Abstract
The global long-term leaf area index (LAI) series is a critical variable to validate the terrestrial ecological processes of simulation by Earth system models (ESMs) and ESM input. However, the lack of long-term LAI data restricts studies on the interaction between atmosphere and biosphere. This study focuses on obtaining a robust long-term LAI data set through combining Advanced Very High Resolution Radiometer (AVHRR; available from August 1981 to May 2001) and Moderate Resolution Imaging Spectroradiometer (MODIS; available from January 2000 to December 2009) data sets, and on investigating the relationship between LAI data and the key variables of the climate system. Regional discrepancies in LAI exist between these two data sets. In high northern latitudes, there are significant differences (>1.7 m2 m−2) between AVHRR- and MODIS-derived LAI during the overlapping period from January 2000 to May 2001 because of effects of vegetation structure, low saturation threshold of remote-sensing data and cloud contamination, and the effects of aerosols and atmospheric water vapour on the AVHRR sensor. Using the LAI data set derived from MODIS as the benchmark, AVHRR-derived LAI data from the same periods as those of MODIS were first calibrated through a region-based linear regression method. Then the regression relationship was employed to other periods of AVHRR-derived LAI, resulting in a complete long-term LAI data set. The results showed that the data set has a better convergence and continuity than the original data set, with the regional discrepancies in LAI significantly reduced. Further analyses of correlations between LAI and variables of the climate system demonstrate that the modified LAI is more suitable to describe the response of vegetation to variables in the climate system. This is probably attributed to temperature as the main driver affecting vegetation and to the persistent presence of frozen soil in this region. In comparison with results from previous studies, the response to temperature, precipitation, and soil moisture in the long-term modified LAI data is more reasonable than for unmodified LAI.
1. Introduction
Leaf area index (LAI) is usually defined as the one-sided green leaf area per unit ground area in canopies (Myneni et al. Citation1997; Zheng and Moskal Citation2009). Previous studies have suggested that LAI is one of the most important land-surface parameters for addressing the interaction between terrestrial ecosystem and climate (Running and Nemani Citation1991; Turner et al. Citation1999; Cohen et al. Citation2003; Dan, Ji, and He Citation2007; Garrigues et al. Citation2008). This index has been widely used in physical and biological process studies associated with land-surface vegetation condition such as canopy conductance, photosynthesis, respiration, gross productivity, transpiration, and the canopy’s energy absorption capacity in carbon and hydrology cycle and energy balance (Running and Nemani Citation1991; Knyazikhin et al. Citation1998; Lindroth, Grelle, and Mor Citation1998; Andr, Denis, and Nathalie Citation2000; Chen et al. Citation2002). Perhaps the most specific cases for LAI application are prediction of photosynthesis and estimation of biomass and productivity (Bonan Citation1993; Gower, Kucharik, and Norman Citation1999; Turner et al. Citation1999). In addition, LAI is an indispensable input for various primary production models and global models of biogeochemistry, ecology, climate, and hydrology (Box, Holben, and Kalb Citation1989; Nemani and Running Citation1989; Running and Nemani Citation1991; Chase et al. Citation1996; Buermann et al. Citation2001; Hickler et al. Citation2005).
Some studies have estimated vegetation structure based on in-situ-measured time series of LAI, which provide the potential to explore vegetation characteristics and conditions at site scale (Bréda Citation2003; Chen et al. Citation2006). However, it is difficult to apply such site-scale LAI data to estimate vegetation on either a regional or global scale due to the land’s high surface heterogeneities (Myneni et al. Citation2002). Satellite remote-sensing technology is now one of the most important tools for estimating large-scale LAI information (Colombo et al. Citation2003). Remotely sensed data are a primary source and are essential for monitoring and estimating ecophysiological and biophysical processes (Myneni et al. Citation2002; Soudani et al. Citation2006; Garrigues et al. Citation2008). Currently, the information obtained from the Advanced Very High Resolution Radiometer (AVHRR) and the Moderate Resolution Imaging Spectroradiometer (MODIS) sensors is used to generate LAI products, and is widely used for vegetation monitoring and estimating global change and climate change (Carlson and Ripley Citation1997; Huete et al. Citation1997; Kergoat et al. Citation2002; Myneni, Knyazikhin, and Shabanov Citation2011, Yuan et al. Citation2011; Liu, Liu, and Chen Citation2012).
The LAI product produced by the National Oceanic and Atmospheric Administration (NOAA) AVHRR has been used to monitor the spatial and temporal variability of LAI fields. In several studies, for monitoring changes in vegetation condition, the AVHRR-LAI data set was introduced (Cramer et al. Citation1999; Liu et al. Citation1999; Lucht et al. Citation2002). However, this product is affected by a degree of noise. According to the available information on how data products have been handled, in particular as a process of sensor calibration and in examination of the cloud-cover information accompanying each data set, it is possible that the noise in AVHRR-LAI is triggered by stubborn biases with contribution from satellite drift, incomplete corrections for calibration loss and atmospheric effects (clouds, aerosols, etc.) (Holben Citation1986; Zhou et al. Citation2001; Lucht et al. Citation2002; Nagol, Vermote, and Prince Citation2009), solar zenith angle and viewing angle effects (Tucker et al. Citation2005), low saturation threshold effects (Wang, Liu, and Huete Citation2003), the effects of surface-cover type (Holben Citation1986) (e.g. greater persistence of snow coverage) (Dye and Tucker Citation2003), and other issues in regard to AVHRR products. All of these factors could contribute to the overall reflectance of AVHRR products (Spanner et al. Citation1990) because these effects might introduce bias (Kang et al. Citation2003; Zhou et al. Citation2003). For example, the errors for AVHRR NDVI are affected by about 90% of the solar zenith angle at all latitudes (Tucker et al. Citation2005). Thus removal of these effects is very important for reconstructing the LAI data without variation in solar zenith angle. Hence, to perform long-term time series analyses, it is important to evaluate and correct AVHRR-LAI against other data sources. Fortunately, the improved MODIS-LAI product provides an opportunity to solve this problem (van Leeuwen, Huete, and Laing Citation1999; Vermote, El Saleous, and Justice Citation2002; Myneni, Knyazikhin, and Shabanov Citation2011; Xiao et al. Citation2011).
Since the MODIS satellite was launched in 2000, the MODIS-derived LAI has better navigation and atmospheric correction in reducing geometric distortions and improving radiometric sensitivity in the MODIS processing stream (Wolfe et al. Citation2002; Jensen et al. Citation2011). MODIS products are expected to be an improvement on the products derived from the AVHRR sensor (Justice et al. Citation1998). Cohen et al. (Citation2003) suggested that there is a high level of agreement between the MODIS-LAI product and field-based measurements (Cohen et al. Citation2003). Several studies also compared LAI derived from MODIS with that from other satellites at finer spatial resolution, including Enhanced Thematic Mapper Plus (ETM+) and Système Pour l’Observation de la Terre (SPOT) sensors, and found good agreement (Doraiswamy et al. Citation2004; Wang et al. Citation2004; Soudani et al. Citation2006). Although many robust and satisfactory validations of MODIS have been established, MODIS-LAI only provides a short time series from 2000 to the present.
