Abstract
Validating land-cover maps at the global scale is a significant challenge. We built a global validation data-set based on interpreting Landsat Thematic Mapper (TM) and Enhanced TM Plus (ETM+) images for a total of 38,664 sample units pre-determined with an equal-area stratified sampling scheme. This was supplemented by MODIS enhanced vegetation index (EVI) time series data and other high-resolution imagery on Google Earth. Initially designed for validating 30 m-resolution global land-cover maps in the Finer Resolution Observation and Monitoring of Global Land Cover (FROM-GLC) project, the data-set has been carefully improved through several rounds of interpretation and verification by different image interpreters, and checked by one quality controller. Independent test interpretation indicated that the quality control correctness level reached 90% at level 1 classes using selected interpretation keys from various parts of the USA. Fifty-nine per cent of the samples have been verified with high-resolution images on Google Earth. Uncertainty in interpretation was measured by the interpreter’s perceived confidence. Only less than 7% of the sample was perceived as low confidence at level 1 by interpreters. Nearly 42% of the sample units located within a homogeneous area could be applied to validating global land-cover maps whose resolution is 500 m or finer. Forty-six per cent of the sample whose EVI values are high or with little seasonal variation throughout the year can be applied to validate land-cover products produced from data acquired in different phenological stages, while approximately 76% of the remaining sample whose EVI values have obvious seasonal variation was interpreted from images acquired within the growing season. While the improvement is under way, some of the homogeneous sample units in the data-set have already been used in assessing other classification results or as training data for land-cover mapping with coarser-resolution data.
1. Introduction
Land cover is the physical evidence on the Earth’s surface, and land-cover maps play a significant role in Earth system studies and ecosystem management. They can be used in parameterizing land process models in both climate and hydrological and carbon cycle models (e.g. Dickinson et al. Citation1989; Liu et al. Citation1997; Dai et al. Citation2003; van Dijk, Peña-Arancibia, and Bruijnzeel Citation2012; Yang et al. Citation2013), in public health and ecosystem assessment (e.g. Liang et al. Citation2010; Gong et al. Citation2012; Torresan et al. Citation2012), or in the management of natural resources or agricultural activities (e.g. Fritz et al. Citation2011; Zhong, Gong, and Biging Citation2012).
During the past 15 years, several global land-cover maps have been developed with resolutions varying from 1 km to 30 m (e.g. IGBP-DISCover product (Loveland et al. Citation2000), UMD land-cover product (Hansen et al. Citation2000), GLC2000 product (Bartholomé and Belward Citation2005), MODIS land-cover product (Friedl et al. Citation2002, Citation2010), GLOBcover 2005 and 2009 (Arino et al. Citation2008; Defourny et al. Citation2009), and FROM-GLC maps (Gong et al. Citation2013)), and are available to the users of land-cover information for different applications. Validation is a key in the whole process of land-cover mapping, since without proper validation against higher-quality reference data, any land-cover map remains an untested hypothesis that cannot be unquestionably used (Congalton and Green Citation1999; Strahler et al. Citation2006). For global or continental-scale land-cover maps, reference samples are difficult to obtain from field observations and are mostly based on image interpretation (Scepan, Menz, and Hansen Citation1999; Mayaux et al. Citation2006; Friedl et al. Citation2002; Arino et al. Citation2008; Wickham et al. Citation2013), a highly labour-intensive and time-consuming process. Therefore, most global land-cover maps are either cross-validated from training samples or estimated with a limited number of samples, in the order of a few hundred to thousands (Loveland et al. Citation2000; Hansen et al. Citation2000; Bartholomé and Belward Citation2005; Friedl et al. Citation2002, Citation2010; Tateishi et al. Citation2011; Arino et al. Citation2008; Defourny et al. Citation2009). Despite fairly good accuracies reported through self-evaluation (overall accuracy 66–78%), some researchers found considerably lower accuracies, ranging from 10% to 50%, in different parts of the world or for different land-cover classes when validating the existing global land-cover maps with different reference samples (Sedano, Gong, and Ferrao Citation2005; Frey and Smith Citation2007; Gong Citation2009). Clearly more evaluations and comparisons of land-cover products are necessary, and this requires an independent set of well-distributed validation samples. Therefore, using FROM-GLC (Gong et al. Citation2013), it was determined that while high-quality validation samples should be collected for validating 30 m global land-cover products, some efforts should be made to make the samples applicable to the validation of other types of land-cover data products.
Land cover changes according to different phenological stages of vegetation or seasonal fluctuations of water supply due to natural climatic cycles or human impacts, which increases the difficulty of validation. To date, land-cover types in early generations of global land-cover maps are defined according to the principle of ‘the greenest’ (e.g. grasslands are usually defined as lands covered with perennial or annual herbaceous species at least during the growing season) or ‘the wettest’ (e.g. wetlands are lands saturated with water permanently or seasonally), meaning that they are generalized across time but not real-time dynamics. Grasses may die back in dry or cold seasons while wetlands may occur in grasslands or crop fields recurrently, and this dynamic information of the land cover is also useful, which can be provided by a time series of land-cover maps. Therefore, the importance of mapping ‘dynamic land-cover types’ for a variety of applications is increasingly being recognized. Sun et al. (Citation2014) developed a high-frequency (every 8 days) specific land-cover (water bodies) map for hydrological or environmental applications, which requires a validation data-set with phenological information. Taken the applicability of the samples into consideration, a validation data-set with phenological information is necessary for validating land-cover maps made from data acquired from different seasons.
In addition, large discrepancies were found when comparing different global land-cover products due to the fact that (1) accuracies of the same land-cover map are different when assessed with different validation data-sets; (2) accuracies of land-cover maps for the same area are different; and (3) large disagreement between land-cover maps was found when conducting map-to-map comparison (Giri, Zhu, and Reed Citation2005; See and Fritz Citation2006; McCallum et al. Citation2006; Tchuenté, Roujean, and Jong Citation2011; Pflugmacher et al. Citation2011). Users may be confused about which map to choose for their application with regard to accuracy, beyond considerations of classification scheme and spatial resolution. The first difficulty in choosing a land-cover map is that each map was validated independently, and direct accuracy comparison among maps is hindered by the lack of a common validation data-set. The second is that overall accuracy cannot reflect the quality of a map for a particular application, since map errors are unevenly distributed in different areas or for different land-cover classes. Better accuracy assessment and comparison would be greatly facilitated by a common global validation data-set, especially an adequate, well-described, compatible, and temporally updated one. Communities have begun contributing to global validation data-sets; GOFC-GOLD has been constructing a data-access portal (http://www.gofcgold.wur.nl/sites/gofcgold_refdataportal.php) for various validation data-sets. Designed for different applications, validation data-sets for specific land-cover types were also developed (e.g. Miyazaki, Iwao, and Shibasaki Citation2011). Several studies conducted validation sampling design. For example, a stratification based on Köppen–Geiger climate classification and population density, and an augmentation method, were developed to make the validation samples independent of any single land-cover map and meet the objectives of regional accuracy assessment (Olofsson et al. Citation2012; Stehman et al. Citation2012). The United Nations Food and Agriculture Organization (FAO) used systematic sampling at the intersections of latitude and longitude in their forest inventory (Ridder Citation2007). However, we believe that equal-area stratified random sampling of the land areas of the world is desirable.
