Abstract
Many studies have shown the considerable potential for the application of remote-sensing-based methods for deriving estimates of lake water quality. However, the reliable application of these methods across time and space is complicated by the diversity of lake types, sensor configuration, and the multitude of different algorithms proposed. This study tested one operational and 46 empirical algorithms sourced from the peer-reviewed literature that have individually shown potential for estimating lake water quality properties in the form of chlorophyll-a (algal biomass) and Secchi disc depth (SDD) (water transparency) in independent studies. Nearly half (19) of the algorithms were unsuitable for use with the remote-sensing data available for this study. The remaining 28 were assessed using the Terra/Aqua satellite archive to identify the best performing algorithms in terms of accuracy and transferability within the period 2001–2004 in four test lakes, namely Vänern, Vättern, Geneva, and Balaton. These lakes represent the broad continuum of large European lake types, varying in terms of eco-region (latitude/longitude and altitude), morphology, mixing regime, and trophic status. All algorithms were tested for each lake separately and combined to assess the degree of their applicability in ecologically different sites. None of the algorithms assessed in this study exhibited promise when all four lakes were combined into a single data set and most algorithms performed poorly even for specific lake types. A chlorophyll-a retrieval algorithm originally developed for eutrophic lakes showed the most promising results (R2 = 0.59) in oligotrophic lakes. Two SDD retrieval algorithms, one originally developed for turbid lakes and the other for lakes with various characteristics, exhibited promising results in relatively less turbid lakes (R2 = 0.62 and 0.76, respectively). The results presented here highlight the complexity associated with remotely sensed lake water quality estimates and the high degree of uncertainty due to various limitations, including the lake water optical properties and the choice of methods.
1. Introduction
Lake water quality is an overarching term to describe the biochemical and physical properties of lake water and is often used as a measure of the value of lakes as a freshwater resource. However, the water quality of lakes can be affected by environmental change. In fact, trends and changes in lake water quality have shown strong correlation with synoptic weather phenomena and climatic variability (e.g. Livingstone and Padisák Citation2007; Gerten and Adrian Citation2002) with lakes now recognized as important sentinels of climate change (e.g. Adrian et al. Citation2009; Williamson et al. Citation2009). Upscaling information derived from individual measurement stations to the whole-lake scale and beyond to explore ecological issues such as regional coherence and response to environmental change requires a better understanding of system sensitivities and behaviours. In addition, the need for adequate and affordable monitoring regimes is a key requirement of national and international regulations, as well as lake water quality monitoring programmes such as the EU Water Framework Directive (WFD) and US National Lake Assessment. Such needs and requirements can only be addressed by means of techniques like remote sensing that can be applied over long temporal and spatial scales (i.e. regional, continental, and ultimately global) to produce lake water quality estimates.
There have been many methodological approaches used to retrieve lake water quality from remote-sensing data. These can be broadly classified into empirical, semi-empirical (also known as semi-analytical), and analytical. According to Matthews (Citation2011), the empirical approach is most often used owing to its computational simplicity and reliability in lake waters that make it more favourable than other relatively more advanced and complex methods. An example of a widely used empirically based operational algorithm for oceanic waters is the MODIS three-band ocean color algorithm (OC3) product for the estimation of chlorophyll-a (Campbell and Feng Citation2005; NASA Ocean Color Citation2009). However, despite the plethora of studies that have developed empirical algorithms to map water quality properties in lakes, as yet no operational algorithm has been developed for freshwater. This is due to the optically complex characteristics of freshwater, which is often determined by a combination of spatially and temporally variable properties, such as phytoplankton, suspended material, and coloured dissolved organic matter (CDOM), all of which affect water colour and transparency in varying degrees (IOCCG Citation2000, Citation2006). Any operational algorithm(s) to accurately map freshwater would need to account for all of these properties (phytoplankton, suspended material, and CDOM) rather than focusing on each one of them individually. For this to be achieved would require prior knowledge on lake typology to help assess the partial contribution of each property to the overall lake water quality in the waterbody under investigation, which is not generally available even for the best studied lakes in the world. For the vast majority of the estimated 117 million freshwater bodies (>0.2 ha) in the world (Verpoorter et al. Citation2014), information on their properties is not available, and so the potential to develop a generic empirically based algorithm becomes a major challenge.
Nevertheless, empirical methods based on a combination of in situ measurements and remote-sensing data have been used successfully to estimate individual water properties, including chlorophyll-a (e.g. Vos and Rijkeboer Citation2000; Vos et al. Citation2003; Chavula et al. Citation2009) and Secchi disc depth (SDD) (e.g. Allee and Johnson Citation1999; Sawaya et al. Citation2003; Wu et al. Citation2008): two lake water properties that we are henceforth referring to when discussing lake water quality. A lot of effort has focused on the development of remote-sensing techniques that can reliably map these two lake water quality parameters at local scales (i.e. an individual or a few neighbouring lakes) (e.g. Gitelson et al. Citation1993; Hedger et al. Citation1996; Strömbeck and Pierson Citation2001) and for specific months (e.g. Allee and Johnson Citation1999; Dekker and Peters Citation1993; Kallio et al. Citation2001) or seasons (e.g. Dall’olmo et al. Citation2005; Gitelson et al. Citation1993). As a result, a large number of studies have demonstrated estimation of chlorophyll-a and SDD for single or groups of similar lakes at local scales and over relatively short periods, at moderate to high accuracies. Even though in the last decade or so interest has shifted towards the development of methods to analyse regional lake behaviour in response to multiple exogenous drivers of change (including climate), the developed algorithms have tended to be sensor-, date-, or scene-specific (e.g. Kloiber, Brezonik, and Bauer Citation2002; Kloiber et al. Citation2002; Olmanson, Bauer, and Brezonik Citation2008) and their reliable application at other times and locations has not yet been tested.
To realize the potential of remote sensing to generate synoptic results, i.e. yielding insights into ‘within-’ and ‘between-lake’ spatial variability of lake properties across multiple sites and at multiple scales simultaneously, requires a process of developing standardized approaches, a single universal or a suite of algorithms that can produce reliable and repeatable results across both time and space, for a variety of lake types and conditions. In search of transferable and repeatable algorithms for the retrieval of chlorophyll-a and SDD in lake waters that do not require prior knowledge on lake typology, this study aims to test a number of published empirical algorithms, which have previously shown promising results when applied independently to estimate these two key water quality parameters.