Given the increasing interest in spatiotemporal patterns of time series and the need for long-term input into climate models, a continuous and long time series of LAI data is urgently needed. There are few studies dealing with how to obtain long-term and continuous LAI data sets for input to global climate modelling. The objective of this study is to provide a long-term LAI series by extending current global LAI products so that they can be applied as the input and validation data for climate change modelling. Therefore, this study reconstructs a continuous long-term data set by combining/extending LAI derived from AVHRR and MODIS from 1981 to 2009 at a global scale (the MODIS-LAI products used were improved in regard to continuity and consistency during the period 2000–2009 by Yuan et al. in Citation2011). First, we established a linear regression between AVHRR- and MODIS-derived LAI during the overlapping periods (i.e. January 2000 to May 2001) at 0.5° resolution. The regression was built monthly. Next, the regression was applied to adjust the whole original AVHRR-derived LAI in order to reconstruct a complete long-term time series from 1982 to 2009. Finally, we compared the modified LAI with the original AVHRR-LAI and evaluated the robustness of the modified LAI based on the relationship between LAI and key variables of the climate system such as temperature, precipitation, and soil moisture.
2. Data
The monthly AVHRR-LAI maps are global and are available for August 1981 to May 2001 at 0.5° resolution. They are produced from cloud-free composite images of the NOAA AVHRR sensor. Although the satellite orbital drift and cloud contamination have been revised, residual biases are reported in previous studies (Fensholt et al. Citation2009). Unlike MODIS, little information on atmospheric composition for use in correction can be obtained, due to its lack of additional spectral channels (Nagol, Vermote, and Prince Citation2009). In addition, for the AVHRR data set, no corrections for aerosol or water vapour effects were applied (Fensholt, Nielsen, and Stisen Citation2006).
The original MODIS-LAI products were derived from MODIS Collection 5 LAI (MOD15A2) global products for ten years covering the period from year 2000, day 49, to year 2009, day 361. The MODIS-LAI product used is a composite from 8 days to 1 month at 1 km resolution. Although it is a monthly composite product, considerable noise is still included. Such noise in LAI can contribute to atmospheric conditions, aerosol, cloud, and other issues, leading to discontinuity of data and spatial inconsistency in time series (Yuan et al. Citation2011). In order to eliminate this restriction on its application, an integrated two-step method was used to derive the improved MODIS-LAI products effectively on a global scale for reducing noise and constructing high-quality LAI time series data sets. This was designed for monitoring of global and regional vegetation activities as climatic modelling input covering the period 2000–2009 in an analysis processed by Dai’s group (Yuan et al. Citation2011). This MODIS-LAI data set contains good robustness to monitor spatiotemporal changes in LAI. We upscaled the MODIS 1 km resolution of LAI data to the spatial resolution of AVHRR-LAI data at 0.5° resolution. In this study, we used this product as reference data to calibrate AVHRR-LAI data and to compare the results based on the correlation between the original and modified LAI. Furthermore, we used the long-term time series of temperature, precipitation, and soil moisture to investigate the correlations with original and modified LAI from 1981 to 2009.
For this study, monthly temperature and precipitation were derived from the gridded Climate Research Unit (CRU) TS 3.1 data set (http://climexp.knmi.nl/). The data set spans the period 1901–2009 and has a grid resolution of 0.5° × 0.5°, as reported by Mitchell and Jones (Citation2005). The gridded monthly soil moisture data have been produced by the NOAA Climate Prediction Center from 1948 to the present (http://www.cpc.ncep.noaa.gov/soilmst/leaky_glb.htm). The monthly soil moisture data have the same resolution of 0.5° as the temperature and precipitation data (van den Dool, Huang, and Fan Citation2003). To match the period of the extended LAI data set, the monthly data sets including temperature, precipitation, and soil moisture were selected over a period of nearly 30 years (August 1981 to December 2009). The length of the time series is close to that of baseline period defined by IPCC (2007) (1971–2000).
3. Results
3.1. Comparison of original AVHRR-LAI and MODIS-LAI
The spatial distribution of average values and standard deviations of the monthly LAI data set derived from AVHRR and MODIS satellites for the three periods August 1981 to December 1999, January 2000 to May 2001, and June 2001 to December 2009 is shown in and . In Eastern Asia, the average AVHRR-derived LAI is about 2.6 m2 m−2. The magnitude of monthly average and standard deviation of AVHRR-derived LAI exceeded 2.3 and 0.9 m2 m−2, respectively, in high northern latitudes (e.g. Northern Asia, Northern Europe, and Central North America) during the period from August 1981 to December 1999. In these regions, MODIS yielded a much lower LAI than AVHRR, ranging from 0.4 m2 m−2 in Northern Asia to 1.0 m2 m−2 in Central North America during the same period. MODIS-derived LAI is about 1.0 m2 m−2 in Eastern Asia, which is partly in agreement with previous studies (Garrigues et al. Citation2008).
Figure 1. Distribution of average (AVE) of AVHRR- and MODIS-derived LAI in the three study periods: August 1981 to December 1999, January 2000 to May 2001, and June 2001 to December 2009; units: m2 m−2.
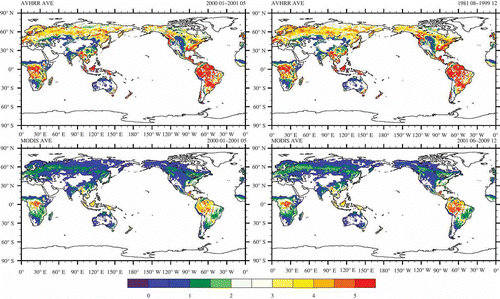
Figure 2. Distribution of standard deviation (SD) of AVHRR- and MODIS-derived LAI in the three study periods: August 1981 to December 1999, January 2000 to May 2001, and June 2001 to December 2009. Units: m2 m−2.
