of N homogeneous subelements with a given spectral domain and view angle, the ensemble emissivity (or r-emissivity) | ||||
2. | e-emissivity Assuming that there is an equivalent black body, which has an identical temperature distribution with non-isothermal pixels, e-emissivity is defined as the ratio of the radiance from the pixel to that from the equivalent black body for the same spectral domain and view angle: |
2.1.2. Case of a rough surface
Surface emissivity is well defined for a homogeneous surface in thermal equilibrium. However, for heterogeneous and non-isothermal flat surfaces, the definition is not unique. Moreover, these definitions may not hold true for rough systems. Due to the multi-scattering phenomenon, the effective emissivity always increases with the surface roughness. Based on laboratory measurements, Salisbury, Wald, and D'Aria (Citation1994) showed that most common terrestrial surfaces follow Kirchhoff's law. Consequently, Becker and Li (Citation1995) and Chen et al. (Citation2004) defined the directional emissivity in channel i of an opaque medium in thermal equilibrium for a heterogeneous and non-isothermal rough surface from the hemispherical-directional reflectivity (r
h) via the bidirectional reflectivity () of this medium as
One of the advantages of r-emissivity for flat and rough surfaces is its measurability from space and its scale invariability. However, this type of definition makes the definition of LST wavelength and viewing angle dependent and also dependent on the distributions of surface temperature and emissivity within a pixel. As discussed in Becker and Li (Citation1995), the wavelength dependency of LST is negligible in the atmospheric window from 8 to 14 μm, considering the other uncertainties in the LST retrieval from space (Becker and Li Citation1995).
Another definition, called apparent emissivity, was proposed by Li, Strahler, and Friedl (Citation1999a) and Li and Wang (Citation1999) to keep the Planck's function and bidirectional reflectance distribution function (BRDF)-derived emissivity unchanged. This apparent emissivity (ϵapp) is defined by adding an apparent emissivity increment caused by the non-isothermal surface to the r-emissivity to make the definition of LST independent of viewing angle and wavelength:
One should note that all definitions of effective emissivity are ambiguous because both the effective temperature and the effective emissivity are defined only from one ensemble radiance emitted by a heterogeneous flat or rough non-isothermal surface. In fact, there are many possibilities for defining two parameters (LST and ϵ) from only one equation (ensemble radiance); the definition makes sense only if the defined parameters are measurable from space. Considering this criterion, r-emissivity defined by EquationEquations (4)(4) and Equation(6)
(6) for both heterogeneous flat and rough non-isothermal surfaces is recommended for the LST and emissivity retrievals from space measurements.
2.2. Radiative transfer equation (RTE)
To retrieve emissivity from space, both the radiance emitted from a surface and the radiance emitted by a black body at the same temperature need to be known simultaneously. Consequently, the basic theoretical background of radiative transfer should be given first.
On the basis of the radiative transfer theory, under clear-sky conditions, the spectral radiance at the TOA, , along the viewing zenith angle θ and the azimuth angle φ can be written as (Chandrasekhar Citation1960; Lenoble Citation1985):
Obviously, EquationEquation (8)(8) is mathematically unsolvable even if the remaining quantities apart from emissivities and temperature are known. If the radiance is measured at N wavelengths, there will always be N + 1 unknowns, corresponding to the N emissivities (one at each wavelength) and an unknown surface temperature, but only N equations. This fact makes the retrieval process more troublesome.
2.3. Angular variation of emissivity
The observed anisotropy of land emittance is caused by a combination of two different processes: the inherent anisotropic emissivity of terrestrial materials and the thermal heterogeneity of complex, three-dimensional (3D) land targets. Many efforts have been devoted to measure the directional emissivity of soil, leaves, and other natural surfaces. An angular variation of emissivity has been observed by a number of scientists in the field and in the laboratory (Becker, Ramanantsizehena, and Stoll Citation1985; Labed and Stoll Citation1991). Commonly, the decrease in emissivity of natural surfaces is observed with increasing view zenith angle in the TIR (8−4 μm) band (Sobrino and Cuenca Citation1999; Cuenca and Sobrino Citation2004; Hori et al. Citation2006). Experimental results show that different land surface materials have different angular variations in emissivity; for example, arid bare soils and water generally show the highest angular dependence, as depicted in (Snyder et al. Citation1998; Sobrino and Cuenca Citation1999; Cuenca and Sobrino Citation2004). Hori et al. (Citation2006) reported that the angular variations in emissivity for smooth surfaces, such as bare ice, are quite consistent with the spectra predicted by the Fresnel reflectance theory.
Figure 1. Angular variation of absolute emissivity for several natural surfaces (adopted from Sobrino and Cuenca (Citation1999)).
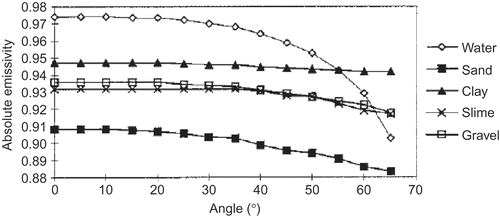
However, this angular behaviour observed at a local scale may not be always consistent with that at a large scale. For example, green sparse shrubs exhibit a significant increase in emissivity away from the nadir (Snyder et al. Citation1998), and the directional canopy emissivity may show different behaviours at large scales due to the decreased proportion of reflective soil in the viewing direction when the viewing zenith angle increases (Sobrino, Jimenez-Munoz, and Verhoef Citation2005). It is worth noting that the structure of surface, which may cause a change in the viewed proportions of the components, can result in a surprisingly large angular dependence of the emissivity even with Lambertian components (Snyder and Wan Citation1998).
Although the angular variation of emissivity may be negligible for most of the surfaces when the view zenith angles are less than 60° (Prata Citation1993), the terrain may exaggerate the impact of view angles because the combination of surface slope and sensor's scan angle may result in local viewing angles greater than 60° (Wan Citation1999).
2.4. Spectral variation of emissivity
2.4.1. Spectral behaviour of rocks
The structures of mineral molecules and the force constants between atoms, as well as the long-range order of crystal lattices contribute to the spectral behaviour of rocks (Farmer Citation1974; Salisbury and D'Aria Citation1992). For typical rocks, the characteristics of spectral emissivity in the TIR are mainly impacted by the aggregate silicon−oxygen stretching vibration bands (reststrahlen bands) of the igneous minerals in broad emissivity troughs for increasingly mafic rock types. Quartz reststrahlen bands, including strong asymmetric stretching fundamentals between 8 and 10 μm and a weaker symmetric stretching fundamental between 12.2 and 13.0 μm, may appear in many rocks. Emissivity of some rocks may be dominated by the carbonate (–CO3) bending reststrahlen band near 11.2 μm. In addition, the maximum emissivity in the spectra of rocks is often associated with a Christiansen feature, which was first described by Conel (Citation1969) and occurs just prior to a fundamental molecular vibration band. In the mid-infrared (MIR) region, the shape of the emissivity spectrum is mainly dominated by quartz, carbonate, tremolite, etc. The recrystallized minerals in metamorphic rocks mean that the emissivity spectrum at 3–5 μm shows greater variability. A more detailed description of the spectral behaviour of rocks and the spectral library can be found in Salisbury and D'Aria (Citation1992) and also in the Advanced Spaceborne Thermal Emission and Reflection Radiometer (ASTER) Spectral Library (http://speclib.jpl.nasa.gov/), which includes contributions from the Jet Propulsion Laboratory (JPL), Johns Hopkins University (JHU), and the United States Geological Survey (USGS) (Baldridge et al. Citation2009).
shows the spectral variation of mean and standard deviation of the emissivity of a number of rocks computed from the ASTER Spectral Library at 3−5 μm and 8−4 μm. The reststrahlen bands of quartz and carbonate can be observed even in the average spectrum. From this figure, one can remark that the smaller the mean emissivity is, the larger the variation in the emissivity is, and vice versa.
2.4.2. Spectral behaviour of soils
Generally, soil composition is often dominated by quartz, which is both a common mineral and resistant to weathering. Thus, the soil spectral behaviours at 3−5 μm and 8−14 μm are both impacted by quartz, which has a strong reststrahlen band between 8 and 10 μm and weaker bands between 12.2 and 13.0 μm and between 4.5 and 4.7 μm. In addition, the carbonate bands are prominent in the MIR region, especially the strongest carbonate doublet near 4.0 μm.
The emissivity spectra of soils are still influenced by organic matter, which may reduce the spectral contrast of the soils and introduce a broad emissivity minimum near 9.6 μm. However, organic matter has a much smaller impact on the MIR region. Finally, soil moisture can increase the emissivity of soils. Soil emissivity increases from 1.7% to 16% when water content becomes higher (Mira et al. Citation2007), and a similar conclusion can be found in many other studies (Urai, Matsunaga, and Ishii Citation1997; Xiao et al. Citation2003; Ogawa, Schmugge, and Rokugawa Citation2006; Mira et al. Citation2010). A more detailed description of the spectral behaviour of soils can be found in Salisbury and D'Aria (Citation1992), in the ASTER Spectral Library (Baldridge et al. Citation2009), and in the Moderate Resolution Imaging Spectroradiometer (MODIS) UCSB (University of California, Santa Barbara) Emissivity Library at http://icess.ucsb.edu/modis/EMIS/html/em.html.
shows the mean value and the standard deviation of the emissivity spectrum of a number of soils computed from the ASTER Spectral Library at 3−5 μm and 8−14 μm. The reststrahlen bands of quartz and carbonate can be clearly observed even in the averaged spectrum.
2.4.3. Spectral behaviour of vegetation
Four emissivity spectra of vegetation from the ASTER Spectral Library are depicted in As shown in this figure, green foliage exhibits low spectral contrast in both MIR and TIR regions, especially conifers, which have a nearly uniformly high emissivity, except for a weak reststrahlen trough near 3.43 and 3.51 μm associated with the hydrogen−carbon vibration bands. As for dry grass and some senescent foliages, the emissivity is evidently reduced. Their cellulose gives the spectra double reflectance peaks between 10 and 12 μm and two minor absorption features between 8.5 and 9 μm. The emissivity peak near 3.4−3.5 μm is caused by volume scattering. Ribeiro da Luz and Crowley (Citation2007) found that some useful spectral information associated with leaf chemical constituents and structural aspects may be detectable from the spectral behaviours of emissivity. However, remotely measuring the subtle emissivity features of leaves remains a major technical challenge.
2.4.4. Spectral behaviour of water, ice, and snow
Although water is often assumed to have an emissivity of 1.0, it departs from black body behaviour at 11.2 μm in the TIR region. As for ice, the shape of the emissivity spectra is dominated by the volume scattering of the surface roughness in the MIR and TIR regions, as shown in It should be noted that all emissivity spectra of water, ice, and snow shown in are also extracted from the ASTER Spectral Library (http://speclib.jpl.nasa.gov). Hori et al. (Citation2006) found that the derived emissivities of snow and ice show a distinct spectral contrast at wavelengths between 10.5 and 12.5 μm, which suggests the possibility of discriminating between snow and ice from space.
3. Estimation of surface emissivity from space
Radiance measured from space can cover a large spectral range, from the visible/near-infrared (VNIR), MIR, and TIR to the microwave (MW) region. This radiance containing the combined effects of surface and atmosphere can be used to infer the LSE. To date, various methods have been proposed to this end. Several use the statistical relationships between the measurements and the emissivities; others use reasonable assumptions or constraints on the basis of Planck's function and the atmospheric RTE to solve the underdetermined problem or the ill-posed inversion process. In general, there are three distinctive ways to estimate LSE from space: (1) semi-empirical methods (SEMs), (2) multi-channel temperature/emissivity separation methods, and (3) PBMs. Although all of these methods can be used to get emissivity from space with their own advantages and limitations, their applicability differs significantly. For example, several methods need to correct for the atmospheric effects in advance. Before the analysis and the comparison of these methods, a brief description of each method is given in the following sections.
3.1. Semi-empirical methods (SEMs)
This type of method estimates the LSE from the semi-empirical classification-based look-up table or from the statistical relationship between the normalized difference vegetation index (NDVI) derived from the VNIR and the emissivity in the TIR band. The representative methods are the classification-based emissivity method (CBEM) (Snyder et al. Citation1998; Sun and Pinker Citation2003; Peres and DaCamara Citation2005) and the NDVI-based emissivity method (NBEM) (Van de Griend and Owe Citation1993; Valor and Caselles Citation1996; Sobrino and Raissouni Citation2000).
3.1.1. Classification-based emissivity method (CBEM)
Generally, the CBEM is based on the use of conventional land-cover classification information. The key point of this method is to properly classify the land surface and then to assign the emissivity from classification-based look-up tables.
