ABSTRACT
Although English-medium instruction (EMI) has been implemented and developed rapidly in higher education, little is known about its learning process and effects. This study employed a systematic approach to explore how diverse factors affect student performance in English and disciplinary learning. We conducted a survey to collect students’ self-report of their learning in the EMI programmes and used partial least square structural equation modelling in evaluation. The findings demonstrate three personal factors (prior knowledge, effort, and interest) and three environmental factors (course, teacher, and resource) have effects on student performance. We find that effort has the strongest direct impact on learning outcomes, prior knowledge the second, while course factor the least. Interest has an indirect effect on outcomes through the mediating effect of effort, and teacher and resource factors have indirect effects on outcomes mediated by course. In addition, prior knowledge has a larger impact on English performance than on subject achievement. The present study theoretically and methodologically contributes to the research field of EMI by systematically examining the learning process of EMI and constructing a structural model of complex relationships of multiple factors affecting student performance in EMI programmes. This study also provides implications for EMI practice.
Introduction
English as the global lingua franca has been a medium of instruction for subjects in the school curriculum among many non-English speaking countries for the purpose of integrating language learning with subject learning (Dimova and Kling Citation2018; Hodara Citation2015; Johnson Citation2020; Nicholls Citation2020). The spread of English-medium instruction (EMI) programmes from European countries to developing countries in Latin America and Asia, particularly in the higher education sector (Breeze and Dafouz Citation2017; Byun et al. Citation2011; Coleman Citation2006; Poole Citation2013), has been driven by several forces. One aim of adopting EMI in higher education is to internationalise universities, through which more international students or teacher resources may be attracted to improve the diversity and influence of universities (Wilkinson Citation2013). This approach to education promotes more and easier access to cutting-edge knowledge in various disciplines, fields of studies, and international academia, which is regarded by some governments as a way to further facilitate the scientific, economic, and social development of a country (Hu Citation2007). The adoption of EMI may also aim to help create more revenue, improve university rankings, and enhance students’ overall competitiveness and employability in the global marketplace (Coleman Citation2006; Pecorari et al. Citation2011).
Against this backdrop, China's higher education has aimed to enhance the quality of higher education and cultivate high-end bilingual talent to boost China's development and confront future international challenges (Liu Citation2020). At the turn of this century, China's Ministry of Education promulgated 12 policy initiatives as crucial steps to advance the quality and development of higher education, among which EMI was a key component. EMI has been implemented as an effective solution that integrates the teaching of disciplinary knowledge with enhancing English proficiency by way of increasing exposure to English as a language of instruction. Consequently, Chinese universities have started to follow this policy initiative and EMI has seen rapid development and wide implementation in China over the past decade.
While the existing literature on EMI has focused on research in European contexts (Coleman Citation2006; Dalton-Puffer Citation2011), as this educational approach has been prevalent in European countries for a relatively long time, few empirical studies have been conducted in the context of developing countries including China which are newcomers to EMI (Hu Citation2008; Hu and McKay Citation2012; Piller and Cho Citation2013). Thus, empirical studies in this respect should be conducted in non-European countries. Indeed, despite the spread of EMI programmes in Chinese universities, there is still a paucity of relevant research into EMI in the country (Hu, Li, and Lei Citation2014; Tong and Shi Citation2012; Yang Citation2015). The existing research about EMI in China's higher education mainly focuses on theoretical discussions, and not much appears strictly acquisition-based, lacking topics of the actual practice and effects of EMI in China, which should be an important dimension of EMI (Hu, Li, and Lei Citation2014; Tong and Shi Citation2012).
In this sense, this study aims to address the research gap by conducting an empirical evaluation to investigate the student learning process in EMI programmes. Specifically, the research question to be answered is: How do diverse factors influence student performance in language and discipline learning? A survey research was conducted to collect data in this study, and data were analysed by using quantitative methods and multivariate analysis based on the partial least square structural equation modelling (PLS-SEM) approach. To answer the research question, a structural model of the effects of diverse factors on student performance was constructed.
The present study also aims to make research methodological contributions to the field of EMI by using the PLS-SEM method to construct a structural model of the complex relationships of multiple factors that affect student performance in EMI programmes. As little research has previously been conducted to systematically examine the learning process of EMI, this technique for multivariate analysis has rarely been employed previously to explore these issues. This statistical method provides insights for analysing the effects of various personal and environmental variables involved in the learning process, how they are interrelated, and which effect is stronger than others. Based on the research results, some pedagogical implications regarding EMI practice are further discussed in later sections.