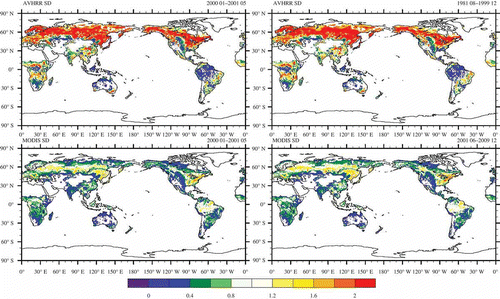
The above comparison of AVHRR-LAI and MODIS-LAI during the overlapping period reveals that the greatest differences in average and standard deviation between the two remote sensors occur in the high latitudes, which incorporate both broad-leaved and coniferous forest. These differences are over 1.7 m2 m−2 in Eastern North America, Northern Asia, and Northern Europe. As a whole, for about 70.4% of the study area, the mean and standard deviation of AVHRR-derived LAI are bigger than those of MODIS-derived LAI. Spatial factors in the difference between AVHRR-LAI and MODIS-LAI in the more densely broad-leaved forest and coniferous forest are shown in and .
3.2. Comparison between unmodified and modified LAI
The data set of monthly MODIS-derived LAI is more accurate than that from AVHRR, and the greatest discrepancy between the two sensors was investigated in the regions mentioned above. Thus, we used MODIS-derived LAI as the benchmark to calibrate the original AVHRR-derived LAI. A region-based linear regression method was adopted based on the relationship between MODIS-LAI and AVHRR-LAI values during the overlapping periods. The adjusted AVHRR data were processed using the method described by (Peng et al., ‘Effects of Increased CO2’, 2012):
where LAIAi is the value of AVHRR-LAI of the ith region, LAIMi is the value of MODIS-LAI for the ith region, b0i is the slope for the ith region, b1i is the intercept of ith region, and ϵi is the random error in the ith region.
Numbers of samples included for individual pixels in this analysis are sufficient (the statistical samples in most regions are >100, except Sahara), whereas the numbers based on the pixel-based linear regression method are very limited (<17). Table 1 presents statistical linear regressions of 21 regions across all areas except Antarctica and those further north than 80° N. The regions shown in Figure 3 were described in detail by (Peng, Dong, Yuan, et al. 2012). For region-based linear regression results, if slope values are under 1.0 and intercepts are unequal to 0.0, this means there is a difference in LAI values between MODIS and AVHRR sensors during the overlapping period. For all statistical regions, the linear regression calculated by Equation (1) for the LAI data of the two sensors is statistically significant at 0.05. The slope values range from 0.07 to 0.67 and the intercept values vary from –0.39 m2 m−2 (minimum) to 1.55 m2 m−2 (maximum). The correlation coefficients (r) between original AVHRR-LAI and MODIS-LAI during the overlapping period for all 21 regions ranged from 0.19 in Southern South America to 0.92 in Southern Asia. The statistical regression results for the Amazon Basin for LAI from MODIS and AVHRR show slope and intercept values of 0.67 and –0.30 m2 m−2, respectively, while for Northern Asia, these values are 0.26 and 0.19 m2 m−2, respectively. At regional and global scales, general agreement between the time series of modified AVHRR and MODIS values from two sensors was found ( and ).
Table 1. Regression between unmodified AVHRR- and MODIS-derived LAI for 21 regions during the overlapping period from January 2000 to May 2001 (b0 is the slope and b1 is the intercept, as mentioned in Equation (1)). r is the correlation coefficient of regression, p is the significance level, and n is the statistical sample number
Figure 4. Monthly average time series of original AVHRR-LAI (AVHRR_LAI), modified AVHRR-LAI (MD_AVHRR_LAI), and MODIS-LAI (MODIS_LAI) over the world, Amazon Basin, Eastern Asia, and Northern Asia; units: m2 m−2.
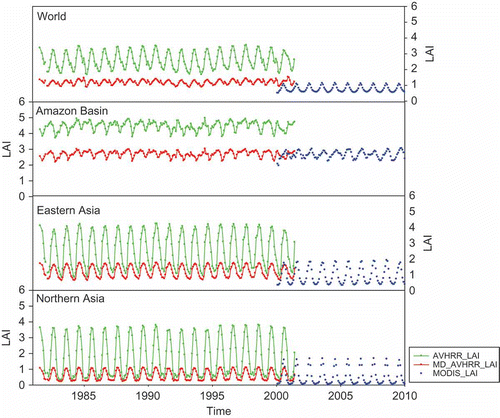
Figure 5. Regional differences in average and standard deviation between original AVHRR-LAI and MODIS-LAI and between modified AVHRR-LAI and MODIS-LAI during the overlapping period from January 2000 to May 2001 (D_LAI is the difference between the original AVHRR-LAI and MODIS-LAI; MD_D_LAI is the difference between the modified AVHRR-LAI and MODIS-LAI).
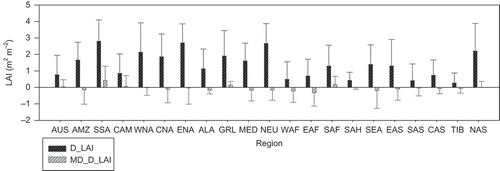
Based on the above analysis, it is necessary to adjust the AVHRR-LAI values in other periods without overlapping the MODIS-LAI. We quantitatively estimated the difference of LAI produced by the two satellite sensors. The biggest difference between the original AVHRR-LAI and MODIS-LAI is clearly seen in the overlapping period from January 2000 to May 2001. Fortunately, the difference in the data set induced by the two sensor sources is significantly reduced after gap filling by the above linear regression method. In Eastern Asia and Northern Asia, where the biggest differences between AVHRR-LAI and MODIS-LAI values were found, the values of modified AVHRR-LAI are closer to those of MODIS-LAI during the overlapping period from January 2000 to May 2001. This suggests the potential applicability of modified LAI values for investigating long-term LAI changes across the globe. However, the monthly modified AVHRR-LAI variance in the Amazon Basin is not totally consistent with that of MODIS-LAI, implying that the linear regression method might not be appropriate in this region.
3.3. Correlation of unmodified and modified LAI with variables of the climate system
Some climate variables such as temperature, precipitation, and soil moisture are key limiting factors on vegetation activity and production (Nemani et al. Citation2003; Flanagan and Johnson Citation2005). Thus, we use the respective correlation of monthly unmodified LAI and modified LAI with temperature, precipitation, and soil moisture to evaluate the modified LAI. First, to assess the robustness of the modified data set, we prepared maps of the correlation coefficients of LAI with temperature, precipitation, and soil moisture at the global scale (). We used long-term monthly unmodified and modified LAI plus temperature, precipitation, and soil moisture to build the regression relationships between vegetation and these key climate variables. The spatial correlation patterns of LAI with temperature, precipitation, and soil moisture are significantly different. There is much stronger correlation between modified LAI and rainfall in the semi-arid and arid areas such as the Sahara, Mediterranean Basin, and Central Asia. Overall, by comparing the relationship of modified LAI with rainfall and that of original LAI with rainfall, the positive correlation is obviously enhanced in areas far from the sea. Compared with the correlation between original LAI and soil moisture, modified LAI also corresponds better to soil moisture in these regions. A strongly negative correlation in wet regions such as the Amazon Basin can be seen between modified LAI and rainfall, as well as between modified LAI and soil moisture. In most Amazon Basin regions, vegetation activities are mainly driven by solar radiation (Nemani et al. Citation2003). Thus modified LAI responds more negatively to precipitation, which may indicate an increase in cloud and reduction in solar radiation. Previous studies have suggested that increased soil moisture is generally associated with increased water vapour flux and subsequent increased cloud and reduced solar radiation (Ek and Holtslag Citation2004). In cold conditions (e.g. in high northern latitudes including Siberia and Northern Canada), more positive correlation coefficients between modified LAI and temperature than those between original LAI and temperature were observed. On the other hand, more negative correlation coefficients between modified LAI and soil moisture than those between original LAI and soil moisture were captured in the highest northern latitudes (e.g. Siberia).