To determine the LST from MODIS TIR data using the split-window algorithm, Snyder et al. (Citation1998) first proposed this CBEM by considering the addition of three dynamic effects: snow and water, senescence, and the different crop states. They developed an emissivity knowledge database (for 10.8−12.3 μm) using three different BRDF kernel models (Li and Strahler Citation1992; Roujean, Leroy, and Deschamps Citation1992; Snyder and Wan Citation1998), which have spectral coefficients derived from laboratory measurements of material samples and structural parameters derived from approximate descriptions of the cover type. The hemispherical-directional reflectance and the emissivity are then obtained via integration of BRDF over an angle range from 0° to 65° based on Kirchhoff's law. After a series of combinatorial analysis, 14 distinct emissivity classes with similar properties are selected from MODIS/International Geosphere-Biosphere Programme (IGBP) classes, in which some biophysically distinct cover types with similar emissivity are combined, e.g. an evergreen needle forest and a green deciduous forest, and some conventional classes showing different emissivities are separated due to seasonal or dynamic state changes, e.g. growing crops and idle crop fields. These classes exhibit a good balance between the number of classes and the emissivity accuracy. The analysis of the mean value and variations of emissivity classes shows that eight of the 14 classes, covering almost 70% of the Earth's land surface, can be determined with sufficient accuracy to meet the goal of 1K accuracy required for MODIS LST estimation. However, more information is needed to improve the emissivity accuracy for the other six classes (Snyder et al. Citation1998). Once the emissivity knowledge database is developed, the LSE can be estimated directly from the MODIS/IGBP class with the consideration of seasonal and dynamic states (Snyder et al. Citation1998).
Obviously, the mixture of several surface types within one pixel may influence the accuracy of LSE retrieval. In this case, the LSE can be estimated using a linear mixing model, as in EquationEquation (4)(4). This method has already been applied to geostationary satellite data, such as Meteorological Satellite (METEOSAT) Second Generation-1 (MSG) data (Peres and DaCamara Citation2005; Trigo et al. Citation2008) and Geostationary Operational Environmental Satellite (GOES) data (Sun and Pinker Citation2003).
Theoretically, the CBEM can produce accurate LSE products over the areas in which land surfaces are accurately classified and where each class has well-known emissivities (Gillespie et al. Citation1996). For example, the emissivities of water or of closed-canopy vegetation may be assigned reliably. In other words, the classification-based emissivity prediction is thought to be accurate for most classes, especially for high-resolution remotely sensed data (pure pixels). However, Snyder et al. (Citation1998) pointed out several major difficulties in using CBEM, such as the determination of surface wetness, the identification of senescent vegetation, and the uncertainty of the dynamics of snow and ice surface states. All of these difficulties and uncertainties may prevent an accurate estimation of the LSE for some of the classes. Because the existence of water can increase the LSE of any nearby material, night dew may become the primary source of error for low-emissivity classes. Furthermore, there likely exists a large variation in the emissivity within some of the classes, and the classification based on VNIR data is generally not well correlated with the LSE in the TIR region. Thus, the CBEM method may be less reliable and can produce large errors for these classes. For instance, the estimation of LSE using the CBEM for geologic substrates is uncertain because the VNIR reflectances used to classify the land surfaces respond mainly to hydroxyl ions and iron oxides, while the emissivities in the TIR band are mainly responsive to the silicion−oxygen bond (Gillespie et al. Citation1996). In addition, LSE maps would have inappropriate discontinuities or appear seamed or contoured because of the discontinuities in the classification (Gillespie et al. Citation1996).
3.1.2. NDVI-based emissivity method (NBEM)
Within the framework of the project ‘Botswana Water and Surface Energy Balance Research Program’, Van de Griend and Owe (Citation1993) found a very high correlation between the LSE in the band covering 8−14 μm and the logarithm of the NDVI, i.e.
Inspired by the work of Van de Griend and Owe (Citation1993), using NDVI as a connection to describe the ‘cavity effect’, Valor and Caselles (Citation1996) proposed a theoretical method derived from the model of Caselles and Sobrino (Citation1989) to estimate the effective LSE for a row-distributed rough system in which the observed radiance is the weighted sum of the radiances coming from the ground, from the top, and from the side. In their method, the effective LSE can be numerically expressed as the sum of the area-weighted emissivities of each subcomponent and the cavity effect. Both weights and the cavity effect are functions of the NDVI. However, this method requires a priori knowledge of some geometrical parameters of the vegetation, such as height, width, and the separation between rows, which limits the application of the method (Jimenez-Munoz et al. Citation2006). Furthermore, this method ignores the high variability of soil emissivity in the 8−9 μm range. Consequently, the emissivity at 8−9 μm cannot be accurately estimated with this method, whereas the emissivity at 10−12 μm, where the soil and vegetation emissivities show little variation, can be (Coll et al. Citation2003a). Although the model of Valor and Caselles (Citation1996) has a theoretical basis, several quantities, such as the emissivities and NDVIs for vegetation and soil, as well as some geometrical parameters, are required a priori, which is usually not possible in practice. In this sense, this method may still be categorized as being one of the SEMs.
Considering the complexity of method proposed by Valor and Caselles (Citation1996), Sobrino and Raissouni (Citation2000) further developed an operational NDVI threshold method to derive the LSE from space using the following criteria and formulae:
When NDVI < NDVIs, the relationship between the emissivities and the red reflectivities is assumed to be linear and the coefficients can be determined from laboratory measurements of the soil spectra. However, Dash et al. (Citation2005) argued that the correlation for this linear relationship in remotely sensed data is not strong enough. Subsequently, Sobrino et al. (Citation2008) proposed the use of multispectral VNIR capabilities to improve this relationship. When NDVIs < NDVI < NDVIv, the mean cavity effect dϵλ
can be expressed as a linear function of P
v (Sobrino and Raissouni Citation2000). When NDVI > NDVIv, typical constant values of and
are used in TIR bands (Sobrino et al. Citation2008).
Because of its simplicity, the NDVI threshold method has already been successfully applied to various sensors, e.g. the Advanced Very High Resolution Radiometer (AVHRR) (Sobrino and Raissouni Citation2000), Digital Airborne Imaging Spectrometer (DAIS) (Sobrino et al. Citation2002), MODIS (Sobrino, Kharraz, and Li Citation2003; Momeni and Saradjian Citation2007), Thematic Mapper (TM) (Sobrino, Jimenez-Munoz, and Paolini Citation2004), Advanced Along Track Scanning Radiometer (AATSR), Spinning Enhanced Visible and Infrared Imager (SEVIRI), and Airborne Hyperspectral Scanner (AHS) (Sobrino et al. Citation2008). Because only VNIR bands, which always have a higher spatial resolution than TIR bands, are required, emissivity with finer resolution can be mapped (Sobrino et al. Citation2008). Moreover, an accurate atmospheric correction is not needed when estimating P v. However, the main problem with this method is the lack of continuity for emissivity values at NDVI = NDVIs and NDVI = NDVIv because they are calculated using different functions (Sobrino et al. Citation2008). From numerical analysis, Sobrino et al. (Citation2008) pointed out that the NDVI threshold method can provide acceptable results only in the 10–12 μm interval bands because the relationship between the emissivity and the reflectivity for bare soil samples does not provide satisfactory results in the 8.0–9.5 μm domain for some soil types. In addition, this method may hold well only for mixed soil and vegetation areas, except for senescent vegetation, and is not applicable for surfaces such as water, ice, snow, and rocks (Sobrino et al. Citation2008). Furthermore, it requires a priori knowledge of the emissivities of soil and vegetation (Sobrino and Raissouni Citation2000). The determination of soil emissivity may be the main source of error in this method (Jimenez-Munoz et al. Citation2006).
3.2. Multi-channel TES methods
The multi-channel TES methods referred to here include a group of algorithms that retrieve the LST and LSEs from the at-surface radiance. After introducing some reasonable assumptions or constraints, these methods retrieve the LSEs directly from the emitted radiance.
3.2.1. Emissivity spectrum character-based methods
This type of method determines the emissivity from the characteristics of emissivity spectra. Therefore, the emissivity can be derived using several methods: by assuming that the emissivity at a channel is time invariable, e.g. the two-temperature method (TTM) (Watson Citation1992); by assuming that the emissivity has a flat spectrum for specific wavelengths, e.g. the grey-body emissivity (GBE) method (Barducci and Pippi Citation1996); by assuming that the emissivity spectrum is smooth, e.g. the iterative spectrally smooth temperature emissivity separation (ISSTES) method (Borel Citation1997); or by applying a priori knowledge about the emissivity distribution range, e.g. the emissivity bounds method (EBM) (Jaggi, Quattrochi, and Baskin Citation1992).
1. | GBE method Assuming that the emissivity has a flat spectrum for wavelengths larger than 10 μm, Barducci and Pippi (Citation1996) suggested estimating the emissivity spectrum from remotely sensed data using the flat characteristics of the emissivity spectrum in some wavelength intervals. On the basis of the assumption that It should be noted that the application of the GBE method to space measurements requires correcting for atmospheric effects and also requires that at least two channels have the same emissivity but not necessarily the grey body. Because it is easier to find at least two channels with the same emissivity in hyperspectral data than in multispectral data, this method is thought to be more applicable for hyperspectral TIR data. | ||||
2. | TTM Because the measured radiance emitted by a surface is dependent on both emissivity and temperature, if a surface with time-invariant emissivities is observed using N channels at two different temperatures (e.g. at two different times, one in daytime and another in night-time), there are 2N equations with N + 2 unknowns (N channel emissivities and two temperatures). Provided that N≥ 2 and the atmospheric effects are known or can be accurately estimated, N emissivities and two temperatures can be simultaneously determined from 2N equations (Watson Citation1992). In addition to accurate atmospheric corrections, this method requires an accurate geometric registration of the data acquired at two different times (Watson Citation1992; Gillespie et al. Citation1996). It has been shown that the impact of the misregistration on the LSE error is small for homogeneous areas but large for heterogeneous areas (Wan Citation1999). Because the emissivities at two different times are required to be time invariant for the TTM, a change in the satellite viewing angle will cause a change in the LSE, consequently violating the assumption of time-invariant emissivity and decreasing the accuracy of the TTM. The use of geostationary satellite data avoids these two problems (misregistration and change in viewing angle). With high temporal resolution and a fixed viewing angle for a given pixel, the geostationary satellite is expected to provide an adequate implementation of the TTM. Faysash and Smith (Citation1999, Citation2000) and Peres and DaCamara (Citation2004) applied this method to GEOS and MSG/SEVIRI data, respectively. They showed that LST and LSEs could be obtained with reasonable accuracy for most cases, for example the averaged biases and root mean square errors (RMSEs) of LSE range from 0.001 to 0.101 and from 0.028 to 0.045, respectively, while those of LST range from 0.0 to 0.5 K and from 0.8 to 2.5 K, respectively. It should be noted that the solution of this method is sometimes unstable and dependent on the initial values. Although TTM can directly estimate the spectral emissivity without any assumption about the spectral shape, which is its primary advantage over other methods, it is sensitive to noise (Watson Citation1992; Gillespie et al. Citation1996; Caselles et al. Citation1997). Peres and DaCamara (Citation2004) pointed out that increasing the number of observations and/or the temperature difference improves the retrieval accuracy, but this improvement is limited for larger measurement errors. | ||||
3. | ISSTES method Hyperspectral TIR data provide much more detailed information on the atmosphere and land surface. Taking into account the fact that the surface emissivity spectrum is smoother than the atmospheric spectral features in hyperspectral TIR data, different methods have been proposed to estimate LST and LSE from hyperspectral data (Borel Citation1997, Citation1998). From EquationEquation (8) If the surface temperature (T) deviates from its actual value, the atmospheric spectral features appear in its corresponding retrieved emissivity spectrum as shown in EquationEquation (12) Here, SM1 is one of the definitions of spectral smoothness. Other definitions of SM will be listed below. Borel also iteratively found the optimal estimate of LST corresponding to the minimum of the spectral smoothness (EquationEquation (13) Different formulations for SM, including the first and second derivative criteria for the spectral smoothness criterion, have been proposed (Borel Citation1997, Citation1998; Kanani et al. Citation2007; OuYang et al. Citation2010). Some of them, numbered 2–4, are listed below:
All of these statistically lead to the same performance for hyperspectral TIR data, implying that the performance is not sensitive to the choice of smoothness function. Ingram and Muse (Citation2001) analysed the sensitivity of this type of method to algorithmic assumptions and measurement noises. They pointed out that the RMSE of emissivity resulting from the algorithmic assumptions is negligible for a typical material and is only about 0.0004 if there were no errors in the radiance at ground level. It turns out that the accuracy of the retrieved emissivity depends significantly on the signal-to-noise ratio (SNR). At SNR = 1200:1, the RMSE (bias) of the surface emissivity is in the range of 0.002−0.004 (−0.0004 to +0.0008), and, at SNR = 240:1, the accuracy of the emissivity is degraded to RMSE = 0.015–0.025 and Bias = −0.009 to +0.015. Borel (Citation2008) pointed out that this type of method suffers from a shift of the spectral position and change in the full width at half maximum (FWHM). He demonstrated that a small spectral position shift (1/20th of the wave centre spacing) can produce large retrieval errors. This method also requires atmospheric compensation. However, as the hyperspectral IR data can provide enough information to extract the atmospheric information, Borel (Citation2008) coupled the in-scene atmospheric correction (ISAC) algorithm proposed by Young, Johnson, and Hackwell (Citation2002) with the spectral smoothness technique to generate LST and LSEs from airborne hyperspectral data without knowing the atmospheric state. | ||||
4. | Emissivity bounds method (EBM) For a given measured radiance in a reasonable range of emissivity (ϵ), a locus of points It is worth noting that there is no assumption introduced in the development of this method, and the more accurate the knowledge of the emissivity distribution range, the better the performance of this method. However, this method provides only a possible range of LST and LSE. |
3.2.2. Reference channel method (RCM)
RCM was first developed by Kahle, Madura, and Soha (Citation1980). This method assumes that the emissivity in one channel has a constant value for all pixels. Provided that the atmospheric effects are known or can be accurately estimated, for each pixel the LST can be derived from the measured radiance in this reference channel using the known emissivity. This LST is then used in EquationEquation (12)(12) to derive emissivity values for the remaining channels.