Background and literature
English-medium instruction (EMI) generally refers to the use of English as an instructional language for teaching disciplinary knowledge to students who either speak English as a lingua franca or learn English as a second language. In the former situation, English mainly works as a tool for communication, while in the latter situation it is the focus of the study, mainly focusing on EMI as a means of learning both language and disciplinary knowledge simultaneously. In addition to EMI, there are also many other forms of instruction with similar ideas and approaches, such as Content and Language Integrated Learning (CLIL), and Integrating Content and Language (ICL), which use a target language to teach the subject content (Dalton-Puffer Citation2011; Smit and Dafouz Citation2012). In EMI, the target language is English.
In China, EMI policy has been closely connected with the government's objectives to internationalise the curricula and improve the quality of education in the country (Huang Citation2006). EMI courses have been widely implemented at all levels of education and particularly in universities across China for nearly two decades with a dual objective of improving students’ subject knowledge and English proficiency (Zhang and Pladevall-Ballester Citation2021). The popularity of EMI in tertiary level education has caused some researchers to examine its effectiveness in achieving the course goals (Lei and Hu Citation2014), questioning the quality of the faculty who are teaching academic subjects in English (Hu Citation2007) and investigating the actual use of English language due to different levels of English proficiency of teachers and students (Pan Citation2007). Trans-languaging practices in EMI classrooms in Chinese universities, as observed by Wang and Curdt-Christiansen, indicate a special characteristic that EMI courses in China should be ‘reconceptualised’ (Gao and Ren Citation2019) towards ‘transitional bilingual instruction’ in which students receive English instruction in most curriculum time to achieve ‘limited biliteracy’ and ‘limited cultural enrichment’ (Hu Citation2008; Zhou, Li, and Gao Citation2021).
The existing literature shows that there are diverse and complex findings relating to learning outcomes and effects of EMI (Costa and Coleman Citation2013; Tong and Shi Citation2012; Wilkinson Citation2013). As for linguistic gains, students are expected to improve their discipline-specific language ability for better content comprehension and general language proficiency for classroom interaction (Zhang and Pladevall-Ballester Citation2021). Consequently, the variable of English competence has an influence on classroom English use and interaction, which may either promote or inhibit English improvement and the learning of disciplinary knowledge according to the English level of students and teachers. In terms of EMI's effect on language learning, many studies have reported positive results and suggested several reasons for such beneficial effects (Hu and Wu Citation2020; Jiang and Zhang Citation2019; Sahan and Sahan Citation2021; Xie and Curle Citation2022). One possible reason is that as EMI uses English as the teaching language, the classroom naturally provides a favourable learning environment in which students’ exposure to the English language increases (Pecorari et al. Citation2011; van der Worp Citation2017). Another possible reason is that students have more opportunities to apply English in natural and academic settings with English as the tool of communication and interaction between teachers and students. For EMI's effect on subject learning, many studies have shown that EMI does not lower students’ academic achievements (Dafouz and Camacho-Miñano Citation2016; Dafouz, Camacho, and Urquia Citation2014) but instead has a positive influence on the learning of disciplinary knowledge, and that students and teachers hold positive attitudes and perceptions of EMI (Aguilar and Rodríguez Citation2012; Ball and Lindsay Citation2013; Costa and Coleman Citation2013).
On the other hand, negative results have also been found in empirical studies about EMI. Although some research has shown that a great number of faculty and students regard their English proficiency as sufficient and adequate for EMI, other studies document that inadequate English proficiency becomes a barrier to effective teaching and learning in EMI classes. Students may have difficulty comprehending lectures and require more time to prepare for and complete courses and tasks. This also brings more pressure and extra work to teachers (Tong and Shi Citation2012; Wilkinson Citation2013). As a result, the use of English for interaction is reduced while the student's first language is used more frequently in the classroom. Meanwhile, disciplinary knowledge learning also seems to be negatively affected when teachers adopt certain strategies to lower the level of difficulty of subject content (Beckett and Li Citation2012). In contrast to the positive attitudes towards EMI in the studies mentioned above, other studies have found that resistance to EMI also exists among many students and teachers (Doiz, Lasagabaster, and Sierra Citation2013; Tange Citation2012). Furthermore, some studies have even suggested that EMI leads to educational inequalities, as it tends to benefit some institutions and elite individuals more than others that can access better resources for becoming winners in this English race (Costa and Coleman Citation2013; Piller and Cho Citation2013; Wilkinson Citation2013). This is more related to socio-economic inequalities in terms of resources available in different types of universities and for students with different socioeconomic statuses.