Figure 6. Spatial distribution of correlation coefficients (RU) between unmodified LAI and key variables of climate system including temperature, precipitation, and soil moisture, respectively ((a), (d), (g)), and coefficients (RM) between modified LAI and the same variables, respectively ((b), (e), (h)), and differences of RU minus RM ((c), (f), (i)). Unitless.
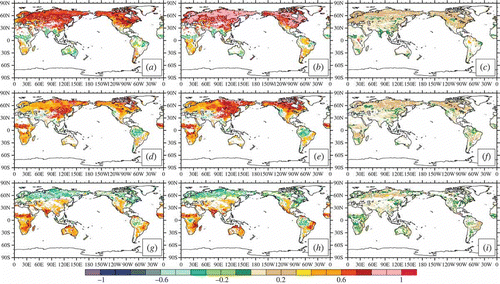
In addition, we compared the correlations resulting from monthly unmodified LAI and modified LAI with temperature, precipitation, and soil moisture at global and different regional scales (i.e. regional mean times series) (Figures ). It will be seen that there are significant differences among correlations of unmodified and modified LAI with temperature, precipitation, and soil moisture. The obvious divergence of original LAI in regard to temperature, precipitation, and soil moisture is clear at the global and regional scale, including Eastern Asia, Northern Asia, and the Amazon Basin. This could primarily be attributed to attenuation by cloud, aerosols, and atmospheric water vapour on AVHRR products. Across the globe, especially over Eastern and Northern Asia, regression of unmodified LAI with temperature, precipitation, and soil moisture produced false high slopes. Correcting original AVHRR data in order to fill the gaps between AVHRR and MODIS, a considerable convergence of the response of modified LAI to temperature, precipitation, and soil moisture was found using a regionally based linear regression method. More reasonable slopes were found by regression of modified LAI with temperature, precipitation, and soil moisture in Eastern and Northern Asia and the Amazon Basin.
Figure 7. Scatter plots of (a) temperature anomaly and original LAI anomaly derived from AVHRR and MODIS; (b) precipitation anomaly and original LAI anomaly; (c) soil moisture anomaly and original LAI anomaly; (d) temperature anomaly and modified LAI anomaly; (e) precipitation anomaly and modified LAI anomaly; and (f) soil moisture anomaly and modified LAI anomaly over the world during the period from August 1981 to December 2009. Dashed lines indicate benchmark relationships, solid lines indicate linear relationships.
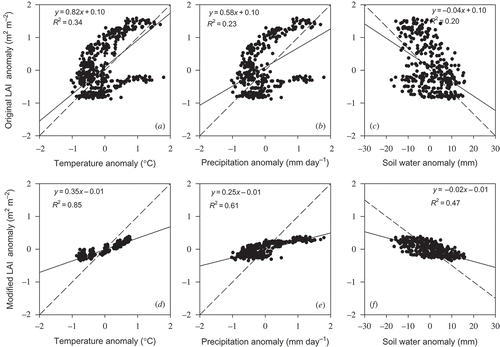
Figure 8. Scatter plots of (a) temperature anomaly and original LAI anomaly derived from AVHRR and MODIS; (b) precipitation anomaly and original LAI anomaly; (c) soil moisture anomaly and original LAI anomaly; (d) temperature anomaly and modified LAI anomaly; (e) precipitation anomaly and modified LAI anomaly; and (f) soil moisture anomaly and modified LAI anomaly in the Amazon Basin during the period from August 1981 to December 2009. Dashed lines indicate benchmark relationships; solid lines indicate linear relationships.
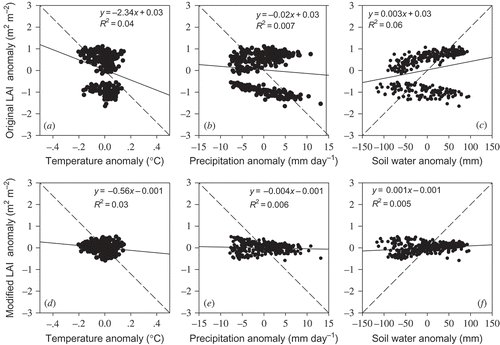
Figure 9. Scatter plots of (a) temperature anomaly and original LAI anomaly derived from AVHRR and MODIS; (b) precipitation anomaly and original LAI anomaly; (c) soil moisture anomaly and original LAI anomaly; (d) temperature anomaly and modified LAI anomaly; (e) precipitation anomaly and modified LAI anomaly; and (f) soil moisture anomaly and modified LAI anomaly in Eastern Asia during the period from August 1981 to December 2009. Dashed lines indicate benchmark relationships, solid lines indicate linear relationships.
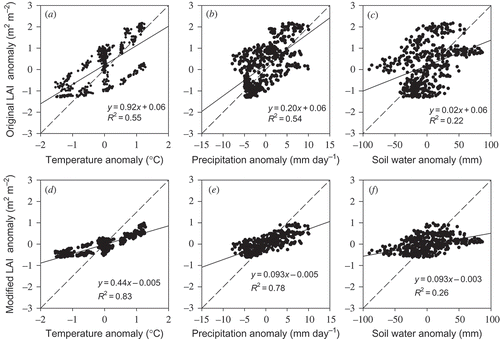
Figure 10. Scatter plots of (a) temperature anomaly and original LAI anomaly derived from AVHRR and MODIS; (b) precipitation anomaly and original LAI anomaly; (c) soil moisture anomaly and original LAI anomaly; (d) temperature anomaly and modified LAI anomaly; (e) precipitation anomaly and modified LAI anomaly; and (f) soil moisture anomaly and modified LAI anomaly in Northern Asia during the period from August 1981 to December 2009. Dashed lines indicate benchmark relationships, solid lines indicate linear relationships.