The objective of this article is to present the newly developed global land-cover validation data-set whose sample units are allocated using an equal-area stratified sampling scheme, evaluate the inherent land-cover interpretation uncertainty of the sample units, and analyse the attributes of the sample units to expand their applicability in other land-cover studies involving data acquired from different phenological stages or at different spatial resolution. For illustration purposes, the data-set was applied to compare the accuracies of three global land-cover maps.
2. Constructing a global validation data-set
The main processes in constructing the global validation data-set include (1) data collection and classification scheme design; (2) sampling design; (3) interpretation and quality control; (4) phenology characterization; and (5) homogeneity measurement ().
2.1. Data collection and classification scheme design
A total of 8929 scenes of Landsat TM/ETM images from Gong et al. (Citation2013) were used in this research. They were collected from the Global Land Cover Facility (GLCF) at the University of Maryland (UMD), United States Geological Survey (USGS), and the Satellite Ground Station of China, as the source data for validation sample interpretation. The baseline period is 2009–2011, since about 60% of the source images were acquired after 2009, while 95.8% were acquired after 2000. Source images acquired in multiple years were combined in this study based on the fact that the overall land-cover change through a decade is less than 8% over rapidly changing areas of the world at the regional scale (Lambin, Geist, and Lepers Citation2003; Stehman, Sohl, and Loveland Citation2003; Drummond and Auch Citation2012; Pérez-Hoyos, García-Haro, and San-Miguel-Ayanz Citation2012), which implies that even with a time discrepancy of 10 years (assuming 40% of images falling outside of the baseline year of 2009–2011), the validation sample units before 2009 may contain an error of approximately 0.32–3.2%, even for rapidly changing areas. Since most of the existing land-cover maps have an error of over 20%, that caused by image combination over multiple years would contribute less than 3% error in classification assessment at the global scale. To reduce the uncertainty caused by this, we planned to constantly update and backtrack the samples in the data-set to provide land-cover time series with the enlargement of image resources.
Additionally, the time series of a 16-day composite of MODIS enhanced vegetation index (EVI) with 250 m spatial resolution for the year 2010 were downloaded to provide information about seasonal variation (MOD13Q1 product, http://modis.gsfc.nasa.gov/data), with which some specific land-cover types are easier to interpret. High-resolution imagery on Google Earth was used as a supplemental reference for the interpretation of TM/ETM+ images.
The classification scheme for the validation data-set includes 11 land-cover types at level 1, and some life-form categories included at level 2 that are potentially separable from TM imagery (see ). This system was developed based on the characteristics of the finer-resolution data with further expansion in mind when fractional and vegetation height information become available (Gong et al. Citation2013). With additional information on canopy coverage and height that is planned for further production in the FROM-GLC project, this classification scheme can easily be cross-walked to other schemes such as the FAO land cover classification system and the International Geosphere-Biosphere Programme (IGBP) land-cover classification system.
Table 1. Land-cover types in the classification scheme of the validation sample set.
2.2. Sampling design
We had two objectives when we designed the sampling method. First, to ensure an overall accuracy assessment in space (i.e. the sample units are globally evenly but locally randomly distributed), an equal-area stratified random sampling scheme was selected. At the global scale, ours is the only one that is based on equal-area partitioning of the terrestrial surface. Our second objective was to use this design to estimate the proportions of various land-cover types around the world. There has been no previous systematic sampling for the entire world using such a large number of independent units. Since geographic coordinate grids are not equal in area, the strata are approximately 7000 equal-area hexagons dividing the land areas of the world (Overton et al. Citation1991; White, Kimerling, and Overton Citation1992; Sahr and White Citation1998). In each hexagon, it is necessary to avoid potential bias of repetitive patterns of land-cover distribution. Thus, a simple random sampling of five units within each hexagon was adopted. Initially, each sample unit was a 30 m pixel. For the sample size in each hexagon, we evaluated from 2 to 10 sample units and found that 5 per hexagon were affordable and practical for the whole world. This resulted in a total of 38,664 sample units. This sampling design could be implemented independently of any land-cover maps or stratification systems, and the sample results can be used to estimate the percentage of each land-cover type occupying the Earth’s land surface (land-cover percentage estimation was reported in Gong et al. Citation2013).
2.3. Interpretation and quality control
All image interpreters were selected on the basis of sufficient image interpretation experience. As shown in , after in-depth training on interpretation given by invited experts familiar with local land cover in different parts of the world, the validation sample units were primarily interpreted by four image interpreters for the first round. For each observation, the 30 m pixel at the predefined location was identified into the appropriate land-cover type at level 2 (shown in ), along with three supplementary attributes to describe (1) whether high-resolution imagery (spatial resolution usually finer than 2 m) is available in Google Earth; (2) the interpreter’s perceived confidence; and (3) whether the 30 m pixel is surrounded by homogeneous surface cover in the same class. For the second round, the interpreted sample units were double-checked by a further three image interpreters selected based on their outstanding skills in image interpretation. The final quality control – the third round – was conducted by only one interpreter, the most experienced, who reviewed all sample units and ensured that they could be interpreted under a uniform standard. Some land-cover types were partially defined by spatial characteristics such as coverage and canopy height. Coverage may be estimated differently by different interpreters. Sparse grassland, for example, might be categorized into grasslands or barren lands, depending on the estimated herbaceous coverage of the sample unit. Users, however, only need to apply the final round of interpreted samples to their application. For integrity of the data-set, we kept the earlier rounds of interpretation to indicate the difficulty of interpretation; for example, if a sample unit was interpreted correctly for the first round and all quality controllers showed consistent interpretation, that sample unit would be deemed more reliable and easy to interpret.
The interpretation process is based on both inherent elements (size, shape, tone, texture, shadow, association, and pattern) in images and prior knowledge, such as climate condition, vegetation zone, seasonality, and regional human impacts. Beside Landsat TM/ETM+ images and MODIS EVI time series, supplementary information from Google Earth plays an important role in land-cover interpretation (Yu and Gong Citation2012. Examples are shown in ).
Figure 2. Supplementary data over three sample locations for interpretation from Google Earth. Sample unit A is located in the East Siberian taiga in Russia; sample unit B is an example at Snake-Columbia shrub steppe in the USA; sample unit C is a typical Mediterranean orchard and vineyard in France. (a) Geo-tagged photos shared by visitors on the platform of Google Earth; (b), (d), and (f) latest remotely sensed images on Google Earth; (c) elevation profile for A; (e) historical imagery; (g) screenshots of street view.