As can be seen from the above, mixed findings are reported in the existing literature, and several factors have been identified that exert an influence on the process and outcomes of EMI. All the studies demonstrate that the complex process of EMI learning involves many factors, while few studies have examined how a combination of diverse factors interplay and affect student learning (Hu, Li, and Lei Citation2014). Thus, more research must be conducted to further investigate the process of EMI, and to learn about how various factors interact to bring about its outcomes and effects.
Research question, model and conceptual framework
To better deconstruct the effects of EMI on student English and disciplinary learning outcomes, this study empirically investigates the actual complex process of student learning in EMI programmes in the context of Chinese higher education, by exploring how diverse factors affect student performance in these programmes. We present the research question as follows:
How do diverse factors (both personal and environmental factors) influence student performance in EMI programmes in Chinese universities?
To examine the relationships, this section firstly identifies the variables involved in the learning process and their outcomes, including the factors that have an influence and their respective outcomes.
This empirical study includes two categories of influencing factors, (1) personal and (2) environmental (Athey Citation1983), and examines how these factors influence student learning in EMI and bilingual programmes.
Within the category of personal factors, three factors are taken into consideration to establish the conceptual model in this study, including prior knowledge, effort, and interest. These three factors were chosen because they are widely used in the literature on learning outcomes (Alexander and Murphy Citation1998; Schiefele Citation1991). i. Prior knowledge is identified as a key acquired factor (Cummins Citation2008). As suggested in some previous research, prior foundation preceding later study may exert a relatively large impact on performance and outcomes (Simonsmeier et al. Citation2022; Thompson and Zamboanga Citation2003). As an EMI programme is an integrated learning process of English and academic subject, prior knowledge in this study contains two aspects, prior knowledge in English, and prior knowledge in subject. ii. Effort. During the learning process, learner effort may determine how well the learner acquires knowledge and skills and their subsequent performance to a large extent. Knowledge in the Chinese EMI programmes included in this study mainly refers to domain-specific information stored in learners’ memory, such as concepts, relational facts and problem-solving skills. Students who attend EMI courses must focus on learning both English and subject, so the factor of effort in the study contains effort made for learning English, and effort made in learning subject knowledge (Doumen, Broeckmans, and Masui Citation2014; Masui et al. Citation2014). Subjects could refer to a range of subjects that students major in, ranging from Humanities and Social Sciences, Business and Management, Science and Engineering, to Medicine. English serves as a carrier of the subject knowledge and is used as the medium of instruction for the subject courses. iii. Interest. Apart from making an effort, interest is usually regarded as an important factor that acts as a driving force for learners to make continuous effort in their studies (Lee Citation2002; Robinson, Gerace, and Mestre Citation1980). When learners feel interested to learn, better results may be yielded. Interest is defined as a personal psychological state in which an increased commitment to learning arises (Harackiewicz, Smith, and Priniski Citation2016). Similarly, the factor of interest includes interest in learning English and interest in subject knowledge.
Besides personal factors, environmental factors are also crucial, as learning does not only involve learners, but also influences from the external environment (Gilmore et al. Citation2015). Within the category of environmental factors, there are also three factors that will be included in the model of this study, which are course, teacher and resource. i. For any educational programme, course design and quality can be important factors that affect learning outcomes (Darling-Hammond Citation2006; Ramsden Citation1991; Sayed and Ahmed Citation2015). In EMI programmes, course is the key component that delivers the learning content in English language for students. Thus, whether and how effective the course design is may affect the input for students, so it is vital to investigate this factor in two aspects in regard to subject content and English use. ii. In addition to course design, the teacher plays a central role in delivering course content and determining course quality, and hence teacher's capacity and quality is another factor that exerts an impact upon learning, specifically the level of teacher's knowledge and their lecturing skills. When delivering EMI courses, teachers not only need a good level of knowledge of the academic subject, but also a good command of English, as well as a good level of lecturing skills (Duong and Chua Citation2016; Hou et al. Citation2013). Therefore, teacher factor contains the three aspects mentioned above. iii. Furthermore, resource is an additional factor that contributes to course quality (Temple and Reynolds Citation2007). Learning resource could refer to the relevant study materials, books, audio or video databases, and equipment for learning. If the learning resource is richer for the course and in the institution, the course can be enriched with better content and a more scientific and reasonable design to enhance course quality. In EMI programmes, resources for both English learning and disciplinary learning are important and may make a difference to the course content and the learning outcomes.