Although RCM is the simplest method for the emissivity retrieval from space, it suffers from some limitations. First, it is difficult to find a unique emissivity value that is appropriate for all surface materials in one reference channel. For example, the mean emissivity for vegetation is about 0.98 compared to 0.95 for most silicate rocks at wavelengths larger than 12 μm (Gillespie et al. Citation1996). An uncertainty of 1% in the emissivity in this reference channel can result in an error of about 0.5 K in LST and emissivity errors of about 1–2% in other channels (Li et al. Citation1999b). Second, because the emissivity in the reference channel is assigned as a constant value for all pixels, there is no emissivity spatial information in this channel. Furthermore, the emissivities derived for adjacent channels are significantly affected by the constant value of emissivity in this channel and appear to be very noisy (Hook et al. Citation1992).
3.2.3. Normalization emissivity method (NEM)
This method was first described by Gillespie (Citation1985) and used by Realmuto (Citation1990). It assumes a constant emissivity in all N channels for a given pixel, which enables N temperatures to be calculated for each pixel from their measured radiances, provided that the atmospheric quantities involved in the RTE are known. The maximum of those N temperatures is considered to be the LST and used to derive emissivities for the other channels using EquationEquation (12)(12), as is done with RCM. Mushkin, Balick, and Gillespie (Citation2005) applied this method to the Multispectral Thermal Imager (MTI) data and assumed different constant emissivity in the MIR and TIR bands. They found that the retrieved accuracy with NEM is consistent with that reported for the ASTER TES method described below. Coll et al. (Citation2001) and Coll et al. (Citation2003b) proposed an improved NEM called the adjusted normalized emissivity method (ANEM). In their work, the field emissivity measurements were used to adjust the initial maximum emissivity, then NEM was performed. However, because ANEM requires in situ measurements, it is of limited use for exploration studies (Coll et al. Citation2003b).
NEM is an improvement of RCM as the channel with the maximum emissivity can be different in NEM for different materials. It is recommended by Li et al. (Citation1999b) and selected by Gillespie et al. (Citation1998) as one of the modules in the TES algorithm applicable to ASTER data.
3.2.4. TES method
TES is an algorithm initially developed for retrieving LST and LSE from ASTER images (Gillespie et al. Citation1998; Abrams Citation2000). This algorithm hybridizes three mature modules: NEM, spectral ratio (SR), and min–max difference (MMD).
TES first uses the NEM module to estimate the initial surface temperature and the normalized emissivities from the atmospherically corrected radiances at ground level. The SR module is subsequently used to calculate the ratio of the normalized emissivities to their average:
Although the SR β cannot directly provide the actual emissivity, it has been shown to describe the shape of the emissivity spectra well even if the surface temperature is roughly estimated. Finally, on the basis of SR, the MMD module is used to find the spectral contrast in N channels:
To recover the actual values of the emissivities, an empirical relationship between the minimum emissivity (ϵmin) in the N channels and MMD is established:
The main assumption of TES is that the empirical relationship between the minimum emissivity and the spectral contrast, i.e. EquationEquation (17)(17), holds true for the entire gamut of surface materials (Gillespie et al. Citation1996). Gillespie et al. (Citation1996) tested this assumption and reported it to be valid for most surfaces. However, Payan and Royer (Citation2004) found that metals do not obey this empirical relationship. However, natural surfaces are mainly composed of water, soils, vegetation, and snow rather than metals. Therefore, the violation of the empirical relationship by metals does not degrade the retrieved accuracy any more than when dealing with Earth observation data using the TES method. Because of its simplicity and the lack of any need for a priori knowledge of the surface, the TES method has already attracted more and more attention (Schmugge et al. Citation2002; Dash et al. Citation2005). At the same time, some changes in the original TES method are proposed to improve the retrieval accuracies, including integrating broadband emissivity in the iterative algorithm for high contrast emissivity surfaces (Payan and Royer Citation2004), adjusting radiances with respect to channel 13 (10.6 μm) of ASTER for low contrast surfaces (Coll et al. Citation2007), removal of the iterative correction for downwelling irradiance and the threshold test for spectral contrast (Gustafson, Gillespie, and Yamada Citation2006), and the inclusion of a water adjustment and compensation for partially vegetated surfaces by a fractional vegetation cover adjustment (Hulley and Hook Citation2009b).
The main advantages of the TES algorithm are (1) it provides LST and LSE simultaneously from the atmospherically corrected multispectral TIR data at ground level; (2) it refines the values of the maximum emissivity used in the NEM, pixel by pixel and consequently improves the NEM approach; (3) it is a priori applicable to any kind of natural surface, especially for geologic surfaces (Gillespie et al. Citation1998; Sobrino et al. Citation2008). However, the main sources of uncertainty in estimating LST and LSEs using TES consist of (1) the atmospheric compensation especially for the humid atmosphere. Sabol et al. (Citation2009) reported that the atmospheric compensation is insufficient and retrieval errors may be larger than anticipated under unusual atmospheric conditions with anomalously high humidity or spatial variability; (2) the empirical relationship of EquationEquation (17)(17). This type of uncertainty is more serious for grey bodies than for high MMD, where radiometric noise has a significant impact on the apparent MMD (Gillespie et al. Citation1998; Coll et al. Citation2007), thus the accuracy of TES is expected to decrease for surfaces with low spectral contrast, as is the case of agricultural areas; and (3) compensation for reflected sky irradiance and sensor calibration (Gillespie et al. Citation1998; Jimenez-Munoz et al. Citation2006; Sobrino et al. Citation2008). It should be noted that the TES algorithm requires at least three TIR bands located in the atmospheric windows; it cannot be applied to most operational sensors (Sobrino et al. Citation2008).
3.2.5. Temperature-independent spectral indices (TISI) method
TISI was first proposed by Becker and Li (Citation1990a) and was used to perform spectral analysis in the TIR region. This method is based on the power-law approximation of Planck's function, :
By taking the product of the N channel measurements described in EquationEquation (20)(20) to the power dk
(k= 1, … , N) such that:
Defining the temperature-independent spectral index (TISI) as:
Because in EquationEquation (21)
(21) theoretically depends on the unknown surface temperature, to calculate TISI from the definition in EquationEquation (23)
(23), the surface temperature must be estimated by an assumed initial emissivity (Nerry, Petitcolin, and Stoll Citation1998) using an iterative approach (Dash et al. Citation2005) or substituted by the highest ground brightness temperature among all channels used for a given pixel (Li et al. Citation1999b; Li, Petitcolin, and Zhang Citation2000). It has been shown that the difference between TISI estimated using the approximate surface temperature and TISIE is small (<1%) (Becker, and Li Citation1990a; Nerry, Petitcolin, and Stoll Citation1998).
Becker and Li (Citation1990a), Li and Becker (Citation1990), and Li et al. (Citation1999b) demonstrated that TISI is nearly independent of surface temperature and is a pure combination of channel emissivities. The two most important properties of TISI are its ability to be tailored to weight interesting spectral channels more heavily than others because the N ‘’ parameters are determined with only one equation (EquationEquation (22
(23))) and that TISIs are quite complementary to NDVI and can lead to stronger results if used together rather than separately.
According to the general definition of TISI given by EquationEquation (23)(23), taking d
i
= 1 and using the channel radiance Ri
, Becker and Li (Citation1990a) and Li et al. (Citation1999b) defined a two-channel TISI, TISI
ij
, for two channels i and j as:
Taking , EquationEquation (26)
(26) becomes:
They showed mathematically that:
3.3. Physically based methods (PBMs)
The methods reviewed above generally assume that the atmospheric effects on the radiances measured at the TOA have been accurately corrected for or that the radiances are measured at ground level. As far as the estimation of LST and LSE from space measurements is concerned, in addition to the unknown LST and LSEs, there are some additional unknowns due to the spectral absorption and emission in the intervening atmosphere. With N spectral measurements from space, the solution for the temperature and the N spectral LSEs is underdetermined. In this section, we will review three of the early PBMs that have been fairly widely used to approach this problem using various physically based assumptions or constraints. These methods include the TISI-based method (Becker, and Li Citation1990a; Li and Becker Citation1993; Li, Petitcolin, and Zhang Citation2000), the physics-based day/night operational method (Wan and Li Citation1997), and the two-step physical retrieval method (TSRM), which uses the principal component analysis (PCA) technique to decrease the number of unknowns (Ma et al. Citation2000, Citation2002; Li et al. Citation2007).
3.3.1. TISI-based method
On the basis of the concept of the two-channel TISI, Li and Becker (Citation1990) and Li, Petitcolin, and Zhang (Citation2000) have proposed and improved an active/passive method to retrieve the surface emissivities using the Sun as an active source. In the MIR channel (around 3.7 μm), the radiance emitted by the land surface itself and the reflected radiance due to Sun's irradiation during the day are of the same order of magnitude if the surface reflectance in this channel is about 0.1, and there is no solar reflection at all at night. Therefore, the general idea of this method is to extract the bidirectional reflectivity in the MIR channel by eliminating the emitted radiation during the day with that during the night due to a particular intercomparison between day and night TISI. Choosing channel i as any channel in MIR (3−5 μm) and channel j as any channel in TIR (10−13 μm) and assuming that
(superscripts d and n denote daytime and night-time, respectively), Becker and Li (Citation1990a) and Li, Petitcolin, and Zhang (Citation2000) proposed to derive
using:
As indicated by EquationEquation (29)(29) and reported by Li, Petitcolin, and Zhang (Citation2000), the bidirectional reflectivity in channel i can be retrieved directly from space measurements provided that all of the following four conditions are fulfilled.
1. | The infrared radiometer on board the satellite has at least two channels, one within the 3−5 μm window and the other within the 10−13 μm window. | ||||
2. | Multi-temporal data in both MIR and TIR channels should be available, at least one set of data during the day and the other at night. | ||||
3. | The appropriate emissivity ratios are assumed to be the same or do not change significantly between day and night (the difference of the appropriate emissivity ratios is less than 0.01), i.e. | ||||
4. | The channel radiance L at ground level can be obtained with good accuracy from the channel radiance at the TOA after atmospheric corrections. |
Assuming that the surface is Lambertian in the MIR channel or that the ρbi
in the MIR channel at the two data acquisition times during the day are the same, Goita and Royer (Citation1997) extended the TISI-based method developed by Becker and Li (Citation1990a) to be applicable for two consecutive data sets acquired during the day. They also proposed an alternative method to estimate ρbi
from EquationEquation (29)(29) but with
estimated by the ratio of the atmospherically corrected radiances in the MIR and TIR channels during the day instead of using
calculated from the night measurements. The empirical linear relationship between
and the ratio of the radiances in the MIR and TIR channels during the daytime was established from theoretical simulations computed with the acquisition conditions and a reference emissivity database (Goita and Royer Citation1997). Although this alternative method gives less satisfactory results than others, only one daytime image is required.
To retrieve the directional emissivity, , from the bidirectional reflectivity
extracted by EquationEquation (29)
(29), three methods have been proposed. The first is to use an angular form factor
, which was introduced by Li, Petitcolin, and Zhang (Citation2000) to describe how a bidirectional reflectivity differs from that of a Lambertian reflector. With the help of this angular form factor, the directional emissivity in channel i can be estimated by:
The third is to use a kernel-driven bidirectional reflectivity model, the RossThick–LiSparse–R model, to describe the non-Lambertian reflective behaviour of land surface in MIR as being the same as that in VNIR regions (Roujean, Leroy, and Deschamps Citation1992; Wanner, Li, and Strahler Citation1995; Lucht and Roujean Citation2000):
Once the directional emissivity in channel i is known, the directional emissivity in channel j is easily derived from the definition of TISI with the assumption that , i.e.
Based on an analysis of the sensitivity of TISI and emissivities to different error sources, Nerry, Petitcolin, and Stoll (Citation1998) reported that the errors due to the approximations and the instrumental error do not exceed 1%. Li et al. (Citation1999b) showed that TISI may be sensitive to the uncertainties in atmospheric corrections. Nevertheless, the impacts of the uncertainties in atmospheric corrections on the emissivities are less serious than those on the temperature itself. The use of an approximate (standard) atmosphere instead of an actual atmosphere may lead to 3% or smaller errors in LSE and 0.5 K in LST using the split-window method (Becker and Li Citation1990b; Li and Becker Citation1993).