To fulfil our aim, we were granted access to the Terra/Aqua Moderate Resolution Imaging Spectroradiometer (MODIS) satellite archive hosted at the Dundee Satellite Receiving Station (DSRS) at the University of Dundee, which is part of the UK’s Natural Environment Research Council Earth Observation Data Acquisition and Analysis Service (NEODAAS). This long-term archive was fundamental as it provided data at large temporal and spatial scales fulfilling the remote-sensing data requirements for this study. MODIS employs nine narrow wavebands (Bands 8–16) in the visible and near-infrared (NIR) region for mapping ocean colour at 1 km spatial resolution. Previous limnological studies have also highlighted the potential of MODIS wavebands 1–2 (250 m) and 3–4 (500 m) to map chlorophyll-a and SDD (Wu et al. Citation2008, Citation2009) and other lake water quality parameters such as total suspended solids (e.g. Zhang et al. Citation2010). However, the coarse spatial resolution of the instrument has limited the number of studies that have used MODIS data to map lake water quality (Matthews Citation2011). On the other hand, most lake studies have employed satellite sensors with finer spatial resolutions such as Landsat Thematic Mapper (TM)/Enhanced Thematic Mapper Plus (ETM+) at 30 m (e.g. Allee and Johnson Citation1999; Baban Citation1993; Dekker and Peters Citation1993; Sawaya et al. Citation2003; Tyler et al. Citation2006), as well as airborne and in situ remote-sensing instruments at finer spatial resolutions (e.g. Kallio et al. Citation2001; Koponen et al. Citation2002; Thiemann and Kaufmann Citation2000). Matthews (Citation2011) provides a comprehensive overview of limnological studies that have used remote sensing to develop empirical algorithms.
The objectives of our study were to (1) select promising algorithms that were developed for lakes spanning a wide range of optical characteristics; (2) access and exploit the long MODIS archive hosted at DSRS, University of Dundee; (3) devise and apply an algorithm suitability test to identify and exclude algorithms that were unsuitable for use with the remote-sensing data available for this study; and (4) assess the applicability of promising algorithms across space and time in the search for a suite of robust algorithms that are transferable and repeatable. In selecting which algorithms to test, we chose only those that were developed for lake waters, are fully available in peer-reviewed publications (including all relevant details to enable implementation) and had demonstrated a relatively strong relationship between in situ chlorophyll-a or SDD and remote-sensing data (R2 ≥ 0.5), but was irrespective of the remote-sensing instrument employed.
2. Methods
2.1. Study sites
Lakes Geneva/Léman (G), Balaton (B), Vättern (Vt), and Vänern (Vn) were selected because (1) they represent three major climatic ecoregions of Europe (Central European (G), Hungarian (B), and Finnish (Vt and Vn)); and (2) their characteristics cover the entire range of mixing and eutrophication conditions; Lake Geneva is monomictic and mesotrophic, Lake Balaton is polymictic and eutrophic, whilst Lakes Vättern and Vänern are dimictic and oligotrophic. In addition, they all have large surface areas (ranging from 584 to 5648 km2; ) and few, if any, islands, which makes them suitable for remote-sensing techniques.
Table 1. Morphological properties of the lakes covered in this study.
2.2. Field measurements
This work relied upon in situ measurements of water quality previously acquired by various European research institutes (International Commission for the Protection of Lake Geneva (CIPEL), Hungarian Ministry of Environmental Protection and Water Management and Hungarian Ministry of Health, and Swedish Environmental Protection Agency) that were subsequently matched to the satellite data. Measurements of chlorophyll-a and SDD were supplied for various sampling sites within each of the four study lakes () with variable sampling periods (Vt: 2001–2003; rest: 2001–2004), frequencies (B: weekly; G: biweekly or monthly, Vt/Vn: monthly) and depths (G: 0 m; B: 0.3–0.5; Vt/Vn: 0.5 m). All institutes used spectrophotometric methods to measure chlorophyll-a in the water samples collected. We are not aware of any specific inter-laboratory calibration to report on the consistency of laboratory-based estimation of chlorophyll-a between laboratories but as all these national agencies have a requirement to report water quality according to EU WFD standards, we assume that the in situ measurements are comparable between sites.
2.3. Satellite data
Terra and Aqua MODIS cloud-free scenes that coincided with dates of in situ measurements were acquired for the periods 2001–2004 and 2002–2004, respectively. Cloud masking and pre-processing was performed by NEODAAS prior to delivery of both Level 1B and Level 2 data for both sensors. Level 1B data products are calibrated and geolocated at-satellite (or top of the atmosphere) radiances (Ltoa) for all 36 MODIS bands (i.e. radiance for bands 1–19 and 26 and emissive radiance for bands 20–25 and 27–36) and at-satellite reflectance (Rtoa) for the reflective solar bands (i.e. MODIS bands 1–19 and 26). MODIS Level 2 data comprise calibrated, geolocated, and atmospherically corrected remote-sensing reflectance (Rrs) and normalized water-leaving radiances (nLw) for bands 1–16. The Level 2 data were atmospherically corrected using the standard National Aeronautics and Space Administration (NASA) Arnone model, which is a NIR correction model based on reflectance in the 670 nm region of the spectrum and is specifically proposed for applications where chlorophyll-a concentrations are mapped (NASA Ocean Color Citation2009). MODIS OC3 products were also included in the Level 2 data.
3. Water quality estimation algorithms
This section lists and describes all algorithms tested in this project, including ‘standard’ and ‘non-standard’. Standard algorithms are used operationally to estimate water quality parameters, while non-standard algorithms are empirical algorithms proposed in the published literature, but their spatial and temporal transferability has not yet been tested. The empirical chlorophyll-a and SDD estimation algorithms were assigned a code name, based on the initial of the first author, the parameter used, the remote-sensing bands employed and (if applicable) the month of application for quick reference (–).
Table 2. List of chlorophyll-a (Cchl-a) estimation algorithms developed using Landsat TM and Daedalus 1268 ATM raw digital number (DN) data or Ltoa.
Table 3. List of chlorophyll-a (Cchl-a) estimation algorithms developed using Daedalus 1268 ATM and AISA Ltoa or nLw data.
3.1. Chlorophyll-a estimation algorithms
A total 36 chlorophyll-a estimation algorithms were tested. The only one currently operational is the Terra/Aqua MODIS OC3 developed for Case I oceanic waters (NASA Ocean Color Citation2009). The remaining were sourced from the published literature (–).
Table 4. List of chlorophyll-a (Cchl-a) estimation algorithms developed using field spectroradiometer (FSR) Rtoa or Rrs data.
3.2. SDD estimation algorithms
There are no operational algorithms for the estimation of SDD. However, 11 empirical algorithms were tested ().
Table 5. List of SDD estimation algorithms developed using Landsat TM and AISA raw DN data or Ltoa.
4. Application of algorithms
4.1. Remote-sensing data simulation
Spectral, spatial, and radiometric rescaling are common techniques used to transform data from one sensor in order to simulate the specifications of another. For example, Koponen et al. (Citation2002) simulated Environmental Satellite (Envisat) Medium Resolution Imaging Spectrometer (MERIS) using airborne imaging spectrometer for applications (AISA) data and used the information to classify lake water quality. In this study, because algorithms originally developed for sensors other than Terra/Aqua MODIS were tested, data simulation was required prior to the application of the algorithms. Specifically, the available Terra/Aqua MODIS data were used to simulate data from Landsat TM/ETM+, Daedalus 1268 Airborne Thematic Mapper (ATM), AISA, and four field spectroradiometers. describes the process followed to simulate multispectral or hyperspectral data using other multispectral data.
Figure 2. Flowchart of the simulation of multispectral or hyperspectral data using other multispectral data.