After identifying the factors affecting the learning process and outcomes, the variables of student performance are clarified (more details on data collection are revealed in Section 4). The success of EMI programmes is dependent upon outcomes in two main areas of student learning: acquisition of English and acquisition of subject knowledge. (1) For English performance, three features are taken into consideration. The first feature is English proficiency level. The second feature is confidence in English, reflecting how well the learners perceive their English ability. The third feature is the ability in using English, reflecting students’ ability in the general use of English not restrictive to their subject learning (Tong and Shi Citation2012; Wilkinson Citation2013). Consequently, the variable of English competence has an influence on English use and interaction in the classroom, which may either promote or inhibit English improvement and the learning of disciplinary knowledge according to the English level of students and teachers. (2) In terms of subject knowledge, four features are considered in this study (Kim, Kweon, and Kim Citation2017; Kym and Kym Citation2014). The first feature is student performance in courses of their programmes, which directly indicates their command of the subject knowledge upon completing the courses. The second feature is their performance in academic/professional competitions in the subject area. Student performance in competitions is singled out to examine how well respondents command and are able to apply disciplinary knowledge in competitions in their own study fields. As college students may not only learn disciplinary knowledge in their courses, but also apply knowledge in various academic-related activities, student performance in competitions could to some extent reflect how well they command and are able to apply disciplinary knowledge. The third feature of subject knowledge is academic research ability in the subject area. In addition to learning in the class and participating in academic activities, academic skills in researching the subject area is another indicator that demonstrates a learner's level of disciplinary knowledge. The fourth feature is the ability to apply subject knowledge in practice, which is also a key indicator of achievement in subject learning and one of the most important objectives of undergraduate programmes.
Based on previous identification, clarification, and discussion of all variables involved in the learning process, six factors are considered to interplay and influence student performance in both English and subject knowledge. lists the eight variables and their corresponding indicators (with respective notations).
Table 1. Latent variables and measurement indicators in the model.
This study constructs an exploratory conceptual model of the learning process in EMI programme, as shown in . Altogether there are eight latent variables in the structural model that establish interrelationships, including (1) prior knowledge, (2) effort, (3) interest, (4) course, (5) teacher, (6) resource, (7) English, and (8) subject.
The hypotheses constructed in our models are as follows.
H1a: A student's prior knowledge would directly predict his or her performance in English.
H1b: A student's prior knowledge would directly predict his or her performance in subject.
H2a: A student’s effort would directly predict his or her performance in English.
H2b: A student’s effort would directly predict his or her performance in subject.
H3: A student’s interest would directly predict his or her effort, and thus indirectly predict his or her performance in English and subject.
H4a: Course quality would directly predict student performance in English.
H4b: Course quality would directly predict student performance in subject.
H5: Teacher quality would directly predict course quality, and thus indirectly predict student performance in English and subject.
H6: Resource would directly predict course quality, and thus indirectly predict student performance in English and subject.
Validating these hypotheses and obtaining the relationships among the factors could help us understand our fundamental research question that how different factors influence the student learning process and outcome in EMI courses, which adds new empirical evidence to the literature on EMI.
Methodology
Survey
In this study, we used questionnaire data collected from an online survey to examine the research question. Following the procedures of survey research (see ), the first step is to consider the target population and sampling methods to be used. In the present study, the population is learners who have experience with EMI programme in Chinese higher education, and sampling methods are convenience sampling and snowball sampling through web survey and online social networking platforms. The questionnaire is then designed and administered to the sample population. Data was then collected, processed, analysed, and interpreted.
Figure 2. Questionnaire design and data collection procedure Source: compiled by the author, reference: Bryman (Citation2012).
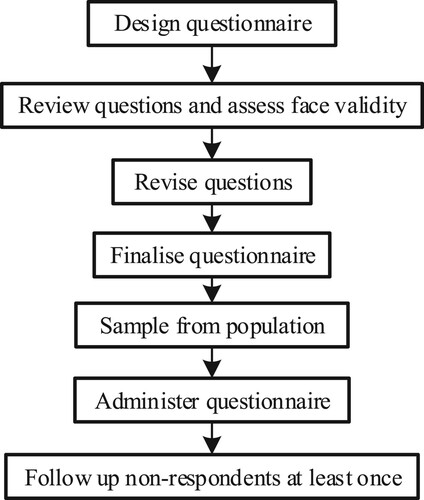
The structure of the survey is shown in below (Due to the limit of space, please refer to the Appendix for the questionnaire). At the very beginning of the web survey, the research purpose and process are explained to the participant. A question is also set in Part 0 of the questionnaire to ask for the participant's consent. To make sure the respondents are indeed EMI learners, a question is set in Part 1 of the questionnaire to screen the data to identify the right respondents in this survey.