It is worth noting that the TISI-based method does not need any a priori information on the surface and can be applied to any surfaces, even those with strong spectral dynamics. Due to the fact that this method is based on multi-temporal data (a series of retrieved ), which require accurate image co-registration, the retrieval errors may be large otherwise (Dash et al. Citation2005). Additionally, the surfaces must be observed under similar observation conditions, e.g. view angle, during both day and night (Dash et al. Citation2005). However, Petitcolin, Nerry, and Stoll (Citation2002) argued that TISI has mild angular dependence and remains stable over several weeks. Furthermore, the method needs both MIR and TIR data at the same time (Sobrino and Raissouni Citation2000). All of these problems may limit its usage in the retrieval of emissivity from space.
3.3.2. Physics-based day/night operational (D/N) method
The physical methods usually face more unknowns simultaneously; in other words, the physical methods need more channels than other methods. To simultaneously retrieve LSEs and LST without an accurate a priori knowledge of emissivity information and atmospheric parameters, Wan and Li (Citation1997) proposed a physics-based retrieval method using day/night pairs of combined MIR and TIR data. The main purpose of this method is to retrieve LST and LSEs in semi-arid and arid regions where the surface emissivity varies spatially over a wide range (Wan Citation1999). Based on the three assumptions of surface optical properties, (1) the surface emissivity does not significantly change in the daytime/night-time in several days unless rain and/or snow occurs during the short period of time, (2) the angular form factor defined in EquationEquation (30)
(30) has very small variations (<2%) in the wavelength range of interest in MIR, and (3) the Lambertian approximation of surface reflection for downwelling diffuse solar irradiance and atmospheric thermal irradiance does not introduce significant error in the 3−14 μm region, the radiance measured by band i (EquationEquation (8
(8))) can be expressed as:
It is worth noting that in the D/N algorithm
1. | Because the radiance measured in the MIR channel during the daytime includes the contribution of the Sun's irradiation or solar irradiance, the correlation of 2N equations is significantly decreased by introducing the MIR channels, and making the solution of 2N equations stable, accurate, and possible. In this case, LSTs and LSEs can be accurately determined even though the LSTs are equal at the two acquisition times (daytime and night-time); however, this is not the case for using only the measurements in TIR channels in which case the difference of LST between day and night must be large enough. | ||||
2. | The number of unknowns in the 2N equations is decreased by assuming that the angular form factor is the same for all the MIR channels, leading to a well-determined solution for the 2N equations. | ||||
3. | The retrieved accuracy of LSTs and LSEs is largely improved by introducing two variables (T a and CWV) to take into account the uncertainties in the atmospheric profiles. | ||||
4. | Because the existence of water increases the emissivity of any material (Snyder et al. Citation1998), night dew may be the primary source of error for LSE retrieval, especially for low emissivity surfaces in dry areas. However, considering the low frequency of dew occurrence in the semi-arid and arid regions, dew is not a serious problem, which may only slightly complicate the process (Wan Citation1999). | ||||
5. | The MODIS D/N method does not require the 12-hour interval in the measurements (day and night); as long as the surface emissivity does not change significantly, daytime and night-time data collected over several days are also appropriate (Wan Citation1999). |
3.3.3. Two-step physical retrieval method (TSRM)
Although MODIS is not specifically designed as a sounding instrument, it has 16 bands in the MIR and TIR regions, several of which match the corresponding bands on the High-Resolution Infrared Radiation Sounder (HIRS) provided by ITT (International Telephone & Telegraph) Exelis (McLean, VA, USA). MODIS can be therefore used to extract atmospheric profiles (Menzel et al. Citation2006). However, due to the coupling between the atmospheric information and the surface temperature through both the surface emissivity and the atmospheric transmittance, the retrieval process is a difficult task. Ma et al. (Citation2000) made a first attempt to simultaneously retrieve LST and atmospheric temperature−humidity profiles by assuming that emissivities are constant in the MIR and TIR regions, respectively, and by assuming that the solar contribution in the MIR can be ignored. Nevertheless, these assumptions may degrade the accuracy of atmospheric parameter retrievals in the troposphere (Ma et al. Citation2000). Li et al. (Citation1999c) demonstrated that surface emissivity spectra of more than 50 soil and vegetation samples measured in the laboratory can be reconstructed with an uncertainty of 0.005 by 6 selected bands in the 8−13 μm range. Due to the fact that emissivity spectra can be recovered by a low number of unknowns in the interest spectral region, Ma et al. (Citation2002) proposed an extended TSRM to extract LSEs, LST, and atmospheric temperature–humidity profiles with MODIS MIR and TIR data by taking the solar contribution into account.
The main idea of this method inherits that of atmospheric profile retrieval. First, the atmospheric RTE is tangent linearized with respect to the atmospheric temperature–humidity profiles, LST, and LSEs. Given an initial guess of LSEs, LST, and atmospheric temperature–humidity profiles, a set of equations based on the tangent-linearized RTE can be derived with the MODIS measurements. However, this is still an underdetermined problem because the number of unknowns is still larger than the number of equations. Consequently, the PCA technique is applied to the atmospheric temperature–humidity profiles as well as to the surface emissivity spectra so that the number of retrieved parameters is reduced and the solution of the set of equations becomes well determined. On this basis, Ma et al. (Citation2000, Citation2002) proposed a TSRM to extract emissivity, together with LST, atmospheric temperature, and moisture profiles by using the Tikhonov regularization and Newton iterative algorithms, one after the other. The Tikhonov regularization is used to stabilize the ill-posed problem and to obtain a meaningful solution, while the Newton iterative algorithm is used to further improve the solution. Thus, the name of the technique is ‘two steps’.
There are some assumptions involved in the linearization of the RTE. These assumptions include (1) a horizontally homogeneous atmospheric condition, (2) a specular surface reflection to simplify the integral of the downwelling atmospheric radiation, and (3) a constant anisotropic factor (angular form factor) in the MIR region to describe the non-Lambertian distribution of the bidirectional reflectance. However, these plausible assumptions may introduce some errors in the retrieval, but play only a secondary role. As discussed by Ma et al. (Citation2002), one possible improvement of this type of method is to improve the first-guess of the profiles and LSEs. They suggested using a model-based first-guess of the MODIS retrieval, the Atmospheric Infrared Sounder (AIRS) retrievals, and the first-guess of LSE retrieved by the D/N method. In addition, this method can be used to process the hyperspectral TIR data, such as data from AIRS developed by British Aerospace Systems, Infrared and Imaging Systems Division (LMIRIS; Lexington, MA, USA) and the Interferometer Atmospheric Sounding Instrument (IASI) developed by CNES (Centre National d'Études Spatiales) in the framework of a co-operation agreement with EUMETSAT (European Organisation for the Exploitation of Meteorological Satellites). These sensors have thousands of channels in the 3−14 μm region. Although the physical method gives definite physical meaning for each parameter, it is of great complexity. Similar to the above procedure, Li et al. (Citation2007) retrieved global TIR emissivity spectra from AIRS data using the physical method. However, more work, such as quality assurance, needs to be done before taking this approach for operational process.
4. Comparison and analysis of different methods
The methodologies for estimating LSEs from space were briefly reviewed in the previous section. With their advantages and limitations, these methods have different accuracies and are applicable for various sensors and applications. It is important to evaluate them on an identical standard and to give theoretical advice on their applications.
Generally, SEM is based on the VNIR spectral bands, while the others are based on the MIR and TIR spectral bands. SEM and PBM do not require a priori accurate atmospheric corrections because the methods are insensitive to atmospheric perturbation or because the atmospheric parameters are treated as unknowns that are retrieved simultaneously with emissivities. On the other hand, atmospheric corrections are crucial for the multi-channel temperature/emissivity separation methods described in Section 3.2.
Because the various methods have been proposed and developed under different circumstances and for various applications, only a few studies directly compare several methods. For instance, Li et al. (Citation1999b) evaluated six methods, including TISI, RCM, and NEM, for extracting the relative spectral emissivity from TIR data. They showed that (1) all of the methods are sensitive to atmospheric uncertainties, (2) the systematic error has little effect on the relative emissivity retrieval, (3) instrumental noise from 0.1 K to 0.3 K can lead to an error in the relative emissivity ranging from 0.002 to 0.005, and (4) the TISI and NEM methods are recommended because they are slightly superior to others.
Because the TISI-based and TES methods are theoretically robust and do not require a priori information on the emissivity or surface type (Dash et al. Citation2005; Sobrino et al. Citation2008) whereas NBEM is operationally simple and can give a satisfactory estimate of the LSEs for soil and vegetation mixed areas to some extent (Sobrino et al. Citation2002; Dash et al. Citation2005), TISI-based, TES, and NBEM are often taken as reference methods for comparison to others. Sobrino et al. (Citation2002) compared several retrieval methods and found that NBEM and NEM give the same absolute emissivity values with differences between 1% and 0.2%, while NBEM and TES give the greatest differences of around 2%. Jacob et al. (Citation2004) compared MODIS TISI-based and TES retrievals over Africa and Jornada and found that the retrieval results agree well, with root mean square deviations ranging from 0.006 to 0.016. Dash et al. (Citation2005) compared the performance of the TISI-based method and NBEM and found that the dynamic range of LSE is compressed in NBEM. The difference between the LSEs obtained by NBEM and the TISI-based methods ranges between –0.038 and 0.032, but the peak of the histogram of LSE difference for vegetated areas is 0.002, which further confirms that NBEM is more suitable for vegetated areas. Momeni and Saradjian (Citation2007) evaluated the emissivities of MODIS bands 31 and 32 retrieved from NBEM and the D/N method and found that they agree relatively well with each other. Wang and Liang (Citation2009) evaluated the ASTER and MODIS emissivity products at six Surface Radiation Budget Network (SURFRAD) sites and concluded that Collection 5 broadband emissivity is 0.01 larger than that of MODIS Collection 4 products and ASTER emissivity. Obviously, these comparisons contradict each other to some extent and highlight the importance of LSE validation.
Other methods, for a variety of reasons, have not been subject to a comparative analysis. However, their assumptions, advantages, and drawbacks are comprehensively described in Section 3, which gives full insight into the understanding of the emissivity retrieval methods. To give a concise overview of the methods reviewed above, the assumptions, advantages, and limitations of each of these methods are recapitulated in
Table 1. Comparisons of a variety of commonly applied LSE retrieval methods
5. Validation of satellite-derived LSEs
Undoubtedly, the quality and accuracy of LSEs estimated using different methods can only be assessed using validation. Generally, validation is important because the product accuracy is crucial to the scientific community and because feedback from the validation activity is invaluable to the improvement of quality of the generated products (Liang et al. Citation2002; Wang et al. Citation2007). Here, the validation activity consists of comparing the products to be validated with similar products derived from other independent sources. There are two distinct methods to validate the products. The first, known as the direct method, directly compares the ground-based measurements with satellite-derived products. The second, known as the indirect method, indirectly validates the non-validated product with the different satellite-derived products, model simulations, or other information and applications.
The direct validation of emissivity is straightforward and can be accomplished by comparing the retrieval emissivity with that measured in the laboratory or in situ. Direct emissivity comparisons are very challenging due to the heterogeneity of the land surface and the difficulty in the definition of the emissivities themselves (Petitcolin and Vermote Citation2002). For the spatial variability in LSE, the emissivities measured in the laboratory are not representative of the effective emissivity on a pixel scale. This problem can only be prevented over highly homogeneous and relatively flat surfaces, e.g. lakes, dense vegetation, or a uniform large area of Silt Playa (Wan et al. Citation2002). Wan et al. (Citation2002) report that the key requirements for a good LST validation site are a size larger than several pixels; a homogeneous surface in terms of material, emissivity, and temperature; easy accessibility for the deployment of instruments; and minor interference between the validation activities and the normal life activities. This point of view is also appropriate for the validation of LSEs. How then to obtain the ‘true’ emissivity for a given pixel on the homogeneous surface? Generally, once the homogeneous area is identified, several points in this area are selected and the corresponding emissivity is measured. To reduce the uncertainty, the average of these measured emissivities is regarded as the ‘true’ emissivity in this site and can then be compared with the satellite-derived emissivity. Dash et al. (Citation2005) proposed an approach based on object-oriented image analysis to identify homogeneous areas for ground-truth validation of satellite-derived emissivities. This technology may be beneficial for the ground-truth validation. Using this validation method, several studies are devoted to describing the quality and accuracy of different emissivity retrieval methods for various sensors. Coll et al. (Citation2001), Schmugge et al. (Citation2002), Hulley, Hook, and Baldridge (Citation2009a), and Sabol et al. (Citation2009) reported that the retrieved LSEs by TES are usually in qualitative agreement with field or laboratory measurements. Sobrino et al. (Citation2002) compared the results obtained by NBEM, NEM, and TES with the emissivities obtained in situ and concluded that NBEM gives the best results for vegetation plots and NEM for bare soil and water plots, with errors around 1% in both cases, while TES yields the best results for non-irrigated barley plots, with errors around 2%. Jimenez-Munoz et al. (Citation2006) validated NBEM and TES and found that NBEM gives RMSE <0.005 over vegetated areas and RMSE <0.015 over bare soil and that TES gives RMSE of about 0.01 for vegetated areas but RMSE >0.03 over bare soil. Wang et al. (Citation2007) compared the MODIS-derived emissivities in bands 31 and 32 with the spectral emissivities measured from samples collected at and around the Gaize site (a cold semi-desert region) and found that the ground-based emissivity measurements agree well with the emissivities estimated by the CBEM. However, Terra (Aqua) MODIS D/N-derived emissivities are 0.017 (0.011) less than ground-based measurements. Nevertheless, Wan (Citation2008) and Wan and Li (Citation2010) compared the emissivities retrieved from D/N to those measured by the Sun–shadow method in the Railroad Valley Playa and the grassland in northern Texas and argued that the emissivities retrieved using the D/N method compare well with the in situ measurements, reporting a difference of less than 0.0075 in the 10–12.5 μm range. The contradictive issues raised by these studies require more evidence to validate the emissivities and to provide application suggestions. Hulley and Hook (Citation2009a) compared the daily MOD11B1 emissivity product with laboratory emissivity measurements of the field samples collected over the Namib Desert and found that the mean absolute emissivity differences of bands 29 (8.55 μm), 31 (11 μm), and 32 (12 μm) are 1.06%, 0.65%, and 1.93% if the MOD11B1 emissivity product is generated by the D/N algorithm in versions V4, V4.1, and V5, respectively, suggesting that the MODIS V4- or V4.1-generated emissivity product is more accurate than that generated by V5 over arid and semi-arid areas (Hulley and Hook Citation2009a; Hulley, Hook, and Baldridge Citation2010).