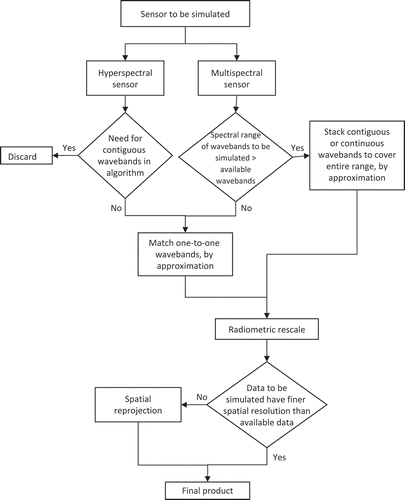
Radiometric rescaling and spatial re-projections were unnecessary for this study. The first was not performed because only radiance and reflectance data (i.e. Rtoa, Ltoa, Rrs, and nLw) were used. The second was omitted as re-projection of the spatially coarse Terra/Aqua MODIS data into finer spatial scales would not add extra information to the data set (). Spectral simulation was instead performed. The Terra/Aqua MODIS wavebands that best reflect the range of each Landsat TM/ETM+ and Daedalus 1268 ATM multispectral wavebands were identified (). Terra/Aqua MODIS bands are relatively narrow and not contiguous, so only one Terra/Aqua MODIS band was used for the simulation of a particular Landsat TM or Daedalus 1268 ATM band in the optical and NIR parts of the spectrum. The wide range of Landsat TM band 6 necessitated the use of two non-contiguous Terra/Aqua MODIS thermal wavebands to cover its full range, whose mean variance was calculated during the simulation procedure.
Table 6. Adaptation of MODIS spectral wavebands to simulate Landsat TM/ETM+ and Daedalus 1268 ATM wavebands used in this project.
All Terra/Aqua MODIS bands used for the simulation fell completely within the spectral range of each corresponding Landsat TM and Daedalus 1268 ATM band (). The latter was important because the upper and lower edges of the spectral range that a waveband covers exhibit lower sensitivity than the central part of the band, where generally the response peaks. Ensuring that this was the case was important because the radiation signal measured by any remote-sensing instrument is not uniformly detected throughout the full spectral range of each band. As a result, during simulation it is important to ensure that the band centres (and if possible also the spectral ranges) of the original (i.e. Terra/Aqua MODIS) and the simulated (e.g. Landsat TM, Daedalus 1268 ATM, etc.) bands coincide.
Only the specific Landsat TM/ETM+ and Daedalus 1268 ATM wavebands needed for the application of the algorithms were simulated. As a result, only TM bands 1–6 were simulated, while TM band 7 was omitted. The ETM+ bands 2 and 3 are exactly the same as the TM bands 2 and 3, so the simulation was performed only once for both sensors. The Daedalus 1268 ATM bands 2, 3, and 5 needed for the application were exactly the same (in terms of their spectral and radiometric resolution) as Landsat TM bands 1, 2, and 3, so, the simulation was performed only once for both sensors in this case too. The Daedalus 1268 ATM band 1 was also simulated.
The MODIS wavebands that best matched the AISA and field spectroradiometer data were used as an approximation, where applicable. and show the hyperspectral channels (AISA and field spectroradiometers, respectively) used in the empirical algorithms in comparison to the Terra/Aqua MODIS bands 8–16. Some of the empirical algorithms could not be applied due to lack of appropriate Terra/Aqua MODIS spectral coverage to approximate specific AISA and field spectrometer wavebands. In this category fell all nine algorithms developed by Kallio et al. (Citation2001), the May chlorophyll-a estimation algorithm by Pulliainen et al. (Citation2001) (‘PchlMay’), and the chlorophyll-a and SDD estimation algorithms by Koponen et al. (Citation2002) (‘Kopchla’ and ‘KopSDD’).
Figure 3. AISA waveband centre wavelengths and corresponding Terra/Aqua MODIS waveband centres used in approximation. In grey the hyperspectral channels that approximated with the same MODIS waveband as another hyperspectral channel used in the same algorithm, or did not approximate any MODIS waveband, and therefore were not used.
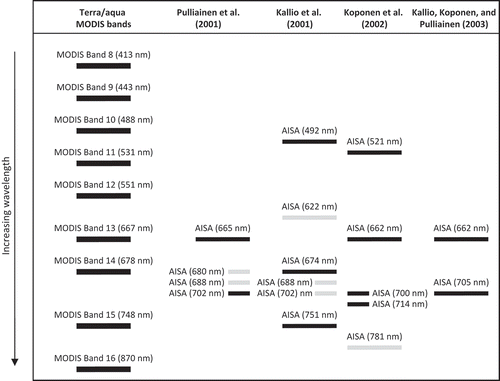
Figure 4. Waveband centres of field spectroradiometer-based algorithms and corresponding Terra/Aqua MODIS waveband centres used in approximation.
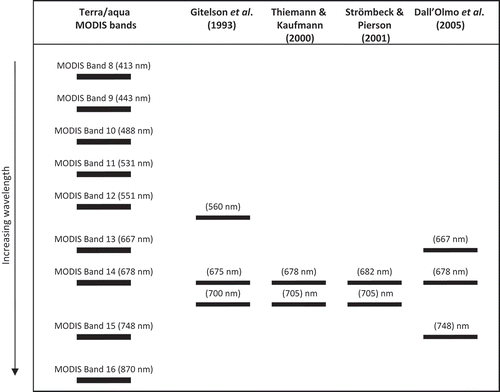
A distinct feature of the Terra/Aqua MODIS sensor is the existence of MODIS band 13 low and 13 high (and 14 low and 14 high), which record radiance at the same wavelength but saturate at different temperatures. The choice of low or high waveband depends on the application. Here, MODIS bands 13 low and 14 low were always used for consistency because in some scenes the 13 high and 14 high values were missing.
4.2. Suitability of empirical algorithms
In order to assess the suitability of the empirical algorithms for use with the remote-sensing data available to us, a suitability test was devised. First, the range of in situ chlorophyll-a concentrations and SDD values for mesotrophic Lake Geneva and oligotrophic Vättern were identified using the field data set. There were no Aqua MODIS data for eutrophic Lake Balaton, so it could not be used at this stage and Lake Vänern is also oligotrophic, so using it would not add any more information. Then the maximum and minimum field values were applied to the inverted empirical algorithms to generate the range of required remote-sensing data (henceforth referred to as required inputs) for each simulated Landsat TM/ETM+, Daedalus 1268 ATM, AISA, and field spectroradiometer bands in order to produce the expected range of field measurements (as identified above). Mean pixel values from the appropriate remote-sensing products (i.e. Ltoa, Rtoa, nLw, or Rrs) were extracted using a 9 × 9 pixel window centred on the location of the station(s) in Lakes Geneva and Vättern for all scenes on dates when field data were available. The range of the latter (henceforth referred to as available inputs) was compared to the range of the required inputs and the percentage of overlap between the two ranges was calculated for each algorithm. This procedure showed whether the available data were appropriate for use with the empirical algorithms and therefore helped determine the suitability of algorithms for further application.
In total, 34 chlorophyll-a and SDD empirical algorithms were tested for their suitability. Two algorithms (‘DchlB1jun’ and ‘DchlB3jun’) proved unsuitable because the range of required data failed to overlap with any available simulated data; hence, these algorithms were excluded from further analysis. Also, it was impossible to determine the range of required inputs per band for five algorithms, so these algorithms were considered potentially suitable and were applied to the simulated satellite data.