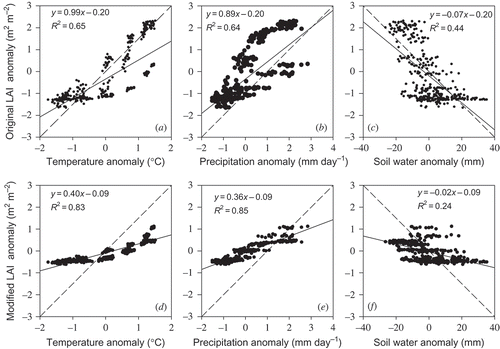
4. Discussion
4.1. Spatial discrepancy between original AVHRR- and MODIS-derived LAI
We have shown the spatial distribution pattern analysis on average and standard deviation of two different data sets – AVHRR-LAI and MODIS-LAI. This analysis was performed on overlapping months (January 2000 to May 2001) to compare AVHRR-LAI and MODIS-LAI, given that the products of MODIS are of higher quality. Based on the results shown above, the impact of potential biome misclassification on AVHRR-LAI and MODIS-LAI estimation cannot be ignored. Previous studies have investigated this impact on LAI (Fang, Li, and Myneni Citation2013), and thus LAI values rely heavily on land-cover type. This is because different land covers vary in regard to visible and near-infrared reflectance characteristics, in particular with respect to the different sensors (Gallo et al. Citation2005; Tucker et al. Citation2005). AVHRR-LAI shows clear disagreement with MODIS-LAI and is significantly higher than MODIS-LAI for boreal coniferous forest (∼262.9%), evergreen grassland (∼181.1%), and tropical forest (∼73.6%). These mismatches are marked among the land-cover types adopted by these different sensors. The difference in overall reflectance is attributed to variation in surface cover (Wang et al. Citation2005). Hame et al. (Citation1997) stated that the difference in vegetation structure is attributed to variation in LAI. In general, AVHRR-LAI is generally described by higher values over the overlapping period as compared with MODIS-LAI. The values of AVHRR-LAI are also higher than those reported by Fang, Li, and Myneni (Citation2013).
4.2. Correlation of modified and unmodified LAI with key variables of the climate system
Comparison between global and regional long-term series from modified and unmodified LAI demonstrates that the region-based linear regression method can be used to produce/reconstruct an appropriate long-term LAI data set, as described above. Since significant gaps between overlapped original AVHRR and MODIS-LAI exist (), particular attention must be paid to correct the deficiency in original LAI arising from the effects of contamination of cloud, aerosol and atmospheric water vapour, persistence of snow cover, and low saturation threshold on the AVHRR sensor, as mentioned above. Gap filling is needed to extend the robust LAI data set derived from AVHRR and MODIS by the region-based linear regression method. This method can better describe the real spatial pattern of the response of vegetation to the most important variables of the climate system including temperature, precipitation, and soil moisture. Our results on vegetation correspondence to limiting factors such as temperature and precipitation agree with the results reported by Nemani et al. (Citation2003). In high-latitude climatic zones where the limiting factor is temperature (Zhou et al. Citation2001; Lucht et al. Citation2002), stronger correspondence of modified LAI to temperature is identified. Extreme sensitivity of vegetation to rainfall and soil moisture in semi-arid and arid regions such as Central Asia is exhibited because of water scarcity limiting vegetation growth (Borgogno et al. Citation2007; D’Odorico et al. Citation2007). A much closer linkage of LAI with precipitation after calibrating AVHRR-derived LAI suggests that vegetation growth and productivity in the Amazon Basin depend mainly on solar radiation (Zhao and Running Citation2010). Previous studies have suggested that increased soil moisture is not generally associated with better vegetation condition in the highest northern latitudes because of the persistence of frozen soil, which is in accordance with our results (Niu and Yang Citation2006). Together, these results suggest the correspondence of modified LAI to temperature, precipitation, and soil moisture is much more reasonable. Thus, the linear regression method described in this study is suitable to estimate changes in global and regional vegetation used as a climate model input based on long-term LAI derived from AVHRR and MODIS.
4.3. Improvement in extended LAI by region-based linear regression method
LAI is a required variable for studies on terrestrial carbon flux in Earth system models (ESMs) and climate models (e.g. Chen et al. Citation2006; Yuan et al. Citation2011; Anav et al. Citation2013), and continuous, long-term LAI data are urgently required in ESMs. Although MODIS-LAI, with the launch of Terra in 2000, has been used as a standard product, its length of operation is insufficient for application in ESMs and climate models. Thereby, AVHRR-LAI was applied to extend the LAI time series. Both AVHRR and MODIS NDVI/LAI have been reported in previous studies using different algorithms (Holben Citation1986; Tucker et al. Citation2005; Yuan et al. Citation2011). However, the means to integrate the two data sets from these different sensors to obtain consistent and continuous long-term series has rarely been reported. This limits their application as input to ESMs and climate modelling, which has not been included in current climate models.
However, our results suggest that increased convergence and continuity of long-term modified LAI data are attained by the region-based linear regression method. It is assumed that there is geographical homogeneity in any region. Increased convergence and continuity of modified LAI data are offered by the region-based linear regression method. The pattern and magnitude of the response of modified LAI changes to temperature, precipitation, and soil moisture are more suitable than those of unmodified LAI, and this was confirmed by comparing the correlation of unmodified LAI with temperature, precipitation, and soil moisture and that of modified LAI for these key variables. Furthermore, we also compared results of the pixel-based method with those derived by the method proposed in this analysis, although data from the former method are not included here. However, there are no significant differences in the results from the two methods at the 0.05 significance level by Student’s t-test. Consequently, variation in LAI values induced by different linear regression methods does not affect our key results. Due to sufficient sample numbers, this method of regionally based regression is sufficiently reliable to extend robust long-term LAI data sets at the global scale. However, note that this method is not the only way to derive long-term global LAI data sets from satellite sensors. Further work will be done to estimate and compare methods for deriving long-term LAI data sets.
5. Conclusions
This analysis shows that modified LAI data are suitable for extended time series for the long-term study and to be used as inputs for climate and ecological models after calibrating the original AVHRR-LAI by the region-based linear regression method. The comparison of overlapping unmodified AVHRR- and MODIS-derived LAIs during the period from January 2000 to May 2001 reveals that the most significant difference between the two data sets is in high northern latitudes covered mainly by coniferous forest. This finding suggests that the significant differences observed between the two different sensors may be mainly a consequence of the difference in characteristics of sensors and vegetation structure. On the basis of our results, it seems reasonable to assume that using a region-based linear regression method to extend observed satellite LAI data sets based on an estimate of the relationships between LAI and key variables including temperature, precipitation, and soil moisture is a good way to combine the two LAI products. The results presented here not only provide opportunities to study the spatiotemporal pattern but also to provide necessary inputs to climate models with long-term integrated LAI derived from AVHRR and MODIS. As mentioned above, extended LAI time series over the long term cannot be provided by region-based linear regression alone. The selection of other methods using simulated LAI from coupled models (e.g. the new version by Dan, Ji, and Li (Citation2005)), combined with observed site measurements and evaluation of their practical application in data correction, is the subject of future work.