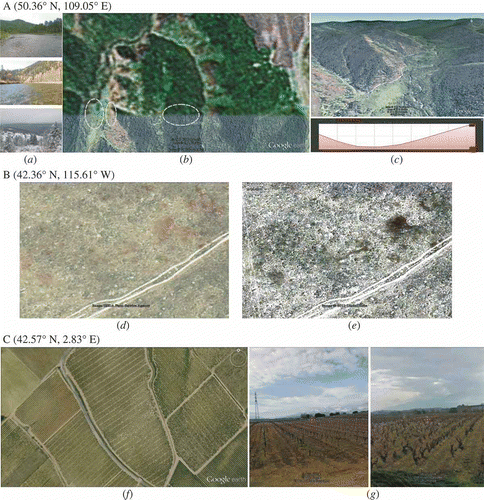
2.4. Phenology characterization
Annual phenology variation, which refers to the life cycle of vegetation or the fluctuation of water cycles, may result in land-cover change, which is the reason that validation sampling without phenological characterization cannot be unquestionably applied to validate dynamic land-cover products. Sample units whose EVI values are high or have little variation in the annual cycle are in same land-cover type throughout the year, meaning that they can be used to validate land-cover maps produced from data acquired at any time in a year. On the contrary, sample units whose EVI values have obvious seasonal changes should be used with caution, because in this case, sample units might be identified as different land-cover types inside and outside the growing season. Thus they can only be applied to validate dynamic land-cover maps in part of their annual cycle according to the acquisition time of the interpreted Landsat image. Having phenology information would add the application flexibility of the data and reduce uncertainties.
To identify the phenological type and parameters of sample units, 16-day MODIS EVI time series data for the year 2010 were extracted at the sample locations and were processed using TIMESAT software (Jonsson and Eklundh Citation2002, Citation2004), which was developed for extracting seasonality parameters from satellite data. Since the double logistic function was better at preserving the integrity of the time series (Hird and McDermid Citation2009) than Savitzky–Golay, which is more sensitive to rapid changes by local fitting, the former model was used.
As shown in , some sample units could not be processed due to lack of valid data in a time series, while others were processed with the chosen model fit. To evaluate the goodness of fit, the coefficient of determination, R2, was calculated. Noisy sample units with low R2 were filtered out and then checked manually. Seasonality parameters, such as the beginning of the season (Begin), end of the season (End), length of the season (Length), average of left and right minimum values (Base), and the difference between the maximum value and the base level in a season (Amplitude), are calculated from TIMESAT. The beginning and end of the season were estimated as rising and falling for 20% of the seasonal amplitude. presents the EVI time series for examples of different phenological characteristics identified through the processes in . Sample units with relatively high base level (Base >3000) were characterized as ‘green vegetation over the whole year’ (). For the remaining sample units, those with overly short or long growing seasons (Length <4 or >22) caused by noise were characterized as ‘EVI with little change over the whole year’ ( and ), while those in dry and cold regions according to Köppen–Geiger climate classes were excluded because the growing season is assumed to be shorter than 64 days in those regions limited by water or temperature conditions ( and ). The remaining sample units were categorized as ‘EVI with little change over the whole year’ () and ‘EVI with obvious seasonal change’ ( and ) by comparing the estimated seasonal amplitude to a threshold related to the estimated base level. The higher the base level, the higher the threshold was set. Since the acquisition time of the main reference image for interpretation may influence the interpreted land-cover type and uncertainty in interpretation, sample units with obvious seasonal changes were categorized into two classes – ‘interpreted in growing season’ ( and ) and ‘interpreted outside growing season’ ( and ), according to the relationship between the acquisition time of the Landsat image and the estimated beginning and end of the season. That is why the sample unit shown in was interpreted as barren instead of grassland, because during the long dry season the grasses had dried up.
Figure 3. Major processes in phenology characterization. This procedure is run for each sample in the validation data-set.
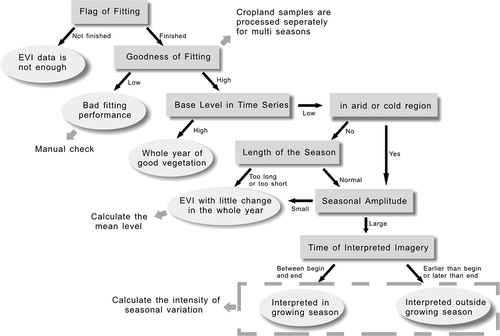
Figure 4. EVI time series of different phenological characteristics. Small arrows along the time axis refer to the approximate acquisition time of Landsat imagery used in this study. The estimated growing season is presented as a grey block in each example.
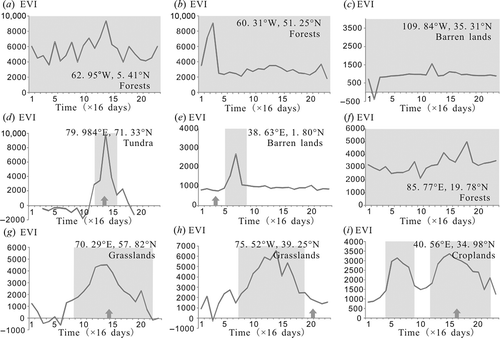
The cropland and bare cropland sample units were processed separately because some have more than one season in a year (). Thus, the program was forced to treat the time series of these sample units as if there were two annual seasons. However, given the fact that some fitted time series data do not reflect the real phenological change, being affected by noise, the sample units were filtered based on the following criteria to remove those that were not real double-cropping sample units: (1) relatively high (>0.7) when evaluating the goodness of fit; (2) the interval between two seasons is no shorter than 32 days; (3) the length of each season is longer than 48 days; (4) relatively high intensity of the seasonal change (calculated by multiplying the seasonal amplitude by base level, >0.8); (5) the two seasons should not have major disparity in seasonal amplitude (ratio between 3/5 and 5/3); and (6) the ratio of the EVI values at the beginning and the end of the season is below 3.
2.5. Homogeneity measurement
If the land cover in a 1 × 1 km pixel around a sample location is nearly homogeneous, the sample unit is suitable for validation of land-cover maps at spatial resolutions ranging from 30 to 500 m. Thus, the homogeneity measurement of the sample location is necessary for appropriate use of the sample units. With such a distinction one could use the large homogeneous sample units as part of the training or validation data when classifying coarser-resolution images (e.g. Wang et al., forthcoming).
Although homogeneity was manually recorded by interpreters in the information for the sample units, it relies on the subjective judgement of the interpreters. In most cases the homogeneous area is relatively uniform, repetitive, and less complicated in texture, so the approach of texture analysis was adopted to measure homogeneity automatically (Gong, Marceau, and Howarth Citation1992).
The grey-level co-occurrence matrix (GLCM) is a second-order statistical texture characterization method that enumerates the occurrence of pixel pairs of specific values along certain directions in a local pixel neighbourhood (Haralick, Shanmugam, and Dinstein Citation1973). Two texture features, contrast and entropy, were extracted to summarize the information contained in the GLCMs calculated for a 1 × 1 km window on TM band 4 images at the sample location. Entropy is a measure of complexity of the image, while contrast is a measure of the intensity of difference between a pixel and its neighbours on the image.
To estimate the threshold of texture features for homogeneous sample units, 1121 sample units in the data-set were selected by the stratified random sampling method and the strata were constructed based on a combination of Köppen–Geiger climate classes (Rubel and Kottek Citation2010) and land-cover types, to ensure the inclusion of all climate types and land-cover types. All selected sample units were labelled as either homogeneous or heterogeneous. Scatter plots and histograms were created separately for different land-cover types to select the thresholds, since the threshold is higher if a land-cover is coarse-grained – such as forests, or fragmented – such as crop fields.