The majority of the empirical algorithms required input satellite data that overlapped to some extent with the simulated remote-sensing data (derived from either Terra/Aqua MODIS or both) available in this project. For some algorithms only a small fraction of the available simulated data actually fell within the range of required inputs, meaning that the rest of the data were expected to result in outputs outside the range of field data in Lakes Geneva and Vättern. Five empirical algorithms exhibited very low percentages of overlap (<25%) and were considered unsuitable. On the other hand, 20 algorithms exhibited very high percentages of overlap (>60%) for either Terra or Aqua MODIS-derived simulated data, or both. Two algorithms (‘DchlB2jul’ and ‘DlnB2jul’) exhibited 38% overlap for the Terra MODIS-derived simulated data but no overlap for the Aqua MODIS-derived simulated data; they were considered potentially suitable and thus included in further analysis. Including the five algorithms that could not be tested and the one that was potentially suitable, 27 empirical water quality estimation algorithms were applied to the Terra/Aqua MODIS-derived simulated data to estimate chlorophyll-a and SDD.
4.3. Accuracy assessment tools
Once the algorithms were applied to the simulated data, the outputs were checked for the presence of extreme values, which can occur due to, for example, field sampling or satellite instrument errors. Extreme values may affect the outputs of statistical tests (Kinnear and Gray Citation2008) and in some cases need to be removed before statistical analysis. Here the z-scores (a measure of how many standard deviations a value is from the data set mean) for both field and satellite data sets were calculated and any values that were more than three standard deviations above or below the mean identified as extremes (Wheeler, Shaw, and Barr Citation2004) were removed. In addition, all field and satellite data sets were tested for normality using the Kolmogorov–Smirnov (K-S) test. In the case where data sets were not normally distributed, parametric tests were omitted and the results of non-parametric tests were considered instead (Kinnear and Gray Citation2008).
The spatial and temporal transferability of all algorithms was then tested by assessing their accuracy in retrieving chlorophyll-a and SDD. During this step, lists of coinciding field data and remotely sensed estimates of the two water quality parameters were produced for each lake and year. The remotely sensed data were averaged values of the parameters calculated from a 9-pixel squared grid centred on the pixel where the sampling station was located to avoid issues of geometric distortion and consequent imprecise determination of the location of sampling points in the scenes. The assumption was made that a point measurement from the sampling station was representative of the average value of the parameter studied for a 9 km2 area containing the sample (Lavender et al. Citation2004), but clearly this would depend upon the intrinsic scale of variation of the parameter under consideration. In fact, Kloiber et al. (Citation2002) used a 9-pixel average as a minimum and showed that the accuracy of water quality estimations in 20 lakes significantly improved when the number of pixels (used for the computation of the average) increased from one to nine. Baban (Citation1993) similarly found that the use of a 3-pixel average, when comparing Landsat TM data to field measurements, was the optimum size of kernel as it reduced noise in the data or the selection of biased pixel values.
The relationship between estimated and in situ measured water quality parameters was assessed using correlation analysis. The parametric product–moment (Pearson’s) correlation coefficient (r) and non-parametric Spearman’s rho correlation coefficient (ρ) were used. Both correlation coefficients were computed for all data sets with sample sizes between 6 and 30 for comparison. For data sets with n less than six, only non-parametric tests were applied, whilst for data sets with n greater than 30, the choice of test depended upon normality. Finally, the coefficient of determination (R2), root mean square error (RMSE), and systematic error (bias) of prediction were calculated for each water quality estimation algorithm that produced promising results as measures of its accuracy. The significance of all statistical tests was calculated at the 99% (0.01) level of significance (two-tailed), unless otherwise stated.
5. Algorithm transferability and repeatability
5.1. Chlorophyll-a
Chlorophyll-a was estimated using the Terra/Aqua MODIS OC3 algorithm in Vättern and Geneva due to unavailability of MODIS data over the other two sites. The empirical algorithms were tested using data from all four study sites.
5.1.1. Terra/Aqua MODIS OC3 algorithm
The field chlorophyll-a data from Lakes Geneva (n = 23) and Vättern (n = 11) were weakly correlated with the corresponding Terra/Aqua MODIS OC3 estimates (ρ ≤ 0.12). The correlation improved slightly, when data from both lakes were combined for all available years (ρ = 0.391, n = 34, p < 0.05; ). However, the correlation coefficient was still weak and, therefore, the Terra/Aqua MODIS OC3 algorithm was considered unsuitable for the estimation of chlorophyll-a concentrations in Lakes Geneva and Vättern in the study period.
5.1.2 Empirical chlorophyll-a estimation algorithms
Lakes Vättern and Vänern were grouped together to represent oligotrophic lakes. Matching coincident pairs of satellite and field data from eutrophic Lake Balaton were very few (n ≤ 4) and were only used when all four lakes were combined into a common data set. Significant correlations between field data and remotely sensed estimates existed in only a few cases. It was noticeable how results varied depending on the sensor used (Terra MODIS or Aqua MODIS), suggesting a low degree of consistency between the two sensors when the same algorithms were applied ( and ).
Table 7. Correlation of field chlorophyll-a data with corresponding simulated remotely sensed data (using Terra MODIS data only) for empirical algorithms in 2001–2004 in Lakes Geneva, Balaton, Vättern, and Vänern.
When only Terra MODIS data were used, most chlorophyll-a estimation algorithms produced poor results (). No algorithm produced a significant correlation with the field data in Lake Geneva. By contrast, in oligotrophic Lakes Vättern and Vänern, three out of four algorithms proposed by Dekker and Peters (Citation1993), originally developed for eutrophic lakes, showed strong correlations with field data (r ranging from 0.768 to 0.792; ρ = 0.937 for all three), suggesting they have potential to be applicable to different types of lake waters. Two single-band algorithms recommended by George (Citation1997), which employ the green waveband and were developed specifically for low (‘GeochlB3lo’) or high (‘GeochlB3hi’) chlorophyll-a concentrations, exhibited high correlation coefficients in oligotrophic lakes only (r = 0.790 and ρ = 0.937 for both algorithms). However, the sample size for all these data sets was small (n = 7) and additional data are required to investigate the significance of these observations. When the four lakes (including Lake Balaton) were combined for Terra MODIS data, the above-mentioned algorithm exhibited low correlation coefficients, but three other algorithms exhibited significant correlations with field measurements. The two band ratio algorithms by George (Citation1997), ‘GeochlB32lo’ and ‘GeochlB32hi’, which employ the blue and green bands, showed good correlation to field data from all four lakes ranging from oligotrophic to eutrophic (ρ = 0.620 for both algorithms, n = 23). The ‘Gitchl02’ algorithm, which was developed for lakes with various characteristics, also showed potential in all four lakes when Terra MODIS data were used (r = 0.458; ρ = 0.466, n = 25). The above results suggest that these eight empirical algorithms are potentially non-site-specific.
By contrast, when only Aqua MODIS data were used, the results of the empirical chlorophyll-a estimation algorithms were poor in all cases for all algorithms (). Finally, when the algorithm outputs for both Terra and Aqua MODIS data were combined, all but one of the chlorophyll-a estimation algorithms exhibited poor results (). The ‘Strömchla’ algorithm by Strömbeck and Pierson (Citation2001), originally developed for eutrophic lake waters, produced promising results (r = −0.574; ρ = −0.609, n = 17) for the oligotrophic lakes Vättern and Vänern.