However, it is difficult to find homogeneous areas, and, in practice, the surface is always heterogeneous on the pixel scale, driving us to determine the degree to which an emissivity product can be consistent with the actual states. Indirect validation is therefore an alternative and can reflect the performance and uncertainty for the emissivity-retrieved methods. Indirect validation here refers to the comparisons between different satellite-derived products, model simulations, or other information. An operational and effective validation approach is to scale the fine-resolution measurements up to the low-resolution measurements (Liang et al. Citation2002; Morisette, Privette, and Justice Citation2002; Wan et al. Citation2002; Wan Citation2008). Once the products at the finer resolution are validated, the accuracy of the products at the lower resolution can be assessed through product aggregation at the finer resolution. Hulley, Hook, and Baldridge (Citation2010) argued that the consistency of emissivity products at different resolutions with the independent retrieval algorithms may increase the reliability of products even though the rigorous validation is absent. This upscale validation method may be effective for emissivity validation; however, only a few attempts have been made to date (Hulley et al. Citation2009b). Several researchers proposed using other indirect methods to validate LSE retrieved from space. Petitcolin and Vermote (Citation2002) proposed checking the consistency of LST derived by TISI at different wavelengths to indirectly validate the accuracy of emissivities and found that the LSE accuracy is of the order of 1%. Dash, Gottsche, and Olesen (Citation2003) and Dash et al. (Citation2005) tried to use the simulated data to validate the emissivity retrieved by TISI. However, this synthetic validation only provides a preliminary error analysis. A direct comparison and an indirect comparison are still required for a final assessment. Momeni and Saradjian (Citation2007) suggested that the validation of LST retrieved by D/N is an indirect way to assess the accuracy of the estimated emissivities because they are simultaneously derived from the same 2N equations. However, this suggestion needs to be further investigated and verified.
6. Future development and perspectives
LSE has already been recognized as a crucial parameter for the discrimination and sometimes the identification of various surface types and for the determination of LST by radiometry. Although various methods have already been developed, there is still no best method to retrieve LSE from space. All of the methods either rely on statistical relationships or on assumptions and constraints to solve the inherent ill-posed retrieval problem. Therefore, they might not hold true under some circumstances, and it is necessary to choose the optimum approach to estimate LSEs from space for a particular case by taking the sensor characteristics, the required accuracy, computation time, as well as the availability of atmospheric temperature and water vapour profiles into account. From the previous discussion, the main restricting factors in the estimates of LSEs from remotely sensed data are actually the following.
1. | The difficulty of the atmospheric corrections: the presence of the atmosphere between the land surface and the sensors at satellite level disturbs the radiances measured by a radiometer at the TOA. These radiances result primarily from the emission/reflection of the surface modulated by the effects of the absorption, diffusion, and emission of the atmosphere. To minimize these atmospheric effects, sensors are always built in the windows where the atmosphere is most transparent to TIR radiation. The atmospheric corrections thus consist of correcting the radiance measured by the sensors for the effects of atmospheric absorption, emission, and emission−reflection. These effects can be variable because of the great variability of the vertical profiles of atmospheric water vapour and temperature. | ||||
2. | The difficulty in decoupling the LST and LSEs in the measured radiances: independent of the atmospheric problems presented in all spectral regions, the spectral radiance emitted by a surface is a product of the spectral LSEs of this surface and the spectral radiance of the black body at the LST. Therefore, it is not possible in passive IRT (infrared temperature) radiometry to separate, on a physical basis, the contributions due to LSE from the contributions due to LST in the observed radiance. For this reason, LSE determination from space requires not only atmospheric corrections but also knowledge of the LST and vice versa. | ||||
3. | The difficulty of physical interpretation of the measurement: the difficulties raised by the atmospheric corrections and the temperature−emissivity coupling are, to some extent, of a technical nature. On the other hand, the scaling problem is much more fundamental because it implies a conceptual analysis of the physical significance of the measured quantities (variables). Indeed, the diversity of continental surfaces involves spatial (vertical and horizontal) and radiometric heterogeneities of surface. Considering that the spatial resolution of the current on-board systems varies from 10−2 to 10 km2, it is therefore necessary to be able to define and correctly interpret surface parameters (variables) independently of the scale used and the processes necessary to validate this definition. | ||||
4. | The difficulty of validation of LSEs retrieved from space at the satellite pixel scale: comparisons between LSEs derived from space and in situ measurements are required to evaluate the reliability and accuracy of the LSE-retrieved methods. Although it may be feasible and reasonable to validate LSEs derived from remotely sensed data with traditional measurements, mainly conducted at the ‘point’ scale over uniform areas, problems will be encountered when the validation is performed over complicated land surface areas. |
Thus, a practical definition or interpretation of LSE at the pixel scale is of great help in understanding the physical process and validating LSE. Besides the definition and interpretation of the LSE for natural surfaces at the satellite pixel scale, studies are recommended to focus on the following subjects in the future for the accurate estimation of LSEs from space.
6.1. Enrichment of the spectral emissivity database
With the development of a new-generation sensors of high spatial resolution, the possibility of having pure pixels is significantly increased. Because the surface classification can be more accurately performed with the high-spatial resolution multispectral data, a potential and practical candidate to estimate LSE from space is the CEBM if the spectral emissivity database contains huge numbers of the natural and man-made materials encountered in the world. Although there are already several spectral libraries, such as the ASTER Spectral Library and the MODIS UCSB Emissivity Library, they only contain the emissivity spectra for several representative samples. Most of the measurements were performed by the use of an integrating sphere and a TIR spectrometer, which, in fact, measures the hemispherical-directional (near-nadir) reflectance of the sample in laboratory. Few measurements of emissivity spectra have been realized in the field due to the extreme difficulty of separating LST and LSE from the measured radiance. The current available emissivity database is far from meeting the requirements of CEBM operational use. It is therefore urgent to enrich the spectral emissivity database by both laboratory and field measurements and to document this database well. Moreover, an elaborate classification system should be established to strike a good balance between the number of classes and the emissivity accuracy.
6.2. Modelling of surface spectral emissivity in the atmospheric window (3–14 μm)
As stated earlier, there are always N + 1 unknowns for N spectral measurements (N equations); even though the atmospheric effects are known, the equations for the N + 1 unknowns are underdetermined. To overcome this problem and to make the solution well determined, one needs to either decrease the number of unknowns and/or increase the number of equations. The development of the surface spectral emissivity model intends to decrease the number of unknowns and to help estimate surface composition and surface soil moisture from spectral emissivity. This type of model needs several component properties or parameters to simulate the emissivity spectrum in the atmospheric window (3−14 μm). To date, the simulating model in the MW region has been developed and validated, such as the advanced integral equation method (AIEM) (Chen et al. Citation2003). The AIEM can simulate the band emissivities of bare soil at different frequencies and polarizations when the volumetric soil moisture and surface roughness parameters are given. Unfortunately, a similar model for the TIR region has not been developed yet. The development of a model to simulate the surface spectral emissivity in the atmospheric window (3−14 μm) using the main factors affecting emissivity, such as surface composition, surface soil moisture, structure and roughness, plant species, aerial density, and plant growth state, is therefore urgent.
6.3. Development of the intrinsic relationship of emissivities among several channels
As mentioned in Section 6.2, to make it possible to retrieve the LST and LSEs from the atmospherically corrected TIR data, it is necessary to reduce the number of unknowns and/or increase the number of equations. Some studies have been already attempted to find extra emissivity constraints. Liang (Citation2001, Citation2004) used a statistical relationship between multi-channel emissivities as a new equation to retrieve multi-channel emissivities. Based on the fact that the surface emissivity spectrum is rather smooth compared to atmospheric spectral features in the hyperspectral TIR data, Borel (Citation1997) used the smoothness characteristic of emissivities as a new equation to separate LST and LSEs from the hyperspectral TIR data. Recently, Wang et al. (Citation2011) assumed that the emissivities are a linear function of wavelength within a small wavelength interval in which there are at least three bands and used segment linear functions to describe the emissivity spectrum. Because each linear function has only two unknowns, the use of segment linear functions dramatically reduces the number of unknowns and makes LST and LSEs retrieval from hyperspectral TIR data stable and feasible. Other constraints or relationships may be proposed to reduce the number of unknowns and to increase the number of equations.
6.4. Methodology development for atmospheric corrections in hyperspectral TIR data
Atmospheric corrections play an important role in LST and LSEs retrieval from remotely sensed TIR data. Accurate atmospheric corrections must be performed and are the precondition for applying most of the LST/LSE separation methods. For LSEs retrieved from TIR multispectral data, atmospheric effects are generally corrected using either radiosounding data or atmospheric profiles retrieved from other sensors or platforms. However, along with the development of the new hyperspectral TIR sensor on board, much more detailed information on the atmosphere and land surface can be acquired. In other words, the narrow bandwidth of the hyperspectral resolution makes atmospheric absorption features prominent in the observed radiance spectrum, which offers an unprecedented opportunity to correct for atmospheric effects using the hyperspectral TIR data themselves. Recently, there has been some progress on this issue. Young, Johnson, and Hackwell (Citation2002) proposed an in-scene method to correct for atmospheric effects. Subsequently, this method was improved by Borel (Citation2008). Gu et al. (Citation2000) developed an autonomous atmospheric compensation method with the same purpose. However, both of them assume that the atmospheric state does not change while the surface temperature does over the whole study region. In the near future, research on this topic should therefore be focused on how to perform atmospheric corrections for the purpose of LST/LSE separation using only hyperspectral TIR data, without resorting to auxiliary data and assumptions.
6.5. Simultaneous retrieval of LSE, LST, and atmospheric profiles from hyperspectral TIR data
The sensors on board satellites measure radiances only at the TOA. These measured radiances are dependent on LST, LSEs, and atmospheric states. The coupling of surface-emitted radiance and atmospheric absorption, diffusion, and emission makes it difficult to retrieve surface parameters (LST and LSEs) and atmospheric profiles separately. The determination of surface parameters from space requires knowledge of atmospheric profiles and vice versa. It is therefore natural, preferable, and challenging to develop a method to simultaneously retrieve LST, LSEs, and atmospheric profiles without any a priori information on the surface and atmosphere. The developed methods can be regarded as the most promising ways to simultaneously retrieve these parameters in the near future. However, these methods may demand adequate observation channels with narrow bandwidth that can supply enough vertical resolution to extract atmospheric information and to separate LST and LSEs. In this aspect, Ma et al.'s (Citation2000, Citation2002) work can be regarded as the first attempt to retrieve LST, LSE, and atmospheric profiles without any auxiliary information. The appearance of hyperspectral TIR measurement offers a more attractive opportunity, and efforts in the near future should therefore be made to develop methodologies for simultaneously retrieving LSEs, LST, and atmospheric profiles from hyperspectral TIR data. To date, several methods have been proposed to decouple LST and LSE from either at-ground radiances (Cheng et al. Citation2008, Citation2010, Citation2011; Wang et al. Citation2011) or TOA radiances (Li et al. Citation2011). At the same time, the method for estimating LSE from hyperspectral TIR data is also developed (Zhou et al. Citation2011). These efforts along with the physical methods of simultaneous retrieval of LSE, LSE, and atmospheric profiles (Ma et al. Citation2000, Citation2002; Li et al. Citation2007) would be of great benefit to the accurate estimation of LSEs from space.