Although a visual inspection of homogeneous samples determined by texture analysis indicates that all are indeed homogeneous, a certain amount of uncertainty could exist in this subset of samples, especially those sample units located on different land-cover types having similar spectral properties or those belonging to the same land-cover type but having different spectral characteristics.
3. Characteristics of the data-set
Out of the total design of sample size of 38,664, although every sample unit was initially assigned a class at various stages, 37,435 sample units were identified by the quality controller. The distribution of the sample units in terms of level 1 classes is shown in . This leaves 1229 sample units un-identified due to lack of quality Landsat data (193), cloud cover (288), or high interpretation uncertainty (748), accounting for 3.18% of the total sample population. From , it will be seen that the lowest number of sample units is 261 (wetlands). This number of sample units in small-sized classes is sufficient for accuracy evaluation at the global scale according to the sample size determination for an individual class based on a binomial distribution (Fitzpatrick-Lins Citation1981). As an approximation to sample size requirements according to this method, when the expected accuracy is 85% with a 5% allowable error, the required sample size is 196 (Gong Citation2006).
Table 2. Number of sample units for each land-cover class at level 1.
The data-set contains information about the interpretation uncertainty, phenology, and homogeneity of each sample unit (). By summarizing the characteristics of the sample units in the data-set, the uncertainty of the data and the applicability to different land-cover maps were analysed (). In the following we present some details.
Table 3. Attribute table in the validation data-set. Two records are shown as examples, with explanation in parentheses.
3.1. Inherent land-cover class interpretation uncertainty of the validation sample
The inherent land-cover class interpretation uncertainty of the validation sample is attributable to many factors, such as site complexity, lack or poor quality of reference data for interpretation, spectral difference within the same land-cover type, and the occurrence of mixed pixels caused by fragmented landscape. The lack or poor quality of reference image for interpretation limits the accuracy of the validation sample. Some reference images are covered by clouds and shadows, damaged from atmospheric correction, acquired at specific points in time difficult to interpret, or are too coarse to identify the land-cover type. Take the spatial resolution of the reference image as an example: 59.39% of the sample units in the data-set have high-resolution images as reference from Google Earth, providing strong support for TM image interpretation and reducing the uncertainty of the sample, especially for some specific land-cover types such as rice fields, shrublands, pastures, and reservoirs/fish ponds. For example, livestock and dam are visible markers for interpreting pastures and reservoirs, respectively. Some shrubs in arid regions are difficult recognize on low-resolution images since most arid shrubs have very small leaves and the background dominates the observed spectral signal. The discrimination of forest subtype also benefits more from high-resolution images, because leaf shape, size, and colour can be interpreted better. As shown in ), regions of high population density such as eastern and southern Asia, Europe, western Africa, and eastern South America are covered with high-resolution images, while by contrast sparsely populated regions such as those in high northern latitudes – western Australia and the Sahara – are not. The tropics, including Southeast Asia, the Congo Basin, and the Amazon, also lack high-resolution images due to frequent cloud cover. Sample units of the 10 largest countries, accounting for over 54% of the total sample amount, represent a sizeable proportion in the data-set. The numbers of sample units with and without high-resolution images of the 10 largest countries in area were compared ()). Over 80% of the sample units for India and the USA have high-resolution images, but the percentage for Russia and Canada is only 25% and 31%, respectively. Although all sample units were interpreted based on Landsat imagery, a large portion of them were covered with high-resolution ancillary data. The use of different data sources could potentially introduce bias. The level of certainty over different sample units varies with land-cover type, availability of high-resolution data, and the quality and timeliness of ancillary data. Despite the potential bias, in most cases the ancillary data help reduce the uncertainty of the interpretation results based on Landsat data, and therefore improve the overall quality of the sample data-set for validating land-cover maps. The availability of high-resolution data, as noted in the attribute table of our validation data-set, could help indicate the bias and quality of the sample units and serve as a starting point for further collection of the high-resolution image or field verification.
Figure 6. Validation samples with high-resolution imagery available at Google Earth. (a) Spatial distribution of samples with and without high-resolution imagery available at Google Earth; (b) total number of samples for the 10 largest countries and the percentage of samples with high-resolution imagery for each country.
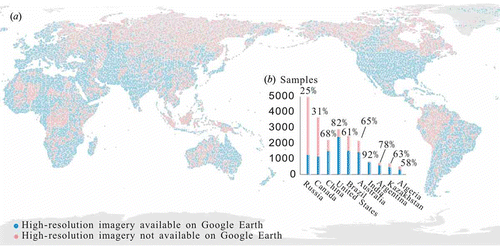
The interpretation uncertainty of the validation sample can be reflected by the interpreter’s perceived confidence. More efforts will be made to improve sample units with low confidence with the use of more reference data or field surveys in the future. In total, 2622 and 4798 sample units are labelled with low confidence in land-cover type at levels 1 and 2, respectively. Validation sample units interpreted with low confidence are distributed mostly in the biomes of (1) tundra, (2) boreal forests/taiga at high latitude, and (3) tropical and subtropical grasslands, savannas, and shrublands in Africa, South America, and Australia ()). The proportion of the samples with low confidence varies greatly among different land-cover types, as shown in ) – grassland is highest (8.84%), followed by impervious surfaces and shrublands. This proportion for forests is not high, but the quantity is large nonetheless due to the large number of forest sample units. Sample units of barren lands show the best confidence, with only 1.37% interpreted with low confidence at level 1. Although interpreters may feel confident in identifying barren land, this may not be so easily identifiable due to its spectral confusion with low-coverage vegetation types.
Figure 7. Validation samples interpreted with low confidence at land-cover type level 1. (a) Spatial distribution of the low-confidence samples; (b) number of samples in each land-cover type (solid bars) and percentage of sample interpreted with low confidence at level 1 (dashed lines).
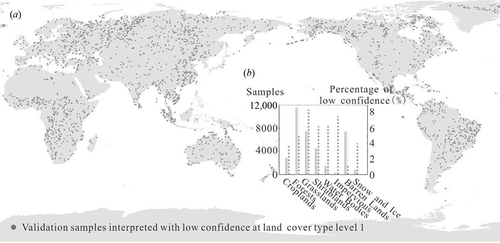
The quality controller who finalized all validation sample units had been selected from among more than 20 photo-interpreters who had participated in the production of FROM-GLC (Gong et al. Citation2013). She was the best performer in various tests of photo-interpretation. With no on-site visit experience in the USA, this quality controller scored 90% correct among the 10 land-cover categories at level 1 and 78% correct out of more than 20 categories at level 2 in a photo-interpretation test. One hundred sample areas in the test were selected from photo-interpretation keys of all ranges of difficulty level from California, Utah, South Dakota, Minnesota, and Florida, mostly based on colour infrared photographs but with some taken from high-resolution images in Google Earth (Aerial Information Systems Inc. Citation2007; Ouray National Wildlife Refuge Citation2001; Lacreek National Wildlife Refuge Citation2001; USGS-NPS Citation1996; Southwest Florida Water Management District Citation2010). The 100 test units included a similar percentage of each land-cover type (croplands 9%, forest 33%, grasslands 20%, shrublands 8%, wetlands 14%, water bodies 3%, tundra 1%, impervious surface 0%, barren lands 11%, and snow and ice 1%) in the composition of the validation data-set. With this test score, we estimate the uncertainty attributable to quality control to be less than 10% in the validation sample at level 1. In the interpretation testing of all except one photo-interpreter who participated in the validation sample, interpretation scored above 80% at level 1 classes. The photo-interpreter who scored the lowest, achieving 78% correct at level 1, was a wetland specialist who scored best with wetland categories. Considering the fact that none of the photo-interpreters had previously visited the USA, their performance is good.