Table 2. Structure of the survey.
Part 2 collects data on the multiple indicators for the model to answer the research question. The five-point Likert scale was used in the questionnaire design to collect data for the multiple indicators. The respondents’ response to each question item was scored, varying from 1 to 5. More specifically, students were required to self-report their evaluation of the indicators. For the variable Prior knowledge, participants were required to self-assess A1 their prior foundation in English and A2 subject area when entering the university; B1 their interest in learning English and B2 their interest in learning their subject, for the second latent variable Interest; C1 their time and effort devoted to learning English and C2 their time and effort devoted to learning subject, for the third latent factor Effort; D1 the level of English competency of the teachers teaching courses of their programme, D2 The knowledge level in the subject area of the teachers teaching courses of their programme, and D3 The lecturing skills of the teachers teaching courses of their programme, for the fourth latent variable Teacher; I1 The degree of reasonable design of your courses for subject study, and I2 The degree of reasonable English use in your class, for the fifth factor Course; F1 their resources and environment for learning the subject and F2 their resources and environment for learning English, for the sixth latent factor Resource; G1 their current level of English competency, G2 their current level of confidence in English, and G3 their current ability of using English, for the seventh latent variable of student performance in English; H1 their performance and results of subject major courses in their programme, H2 their performance in academic competitions in their subject area, H3. their academic ability in your subject area, and H4 their ability of applying subject knowledge in practice, for the eighth latent variable of student performance in Subject.
Apart from this main body of questions, participants’ demographic information is collected in Part 3, including sex, place of birth and whether in urban or rural areas, name of institution, major of study, and year of study. To ensure maximum variation in the sample, we tried to reach out to a wide range of people covering a diversity of majors of study, year groups, genders, and universities, by sending the questionnaire to diverse platforms.
The study aimed to collect at least 100 samples or at least 5 cases for each parameter, as previous researchers have suggested for the minimum sample size for SEM studies (Bentler and Chou Citation1987; Ding, Velicer, and Harlow Citation1995; Tabatchnick and Fidell Citation2001). For this study, 308 completed questionnaires were returned, with 100 percent of informed consent, as all respondents chose ‘Yes’ for the question of Part 0 in the survey. Among 308, 180 respondents chose ‘Yes’ for the question of Part 1 in the survey, which helped me to select and use data from the right participants who had attended the EMI courses. Thus, the valid questionnaire return rate was 58.44%.
From the responses in Part 3, the basic sample characteristics of EMI learners in this study are as follows. Among the EMI respondents, 30% are male (54), and 70% are female (126). They are from 141 districts in 88 cities of 25 provinces in China, covering 15% from rural areas (26), and 85% from urban areas (154), including 39% in provincial cities (70), 32% prefecture cities (58), and 14% county cities (26).
The respondents were from more than 20 universities, and the majors of study cover 27% Humanities and Social Sciences (48), 40% Business and Management (72), 21% Science and Engineering (38), and 12% Medicine (22). Year groups cover 22% of graduates (39) who have already finished their courses and left higher education, 34% of graduate students (61) who have completed their EMI undergraduate programmes and now in their further education, 44% of undergraduate students (80) who are attending EMI courses, including 12% first-year students (21), 4% second-year students (7), 8% third-year students (15), and 20% fourth-year students (37).
The sample distribution and characteristics indicate that the participants have diverse backgrounds, which basically satisfy the general requirement of data analysis.
Partial least squares (PLS) modelling
As the present study constructs a conceptual model of the hypothesised relationships among various factors that influence student learning in EMI programmes, complex statistical techniques are required to test the hypotheses and the model. Structural equation modelling (SEM) is a statistical method used in many studies that require multivariate analysis (Kim and Sohn Citation2009; Lin, Lam, and Tse Citation2019; Musah et al. Citation2015; Tai and Tang Citation2021). For instance, Kim and Sohn (Citation2009) and Tai and Tang (Citation2021) used SEM approach to study EMI in Korean universities and in a Taiwanese university respectively. SEM, specifically partial least square (PLS or PLS-SEM), was employed in this study to analyse the relationships among the variables, for the following reasons.