6.6. Estimation of the broadband-hemispherical emissivity from the retrieved narrowband-directional emissivities
Surface broadband-hemispherical emissivity is an essential parameter for estimating the surface upward longwave radiation, which is an important component of the surface radiation budget and also an important parameter for numerical weather predictions and hydrological models (Blondin Citation1991; Jin and Liang Citation2006). Knowledge of the surface broadband-hemispherical emissivity is therefore valuable. However, the emissivity retrieved from remotely sensed TIR data represents only the narrowband-directional emissivity. It characterizes the emission features of the surface in a specific observation direction in a few narrow bands in the atmospheric window (3−14 μm) rather than the broadband-hemispherical emissivity required for the calculation of surface upward longwave radiation (0–∞ μm). The difficulties in estimating the broadband-hemispherical emissivity from remote-sensing measurements are that the measurements can only be conducted (1) in a few narrow spectral bands in the atmospheric windows and not over the whole spectrum (0–∞ μm), raising the issue of the spectral integration over the whole electromagnetic spectrum and (2) at a small number of observation angles, raising the issue of the angular integration over the whole upward hemisphere. The problem to be solved is therefore the determination of the best spectral channels (narrow bands) and observation angles to achieve the most accurate estimation of broadband-hemispherical emissivity. At present, some efforts have only focused on the estimation of broadband-directional emissivity for some specified spectral domains because of the limitation of the measured spectral emissivity in the 3−14 μm region (Ogawa et al. Citation2003; Wang et al. Citation2005; Jin and Liang Citation2006; Tang et al. Citation2011). To our knowledge, there has been no literature involved in the conversion of the directional emissivity to the hemispherical emissivity.
As demonstrated by Tang et al. (Citation2011), the spectral domains in which we have spectral measurements of the emissivity (3−14 μm) account only for 51.5% of the emitted radiant flux for a black body at 300 K, while the unknown spectrum accounts for the remaining 48.5% of this flux. The contribution of the spectral interval from 3 to 100 μm to the emitted flux is more than 99%. Therefore, the broadband-hemispherical emissivity with integrated spectral range, reaching 100 μm, should be accounted for to minimize the impact of the emissivity signature on the radiant flux determination. Over the last 30 years, a large number of measurements of the spectral directional emissivity of natural media in the atmospheric window (3−14 μm) have been performed in the laboratory and in the field, but no synchronous broadband-hemispherical emissivity is available. Thus, the empirical relationship developed for estimating the broadband-directional emissivity from a few narrow bands in the atmospheric window is limited to 3−14 μm. It is therefore urgent in the near future to (1) develop an instrument that can be used to simultaneously measure the spectral emissivity in the atmospheric window and the broadband-hemispherical emissivity in the whole spectrum (0–∞ μm), (2) solve the problem presented above, and (3) develop some methodologies to estimate the broadband-hemispherical emissivity from the retrieved narrowband-directional emissivities.
6.7. Combined use of laser CO2 as an active source and TIR sensor measurements to estimate LSE
Inspired by the principle of the TISI-based method reviewed in Section 3.3.1, a possible solution to estimate LSE can be obtained based on the simultaneous use of passive and active radiometry. This solution can be realized by viewing the surface with and without the illumination of a CO2 laser (10.6 μm) over a very short time interval (Nerry, Stoll, and Kologo Citation1991). The backscattered coefficient is then extracted by comparing data acquired with and without the CO2 laser. The surface directional emissivity is therefore obtained if a relationship between backscattered coefficients and directional emissivity is developed. This relationship is a large challenge and very promising way to map LSE. Many efforts should be made in the near future in this topic.
6.8. Validation of the LSE at the satellite pixel scale
Validation is the process of independently assessing the uncertainty of the data products derived from the system outputs. Without validation, any methods, models, algorithms, and parameters derived from remotely sensed data cannot be confidently used. Validation is the most important and urgent issue to be dealt with, as stated by Li et al. (Citation2009). Due to the surface heterogeneity, the validation of LSEs at the satellite pixel scale using the ‘point’ scale measurements over non-uniform and heterogeneous surfaces is questionable. The lack of LSEs at the satellite pixel scale for true validation limits the improvement and development of the LSE retrieval methods from space. Although two general methods have been proposed to validate LSE, as stated in Section 5, appropriate methods for validating LSEs at the satellite pixel scale must be developed and the validation must be performed to provide feedback and some clues to improve the accuracy of the retrieved LSEs. Up to now, the high-resolution (100 m) North American ASTER Land Surface Emissivity Database (NAALSED) Version 2.0 has been released and validated (Hulley and Hook Citation2009b; Hulley, Hook, and Baldridge Citation2009a). This emissivity database may be used indirectly to validate other LSE products with coarser spatial resolution.
Acknowledgements
This work was jointly supported by the Knowledge Innovation Project (KZCX2-YW-Q10-2) and by the Hi-Tech Research and Development Programme of China (863 Plan Programme) under Grants 2008AA121805 and 2009AA122102.
References
- Abrams , M. 2000 . The Advanced Spaceborne Thermal Emission and Reflection Radiometer (ASTER): Data Products for the High Spatial Resolution Imager on NASA's Terra Platform . International Journal of Remote Sensing , 21 : 847 – 59 .
- Baldridge , A. M. , Hook , S. J. , Grove , C. I. and Rivera , G. 2009 . The ASTER Spectral Library Version 2.0 . Remote Sensing of Environment , 113 : 711 – 15 .
- Barducci , A. and Pippi , I. 1996 . Temperature and Emissivity Retrieval from Remotely Sensed Images Using the ‘Grey Body Emissivity’ Method . IEEE Transactions on Geoscience and Remote Sensing , 34 : 681 – 95 .
- Becker , F. and Li , Z.-L. 1990a . Temperature-Independent Spectral Indices in Thermal Infrared Bands . Remote Sensing of Environment , 32 : 17 – 33 .
- Becker , F and Li , Z.-L. 1990b . Towards a Local Split Window Method Over Land Surfaces . International Journal of Remote Sensing , 11 : 369 – 93 .
- Becker , F. and Li , Z.-L. 1995 . Surface Temperature and Emissivity at Various Scales: Definition, Measurement and Related Problems . Remote Sensing Reviews , 12 : 225 – 53 .
- Becker , F. , Ramanantsizehena , P. and Stoll , M. 1985 . Angular Variation of the Bidirectional Reflectance of Bare Soils in the Thermal Infrared Band . Applied Optics , 24 : 365 – 75 .
- Blondin , C. 1991 . “ Parameterization of Land-Surface Processes in Numerical Weather Prediction ” . In Land Surface Evaporation: Measurement and Parameterization , Edited by: Schmugge , T. J. and Andre , J. 31 – 54 . New York : Springer .
- Borel , C. C. Iterative Retrieval of Surface Emissivity and Temperature for a Hyperspectral Sensor . First JPL Workshop on Remote Sensing of Land Surface Emissivity . May 6–8 . pp. 1 – 5 . Pasadena , , CA
- Borel , C. C. Surface Emissivity and Temperature Retrieval for a Hyperspectral Sensor . Proceedings of the 1998 IEEE International Geoscience and Remote Sensing Symposium . July 6–10 . pp. 546 – 9 . Seattle , , WA
- Borel , C. C. 2008 . Error Analysis for a Temperature and Emissivity Retrieval Algorithm for Hyperspectral Imaging Data . International Journal of Remote Sensing , 29 : 5029 – 45 .
- Carlson , T. N. and Ripley , D. A. 1997 . On the Relation between NDVI, Fractional Vegetation Cover, and Leaf Area Index . Remote Sensing of Environment , 62 : 241 – 52 .
- Caselles , V. and Sobrino , J. A. 1989 . Determination of Frosts in Orange Groves from NOAA-9 AVHRR Data . Remote Sensing of Environment , 29 : 135 – 46 .
- Caselles , V. , Valor , E. , Coll , C. and Rubio , E. 1997 . Thermal Band Selection for the PRISM Instrument .1. Analysis of Emissivity-Temperature Separation Algorithms . Journal of Geophysical Research , 102 : 11145 – 64 .
- Chandrasekhar , S. 1960 . Radiative Transfer , 1 – 393 . New York : Dover .
- Chen , K. S. , Wu , T. D. , Tsang , L. , Li , Q. , Shi , J. C. and Fung , A. K. 2003 . Emission of Rough Surfaces Calculated by the Integral Equation Method with Comparison to Three-Dimensional Moment Method Simulations . IEEE Transactions on Geoscience and Remote Sensing , 41 : 90 – 101 .
- Chen , L. F. , Li , Z.-L. , Liu , Q. H. , Chen , S. , Tang , Y. and Zhong , B. 2004 . Definition of Component Effective Emissivity for Heterogeneous and Non-Isothermal Surfaces and Its Approximate Calculation . International Journal of Remote Sensing , 25 : 231 – 44 .
- Cheng , J. , Liang , S. , Liu , Q. and Li , X. 2011 . Temperature and Emissivity Separation from Ground-Based MIR Hyperspectral Data . IEEE Transactions on Geoscience and Remote Sensing , 49 : 1473 – 84 .
- Cheng , J. , Liang , S. , Wang , J. and Li , X. 2010 . A Stepwise Refining Algorithm of Temperature and Emissivity Separation for Hyperspectral Thermal Infrared Data . IEEE Transactions on Geoscience and Remote Sensing , 48 : 1588 – 97 .
- Cheng , J. , Liu , Q. H. , Li , X. W. , Xiao , Q. , Liu , Q. and Du , Y. M. 2008 . Correlation-Based Temperature and Emissivity Separation Algorithm . Science in China (Series D) , 51 : 357 – 69 .
- Coll , C. , Caselles , V. , Rubio , E. , Sospedra , F. and Valor , E. 2001 . Temperature and Emissivity Separation from Calibrated Data of the Digital Airborne Imaging Spectrometer . Remote Sensing of Environment , 76 : 250 – 9 .
- Coll , C. , Caselles , V. , Valor , E. , Niclòs , R. , ánchez , J. M. S , Galve , J. M. and Mira , M. 2007 . Temperature and Emissivity Separation from ASTER Data for Low Spectral Contrast Surfaces . Remote Sensing of Environment , 110 : 162 – 75 .
- Coll , C. , Caselles , V. , Valor , E. and Rubio , E. 2003a . Validation of Temperature-Emissivity Separation and Split-Window Methods from TIMS Data and Ground Measurements . Remote Sensing of Environment , 85 : 232 – 42 .
- Coll , C. , Valor , E. , Caselles , V. and Niclos , R. 2003b . Adjusted Normalized Emissivity Method for Surface Temperature and Emissivity Retrieval from Optical and Thermal Infrared Remote Sensing Data . Journal of Geophysical Research , 108 : 4739
- Conel , J. E. 1969 . Infrared Emissivities of Silicates: Experimental Results and a Cloudy Atmosphere Model of Spectral Emission from Condensed Particulate Mediums . Journal of Geophysical Research , 74 : 1614 – 34 .
- Cuenca , J. and Sobrino , J. A. 2004 . Experimental Measurements for Studying Angular and Spectral Variation of Thermal Infrared Emissivity . Applied Optics , 43 : 4598 – 602 .
- Dash , P. , Gottsche , F. M. and Olesen , F. S. 2003 . Emissivity and Temperature Estimation from MSG SEVIRI Data; Method Validation with Simulated and NOAA-14 AVHRR Data . Advances in Space Research , 32 : 2241 – 6 .
- Dash , P. , Gottsche , F. M. , Olesen , F. S. and Fischer , H. 2005 . Separating Surface Emissivity and Temperature Using Two-Channel Spectral Indices and Emissivity Composites and Comparison with a Vegetation Fraction Method . Remote Sensing of Environment , 96 : 1 – 17 .
- Farmer , V. C. 1974 . The Infrared Spectra of Minerals , 1 – 539 . London : Mineralogical Society .
- Faysash , D. A. and Smith , E. A. 1999 . Simultaneous Land Surface Temperature-Emissivity Retrieval in the Infrared Split Window . Journal of Atmospheric and Oceanic Technology , 16 : 1673 – 89 .
- Faysash , D. A. and Smith , E. A. 2000 . Simultaneous Retrieval of Diurnal to Seasonal Surface Temperatures and Emissivities over SGP ARM-CART Site Using GOES Split Window . Journal of Applied Meteorology , 39 : 971 – 82 .
- Gillespie , A. R. Lithologic Mapping of Silicate Rocks Using TIMS . Proceedings of the Thermal Infrared Multispectral Scanner Data User's Workshop . June 18–19 . pp. 29 – 44 . Pasadena , , CA
- Gillespie , A. R. , Rokugawa , S. , Hook , S. J. , Matsunaga , T. and Kahle , A. B. 1996 . Temperature/Emissivity Separation Algorithm Theoretical Basis Document, Version 2.4 , 1 – 64 . Washington , DC : NASA/GSFC .