3.2. Phenological characteristics
According to the phenological analysis described above, 7488 sample units in the data-set are ‘green’ for the whole year ()) and 45% of those are in the forest category, including rainforests (distributed in Southeast Asia, the Amazon Basin, the Congo Basin, Central America, and many Pacific Islands), temperate coastal forests (e.g. the Taiheiyo evergreen forests in Japan and the Atlantic forests in Europe, Pacific temperate coastal forests in Western North America, the Valdivian forests in southwestern South America, and the Eastern Australian temperate forests), and taiga/boreal forests in Russia and Canada, while most of the remaining ‘green’ sample units are grasslands, shrublands, and croplands. Among the sample units, 9646 are of the type ‘EVI with little change over the whole year’, as shown in ). The colour of each point shows the average value in the EVI time series, among which the sample units with lower average EVI are barren lands like desert, mainly distributed in western China, the Arabian Peninsula, the Sahara, the Kalahari in southern Africa, Chihuahuan in North America, Atacama in South America, and western Australia. Sample units with stable medium average EVI are spread over steppe, savannah, and shrublands. There are also some sample units with high average EVI that change little throughout the year. Some evergreen forests in regions of taiga/boreal forests belonging to this type were not extracted, due to interference with the EVI time series by snow cover in winter. These were judged as belonging to the type showing obvious seasonal change (see the green belt at high latitudes in Eurasia and Canada in )). This will be improved if phenological fit is weighted by snow cover (e.g. the MODIS snow/ice flags) for the same time period. The sample units of the two types mentioned above can be readily used without concerns about seasonal change. Conversely, sample units whose EVI has an obvious seasonal change throughout the year ()) may not be suitable for validating land-cover maps derived from remote-sensing images acquired at certain times. Thus, the sample units are distinguished between interpretation within and outside of the growing season in reference to seasonal change. For example, a sample unit can be interpreted as barren in the dry season, but it may be grassland in the wet season. A sample unit of snow cover in the cold season may be tundra after snow melts. Approximately 76.4% (14,865) of 19,450 sample units with an EVI showing obvious seasonal change throughout the year were interpreted from images acquired in the growing season. The sample units from images taken outside the growing season, marked in yellow in ), have higher uncertainties in land-cover interpretation, to which special attention should be paid. However, they may also be very useful when dynamic land-cover types are mapped at different seasons throughout the year. In this case, the attributes of the beginning, end, and length of the growing season could help determine the applicability of a sample unit in regard to seasonal change. According to the length of the growing season extracted by model fit ()), ecoregions of savanna, steppe, dry and thorny woodlands, and tundra have shorter growing seasons.
Figure 8. Phenological characteristics of validation samples. (a) Samples ‘green’ for the whole year. (b) Samples with little EVI variation over the whole year; the annual average EVI for this type of sample is denoted by different colours. (c) Samples with obvious EVI change over the whole year; sample colours indicate whether they were interpreted with images acquired during the growing season. (d) Samples with obvious EVI change over the whole year; sample colours indicate the length of growing season fitted with EVI time series.
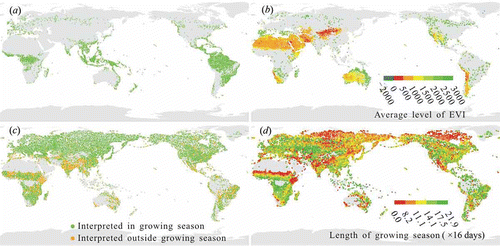
3.3. Homogeneity characteristics
Approximately 41.65% (16,104) of sample units were categorized as ‘homogeneous sample’, which are almost homogeneous in a 1 × 1 km pixel. Homogeneous sample units comprised 35.26% of forest samples and 26.23% of barren land samples, accounting for 47.65% and 56.67% of the sample units in their own land-cover types, respectively. Homogeneous sample units are relatively easy to interpret, being less affected by the error imposed by geolocation, and can be used to validate land-cover maps at lower resolutions such as 250, 300, and 500 m resolution. The homogeneity calculated by texture analysis was compared to that manually interpreted, and the consistency was 76%. Since texture-based homogeneity results are more objectively obtained, in the subsequent application demonstration we mainly used this subset of sample units in subsequent applications when homogeneous sample units were needed.
4. Application of the data-set
The entire data-set was used in validating our new 30 m-resolution global land-cover data product, FROM-GLC-agg (an improved version of FROM-GLC available at data.ess.tsinghua.edu.cn, Yu et al. (Forthcoming)). The overall accuracy is 65.51%.
Part of the data-set was used to validate and compare two land-cover maps, MODIS Land Cover 2010 and GLOBCOVER 2009. Since these two products have a spatial resolution of 500 and 300 m, respectively, only sample units that are homogeneous in an area of 1 × 1 km around the sample location can be used for this application. Cross-walking of land-cover types was done between the different classification schemes. Since the data-set was originally designed to validate a 30 m-resolution map, sample units located in homogeneous areas are unevenly distributed. Therefore, these would lead to a certain extent of overestimation of accuracy in coarser-resolution data products if used for accuracy assessment. Nonetheless, they can be used as a relative measure in assessing the potential performance of different classification procedures when applied to the same type of data-sets.
With the exclusion of sample units interpreted using Landsat imagery acquired outside their growing season (2167 sample units), we used the remaining 13,902 (approximately 36.00% of the total sample) large homogeneous sample units to assess the accuracy of MODIS and GLOBCOVER land-cover data. The removal of sample units outside of the growing season is necessary because both MODIS and GLOBCOVER land-cover products adopted land-cover classes in ‘the greenest’ season.
Overall accuracy for MODIS 2010 and GLOBCOVER 2009, calculated based on all homogeneous sample units, excluding those interpreted with images acquired outside the growing season, is 73.14% and 68.89%, respectively (). As a reference, when using this same set of homogeneous sample units in validating FROM-GLC-agg, this resulted in an overall accuracy of 67.04%, indicating that these homogeneous sample units overestimated accuracy as compared with 65.51% overall accuracy when all validation sample units were used.
Table 4. Overall accuracies calculated based on homogeneous samples in the data-set presented with a 95% confidence interval and estimated according to the standard error (Olofsson et al. Citation2014).