Firstly, PLS modelling is a statistical technique used for multivariate analysis in examining relationships among multiple independent and dependent variables, especially latent variables measured by indicators. This method has been adopted in many research fields of the social sciences, including education, and has been proved to be efficient in producing reliable results (Hair et al. Citation2016).
Secondly, PLS is appropriate and recommended for the purpose of testing and validating exploratory models at an early stage of theory development (Gerlach, Kowalski, and Wold Citation1979; Hair, Ringle, and Sarstedt Citation2012; Hair et al. Citation2019; Nitzl Citation2016). In the present study, the conceptual model is more exploratory than confirmatory, so PLS was employed to test the hypothesised relationships among the variables. PLS also has advantages such as a reduced demand on the sample size and it allows the use of reflective and formative measures.
The PLS model contains two parts: (1) inner model and (2) outer model. Inner model represents the relationships between multiple independent and dependent variables. In this study, the inner model is the conceptual model as previously illustrated. Outer model demonstrates how the latent variable is measured by directly observable indicators. In PLS, outer model can be reflective or formative. In reflective measurement model, the latent variable is reflected by the indicators; or, put another way, indicators are the effects of the latent variable. In contrast, in formative measurement model, the latent variable is formed by the indicators, each representing a dimension of the latent construct and together forming the overall meaning of the latent construct; in this study, the latent variable is the effect of the indicators. In the eight latent variables in the structural model, the first six variables (prior knowledge, effort, interest, course, teacher, resource) are modelled formatively, as their indicators represent different dimensions of the constructs and together form the overall meaning, while the latter two variables (Student Performance in English and subject) are modelled reflectively, as their indicators reflect the meaning of the constructs and are the effects of the variables. As inner model and outer model are constructed, the overall PLS model is illustrated in below. Formative models can be identified as the arrows going from the indicators to the corresponding variable, while reflective models are to the contrary. After constructing the model and collecting data, the PLS model can be calculated, estimated, and interpreted.
Analyses and results
In this study, PLS modelling was performed by employing SmartPLS to analyse the collected data. The key reason for adopting SmartPLS is its reliability in producing results through three steps. It at first assesses the outer measurement model, and when the measurement fit is acceptable, it starts to assess inner structural model before any conclusion about relationships among variables can be drawn (Garson Citation2014). This study used SmartPLS 3.2.4 to analyse the data collected by following five steps: creating a PLS project, importing data, drawing the path model, running the PLS algorithm and bootstrap estimates of significance, and assessing the model. When assessing the PLS model, two steps are required. First, assessing the outer measurement model to ensure its reliability and validity. Only when the measurement fit is acceptable, can the inner structural model be assessed, and the conclusions about relationships among variables could then be drawn (Garson Citation2014).
Assessing the outer measurement model: data reliability and validity
To test the reliability and validity of the multiple-indicator measures that represent the latent factors, several indices are provided in SmartPLS after calculation of the model to help researchers gauge whether the measurement model is appropriately designed and able to reflect the meaning of the construct. According to different types of measurement models, different types of model fit should be applied.
In this study, English and subject variables are constructed as reflective measurement models where indicators are the effects of the latent variable that they intend to measure. For a reflective model, the following set of criteria are appropriate to use to assess the measurement model fit, as can be seen from .
Table 3. Measurement fit for reflective models in this study.
Six factors are formatively modelled in this study. For a formative measurement model, the latent variable is the effect of its indicators, which represent different dimensions of the variable and may not be highly correlated. Thus, measurement fit used for assessing reflective models such as composite reliability and Cronbach's alpha is inappropriate. For formative models, the following criteria are utilised to assess their model fit, as shown in .
Table 4. Measurement fit for formative models in this study.
The results above demonstrate that all the indices used to assess the data reliability and model validity have met the assessment criteria, which suggests the good reliability and validity of indicators of the latent variables, and the established models.
Assessing the inner structural models
As an examination of goodness of fit for all outer measurement models shows good results, which reveal the suitability and strength of the models, goodness of fit for the structural model of the relationships of the variables can then be assessed. The following appropriate criteria are applied to evaluate the structural model fit, as shown in . The results demonstrate that all the indices used to assess the goodness of fit for the inner model have met the assessment criteria.
Table 5. Goodness of fit for the inner structural model in this study.
After assessing both the outer measurement model and the inner structural model, the results indicated that the model in the study is well-fitting, and the effects of diverse factors on student performance in EMI programmes are obtained.