- Gillespie , A. R. , Rokugawa , S. , Matsunaga , T. , Cothern , J. S. , Hook , S. and Kahle , A. B. 1998 . A Temperature and Emissivity Separation Algorithm for Advanced Spaceborne Thermal Emission and Reflection Radiometer (ASTER) Images . IEEE Transactions on Geoscience and Remote Sensing , 36 : 1113 – 26 .
- Goita , K. and Royer , A. 1997 . Surface Temperature and Emissivity Separability over Land Surface from Combined TIR and SWIR AVHRR Data . IEEE Transactions on Geoscience and Remote Sensing , 35 : 718 – 33 .
- Gu , D. , Gillespie , A. R. , Kahle , A. B. and Palluconi , F. D. 2000 . Autonomous Atmospheric Compensation (AAC) of High Resolution Hyperspectral Thermal Infrared Remote-Sensing Imagery . IEEE Transactions on Geoscience and Remote Sensing , 38 : 2557 – 70 .
- Gustafson , W. T. , Gillespie , A. R. and Yamada , G. J. Revisions to the ASTER Temperature/Emissivity Separation Algorithm . Proceedings of 2nd Recent Advances in Quantitative Remote Sensing . September 25–29 . pp. 770 – 5 . Valencia , , Spain : Torrent .
- Hook , S. J. , Gabell , A. R. , Green , A. A. and Kealy , P. S. 1992 . A Comparison of Techniques for Extracting Emissivity Information from Thermal Infrared Data for Geologic Studies . Remote Sensing of Environment , 42 : 123 – 35 .
- Hori , M. , Aoki , T. , Tanikawa , T. , Motoyoshi , H. , Hachikubo , A. , Sugiura , K. , Yasunari , T. J. , Eide , H. , Storvold , R. and Nakajima , Y. 2006 . In-Situ Measured Spectral Directional Emissivity of Snow and Ice in the 8–14 μm Atmospheric Window . Remote Sensing of Environment , 100 : 486 – 502 .
- Hulley , G. C. and Hook , S. J. 2009a . Intercomparison of Versions 4, 4.1 and 5 of the MODIS Land Surface Temperature and Emissivity Products and Validation with Laboratory Measurements of Sand Samples from the Namib Desert, Namibia . Remote Sensing of Environment , 113 : 1313 – 18 .
- Hulley , G. C. and Hook , S. J. 2009b . The North American ASTER Land Surface Emissivity Database (NAALSED) Version 2.0 . Remote Sensing of Environment , 113 : 1967 – 75 .
- Hulley , G. C. , Hook , S. J. and Baldridge , A. M. 2009a . Validation of the North American ASTER Land Surface Emissivity Database (NAALSED) Version 2.0 Using Pseudo-Invariant Sand Dune Sites . Remote Sensing of Environment , 113 : 2224 – 33 .
- Hulley , G. C. , Hook , S. J. and Baldridge , A. M. 2010 . Investigating the Effects of Soil Moisture on Thermal Infrared Land Surface Temperature and Emissivity Using Satellite Retrievals and Laboratory Measurements . Remote Sensing of Environment , 114 : 1480 – 93 .
- Hulley , G. C. , Hook , S. J. , Manning , E. , Lee , S. Y. and Fetzer , E. 2009b . Validation of the Atmospheric Infrared Sounder (AIRS) Version 5 Land Surface Emissivity Product over the Namib and Kalahari Deserts . Journal of Geophysical Research , 114 : D19104 doi: 10.1029/2009JD012351
- Ingram , P. M. and Muse , A. H. 2001 . Sensitivity of Iterative Spectrally Smooth Temperature/Emissivity Separation to Algorithmic Assumptions and Measurement Noise . IEEE Transactions on Geoscience and Remote Sensing , 39 : 2158 – 67 .
- Jacob , F. , Petitcolin , F. , Schmugge , T. , Vermote , E. , French , A. and Ogawa , K. 2004 . Comparison of Land Surface Emissivity and Radiometric Temperature Derived from MODIS and ASTER Sensors . Remote Sensing of Environment , 90 : 137 – 52 .
- Jaggi , S. , Quattrochi , D. and Baskin , R. An Algorithm for the Estimation of Bounds on the Emissivity and Temperatures from Thermal Multispectral Airborne Remotely Sensed Data . Summaries of the Third Annual JPL Airborne Geoscience Workshop . June 1–5 . pp. 22 – 4 . Pasadena , , CA
- Jiang , G. M. and Li , Z.-L. 2008 . Intercomparison of Two BRDF Models in the Estimation of the Directional Emissivity in MIR Channel from MSG1-SEVIRI Data . Optics Express , 16 : 19310 – 21 .
- Jiang , G. M. , Li , Z.-L. and Nerry , F. 2006 . Land Surface Emissivity Retrieval from Combined Mid-Infrared and Thermal Infrared Data of MSG-SEVIRI . Remote Sensing of Environment , 105 : 326 – 40 .
- Jimenez-Munoz , J. C. , Sobrino , J. A. , Gillespie , A. , Sabol , D. and Gustafson , W. T. 2006 . Improved Land Surface Emissivities over Agricultural Areas Using ASTER NDVI . Remote Sensing of Environment , 103 : 474 – 87 .
- Jin , M. and Liang , S. 2006 . Improved Emissivity Parametrization for Land Surface Modeling Using Global Remote Sensing Observations . Journal of Climate , 19 : 2867 – 81 .
- Kahle , A. B. , Madura , D. P. and Soha , J. M. 1980 . Middle Infrared Multispectral Aircraft Scanner Data: Analysis for Geological Applications . Applied Optics , 19 : 2279 – 90 .
- Kanani , K. , Poutier , L. , Nerry , F. and Stoll , M. 2007 . Directional Effects Consideration to Improve Out-Doors Emissivity Retrieval in the 3–13 μm Domain . Optics Express , 15 : 12464 – 82 .
- Labed , J. and Stoll , M. P. 1991 . Angular Variation of Land Surface Spectral Emissivity in the Thermal Infrared: Laboratory Investigations on Bare Soils . International Journal of Remote Sensing , 12 : 2299 – 310 .
- Lenoble , J. 1985 . Radiative Transfer in Scattering and Absorbing Atmospheres: Standard Computational Procedures , 1 – 300 . Hampton : A. Deepak .
- Li , J. , Li , J. , Weisz , E. and Zhou , D. K. 2007 . Physical Retrieval of Surface Emissivity Spectrum from Hyperspectral Infrared Radiances . Geophysical Research Letters , 34 : L16812
- Li , J. , Li , Z. , Jin , X. , Schmit , T. J. , Zhou , L. and Goldberg , M. D. 2011 . Land Surface Emissivity from High Temporal Resolution Geostationary Infrared Imager Radiances: Methodology and Simulation Studies . Journal of Geophysical Research , 116 : D01304
- Li , X. W. and Strahler , A. H. 1992 . Geometric-Optical Bidirectional Reflectance Modeling of the Discrete Crown Vegetation Canopy: Effect of Crown Shape and Mutual Shadowing . IEEE Transactions on Geoscience and Remote Sensing , 30 : 276 – 92 .
- Li , X. W. , Strahler , A. H. and Friedl , M. A. 1999a . A Conceptual Model for Effective Directional Emissivity from Nonisothermal Surfaces . IEEE Transactions on Geoscience and Remote Sensing , 37 : 2508 – 17 .
- Li , X. W. and Wang , J. D. 1999 . The Definition of Effective Emissivity of Land Surface at the Scale of Remote Sensing Pixels . Chinese Science Bulletin , 44 : 2154 – 8 .
- Li , Z.-L. and Becker , F. 1990 . Properties and Comparison of Temperature-Independent Thermal Infrared Spectral Indices with NDVI for HAPEX Data . Remote Sensing of Environment , 33 : 165 – 82 .
- Li , Z.-L. and Becker , F. 1993 . Feasibility of Land Surface-Temperature and Emissivity Determination from AVHRR Data . Remote Sensing of Environment , 43 : 67 – 85 .
- Li , Z.-L. , Becker , F. , Stoll , M. P. and Wan , Z. M. 1999b . Evaluation of Six Methods for Extracting Relative Emissivity Spectra from Thermal Infrared Images . Remote Sensing of Environment , 69 : 197 – 214 .
- Li , Z.-L. , Becker , F. , Stoll , M. P. , Wan , Z. M. and Zhang , Y. L. 1999c . Channel Selection for Soil Spectrum Reconstruction in 8–13 um Region . Journal of Geophysical Research , 104 : 22271 – 85 .
- Li , Z.-L. , Petitcolin , F. and Zhang , R. H. 2000 . A Physically Based Algorithm for Land Surface Emissivity Retrieval from Combined Mid-Infrared and Thermal Infrared Data . Science in China (Series E) , 43 : 23 – 33 .
- Li , Z.-L. , Tang , R. L. , Wan , Z. M. , Bi , Y. Y. , Zhou , C. H. , Tang , B. H. , Yan , G. J. and Zhang , X. Y. 2009 . A Review of Current Methodologies for Regional Evapotranspiration Estimation from Remotely Sensed Data . Sensors , 9 : 3801 – 53 .
- Liang , S. 2001 . An Optimization Algorithm for Separating Land Surface Temperature and Emissivity from Multispectral Thermal Infrared Imagery . IEEE Transactions on Geoscience and Remote Sensing , 39 : 264 – 74 .
- Liang , S. 2004 . Quantitative Remote Sensing of Land Surfaces , 377 – 87 . Hoboken, NJ : John Wiley & Sons .
- Liang , S. L. , Fang , H. L. , Chen , M. Z. , Shuey , C. J. , Walthall , C. , Daughtry , C. , Morisette , J. , Schaaf , C. and Strahler , A. 2002 . Validating MODIS Land Surface Reflectance and Albedo Products: Methods and Preliminary Results . Remote Sensing of Environment , 83 : 149 – 62 .
- Lucht , W. 1998 . Expected Retrieval Accuracies of Bidirectional Reflectance and Albedo from EOS-MODIS and MISR Angular Sampling . Journal of Geophysical Research , 103 : 8763 – 78 .
- Lucht , W. and Roujean , J. 2000 . Considerations in the Parametric Modeling of BRDF and Albedo from Multiangular Satellite Sensor Observations . Remote Sensing Reviews , 18 : 343 – 79 .
- Ma , X. L. , Wan , Z. M. , Moeller , C. C. , Menzel , W. P. and Gumley , L. E. 2002 . Simultaneous Retrieval of Atmospheric Profiles, Land-Surface Temperature, and Surface Emissivity from Moderate-Resolution Imaging Spectroradiometer Thermal Infrared Data: Extension of a Two-Step Physical Algorithm . Applied Optics , 41 : 909 – 24 .
- Ma , X. L. , Wan , Z. M. , Moeller , C. C. , Menzel , W. P. , Gumley , L. E. and Zhang , Y. L. 2000 . Retrieval of Geophysical Parameters from Moderate Resolution Imaging Spectroradiometer Thermal Infrared Data: Evaluation of a Two-Step Physical Algorithm . Applied Optics , 39 : 3537 – 50 .
- Menzel , W. P. , Seemann , S. W. , Li , J. and Gumley , L. E. 2006 . MODIS Atmospheric Profile Retrieval Algorithm Theoretical Basis Document, Version 6 , 1 – 40 . Washington , DC : NASA/GSFC .
- Minnaert , M. 1941 . The Reciprocity Principle of Linear Photometry . Astrophysical Journal , 93 : 403 – 10 .
- Mira , M. , Valor , E. , Boluda , R. , Caselles , V. and Coll , C. 2007 . Influence of Soil Water Content on the Thermal Infrared Emissivity of Bare Soils: Implication for Land Surface Temperature Determination . Journal of Geophysical Research , 112 : F04003
- Mira , M. , Valor , E. , Caselles , V. , Rubio , E. , Coll , C. , Galve , J. M. , Niclos , R. , Sanchez , J. M. and Boluda , R. 2010 . Soil Moisture Effect on Thermal Infrared (8–13 μm) Emissivity . IEEE Transactions on Geoscience and Remote Sensing , 48 : 2251 – 60 .
- Momeni , M. and Saradjian , M. R. 2007 . Evaluating NDVI-based Emissivities of MODIS Bands 31 and 32 Using Emissivities Derived by Day/Night LST Algorithm . Remote Sensing of Environment , 106 : 190 – 8 .
- Morisette , J. T. , Privette , J. L. and Justice , C. O. 2002 . A Framework for the Validation of MODIS Land Products . Remote Sensing of Environment , 83 : 77 – 96 .
- Mushkin , A. , Balick , L. K. and Gillespie , A. R. 2005 . Extending Surface Temperature and Emissivity Retrieval to the Mid-Infrared (3–5 um) Using the Multispectral Thermal Imager (MTI) . Remote Sensing of Environment , 98 : 141 – 51 .
- Nerry , F. , Petitcolin , F. and Stoll , M. P. 1998 . Bidirectional Reflectivity in AVHRR Channel 3: Application to a Region in Northern Africa . Remote Sensing of Environment , 66 : 298 – 316 .