5. Conclusions and discussion
The data described here represent our initial efforts in developing a land-cover validation data-set that is global, well described, compatible, and temporally updated to facilitate global land-cover mapping. A total of 38,664 sample units were collected by interpreting Landsat TM/ETM+ images and MODIS EVI time series data, as well as high-resolution images from Google Earth, recording of the quality of reference data, and interpreter confidence. Acknowledging phenological and homogeneity information of the sample units, users can filter and use the data to validate land-cover maps of different spatial resolution and at appropriate time points. The validation sample can also be used separately for either individual class validation or as training data, particularly for forests, water bodies, impervious surfaces, and snow/ice, whose uncertainty is low. Our confidence in the other categories is also high following final quality control with careful analysis of the reference time series data and other ancillary data. Although the entire validation data-set was originally designed for assessing global mapping results at the 30 m level, the homogeneous sample units within the growing season that account for 36% of the entire validation data-set were used in validating MODIS 2010, GLOBCOVER 2009, and FROM-GLC-agg as an example.
Given the fact that only a small portion of global land cover changes on an annual basis, the majority of the validation sample units collected in this research are useable for multiple years over large spatial scales such as continental and global. However, over areas where land cover frequently changes beyond seasonal variations, sample units should be temporally updated. One urgently needed improvement is to collect additional images to resolve the 3.18% of unidentified sample units and to enrich the validation data-set by finding the within-growing season of land-cover types for those that are currently based on interpretation of images that were acquired outside of the growing season. Improvement of the validation data-set takes time and requires additional effort. When further data for different years are collected, sample units that have undergone changes can be identified through automatic change detection methods, and then reinterpreted. It is quite a significant challenge to further reduce uncertainties in image interpretation, and this could be partially overcome by gathering additional reference data or conducting well-designed field survey targeting on those difficult-to-interpret regions. Crowd-sourcing is an alternative to improvement of the validation data-set, by asking volunteers from all over the world to validate the sample. However, consistency in image interpretation is harder to achieve through crowd-sourcing. Future work will also include expanding the application scope of this validation data-set to the validation of other land-cover maps of different spatial resolution and in different seasons, and ensuring correct use of the sample. For example, it would be beneficial to screen out those sample units that are almost homogeneous, but the land-cover type for a high-resolution pixel is different to that for a low-resolution pixel, since a map of low resolution is a generalization of the mixed surroundings. This could be done automatically by edge detection or multi-scale texture analysis. Furthermore, inference rules about land-cover dynamics could be set up for both phenological and long-term changes, which can then be used to check the correctness of the sample or to update the sample to prolong its applicability.
The validation data-set presented in this paper can be downloaded from data.ess.tsinghua.edu.cn.
Funding
This paper is partially supported by a National High Technology Research and Development Programme of China [grant number 2009AA12200101]; the National Natural Science Funds of China [grant number 34541301445]; the Open Fund of State Key Laboratory of Remote Sensing Science [grant number OFSLRSS201202]; and a research grant from Tsinghua University [grant number 2012Z02287].
References
- Aerial Information Systems Inc. 2007. Santa Cruz Island Photo Interpretation and Mapping Classification Report, 62. Redlands, CA: Aerial Information Systems Inc.
- Arino, O., P. Bicheron, F. Achard, J. Latham, R. Witt, and J. L. Weber. 2008. “GLOBCOVER the Most Detailed Portrait of Earth.” ESA Bulletin-European Space Agency 136: 24–31.
- Bartholomé, E., and A. S. Belward. 2005. “GLC2000: A New Approach to Global Land Cover Mapping from Earth Observation Data.” International Journal of Remote Sensing 26 (9): 1959–1977. doi:10.1080/01431160412331291297.
- Congalton, R. G., and K. Green. 1999. Assessing the Accuracy of Remotely Sensed Data: Principles and Practices. Boca Raton, FL: CRC Press.
- Dai, Y. J., X. B. Zeng, R. E. Dickinson, I. Baker, G. B. Bonan, M. G. Bosilovich, A. S. Denning, P. A. Dirmeyer, P. R. Houser, G. Niu, K. W. Oleson, C. A. Schlosser, and Z. Yang. 2003. “The Common Land Model.” Bulletin of the American Meteorological Society 84: 1013–1023. doi:10.1175/BAMS-84-8-1013.
- Defourny, P., P. Bicheron, C. Brockman, S. Bontemps, E. Van Bogaert, C. Vancutsem, J. F. Pekel, M. Huc, C. Henry, F. Ranera, F. Achard, A. Di Gregorio, M. Herold, M. Leroy, and O. Arino 2009. “The First 300 M Global Land Cover Map for 2005 Using ENVISAT MERIS Time Series: A Product of the GlobCover System.” In Proceedings of the 33th International Symposium of Remote Sensing of Environment, 1–4. Joint Research Centre of the European Commission. http://publications.jrc.ec.europa.eu/repository/handle/111111111/8299
- Dickinson, R. E., R. M. Errico, F. Giorgi, and G. T. Bates. 1989. “A Regional Climate Model for the Western United States.” Climatic Change 15: 383–422. doi:10.1007/BF00240465.
- Drummond, M. A., and R. Auch. 2012. Land-cover Change in the United States Great Plains. Accessed December 13, 2012. http://landcovertrends.usgs.gov/gp/regionalSummary.html
- Fitzpatrick-Lins, K. 1981. “Comparison of Sampling Procedures and Data Analysis for a Land-Use and Land-Cover Map.” Photogrammetric Engineering and Remote Sensing 47: 343–351.
- Frey, K. E., and L. C. Smith. 2007. “How Well Do We Know Northern Land Cover? Comparison of Four Global Vegetation and Wetland Products with a New Ground-Truth Database for West Siberia.” Global Biogeochemical Cycles 21: GB1016. doi:10.1029/2006GB002706.
- Friedl, M. A., D. K. McIver, J. C. F. Hodges, X. Y. Zhang, D. Muchoney, A. H. Strahler, C. E. Woodcock, S. Gopal, A. Schneider, A. Cooper, A. Baccini, F. Gao, and C. Schaaf. 2002. “Global Land Cover Mapping from MODIS: Algorithms and Early Results.” Remote Sensing of Environment 83: 287–302. doi:10.1016/S0034-4257(02)00078-0.
- Friedl, M. A., D. Sulla-Menashe, B. Tan, A. Schneider, N. Ramankutty, A. Sibley, and X. Huang. 2010. “MODIS Collection 5 Global Land Cover: Algorithm Refinements and Characterization of New Datasets.” Remote Sensing of Environment 114 (1): 168–182. doi:10.1016/j.rse.2009.08.016.
- Fritz, S., L. Z. You, A. Bun, L. See, I. McCallum, C. Schill, C. Perger, J. Liu, M. Hansen, and M. Obersteiner. 2011. “Cropland for Sub-Saharan Africa: A Synergistic Approach Using Five Land Cover Data Sets.” Geophysical Research Letters 38: L04404. doi:10.1029/2010GL046213.
- Giri, C., Z. Zhu, and B. Reed. 2005. “A Comparative Analysis of the Global Land Cover 2000 and MODIS Land Cover Data Sets.” Remote Sensing of Environment 94: 123–132. doi:10.1016/j.rse.2004.09.005.
- Gong, P. 2006. “Information Extraction.” In Remote Sensing of Human Settlements, edited by M. Ridd, 275–334. Bethesda, MD: American Society for Photogrammetry and Remote Sensing.