Table 8. Correlation of field chlorophyll-a data with corresponding simulated remotely sensed data (using Aqua MODIS data only) for empirical algorithms in 2001–2004 in Lakes Geneva, Vättern, and Vänern.
Table 9. Correlation of field chlorophyll-a data with corresponding simulated remotely sensed data (using both Terra and Aqua MODIS data) for empirical algorithms in 2001–2004 in Lakes Geneva, Balaton, Vättern, and Vänern.
However, a strong correlation between field data and remotely sensed estimates does not necessarily mean that the algorithm works well, as ideally the algorithm should be able to reliably predict the variable of interest based upon that relationship. The potential for prediction is analysed here by presenting the R2, RMSE, and bias of the nine promising chlorophyll-a estimation algorithms (). Four algorithms exhibited low coefficients of determination (R2 ≤ 0.33) and high degree of scatter, namely ‘Strömchla’, ‘GeochlB32lo’, ‘GeochlB32hi’, and ‘Gitchl02ʹ (). ‘DchlB2jul’, ‘DlnB2jul’, and ‘GeochlB3hi’ produced high coefficients of determination for the linear regression (R2 > 0.6), but overestimated chlorophyll-a concentrations with bias equal to 362.7, 2628.2, and 168.9 µg l–1, respectively. The ‘GeochlB3lo’ algorithm was developed for oligotrophic waters and indeed showed potential here in the oligotrophic lakes Vättern and Vänern, but always overestimated chlorophyll-a concentrations by a factor of 10 ((b)); the bias was 38.3 µg l–1. The performance of these last four algorithms could improve with calibration in order to be transferred successfully to other lakes of low productivity, but requires additional remotely sensed data for further investigation. The ‘DlnB2jun’ algorithm, originally developed in eutrophic lakes, showed potential in oligotrophic lakes here (), but this might be due to the relatively small sample size (n = 7) and again requires further investigation. The same algorithm performed poorly when all four lakes with different trophic status were combined.
Figure 6. Scatterplots of empirical chlorophyll-a algorithms that showed strong correlation with field data in Lakes Vättern and Vänern when both Terra and Aqua MODIS data (a) and only Terra MODIS data (b, c) were used, and in Lakes Geneva, Balaton, Vättern, and Vättern when only Terra MODIS data were used (d–f).
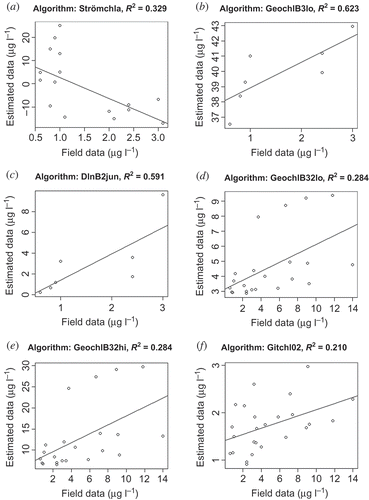
5.2. Empirical SDD estimation algorithms
When only Terra MODIS data were used to retrieve SDD, most algorithms exhibited promising results in Lake Geneva, but only one algorithm (‘BSecB1’) also showed strong correlation with field measurements in Lakes Vättern and Vänern (). When all three lakes were combined, all algorithm outputs exhibited weak correlations with field data. The two algorithms by Dekker and Peters (Citation1993), ‘DSecB3jul’ and ‘DlnSecB2jul’, were developed for lakes with very low SDD (<2 m), but exhibited promising results (r ≥ 0.648; ρ ≥ 0.750, n = 12) in Lake Geneva, which in this case showed an annual mean SDD of 7.6 m (1984–2004). When only Aqua MODIS data were used (), ‘DSecB3jul’ was also strongly correlated with field data from Lake Vättern (annual mean SDD is 10.9 m in 1969–2003) and Lake Vänern (annual mean SDD is 3.9 m in 1973–2008). No algorithm exhibited significant results in Lake Geneva, or when all lakes were combined, when only Aqua MODIS data were used. On the other hand, all algorithms showed strong negative correlations to field measurements in Lakes Vättern and Vänern, but the results were poor when all three lakes were combined. Finally, when both Terra and Aqua MODIS data were used, all algorithms exhibited low correlation coefficients in all cases ().
Table 10. Correlation of field SDD data with corresponding simulated remotely sensed data for empirical algorithms in 2001–2004 in Lakes Geneva, Vättern, and Vänern.
Table 11. Accuracy measures of empirical chlorophyll-a and SDD algorithms, the outputs of which showed strong correlation with field data in Lakes Geneva, Balaton, Vättern, and Vättern.
The R2, RMSE, and bias of the most promising SDD estimation algorithms are presented in . ‘ASecFeb’ exhibited high coefficients of determination (R2 ≥ 0.58) but highly unrealistic (negative) results for both Terra and Aqua MODIS data ( and (d)). The ‘BSecB1’ algorithm produced higher coefficients of determination in the oligotrophic lakes ( and ) than in the mesotrophic lake ((c)) but underestimated SDD in the all three cases. ‘DSecB3jul’ exhibited high coefficients of determination in Lake Geneva when Terra MODIS data were used (R2 = 0.62) () and in Lakes Vättern and Vänern when Aqua MODIS data were used (R2 = 0.71) (), but produced some unrealistic values (i.e. negative) in the second case. The other SDD estimation algorithm by Dekker and Peters (Citation1993), ‘DlnSecB2jul’, produced a very narrow range of outputs and moderate R2 with high degree of scatter (). Finally, the ‘SlnSDTB13’ algorithm produced promising results (R2 = 0.76) in the two oligotrophic lakes when Aqua MODIS data were used ((e)), but when all three lakes were combined for either sensor the results were poor.
6. Discrepancy between the outputs of empirical algorithms
The discrepancy observed during analysis between algorithm outputs on same dates when both Terra and Aqua MODIS data were used was investigated. The data sets were not normally distributed, so only non-parametric tests were applied. The correlation between Terra MODIS and Aqua MODIS chlorophyll-a estimates was moderate on the same dates (ρ = 0.423; p ≪ 0.01 (two-tailed), n = 68), whilst the correlation was stronger for SDD (ρ = 0.769; p ≪ 0.01 (two-tailed), n = 24). These results suggest a certain degree of disagreement between the Terra and Aqua MODIS data (Ltoa, Rtoa, nLw, and Rrs) that were used as inputs to the empirical algorithms. The MODIS sensors on board Terra and Aqua satellites were designed to the same specifications and essentially receive the same calibration, so that the two sensors should provide highly correlated data. However, the quality of Terra MODIS data has since been established to be insufficient for quantitative analysis due to a fault in the scanning mirror of the sensor (NASA Ocean Color Citation2009). Additionally, the radiometric degradation of the sensor since launch can be up to 40% in certain visible bands (Franz et al. Citation2007). Wu, Xiong, and Cao (Citation2008) have tested and confirmed the calibration consistency of three reflective solar Terra and Aqua MODIS wavebands, suggesting a high agreement between the two sensors in the visible, NIR, and mid-IR parts of the spectrum. However, these comparisons employed non-atmospherically corrected data and ocean colour is only a very small fraction of that signal. Also, due to their different overpass time (Aqua follows Terra by approximately 3 hours), differences in the atmospheric conditions and the Sun angle may result in differences in the signal detected by the two sensors (Lillesand, Kiefer, and Chipman Citation2008). Nevertheless, this discrepancy is an important issue and should be taken into consideration in studies where both satellite instruments are used interchangeably.