- Nerry , F. , Stoll , M. P. and Kologo , N. 1991 . Scattering of a CO2 Laser Beam at 10.6 um by Bare Soils: Experimental Study of the Polarized Bidirectional Scattering Coefficient; Model and Comparison with Directional Emissivity Measurements . Applied Optics , 30 : 3984 – 94 .
- Norman , J. M. and Becker , F. 1995 . Terminology in Thermal Infrared Remote Sensing of Natural Surfaces . Agricultural and Forest Meteorology , 77 : 153 – 66 .
- Ogawa , K. , Schmugge , T. , Jacob , F. and French , A. 2002 . Estimation of Broadband Land Surface Emissivity from Multi-Spectral Thermal Infrared Remote Sensing . Agronomie , 22 : 695 – 6 .
- Ogawa , K. , Schmugge , T. , Jacob , F. and French , A. 2003 . Estimation of Land Surface Window (8–12 um) Emissivity from Multispectral Thermal Infrared Remote Sensing: A Case Study in a Part of Sahara Desert . Geophysical Research Letters , 30 : 1067
- Ogawa , K. , Schmugge , T. and Rokugawa , S. 2006 . Observations of the Dependence of the Thermal Infrared Emissivity on Soil Moisture . Geophysical Research Abstracts , 8 : 04996
- OuYang , X. , Wang , N. , Wu , H. and Li , Z.-L. 2010 . Errors Analysis on Temperature and Emissivity Determination from Hyperspectral Thermal Infrared Data . Optics Express , 18 : 544 – 50 .
- Payan , V. and Royer , A. 2004 . Analysis of Temperature Emissivity Separation (TES) Algorithm Applicability and Sensitivity . International Journal of Remote Sensing , 25 : 15 – 37 .
- Peres , L. F. and DaCamara , C. C. 2004 . Land Surface Temperature and Emissivity Estimation Based on the Two-Temperature Method: Sensitivity Analysis Using Simulated MSG/SEVIRI Data . Remote Sensing of Environment , 91 : 377 – 89 .
- Peres , L. F. and DaCamara , C. C. 2005 . Emissivity Maps to Retrieve Land-Surface Temperature from MSG/SEVIRI . IEEE Transactions on Geoscience and Remote Sensing , 43 : 1834 – 44 .
- Petitcolin , F. , Nerry , F. and Stoll , M. P. 2002 . Mapping Temperature Independent Spectral Indice of Emissivity and Directional Emissivity in AVHRR Channels 4 and 5 . International Journal of Remote Sensing , 23 : 3473 – 91 .
- Petitcolin , F. and Vermote , E. 2002 . Land Surface Reflectance, Emissivity and Temperature from MODIS Middle and Thermal Infrared Data . Remote Sensing of Environment , 83 : 112 – 34 .
- Prata , A. J. 1993 . Land Surface Temperatures Derived from the Advanced Very High-Resolution Radiometer and the Along-Track Scanning Radiometer 1. Theory . Journal of Geophysical Research , 98 : 16689 – 702 .
- Realmuto , V. J. Separating the Effects of Temperature and Emissivity: Emissivity Spectrum Normalization . Proceedings of the Second TIMS Workshop . June 6 23–7 . Pasadena , , CA
- Ribeiro da Luz , B. and Crowley , J. K. 2007 . Spectral Reflectance and Emissivity Features of Broad Leaf Plants: Prospects for Remote Sensing in the Thermal Infrared (8.0–14.0 μm) . Remote Sensing of Environment , 109 : 393 – 405 .
- Roujean , J. L. , Leroy , M. and Deschamps , P. Y. 1992 . A Bidirectional Reflectance Model of the Earth's Surface for the Correction of Remote Sensing Data . Journal of Geophysical Research , 97 : 20455 – 68 .
- Rubio , E. , Caselles , V. and Badenas , C. 1997 . Emissivity Measurements of Several Soils and Vegetation Types in the 8–14 μm Waveband: Analysis of Two Field Methods . Remote Sensing of Environment , 59 : 490 – 521 .
- Rubio , E. , Caselles , V. , Coll , C. , Valour , E. and Sospedra , F. 2003 . Thermal–Infrared Emissivities of Natural Surfaces: Improvements on the Experimental Set-up and New Measurements . International Journal of Remote Sensing , 24 : 5379 – 90 .
- Sabol , D. E. Jr , Gillespie , A. R. , Abbott , E. and Yamada , G. 2009 . Field Validation of the ASTER Temperature–Emissivity Separation Algorithm . Remote Sensing of Environment , 113 : 2328 – 44 .
- Salisbury , J. W. and D'Aria , D. M. 1992 . Emissivity of Terrestrial Materials in the 8–14 μm Atmospheric Window . Remote Sensing of Environment , 42 : 83 – 106 .
- Salisbury , J. W. , Wald , A. and D'Aria , D. M. 1994 . Thermal-Infrared Remote-Sensing and Kirchhoff Law .1. Laboratory Measurements . Journal of Geophysical Research , 99 : 11897 – 911 .
- Schmugge , T. , French , A. , Ritchie , J. C. , Rango , A. and Pelgrum , H. 2002 . Temperature and Emissivity Separation from Multispectral Thermal Infrared Observations . Remote Sensing of Environment , 79 : 189 – 98 .
- Snyder , W. C. , Wan , Z. , Zhang , Y. and Feng , Y. Z. 1998 . Classification-Based Emissivity for Land Surface Temperature Measurement from Space . International Journal of Remote Sensing , 19 : 2753 – 74 .
- Snyder , W. C. and Wan , Z. M. 1998 . BRDF Models to Predict Spectral Reflectance and Emissivity in the Thermal Infrared . IEEE Transactions on Geoscience and Remote Sensing , 36 : 214 – 25 .
- Sobrino , J. A. and Cuenca , J. 1999 . Angular Variation of Thermal Infrared Emissivity for Some Natural Surfaces from Experimental Measurements . Applied Optics , 38 : 3931 – 6 .
- Sobrino , J. A. , Jimenez-Munoz , J. C. , Labed-Nachbrand , J. and Nerry , F. 2002 . Surface Emissivity Retrieval from Digital Airborne Imaging Spectrometer Data . Journal of Geophysical Research , 107 : 4729
- Sobrino , J. A. , Jimenez-Munoz , J. C. and Paolini , L. 2004 . Land Surface Temperature Retrieval from LANDSAT TM 5 . Remote Sensing of Environment , 90 : 434 – 40 .
- Sobrino , J. A. , Jimenez-Munoz , J. C. , Soria , G. , Romaguera , M. , Guanter , L. , Moreno , J. , Plaza , A. and Martincz , P. 2008 . Land Surface Emissivity Retrieval from Different VNIR and TIR Sensors . IEEE Transactions on Geoscience and Remote Sensing , 46 : 316 – 27 .
- Sobrino , J. A. , Jimenez-Munoz , J. C. and Verhoef , W. 2005 . Canopy Directional Emissivity: Comparison between Models . Remote Sensing of Environment , 99 : 304 – 14 .
- Sobrino , J. A. , Kharraz , J. E. and Li , Z.-L. 2003 . Surface Temperature and Water Vapour Retrieval from MODIS Data . International Journal of Remote Sensing , 24 : 5161 – 82 .
- Sobrino , J. A. and Raissouni , N. 2000 . Toward Remote Sensing Methods for Land Cover Dynamic Monitoring: Application to Morocco . International Journal of Remote Sensing , 21 : 353 – 66 .
- Sobrino , J. A. , Raissouni , N. and Li , Z.-L. 2001 . A Comparative Study of Land Surface Emissivity Retrieval from NOAA Data . Remote Sensing of Environment , 75 : 256 – 66 .
- Sun , D. L. and Pinker , R. T. 2003 . Estimation of Land Surface Temperature from a Geostationary Operational Environmental Satellite (GOES-8) . Journal of Geophysical Research , 108 : 4326
- Tang , B. H. , Wu , H. , Li , C. R. and Li , Z.-L. 2011 . Estimation of Broadband Surface Emissivity from Narrowband Emissivities . Optics Express , 19 : 185 – 92 .
- Trigo , I. F. , Peres , L. F. , DaCamara , C. C. and Freitas , S. C. 2008 . Thermal Land Surface Emissivity Retrieved from SEVIRI/Meteosat . IEEE Transactions on Geoscience and Remote Sensing , 46 : 307 – 15 .
- Urai , M. , Matsunaga , T. and Ishii , T. 1997 . Relationship between Soil Moisture Content and Thermal Infrared Emissivity of the Sand Sampled in Muus Desert, China . Remote Sensing Society of Japan , 17 : 322 – 31 .
- Valor , E. and Caselles , V. 1996 . Mapping Land Surface Emissivity from NDVI: Application to European, African, and South American Areas . Remote Sensing of Environment , 57 : 167 – 84 .
- Van de Griend , A. A. and Owe , M. 1993 . On the Relationship between Thermal Emissivity and the Normalized Difference Vegetation Index for Natural Surfaces . International Journal of Remote Sensing , 14 : 1119 – 31 .
- Wan , Z. M. 1999 . MODIS Land Surface Temperature Algorithm Theoretical Basis Document, Version 3.3 , Washington , DC : NASA/GSFC, 1–77 .
- Wan , Z. M. 2008 . New Refinements and Validation of the MODIS Land-Surface Temperature/Emissivity Products . Remote Sensing of Environment , 112 : 59 – 74 .
- Wan , Z. M. and Dozier , J. 1996 . A Generalized Split-Window Algorithm for Retrieving Land-Surface Temperature from Space . IEEE Transactions on Geoscience and Remote Sensing , 34 : 892 – 905 .
- Wan , Z. M. and Li , Z.-L. 1997 . A Physics-Based Algorithm for Retrieving Land-Surface Emissivity and Temperature from EOS/MODIS Data . IEEE Transactions on Geoscience and Remote Sensing , 35 : 980 – 96 .
- Wan , Z. M. and Li , Z.-L. 2010 . “ MODIS Land Surface Temperature and Emissivity Products ” . In Land Remote Sensing and Global Environmental Changes , Edited by: Ramachandran , B. , Justice , C. O. and Abrams , M. J. 563 – 77 . London : Springer .
- Wan , Z. M. , Zhang , Y. L. , Zhang , Q. C. and Li , Z.-L. 2002 . Validation of the Land-Surface Temperature Products Retrieved from Terra Moderate Resolution Imaging Spectroradiometer Data . Remote Sensing of Environment , 83 : 163 – 80 .
- Wang , K. and Liang , S. L. 2009 . Evaluation of ASTER and MODIS Land Surface Temperature and Emissivity Products Using Long-Term Surface Longwave Radiation Observations at SURFRAD Sites . Remote Sensing of Environment , 113 : 1556 – 65 .
- Wang , K. , Wan , Z. , Wang , P. , Sparrow , M. , Liu , J. and Haginoya , S. 2007 . Evaluation and Improvement of the MODIS Land Surface Temperature/Emissivity Products Using Ground-Based Measurements at a Semi-Desert Site on the Western Tibetan Plateau . International Journal of Remote Sensing , 28 : 2549 – 65 .
- Wang , K. C. , Wan , Z. M. , Wang , P. C. , Sparrow , M. , Liu , J. M. , Zhou , X. J. and Haginoya , S. 2005 . Estimation of Surface Long Wave Radiation and Broadband Emissivity Using Moderate Resolution Imaging Spectroradiometer (MODIS) Land Surface Temperature/Emissivity Products . Journal of Geophysical Research , 110 : D11109
- Wang , N. , Wu , H. , Nerry , F. , Li , C. R. and Li , Z.-L. 2011 . Temperature and Emissivity Retrievals from Hyperspectral Thermal Infrared Data Using Linear Spectral Emissivity Constraint . IEEE Transactions on Geoscience and Remote Sensing , 49 : 1291 – 303 .
- Wanner , W. , Li , X. and Strahler , A. 1995 . On the Derivation of Kernels for Kernel-Driven Models of Bidirectional Reflectance . Journal of Geophysical Research , 100 : 21077
- Watson , K. 1992 . Two-Temperature Method for Measuring Emissivity . Remote Sensing of Environment , 42 : 117 – 21 .
- Xiao , Q. , Liu , Q. H. , Li , X. W. , Chen , L. F. , Liu , Q. and Xin , X. Z. 2003 . A Field Measurement Method of Spectral Emissivity and Research on the Feature of Soil Thermal Infrared Emissivity . Journal of Infrared and Millimeter Waves , 22 : 373 – 8 .
- Young , S. J. , Johnson , B. R. and Hackwell , J. A. 2002 . An In-Scene Method for Atmospheric Compensation of Thermal Hyperspectral Data . Journal of Geophysical Research , 107 : 4774
- Zhou , D. K. , Larar , A. M. , Liu , X. , Smith , W. L. , Strow , L. L. , Yang , P. , Schlüssel , P. and Calbet , X. 2011 . Global Land Surface Emissivity Retrieved from Satellite Ultraspectral IR Measurements . IEEE Transactions on Geoscience and Remote Sensing , 49 : 1277 – 90 .