Acknowledgements
The authors thank Dr Peter Urich, Dr Yinpeng Li, and Dr Chonghua Yin for the language revision of this article. This study is supported by the open fund of State Key Laboratory of Remote Sensing Science (grant no. OFSLRSS201113), the Chinese Academy of Sciences (CAS) Strategic Priority Research Programme (grant no. XDA05110103), the Knowledge Innovation Programme of the Chinese Academy of Sciences (KZCX2-EW-QN208 and 7–122158), the National Basic Research Programme of China (grant no. 2010CB950500), and the project of National Natural Science Foundation of China (grant no. 41275082).
References
- Anav, A., P. Friedlingstein, M. Kidston, L. Bopp, P. Ciais, P. Cox, C. Jones, M. Jung, R. Myneni, and Z. Zhu. 2013. “Evaluating the Land and Ocean Components of the Global Carbon Cycle in the CMIP5 Earth System Models. ” Journal of Climate. doi:10.1175/JCLI-D-12-00417.1
- Andr, G., L. Denis, and B. D. Nathalie. 2000. “A Generic Model of Forest Canopy Conductance Dependent on Climate, Soil Water Availability and Leaf Area Index. ” Annals of Forest Science 57: 755–765.
- Bonan, G. B. 1993. “Importance of Leaf Area Index and Forest Type When Estimating Photosynthesis in Boreal Forests. ” Remote Sensing of Environment 43: 303–314.
- Borgogno, F., P. D’odorico, F. Laio, and L. Ridolfi. 2007. “Effect of Rainfall Interannual Variability on the Stability and Resilience of Dryland Plant Ecosystems. ” Water Resources Research 43: W06411.
- Box, E. O., B. N. Holben, and V. Kalb. 1989. “Accuracy of the AVHRR Vegetation Index as a Predictor of Biomass, Primary Productivity and Net CO2 Flux. ” Plant Ecology 80: 71–89.
- Bréda, N. J. J. 2003. “Ground Based Measurements of Leaf Area Index: A Review of Methods, Instruments and Current Controversies. ” Journal of Experimental Botany 54: 2403–2417.
- Buermann, W., J. Dong, X. Zeng, R. B. Myneni, and R. E. Dickinson. 2001. “Evaluation of the Utility of Satellite-Based Vegetation Leaf Area Index Data for Climate Simulations. ” Journal of Climate 14: 3536–3550.
- Carlson, T. N., and D. A. Ripley. 1997. “On the Relation Between NDVI, Fractional Vegetation Cover, and Leaf Area Index. ” Remote Sensing of Environment 62: 241–252.
- Chase, T. N., R. A. Pielke, T. G. F. Kittel, R. Nemani, and S. W. Running. 1996. “Sensitivity of a General Circulation Model to Global Changes in Leaf Area Index. ” Journal of Geophysical Research 101 (D3): 7393–7408.
- Chen, J. M., A. Govind, O. Sonnentag, Y. Zhang, A. Barr, and B. Amiro. 2006. “Leaf Area Index Measurements at Fluxnet-Canada Forest Sites. ” Agricultural and Forest Meteorology 140: 257–268.
- Chen, M., G. Pavlic, L. Brown, J. Cihlar, G. Leblanc, P. White, J. Hall, R. Peddle, J. King, A. Trofymow, E. Swift, J. Van Der Sanden, and E. Pellikka. 2002. “Derivation and Validation of Canada-Wide Coarse-Resolution Leaf Area Index Maps Using High-Resolution Satellite Imagery and Ground Measurements. ” Remote Sensing of Environment 80: 165–184.
- Cohen, W. B., T. K. Maiersperger, Z. Yang, S. T. Gower, D. P. Turner, W. D. Ritts, M. Berterretche, and S. W. Running. 2003. “Comparisons of Land Cover and LAI Estimates Derived from ETM+ and MODIS for Four Sites in North America: A Quality Assessment of 2000/2001 Provisional MODIS Products. ” Remote Sensing of Environment 88: 233–255.
- Colombo, R., D. Bellingeri, D. Fasolini, and C. M. Marino. 2003. “Retrieval of Leaf Area Index in Different Vegetation Types Using High Resolution Satellite Data. ” Remote Sensing of Environment 86: 120–131.
- Cramer, W., D. W. Kicklighter, A. Bondeau, B. Moore, III, G. Churkina, B. Nemry, A. Ruimy, A. L. Schloss, and T. P. O. T. Intercomparison. 1999. “Comparing Global Models of Terrestrial Net Primary Productivity (NPP): Overview and Key Results. ” Global Change Biology 5 (S1): 1–15.
- Dan, L., J. Ji, and Y. He. 2007. “Use of ISLSCP II Data to Intercompare and Validate the Terrestrial Net Primary Production in a Land Surface Model Coupled to a General Circulation Model. ” Journal of Geophysical Research 112 (D2): D02S90.
- Dan, L., J. Ji, and Y. Li. 2005. “Climatic and Biological Simulations in a Two-Way Coupled Atmosphere-Biosphere Model (CABM). ” Global and Planetary Change 47: 153–169.
- D’odorico, P., K. Caylor, G. S. Okin, and T. M. Scanlon. 2007. “On Soil Moisture-Vegetation Feedbacks and Their Possible Effects on the Dynamics of Dryland Ecosystems. ” Journal of Geophysical Research 112 (G4): G04010.
- Doraiswamy, P. C., J. L. Hatfield, T. J. Jackson, B. Akhmedov, J. Prueger, and A. Stern. 2004. “Crop Condition and Yield Simulations Using Landsat and MODIS. ” Remote Sensing of Environment 92: 548–559.
- Dye, D. G., and C. J. Tucker. 2003. “Seasonality and Trends of Snow-Cover, Vegetation Index, and Temperature in Northern Eurasia. ” Geophysics Research Letters 30: 1405.
- Ek, M. B., and A. A. M. Holtslag. 2004. “Influence of Soil Moisture on Boundary Layer Cloud Development. ” Journal of Hydrometeorology 5: 86–99.
- Fang, H., W. Li, and R. Myneni. 2013. “The Impact of Potential Land Cover Misclassification on MODIS Leaf Area Index (LAI) Estimation: A Statistical Perspective. ” Remote Sensing 5: 830–844.
- Fensholt, R., T. T. Nielsen, and S. Stisen. 2006. “Evaluation of AVHRR PAL and GIMMS 10-Day Composite NDVI Time Series Products Using SPOT-4 Vegetation Data for the African Continent. ” International Journal of Remote Sensing 27: 2719–2733.