7. Discussion and conclusions
This study followed a meta-analysis approach, where the performance and interoperability of multiple chlorophyll-a and SDD retrieval algorithms were tested using Terra/Aqua MODIS and in situ data. We reviewed the literature and selected published algorithms that have previously shown potential in single or groups of similar lakes, and for specific dates or seasons. The MODIS data were used to simulate other sensors used to derive water quality algorithms and the suitability of each algorithm for further analysis was tested. This resulted in the testing of 27 empirical algorithms plus the operational MODIS OC3, which were applied to simulated remote-sensing data to assess their repeatability and transferability in the search for a single universal or a suite of algorithms that can be applied across wide spatial and temporal scales to retrieve water quality estimates.
None of the algorithms assessed in this study exhibited promise for estimating chlorophyll-a or SDD when all four lakes were combined into a single data set. Indeed most algorithms, including MODIS OC3, performed poorly even for specific lake types (i.e. oligotrophic and mesotrophic lakes). The results also varied depending on the sensor used. Algorithms that performed well for Terra MODIS data did not show the same behaviour for the Aqua MODIS data set and vice versa. For chlorophyll-a only the Terra MODIS data set produced some promising results, whilst for SDD there was one algorithm that seemed to work well for Terra MODIS and one for the Aqua MODIS data set. It is possible that the generally poor results exhibited here were caused by the inflexibility of empirical algorithms, which are generally trained with data from optically similar lakes and within the same scene, date, or season. Another cause could be that we used MODIS data to simulate other multispectral and hyperspectral sensors, which might have introduced uncertainty to our analysis. In addition, we used field measurements from different sources; the variety of sampling techniques and sampling depths could have also introduced uncertainty to our correlation analysis and accuracy assessment.
Despite the above, certain algorithms showed potential for specific lake types with particular success of chlorophyll-a retrieval algorithms in oligotrophic lakes and of SDD retrieval algorithms in mesotrophic lakes. Originally developed for eutrophic lakes, an algorithm by Dekker and Peters (Citation1993) showed promising results (R2 = 0.59, n = 7, bias = 1.3 µg l–1) in oligotrophic lakes, but because of the small sample size additional data are required to confirm the performance observed here. Four other chlorophyll-a retrieval algorithms (Dekker and Peters Citation1993; George Citation1997) showed potential too, but calibration is needed with additional data to improve their very high bias ranging from 38.3 µg l–1 (‘GeochlB3lo’) up to 2628.2 µg l–1 (‘DlnB2jul’). No other algorithms exhibited promising results in this study for either Terra or Aqua MODIS data. In the case of SDD, two algorithms showed potential applicability to other sites. First, an algorithm by Dekker and Peters (Citation1993), ‘DSecB3jul’, originally developed for turbid lakes (SDD < 2 m), exhibited promising results (R2 = 0.62, n = 12, bias = −0.6 m) in less turbid Lake Geneva with an annual mean SDD of 7.6 m. The algorithm by Sawaya et al. (Citation2003) was developed for a group of lakes with various characteristics, but performed well here only in oligotrophic lakes (R2 = 0.76, n = 9, bias = −1.8 m).
Apart from spatial transferability, the above-mentioned algorithms also showed temporal repeatability. All empirical algorithms assessed here were developed for specific months or seasons, whilst the data used for their testing covered longer periods throughout the year. However, additional match-up pairs should be used to explore these results further. This means that more than a 4-year period is required to compensate for frequent cloud cover over European lakes and the infrequency of in situ measurements that prohibited larger sample sizes (i.e. more match-up pairs) in this study.
Even though we tested numerous algorithms available in the published literature, our list was not exhaustive because not all algorithms are published fully, or others have been published since our analysis took place. For example, lake water retrieval algorithms have recently been developed specifically for MODIS data (e.g. McCullough, Loftin, and Sader Citation2012; Wang et al. Citation2012), although the spatial transferability and temporal repeatability of these also require testing. Nevertheless, this study clearly demonstrates the challenge associated with the reliable estimation of lake water quality parameters, and in particular chlorophyll-a and SDD, across time and space using empirical techniques. The results suggest that the search for a single universal empirical algorithm that can be applied to various ecological types of lake waters is perhaps meaningless and efforts should focus on a suite of algorithms that are applicable to specific lake types. In fact, in large lakes with or without distinct basins, where strong spatial variability of parameters such as chlorophyll-a are observed, and thus optical complexity, the use of two or more different algorithms for the retrieval of optical properties might be the best solution, as Palmer et al. (Citation2014) have recently demonstrated in Lake Balaton. In that case, clustering of lakes based on their optical characteristics would be required before a suite of suitable lake-type-specific algorithms are applied. For example, Moore et al. (Citation2014) adapted an optical classification system in lake waters and applied different chlorophyll-a retrieval algorithms to each water type.
In terms of the method used, empirical algorithms might be too simple a case for resolving the complex inherent optical properties of lake waters across large spatial and temporal scales. Even though empirical algorithms work well in Case I oceanic waters, it is possible that different modelling approaches are more appropriate for Case II lake waters, such as multivariate techniques, artificial neural networks (ANNs), and spectral libraries. Multivariate techniques can be used for the estimation of chlorophyll-a concentrations incorporating information from other parameters that may influence the optical properties of water, such as the amount of total suspended matter and coloured dissolved organic matter (e.g. Vos and Rijkeboer Citation2000; Tyler et al. Citation2006). ANNs have been used to map water quality in Case II waters because they can model complex and nonlinear relationships between biophysical variables, and can incorporate otherwise unused information (e.g. all wavebands) (Buckton, O’mongain, and Danaher Citation1999; Mas and Flores Citation2008). Finally, the use of spectral libraries of inland waters with known properties could be applied to multispectral sensors (e.g. Terra/Aqua MODIS and Envisat MERIS) and thus provide a useful method for estimating water quality of optical parameters in lake waters (Kutser Citation2009).
In an era of a continuously increasing need for wide-scale lake water quality monitoring at national and international level, remote sensing is a useful tool with great potential in lake waters. This work demonstrates a clear role for remote sensing in estimating lake water quality parameters providing that issues with sensors and algorithm transferability can be addressed. The long archive of satellite data held by NEODAAS, coupled with future sensors such as Sentinel-3, will enable future work to monitor fragile lake ecosystems at better spatial and temporal resolutions than previously possible, providing insight into the ecological behaviour of lakes and the drivers of lake change. Efforts should focus on optically complex parameters, such as chlorophyll-a and SDD, in order to develop robust and transferable methods. Even though the development of a single universal algorithm that becomes operational might be impossible, there is a lot of potential in the production of algorithms dedicated to specific lake ‘types’ by incorporating valuable ecosystem behaviour information derived from lake typological analysis.
Disclosure statement
No potential conflict of interest was reported by the authors.