The model of hypotheses established: direct, indirect, and total path coefficients
below shows 15 total effects, including 9 direct effects and 6 indirect effects. Therefore, all hypotheses raised are established and confirmed according to the estimation results of the structural model of the relationships of the variables (see ). Thus, the conceptual model is justified by the empirical evidence in this study (see ).
Table 6. Direct and indirect effects.
Table 7. Estimation results of the hypotheses in the study.
Discussion
Apart from the finding that the relationships among variables are significant and established, the path coefficients reveal that the strength of the relationships varied.
Firstly, prior knowledge has direct effects on student performance in both English and subject learning. The preceding literature has shown that prior knowledge indeed has an influence on learning English and disciplinary knowledge, such as that inadequate previous English proficiency would impede students’ interaction in English and comprehension of subject knowledge in EMI classes (Tong and Shi Citation2012; Wilkinson Citation2013). As an EMI programme is a form of integrated learning, prior disciplinary knowledge also plays a role in influencing student performance. What is noticeable in this study is that the path coefficients of the effects are different, with the effect on English relatively larger than that on subject. This may suggest that English language learning is a harder and more cumulative process than other disciplinary knowledge learning and may require a better previous foundation to achieve a higher level of proficiency in later stages of study.
Secondly, the factor of effort also has a direct effect on both English and subject performance, while the former is smaller than the latter, which echoes the above finding. As prior knowledge in subject learning does not have a big impact on subject performance, the more effort made in studying subject knowledge during the learning process, the better performance the student is more likely to make. On the other hand, although effort has an impact on English performance, the impact is not as strong as that on subject, as prior knowledge to some degree also plays a determining role. This may also suggest that when students put effort into learning English, both English and subject learning may be improved, as subject knowledge is conveyed in English and a stronger competence in English could help subject learning. However, effort made for subject learning may have a weaker effect on performance in English.
Thirdly, the findings also indicate that interest has a strong impact on effort. Thus, interest acts as a driving force that motivates students to make more effort in their studies and thus achieve better results. The indirect effects of the factor of interest demonstrate its impact on English and subject through the mediating effect of effort.
Fourthly, course also has direct effects on both English and subject learning, with the former relatively smaller than the latter. As EMI courses deliver the subject content in English, whether the English curriculum used in classrooms is suitable for students is crucial for their learning and understanding of subject knowledge as well as for their acquisition of English as a language to interact with their teachers and peers and comprehend subject knowledge. The design of subject content is another important element for course quality, and exerts much influence on the amount and quality of the subject knowledge the students receive and acquire, thus the subsequent achievement in subject learning. Achievement in English, however, may be influenced less by content design.
Fifthly, apart from the course itself, the teacher factor is key to the delivery of the course and course quality, and the study findings reveal that the teacher has a relatively strong influence on the course, and thus indirectly exerts an influence on student performance in English and subject learning.
Lastly, in addition to the teacher factor, resources and environment for student learning also contribute to course quality, as shown by the path coefficient of the direct effect of resource on the course. Indirectly, resource influences student performance mediated through the course factor.
To summarise the above findings, six factors influence student performance in English and subject either directly or indirectly. Among them, except prior knowledge, five factors, including (1) effort, (2) interest, (3) course, (4) teacher, and (5) resources, exert larger impacts on subject than on English. Meanwhile, to sort the effects of these factors on both English and subject in descending order, it is found that personal factors have a stronger impact on student learning than environmental factors. Among individual factors, effort has the strongest effect (see ).
Table 8. The strength of the effects of diverse factors on student performance.
From these findings, several implications can be drawn. From the perspective of personal factors, both prior knowledge and effort have direct effects on student performance. To ensure the quality outcomes of EMI programmes, both factors may need to be considered important elements. Prior knowledge may be taken as one criterion when universities enrol students into EMI programmes. For students, they may also need to consider whether their foundation is adequate and suitable to attend EMI courses. Although EMI may be regarded as a means to improve English proficiency, the English outcome of students in a programme is also more dependent upon their previous foundation. Although prior knowledge has an effect on outcomes, effort has an even greater impact. Furthermore, as a driving force, interest exerts an indirect influence on student learning. Therefore, when students choose whether or not to attend an EMI programme, they should consider the level of their interest in learning the subject as well as their need to improve their English. During the study process, they must devote more time and energy to learning.