- Gong, P. 2009. “Some Frontier Questions in Remote Sensing Science and Technology.” Journal of Remote Sensing 13 (1): 16–28.
- Gong, P., S. Liang, E. Carlton, Q. W. Jiang, J. Y. Wu, L. Wang, and J. V. Remais. 2012. “Urbanisation and Health in China.” Lancet 379: 843–852. doi:10.1016/S0140-6736(11)61878-3.
- Gong, P., D. Marceau, and P. J. Howarth. 1992. “A Comparison of Spatial Feature Extraction Algorithms for Land-Use Classification with SPOT HRV Data.” Remote Sensing of Environment 40: 137–151. doi:10.1016/0034-4257(92)90011-8.
- Gong, P., J. Wang, L. Yu, Y. C. Zhao, Y. Y. Zhao, L. Liang, Z. G. Niu, X. Huang, H. Fu, S. Liu, C. Li, X. Li, W. Fu, C. Liu, Y. Xu, X. Wang, Q. U. Cheng, L. Hu, W. Yao, H. Zhang, P. Zhu, Z. Zhao, H. Zhang, Y. Zheng, L. Ji, Y. Zhang, H. Chen, A. N. Yan, J. Guo, L. Yu, L. Wang, X. Liu, T. Shi, M. Zhu, Y. Chen, G. Yang, P. Tang, B. Xu, C. Giri, N. Clinton, Z. Zhu, J. Chen, and J. Chen. 2013. “Finer Resolution Observation and Monitoring of Global Land Cover: First Mapping Results with Landsat TM and ETM+ Data.” International Journal of Remote Sensing 34 (7): 2607–2654. doi:10.1080/01431161.2012.748992.
- Hansen, M. C., R. S. Defries, J. R. G. Townshend, and R. Sohlberg. 2000. “Global Land Cover Classification at 1 km Spatial Resolution Using a Classification Tree Approach.” International Journal of Remote Sensing 21 (6–7): 1331–1364. doi:10.1080/014311600210209.
- Haralick, R. M., K. Shanmugam, and I. Dinstein. 1973. “Textural Features for Image Classification.” IEEE Transactions on Systems, Man, and Cybernetics 3 (6): 610–621. doi:10.1109/TSMC.1973.4309314.
- Hird, J. N., and G. J. McDermid. 2009. “Noise Reduction of NDVI Time Series: An Empirical Comparison of Selected Techniques.” Remote Sensing of Environment 113: 248−258. doi:10.1016/j.rse.2008.09.003.
- Jonsson, P., and L. Eklundh. 2002. “Seasonality Extraction by Function Fitting to Time-Series of Satellite Sensor Data.” IEEE Transactions on Geoscience and Remote Sensing 40: 1824−1832. doi:10.1109/TGRS.2002.802519.
- Jönsson, P., and L. Eklundh. 2004. “TIMESAT – A Program for Analyzing Time-Series of Satellite Sensor Data.” Computers and Geosciences 30: 833−845. doi:10.1016/j.cageo.2004.05.006.
- Lacreek National Wildlife Refuge. 2001. Photo Interpretation and Visual Key to Vegetation Mapping Units, 44. Martin, SD.
- Lambin, E. F., H. J. Geist, and E. Lepers. 2003. “Dynamics of Land-Use and Land-Cover Change in Tropical Regions.” Annual Review of Environment and Resources 28: 205−241. doi:10.1146/annurev.energy.28.050302.105459.
- Liang, L., B. Xu, Y. L. Chen, Y. Liu, W. C. Cao, L. Q. Fang, L. M. Feng, M. F. Goodchild, P. Gong, and W. Li. 2010. “Combining Spatial–Temporal and Phylogenetic Analysis Approaches for Improved Understanding on Global H5N1 Transmission.” Plos ONE 5 (10): e13575. doi:10.1371/journal.pone.0013575.
- Liu, J., J. M. Chen, J. Cihlar, and W. M. Park. 1997. “A Process-Based Boreal Ecosystem Productivity Simulator Using Remote Sensing Inputs.” Remote Sensing of Environment 62 (2): 158–175. doi:10.1016/S0034-4257(97)00089-8.
- Loveland, T. R., B. C. Reed, J. F. Brown, D. O. Ohlen, Z. Zhu, L. Yang, and J. W. Merchant. 2000. “Development of a Global Land Cover Characteristics Database and IGBP Discover from 1 km AVHRR Data.” International Journal of Remote Sensing 21 (6–7): 1303–1330. doi:10.1080/014311600210191.
- Mayaux, P., H. Eva, J. Gallego, A. Strahler, M. Herold, S. Agrawal, S. Naumov, E. De Miranda, C. M. Bella, C. Ordoyne, Y. Kopin, and P. S. Roy. 2006. “Validation of the Global Land Cover 2000 Map.” IEEE Transactions on Geoscience and Remote Sensing 44 (7): 1728–1739. doi:10.1109/TGRS.2006.864370.
- McCallum, I., M. Obersteiner, S. Nilsson, and A. Shvidenko. 2006. “A Spatial Comparison of Four Satellite Derived 1 km Global Land Cover Datasets.” International Journal of Applied Earth Observation and Geoinformation 8: 246–255. doi:10.1016/j.jag.2005.12.002.
- Miyazaki, H., K. Iwao, and R. Shibasaki. 2011. “Development of a New Ground Truth Database for Global Urban Area Mapping from a Gazetteer.” Remote Sensing 3 (12): 1177–1187. doi:10.3390/rs3061177.
- Olofsson, P., G. M. Foody, M. Herold, S. V. Stehman, C. E. Woodcock, and M. A. Wulder. 2014, in press “Good Practices for Estimating Area and Assessing Accuracy of Land Change.” Remote Sensing of Environment. doi:10.1016/j.rse.2014.02.015.
- Olofsson, P., S. Stehman, C. Woodcock, D. Sulla-Menashe, A. Sibley, J. Newell, M. Friedl, and M. Herold. 2012. “A Global Land Cover Validation Data Set, Part I: Fundamental Design Principles.” International Journal of Remote Sensing 33 (18): 5768–5788. doi:10.1080/01431161.2012.674230.
- Ouray National Wildlife Refuge. 2001. Photo Interpretation and Visual Key to Vegetation Mapping Units, 58. Vernal, UT: Ouray National Wildlife Refuge.
- Overton, W. S., D. White, D. L. Jr, and Stevens. 1991. Design Report for EMAP: Environmental Monitoring and Assessment Program. EPA/600/3-91/053. Washington, DC: US Environmental Protection Agency.
- Pérez-Hoyos, A., F. J. García-Haro, and J. San-Miguel-Ayanz. 2012. “Conventional and Fuzzy Comparisons of Large Scale Land Cover Products: Application to CORINE, GLC2000, MODIS and GlobCover in Europe.” ISPRS Journal of Photogrammetry and Remote Sensing 74: 185–201. doi:10.1016/j.isprsjprs.2012.09.006.