Acknowledgements
For their kind provision of field data sets, the authors would like to thank the following: Swedish Environmental Protection Agency, International Commission for the Protection of Lake Geneva (CIPEL), and Hungarian Ministry of Environmental Protection and Water Management and Ministry of Health. Access to the satellite data was granted by NERC (06/NEODAAS/14). The authors also acknowledge NEODAAS PML RSG and DSRS for the pre-processing of the satellite data and the cloud cover calculation, respectively. Finally, the authors would like to thank the anonymous reviewers for valuable comments and suggestions that improved the final version of the paper.
References
- Adrian, R., C. M. O’reilly, H. Zagarese, S. B. Baines, D. O. Hessen, W. Keller, D. M. Livingstone, R. Sommaruga, D. Straile, E. Van Donk, G. A. Weyhenmeyer, and M. Winder. 2009. “Lakes as Sentinels of Climate Change.” Limnology and Oceanography 54: 2283–2297.
- Allee, R. J., and J. E. Johnson. 1999. “Use of Satellite Imagery to Estimate Surface Chlorophyll a and Secchi Disc Depth of Bull Shoals Reservoir, Arkansas, USA.” International Journal of Remote Sensing 20: 1057–1072. doi:10.1080/014311699212849.
- Baban, S. M. J. 1993. “Detecting Water Quality Parameters in the Norfolk Broads, U.K., Using Landsat Imagery.” International Journal of Remote Sensing 14: 1247–1267. doi:10.1080/01431169308953955.
- Buckton, D., E. O’mongain, and S. Danaher. 1999. “The Use of Neural Networks for the Estimation of Oceanic Constituents Based on the MERIS Instrument.” International Journal of Remote Sensing 20: 1841–1851. doi:10.1080/014311699212515.
- Campbell, J. W., and H. Feng. 2005. “The Empirical Chlorophyll Algorithm for MODIS: Testing the OC3M Algorithm Using NOMAD Data.” In Ocean Colour Bio-optical Algorithm Mini Workshop, September 27–29, New England Centre, University of New Hampshire, Durham, NH, 1–9. Durham, NH: NASA.
- Chavula, G., P. Brezonik, P. Thenkabail, T. Johnson, and M. Bauer. 2009. “Estimating Chlorophyll Concentration in Lake Malawi from MODIS Satellite Imagery.” Physics and Chemistry of the Earth, Parts A/B/C 34: 755–760. doi:10.1016/j.pce.2009.07.015.
- Dall’olmo, G., A. A. Gitelson, D. C. Rundquist, B. Leavitt, T. Barrow, and J. C. Holz. 2005. “Assessing the Potential of Seawifs and MODIS for Estimating Chlorophyll Concentration in Turbid Productive Waters Using Red and Near-Infrared Bands.” Remote Sensing of Environment 96: 176–187. doi:10.1016/j.rse.2005.02.007.
- Dekker, A. G., and S. W. M. Peters. 1993. “The Use of the Thematic Mapper for the Analysis of Eutrophic Lakes: A Case Study in the Netherlands.” International Journal of Remote Sensing 14: 799–821. doi:10.1080/01431169308904379.
- Franz, B. A., E. J. Kwiatkowska, G. Meister, and C. R. McClain. 2007. “Utility of MODIS-Terra for Ocean Colour Applications.” http://oceancolor.gsfc.nasa.gov/DOCS/Publications/franz_et_al_2007_spie.pdf
- George, D. G. 1997. “The Airborne Remote Sensing of Phytoplankton Chlorophyll in the Lakes and Tarns of the English Lake District.” International Journal of Remote Sensing 18: 1961–1975. doi:10.1080/014311697217972.
- Gerten, D., and R. Adrian. 2002. “Species-Specific Changes in the Phenology and Peak Abundance of Freshwater Copepods in Response to Warm Summers.” Freshwater Biology 47: 2163–2173. doi:10.1046/j.1365-2427.2002.00970.x.
- Gitelson, A., G. Garbuzov, F. Szilagyi, K.-H. Mittenzwey, A. Karnieli, and A. Kaiser. 1993. “Quantitative Remote Sensing Methods for Real-Time Monitoring of Inland Waters Quality.” International Journal of Remote Sensing 14: 1269–1295. doi:10.1080/01431169308953956.
- Hedger, R. D., P. M. Atkinson, T. J. Malthus, and D. G. George. 1996. “Planning Optimal Sampling Strategies for Estimating the Regional Mean Water Quality in Lakes.” In Proceedings of the 22nd Annual Conference of the Remote Sensing Society, September 11–14, 221–228. Nottingham: University of Durham.
- IOCCG (International Ocean Colour Coordinating Group). 2000. “Remote Sensing of Ocean Colour in Coastal, and Other Optically-Complex, Waters.” In Reports of the International Ocean-Colour Coordinating Group, No. 3, edited by S. Sathyendranath, 1–140. Dartmouth, NS: IOCCG.
- IOCCG (International Ocean Colour Coordinating Group). 2006. “Remote Sensing of Inherent Optical Properties: Fundamentals, Tests of Algorithms, and Applications.” In Reports of the International Ocean-Colour Coordinating Group, No. 5, edited by Z.-P. Lee, 1–126. Dartmouth, NS: IOCCG.
- Kallio, K., S. Koponen, and J. Pulliainen. 2003. “Feasibility of Airborne Imaging Spectrometry for Lake Monitoring – A Case Study of Spatial Chlorophyll-a Distribution in Two Meso-Eutrophic Lakes.” International Journal of Remote Sensing 24: 3771–3790.
- Kallio, K., T. Kutser, T. Hannonen, S. Koponen, J. Pulliainen, J. Vepsäläinen, and T. Pyhälahti. 2001. “Retrieval of Water Quality from Airborne Imaging Spectrometry of Various Lake Types in Different Seasons.” The Science of the Total Environment 268: 59–77. doi:10.1016/S0048-9697(00)00685-9.
- Kinnear, P. R., and C. D. Gray. 2008. SPSS 15 Made Simple. New York: Psychology Press, Taylor and Francis Group.
- Kloiber, S. M., P. L. Brezonik, and M. E. Bauer. 2002. “Application of Landsat Imagery to Regional-Scale Assessments of Lake Clarity.” Water Research 36: 4330–4340. doi:10.1016/S0043-1354(02)00146-X.
- Kloiber, S. M., P. L. Brezonik, L. G. Olmanson, and M. E. Bauer. 2002. “A Procedure for Regional Lake Water Clarity Assessment Using Landsat Multispectral Data.” Remote Sensing of Environment 82: 38–47. doi:10.1016/S0034-4257(02)00022-6.
- Koponen, S., J. Poulliainen, K. Kallio, and M. Hallikainen. 2002. “Lake Water Quality Classification with Airborne Hyperspectral Spectrometer and Simulated MERIS Data.” Remote Sensing of Environment 79: 51–59. doi:10.1016/S0034-4257(01)00238-3.
- Kutser, T. 2009. “Passive Optical Remote Sensing of Cyanobacteria and Other Intense Phytoplankton Blooms in Coastal and Inland Waters.” International Journal of Remote Sensing 30: 4401–4425. doi:10.1080/01431160802562305.