- Fensholt, R., K. Rasmussen, T. T. Nielsen, and C. Mbow. 2009. “Evaluation of Earth Observation Based Long Term Vegetation Trends? Intercomparing NDVI Time Series Trend Analysis Consistency of Sahel from AVHRR GIMMS, Terra MODIS and SPOT VGT Data. ” Remote Sensing of Environment 113: 1886–1898.
- Flanagan, L. B., and B. G. Johnson. 2005. “Interacting Effects of Temperature, Soil Moisture and Plant Biomass Production on Ecosystem Respiration in a Northern Temperate Grassland. ” Agricultural and Forest Meteorology 130 (3-C4): 237–253.
- Gallo, K., L. Ji, B. Reed, J. Eidenshink, and J. Dwyer. 2005. “Multi-Platform Comparisons of MODIS and AVHRR Normalized Difference Vegetation Index Data. ” Remote Sensing of Environment 99: 221–231.
- Garrigues, S., R. Lacaze, F. Baret, J. T. Morisette, M. Weiss, J. E. Nickeson, R. Fernandes, S. Plummer, N. V. Shabanov, R. B. Myneni, Y. Knyazikhin, and W. Yang. 2008. “Validation and Intercomparison of Global Leaf Area Index Products Derived from Remote Sensing Data. ” Journal of Geophysical Research 113 (G2): G02028.
- Gower, S. T., C. J. Kucharik, and J. M. Norman. 1999. “Direct and Indirect Estimation of Leaf Area Index, fAPAR, and Net Primary Production of Terrestrial Ecosystems. ” Remote Sensing of Environment 70: 29–51.
- Hame, T., A. Salli, K. Andersson, and A. Lohi. 1997. “A New Methodology for the Estimation of Biomass of Conifer-Dominated Boreal Forest Using NOAA AVHRR Data. ” International Journal of Remote Sensing 18: 3211–3243.
- Hickler, T., L. Eklundh, J. W. Seaquist, B. Smith, J. Ard, L. Olsson, M. T. Sykes, and M. Sjöström. 2005. “Precipitation Controls Sahel Greening Trend. ” Geophysics Research Letters 32: L21415.
- Holben, B. N. 1986. “Characteristics of Maximum-Value Composite Images from Temporal AVHRR Data. ” International Journal of Remote Sensing 7: 1417–1434.
- Huete, A. R., H. Q. Liu, K. Batchily, and W. van Leeuwen. 1997. “A Comparison of Vegetation Indices over a Global Set of TM Images for EOS-MODIS. ” Remote Sensing of Environment 59: 440–451.
- IPCC. 2007. Climate Change 2007: Synthesis report, Intergovernmental Panel on Climate Change Fourth Assessment Report. Accessed January 3, 2010. http://www.ipcc.ch/pdf/assessment-report/ar4/syr/ar4_syr.pdf
- Jensen, J. L. R., K. S. Humes, A. T. Hudak, L. A. Vierling, and E. Delmelle. 2011. “Evaluation of the MODIS LAI Product Using Independent Lidar-Derived LAI: A Case Study in Mixed Conifer Forest. ” Remote Sensing of Environment 115: 3625–3639.
- Justice, C. O., E. Vermote, J. R. G. Townshend, R. Defries, D. P. Roy, D. K. Hall, V. V. Salomonson, J. L. Privette, G. Riggs, A. Strahler, W. Lucht, R. B. Myneni, Y. Knyazikhin, S. W. Running, R. R. Nemani, W. Zhengming, A. R. Huete, W. van Leeuwen, R. E. Wolfe, L. Giglio, J. Muller, P. Lewis, and M. J. Barnsley. 1998. “The Moderate Resolution Imaging Spectroradiometer (MODIS): Land Remote Sensing for Global Change Research. ” Geoscience and Remote Sensing, IEEE Transactions on 36: 1228–1249.
- Kang, S., S. W. Running, J. Lim, M. Zhao, C. Park, and R. Loehman. 2003. “A Regional Phenology Model for Detecting Onset of Greenness in Temperate Mixed Forests, Korea: An Application of MODIS Leaf Area Index. ” Remote Sensing of Environment 86: 232–242.
- Kergoat, L., S. B. Lafont, H. A. B. B. Douville, G. R. Dedieu, S. Planton, and J. O. Royer. 2002. “Impact of Doubled CO2 on Global-Scale Leaf Area Index and Evapotranspiration: Conflicting Stomatal Conductance and LAI Responses. ” Journal of Geophysical Research 107 (D24): 4808.
- Knyazikhin, Y., J. V. Martonchik, R. B. Myneni, D. J. Diner, and S. W. Running. 1998. “Synergistic Algorithm for Estimating Vegetation Canopy Leaf Area Index and Fraction of Absorbed Photosynthetically Active Radiation from MODIS and MISR Data. ” Journal of Geophysical Research 103 (D24): 32257–32275.
- Lindroth, A., A. Grelle, and N. A. Mor. 1998. “Long-Term Measurements of Boreal Forest Carbon Balance Reveal Large Temperature Sensitivity. ” Global Change Biology 4 (4): 443–450.
- Liu, J., J. M. Chen, J. Cihlar, and W. Chen. 1999. “Net Primary Productivity Distribution in the BOREAS Region from a Process Model Using Satellite and Surface Data. ” Journal of Geophysical Research 104 (D22): 27735–27754.
- Liu, Y., R. Liu, and J. M. Chen. 2012. “Retrospective Retrieval of Long-Term Consistent Global Leaf Area Index (1981–2011) from Combined AVHRR and MODIS Data. ” Journal of Geophysical Research 117 (G4): G04003.
- Lucht, W., I. C. Prentice, R. B. Myneni, S. Sitch, P. Friedlingstein, W. Cramer, P. Bousquet, W. Buermann, and B. Smith. 2002. “Climatic Control of the High-Latitude Vegetation Greening Trend and Pinatubo Effect. ” Science 296: 1687–1689.
- Mitchell, T. D., and P. D. Jones. 2005. “An Improved Method of Constructing a Database of Monthly Climate Observations and Associated High-Resolution Grids. ” International Journal of Climatology 25: 693–712.
- Myneni, R. B., S. Hoffman, Y. Knyazikhin, J. L. Privette, J. Glassy, Y. Tian, Y. Wang, X. Song, Y. Zhang, G. R. Smith, A. Lotsch, M. Friedl, J. T. Morisette, P. Votava, R. R. Nemani, and S. W. Running. 2002. “Global Products of Vegetation Leaf Area and Fraction Absorbed PAR from Year One of MODIS Data. ” Remote Sensing of Environment 83: 214–231.