- Pflugmacher, D., O. N. Krankina, W. B. Cohen, M. A. Friedl, D. Sulla-Menashe, R. E. Kennedy, P. Nelson, T. V. Loboda, T. Kuemmerle, E. Dyukarev, V. Elsakov, and V. I. Kharuk. 2011. “Comparison and Assessment of Coarse Resolution Land Cover Maps for Northern Eurasia.” Remote Sensing of Environment 115 (12): 3539–3553. doi:10.1016/j.rse.2011.08.016.
- Ridder, R. M. 2007. Global Forest Resources Assessment 2010, Options and Recommendations for a Global Remote Sensing Survey, 56. Rome: FAO.
- Rubel, F., and M. Kottek. 2010. “Observed and Projected Climate Shifts 1901-2100 Depicted by World Maps of the Köppen-Geiger Climate Classification.” Meteorologische Zeitschrift 19 (2): 135–141. doi:10.1127/0941-2948/2010/0430.
- Sahr, K., and D. White. 1998. “Discrete Global Grid Systems, Computing Science and Statistics.” Proceedings of the 30th Symposium on the Interface 30: 269–278.
- Scepan, J., G. Menz, and M. C. Hansen. 1999. “The Discover Validation Image Interpretation Process.” Photogrammetric Engineering and Remote Sensing 65: 1075–1081.
- Sedano, F., P. Gong, and M. Ferrao. 2005. “Land Cover Assessment with MODIS Imagery in Southern African Miombo Ecosystems.” Remote Sensing of Environment 98 (4): 429–441. doi:10.1016/j.rse.2005.08.009.
- See, L., and S. Fritz. 2006. “A Method to Compare and Improve Land Cover Datasets: Application to the GLC-2000 and MODIS Land Cover Products.” IEEE Transactions on Geoscience and Remote Sensing 44 (7): 1740–1746. doi:10.1109/TGRS.2006.874750.
- Southwest Florida Water Management District. 2010. Photo Interpretation Key for Land Use Classification, 173. Brooksville: Southwest Florida Water Management District.
- Stehman, S. V., P. Olofsson, C. E. Woodcock, M. Herold, and M. A. Friedl. 2012. “A Global Land-Cover Validation Data Set, II: Augmenting a Stratified Sampling Design to Estimate Accuracy by Region and Land-Cover Class.” International Journal of Remote Sensing 33 (22): 6975–6993. doi:10.1080/01431161.2012.695092.
- Stehman, S. V., T. L. Sohl, and T. R. Loveland. 2003. “Statistical Sampling to Characterize Recent United States Land-Cover Change.” Remote Sensing of Environment 86: 517–529. doi:10.1016/S0034-4257(03)00129-9.
- Strahler, A., L. Boschetti, G. M. Foody, M. A. Fiedl, M. C. Hansen, M. Herold, P. Mayaux, J. T. Morisette, S. V. Stehman, and C. Woodcock. 2006. Global Land Cover Validation: Recommendations for Evaluation and Accuracy Assessment Of Global Land Cover Maps, Report of Committee of Earth Observation Satellites (CEOS) – Working Group on Calibration and Validation (WGCV). Luxembourg: Office for Official Publications of the European Communities.
- Sun, F. D., Y. Y. Zhao, P. Gong, R. H. Ma, and Y. J. Dai. 2014. “Monitoring Dynamic Changes of Global Land Cover Types: Fluctuations of Major Lakes in China Every 8 Days during 2000–2010.” Chinese Science Bulletin 59 (2): 171–189. doi:10.1007/s11434-013-0045-0.
- Tateishi, R., B. Uriyangqai, H. Al-Bilbisi, M. A. Ghar, J. Tsend-Ayush, T. Kobayashi, A. Kasimu, N. T. Hoan, A. Shalaby, B. Alsaaideh, T. Enkhzaya, G. Click here to enter text., and H. P. Sato. 2011. “Production of Global Land Cover Data – GLCNMO.” International Journal of Digital Earth 4 (1): 22–49. doi:10.1080/17538941003777521.
- Tchuenté, A. T. K., J. Roujean, and S. M. Jong. 2011. “Comparison and Relative Quality Assessment of the GLC2000, GLOBCOVER, MODIS and ECOCLIMAP Land Cover Data Sets at the African Continental Scale.” International Journal of Applied Earth Observation and Geoinformation 13 (2): 207–219. doi:10.1016/j.jag.2010.11.005.
- Torresan, S., A. Critto, J. Rizzi, and A. Marcomini. 2012. “Assessment of Coastal Vulnerability to Climate Change Hazards at the Regional Scale: the Case Study of the North Adriatic Sea.” Natural Hazards and Earth System Science 12 (7): 2347–2368. doi:10.5194/nhess-12-2347-2012.
- USGS-NPS. 1996. Vegetation Mapping and Visual Keys, 137. Crane Lake, MN: Voyageurs National Park.
- van Dijk, A. I. J. M., J. L. Peña-Arancibia,and L. A. Bruijnzeel. 2012. “Land Cover and Water Yield: Inference Problems When Comparing Catchments with Mixed Land Cover.” Hydrology and Earth System Sciences 16: 3461–3473. doi:10.5194/hess-16-3461-2012.
- Wang, J., Y. Y. Zhao, C. C. Li, L. Yu, D. S. Liu, and P. Gong. Forthcoming. “Mapping Global Land Cover in 2001 and 2010 with Spatial–Temporal Consistency at 250 m Resolution.” ISPRS Journal of Photogrammetry and Remote Sensing. http://dx.doi.org/10.1016/j.isprsjprs.2014.03.007
- White, D., J. A. Kimerling, and S. W. Overton. 1992. “Cartographic and Geometric Components of a Global Sampling Design for Environmental Monitoring.” Cartography and Geographic Information Science 19 (1): 5–22. doi:10.1559/152304092783786636.
- Wickham, J. D., S. V. Stehman, L. Gass, J. Dewitz, J. A. Fry, and T. G. Wade. 2013. “Accuracy Assessment of NLCD 2006 Land Cover and Impervious Surface.” Remote Sensing of Environment 130: 294–304. doi:10.1016/j.rse.2012.12.001.
- Yang, J., P. Gong, R. Fu, M. H. Zhang, J. Chen, S. Liang, B. Xu, J. Shi, and R. Dickinson. 2013. “The Role of Satellite Remote Sensing in Climate Change Studies.” Nature Climate Change 3 (10): 875–883. doi:10.1038/nclimate1908.
- Yu, L., and P. Gong. 2012. “Google Earth as a Virtual Globe Tool for Earth Science Applications at the Global Scale: Progress and Perspectives.” International Journal of Remote Sensing 33 (12): 3966–3986. doi:10.1080/01431161.2011.636081.
- Yu, L., J. Wang, X. C. Li, C. C. Li, Y. Y. Zhao, and P. Gong. Forthcoming. “FROM-Hierarchy, a Multi-Resolution Global Land Cover Data Set.” Science China-Earth Sciences.
- Zhong, L. H., P. Gong, and G. S. Biging. 2012. “Phenology-Based Crop Classification Algorithm and Its Implications on Agricultural Water Use Assessments in California’s Central Valley.” Photogrammetric Engineering and Remote Sensing 78 (8): 799–813. doi:10.14358/PERS.78.8.799.