- Lavender, S. J., M. H. Pinkerton, J.-M. Froidefond, J. Morales, J. Aiken, and G. F. Moore. 2004. “Seawifs Validation in European Coastal Waters Using Optical and Bio-Geochemical Measurements.” International Journal of Remote Sensing 25: 1481–1488. doi:10.1080/01431160310001592481.
- Lillesand, T. M., R. W. Kiefer, and J. W. Chipman. 2008. Remote Sensing and Scene Interpretation. 6th. Hoboken, NJ: Wiley.
- Livingstone, D. M., and J. Padisák. 2007. “Large-Scale Coherence in the Response of Lake Surface-Water Temperatures to Synoptic-Scale Climate Forcing during Summer.” Limnology and Oceanography 52: 896–902.
- Mas, J. F., and J. J. Flores. 2008. “The Application of Artificial Neural Networks to the Analysis of Remotely Sensed Data.” International Journal of Remote Sensing 29: 617–663. doi:10.1080/01431160701352154.
- Matthews, M. W. 2011. “A Current Review of Empirical Procedures of Remote Sensing in Inland and Near-Coastal Transitional Waters.” International Journal of Remote Sensing 32: 6855–6899. doi:10.1080/01431161.2010.512947.
- McCullough, I. M., C. S. Loftin, and S. A. Sader. 2012. “High-Frequency Remote Monitoring of Large Lakes with MODIS 500m Imagery.” Remote Sensing of Environment 124: 234–241. doi:10.1016/j.rse.2012.05.018.
- Moore, T. S., M. D. Dowell, S. Bradt, and A. Ruiz Verdu. 2014. “An Optical Water Type Framework for Selecting and Blending Retrievals from Bio-Optical Algorithms in Lakes and Coastal Waters.” Remote Sensing of Environment 143: 97–111. doi:10.1016/j.rse.2013.11.021.
- NASA Ocean Color. 2009. http://oceancolor.gsfc.nasa.gov/
- Olmanson, L. G., M. E. Bauer, and P. L. Brezonik. 2008. “A 20-Year Landsat Water Clarity Census of Minnesota’s 10,000 Lakes.” Remote Sensing of Environment 112: 4086–4097. doi:10.1016/j.rse.2007.12.013.
- Palmer, S. C. J., P. D. Hunter, T. Lankester, S. Hubbard, E. Spyrakos, A. N. Tyler, M. Présing, H. Horváth, A. Lamb, H. Balzter, and V. R. Tóth. 2014. “Validation of Envisat MERIS Algorithms for Chlorophyll Retrieval in a Large, Turbid and Optically Complex Shallow Lake.” Remote Sensing of Environment. doi:10.1016/j.rse.2014.07.024.
- Pulliainen, J., K. Kallio, K. Eloheimo, S. Koponen, H. Servomaa, T. Hannonen, S. Tauriainen, and M. Hallikainen. 2001. “A Semi-Operative Approach to Lake Water Quality Retrieval from Remote Sensing Data.” The Science of the Total Environment 268: 79–93. doi:10.1016/S0048-9697(00)00687-2.
- Sawaya, K. E., L. G. Olmanson, N. J. Heinert, P. L. Brezonik, and M. E. Bauer. 2003. “Extending Satellite Remote Sensing to Local Scales: Land and Water Resource Monitoring Using High – Resolution Imagery.” Remote Sensing of Environment 88: 144–156. doi:10.1016/j.rse.2003.04.006.
- Strömbeck, N., and D. C. Pierson. 2001. “The Effects of Variability in the Inherent Optical Properties on Estimations of Chlorophyll a by Remote Sensing in Swedish Freshwaters.” The Science of the Total Environment 268: 123–137. doi:10.1016/S0048-9697(00)00681-1.
- Thiemann, S., and H. Kaufmann. 2000. “Determination of Chlorophyll Content and Trophic State of Lakes Using Field Spectrometer and IRS-1C Satellite Data in the Mecklenburg Lake District, Germany.” Remote Sensing of Environment 73: 227–235. doi:10.1016/S0034-4257(00)00097-3.
- Tyler, A. N., E. Svab, T. Preston, M. Présing, and W. A. Kovács. 2006. “Remote Sensing of the Water Quality of Shallow Lakes: A Mixture Modelling Approach to Quantifying Phytoplankton in Water Characterized by High-Suspended Sediment.” International Journal of Remote Sensing 27: 1521–1537. doi:10.1080/01431160500419311.
- Verpoorter, C., T. Kutser, D. A. Seekell, and L. J. Tranvik. 2014. “A Global Inventory of Lakes Based on High-Resolution Satellite Imagery.” Geophysical Research Letters 41: 6396–6402. doi:10.1002/2014GL060641.
- Vos, R. J., J. H. M. Hakvoort, R. W. J. Jordans, and B. W. Ibelings. 2003. “Multiplatform Optical Monitoring of Eutrophication in Temporally and Spatially Variable Lakes.” The Science of Total Environment 312: 221–243.
- Vos, R. J., and M. Rijkeboer. 2000. “Monitoring of Algal Blooms in Case II Waters with Seawifs.” XV ocean optics conference, October 16–20, Monaco, paper no. 1094.
- Wang, M., C. J. Nim, S. Son, and W. Shi. 2012. “Characterization of Turbidity in Florida’s Lake Okeechobee and Caloosahatchee and St. Lucie Estuaries Using Modis-Aqua Measurements.” Water Research 46: 5410–5422. doi:10.1016/j.watres.2012.07.024.
- Wheeler, D., G. Shaw, and S. Barr. 2004. Statistical Techniques in Geographical Analysis. London: David Fulton.
- Williamson, C. E., J. E. Saros, W. F. Vincent, and J. P. Smol. 2009. “Lakes and Reservoirs as Sentinels, Integrators, and Regulators of Climate Change.” Limnology and Oceanography 54: 2273–2282. doi:10.4319/lo.2009.54.6_part_2.2273.
- Wu, A., X. Xiong, and C. Cao. 2008. “Terra and Aqua MODIS Inter-Comparison of Three Reflective Solar Bands Using AVHRR Onboard the NOAA-KLM Satellites.” International Journal of Remote Sensing 29: 1997–2010. doi:10.1080/01431160701355272.
- Wu, G. G., J. De Leeuw, A. K. Skidmore, H. H. T. Prins, and Y. Liu. 2008. “Comparison of MODIS and Landsat TM5 Images for Mapping Tempo-Spatial Dynamics of Secchi Disk Depths in Poyang Lake National Nature Reserve, China.” International Journal of Remote Sensing 29: 2183–2198. doi:10.1080/01431160701422254.
- Wu, M., W. Zhang, X. Wang, and D. Luo. 2009. “Application of MODIS Satellite Data in Monitoring Water Quality Parameters of Chaohu Lake in China.” Environmental Monitoring and Assessment 148: 255–264. doi:10.1007/s10661-008-0156-2.
- Zhang, Y., S. Lin, J. Liu, X. Qian, and Y. Ge. 2010. “Time-Series MODIS Image-Based Retrieval and Distribution Analysis of Total Suspended Matter Concentrations in Lake Taihu (China).” International Journal of Environmental Research and Public Health 7: 3545–3560. doi:10.3390/ijerph7093545.