- Myneni, R., Y. Knyazikhin, and N. Shabanov. 2011. “Leaf Area Index and Fraction of Absorbed PAR Products from Terra and Aqua MODIS. ” Sensors: Analysis, Validation, and Refinement 11: 603–633.
- Myneni, R. B., R. Ramakrishna, R. Nemani, and S. W. Running. 1997. “Estimation of Global Leaf Area Index and Absorbed Par Using Radiative Transfer Models.” IEEE Transactions on Geoscience and Remote Sensing 35: 1380–1393.
- Nagol, J. R., E. F. Vermote, and S. D. Prince. 2009. “Effects of Atmospheric Variation on AVHRR NDVI Data. ” Remote Sensing of Environment 113: 392–397.
- Nemani, R. R., C. D. Keeling, H. Hashimoto, W. M. Jolly, S. C. Piper, C. J. Tucker, R. B. Myneni, and S. W. Running. 2003. “Climate-Driven Increases in Global Terrestrial Net Primary Production from 1982 to 1999. ” Science 300: 1560–1563.
- Nemani, R. R., and S. W. Running. 1989. “Testing a Theoretical Climate-Soil-Leaf Area Hydrologic Equilibrium of Forests Using Satellite Data and Ecosystem Simulation. ” Agricultural and Forest Meteorology 44: 245–260.
- Niu, G., and Z. Yang. 2006. “Effects of Frozen Soil on Snowmelt Runoff and Soil Water Storage at a Continental Scale. ” Journal of Hydrometeorology 7: 937–952.
- Peng, J., W. Dong, W. Yuan, J. Chou, Y. Zhang, and J. Li. 2012. “Effects of Increased CO2 on Land Water Balance from 1850 to 1989. ” Theoretical and Applied Climatology 111: 483–495.
- Peng, J., W. Dong, W. Yuan, and Y. Zhang. 2012. “Responses of Grassland and Forest to Temperature and Precipitation Changes in Northeast China. ” Advances in Atmospheric Sciences 29: 1063–1077.
- Running, S. W., and R. R. Nemani. 1991. “Regional Hydrologic and Carbon Balance Responses of Forests Resulting from Potential Climate Change. ” Climatic Change 19: 349–368.
- Soudani, K., C. Fran Ois, G. le Maire, V. R. Le Dantec, and E. Dufr Ne. 2006. “Comparative Analysis of IKONOS, SPOT, and ETM+ Data for Leaf Area Index Estimation in Temperate Coniferous and Deciduous Forest Stands. ” Remote Sensing of Environment 102 (1-C2): 161–175.
- Spanner, M. A., L. L. Pierce, S. W. Running, and D. L. Peterson. 1990. “The Seasonality of AVHRR Data of Temperate Coniferous Forests: Relationship with Leaf Area Index. ” Remote Sensing of Environment 33: 97–112.
- Tucker, C. J., J. E. Pinzon, M. E. Brown, D. A. Slayback, E. W. Pak, R. Mahoney, E. F. Vermote, and N. El Saleous. 2005. “An Extended AVHRR 8-km NDVI Dataset Compatible with MODIS and SPOT Vegetation NDVI Data. ” International Journal of Remote Sensing 26: 4485–4498.
- Turner, D. P., W. B. Cohen, R. E. Kennedy, K. S. Fassnacht, and J. M. Briggs. 1999. “Relationships Between Leaf Area Index and Landsat TM Spectral Vegetation Indices Across Three Temperate Zone Sites. ” Remote Sensing of Environment 70: 52–68.
- van den Dool, H., J. Huang, and Y. Fan. 2003. “Performance and Analysis of the Constructed Analogue Method Applied to U.S. Soil Moisture over 1981–2001. ” Journal of Geophysical Research 108 (D16): 8617.
- van Leeuwen, W. J. D., A. R. Huete, and T. W. Laing. 1999. “MODIS Vegetation Index Compositing Approach: A Prototype with AVHRR Data. ” Remote Sensing of Environment 69: 264–280.
- Vermote, E. F., N. Z. El Saleous, and C. O. Justice. 2002. “Atmospheric Correction of MODIS Data in the Visible to Middle Infrared: First Results. ” Remote Sensing of Environment 83: 97–111.
- Wang, Q., S. Adiku, J. Tenhunen, and A. Granier. 2005. “On the Relationship of NDVI with Leaf Area Index in a Deciduous Forest Site. ” Remote Sensing of Environment 94: 244–255.
- Wang, Y., C. E. Woodcock, W. Buermann, P. Stenberg, P. Voipio, H. Smolander, T. Hme, Y. Tian, J. Hu, Y. Knyazikhin, and R. B. Myneni. 2004. “Evaluation of the MODIS LAI Algorithm at a Coniferous Forest Site in Finland. ” Remote Sensing of Environment 91: 114–127.
- Wang, Z., C. Liu, and A. Huete. 2003. “From AVHRR-NDVI to MODIS-EVI: Advances in Vegetation Index Research. ” Acta Ecologica Sinica 23: 979–987.
- Wolfe, R. E., M. Nishihama, A. J. Fleig, J. A. Kuyper, D. P. Roy, J. C. Storey, and F. S. Patt. 2002. “Achieving Sub-Pixel Geolocation Accuracy in Support of MODIS Land Science. ” Remote Sensing of Environment 83: 31–49.
- Xiao, Z., S. Liang, J. Wang, B. Jiang, and X. Li. 2011. “Real-Time Retrieval of Leaf Area Index from MODIS Time Series Data. ” Remote Sensing of Environment 115: 97–106.
- Yuan, H., Y. Dai, Z. Xiao, D. Ji, and W. Shangguan. 2011. “Reprocessing the MODIS Leaf Area Index Products for Land Surface and Climate Modelling. ” Remote Sensing of Environment 115: 1171–1187.
- Zhao, M., and S. W. Running. 2010. “Drought-Induced Reduction in Global Terrestrial Net Primary Production from 2000 Through 2009. ” Science 329: 940–943.
- Zheng, G., and L. M. Moskal. 2009. “Retrieving Leaf Area Index (LAI) Using Remote Sensing: Theories, Methods and Sensors. ” Sensors 9: 2719–2745.
- Zhou, L., R. K. Kaufmann, Y. Tian, R. B. Myneni, and C. J. Tucker. 2003. “Relation Between Interannual Variations in Satellite Measures of Northern Forest Greenness and Climate Between 1982 and 1999. ” Journal of Geophysical Research 108 (D1): 4004.
- Zhou, L., C. J. Tucker, R. K. Kaufmann, D. Slayback, N. V. Shabanov, and R. B. Myneni. 2001. “Variations in Northern Vegetation Activity Inferred from Satellite Data of Vegetation Index During 1981 to 1999. ” Journal of Geophysical Research 106 (D17): 20069–20083.