From the perspective of environmental factors, it is interesting to find that the effects of course, teacher, and resource are smaller than those of the individual factors discussed above. Nevertheless, course still has a direct effect on both English and subject learning outcomes. This small impact may indicate that students are actually more dependent on self-study, and the contribution of courses is relatively less, which may further suggest that the quality of an EMI course still must be improved in order to play a bigger role in enhancing student learning. As teacher and resource have a strong direct influence on the course, teacher quality and resources should be improved to refine course design and quality, and thus enhance their indirect effect on student learning in EMI programmes.
Overall, it can be said that student effort plays the most important role in the learning process with interest being the motivator, and the direct effect of prior knowledge ranking second. Yet, surprisingly, the factor of course has the smallest direct effects on learning outcomes. Although it is reasonable to suggest that prior knowledge would have an influence on learning, and students should study and work hard to achieve better results, it is unexpected to find that the course does not contribute a great deal to learning outcomes. Thus, more progress still needs to be made in the development of EMI programmes. In addition, English achievement tends to be more dependent upon prior knowledge, while subject achievement relates more to the student's effort and course quality during the learning process.
Conclusion
Drawing on our data of respondents’ views, this study has systematically analysed the learning process and effect of EMI programmes in Chinese higher education, presented new findings, as well as utilised advanced statistical methods to conduct multivariate analysis on the evaluation.
In this study, a structural model was constructed and examined by using the PLS-SEM method. The results demonstrated that all six factors have direct or indirect effects on both English and subject achievement. Among all the direct effects of the factors in learning outcomes, student effort has the strongest direct impact on outcomes, prior knowledge the second, and course factor the least. The factor of interest has a direct effect on effort, and the factors of teacher and course have direct effects on the factor of course; these three factors exert indirect effects on both English and subject performance through their corresponding mediating factors, i.e. effort and course. Furthermore, among the six factors, prior knowledge has a larger impact on English performance than on subject achievement.
Based on these findings, we offer the following advice for enhancing future EMI practices and student learning outcomes. Before studying EMI, it is critical for teachers to assess prior knowledge and interest of students since they have been discovered to be the determinant factors in EMI thresholds (Aizawa et al. Citation2020). As discussed in the findings, prior knowledge directly predicts student performance in EMI programmes and has a larger impact on English performance. Student effort and interest also have a relatively strong impact on student achievement. Therefore, to yield better learning outcomes, students should decide whether to attend EMI programmes according to their prior foundation and their interests. Universities should also consider these two factors when admitting students into EMI programmes.
During EMI programme study, it is critical to increase effort, and enhance the quality of course, teachers, and resources. During the learning process in EMI programmes, effort plays a major role in affecting student performance. Course, teacher, and resource factors, though with a relatively small effect, have direct or indirect effects on student learning. Hence, to achieve better results in study, students should be more devoted to their learning. Although it is suggested that students may be more independent, and courses have not yet achieved the large impact as expected, students should not only focus on self-study but also attend the courses and make better use of the teachers and resources available for the course. Meanwhile, universities that offer EMI programmes should aim to enhance course quality, teacher quality, resources available and the environment, so as to play a more important role in enhancing student achievements in both English and subject study, through optimising EMI's values and providing students with better platforms for EMI learning.
Apart from contributions, the study has its limitations and cannot cover all the research issues, so implications for further research are also provided. First, it needs to be pointed out that the self-report nature of the data is a limitation of the research, as self-report of knowledge is not unbiased, even though it is argued that self-report results can ‘give a reasonably accurate representation of real-world performance’ (Schmitter-Edgecombe, Parsey, and Cook Citation2011). For example, regarding participants’ evaluation of teachers’ disciplinary knowledge, the research asks participants to evaluate the knowledge of their teachers based on their experiences of being taught and supervised by teachers. This evaluation of teachers’ disciplinary knowledge is subjective but is an important indicator reflecting teachers’ disciplinary knowledge. Teachers’ disciplinary knowledge, as well as other variables such as interest, effort and resource, is complex and hard to quantify (Even and Tirosh Citation1995; Zimmerman Citation2002). Standard methods such as tests are still not likely to produce objective results of these variables. Therefore, the study acknowledges the limitation of subjective self-evaluation of variables but deems it as important and valid indicators to reflect the reality (Steyn and Mynhardt Citation2008). In future studies, other measurements or methods could be employed to further verify these findings, such as using qualitative interviews, observations, and performance-based measures, and even longitudinal data. In the study, we take into consideration that the majority of the participants (over 75%) in the research already completed or near the completion of all their EMI undergraduate programmes, and this means that when they were completing the questionnaire, they were making evaluation retrospectively, which can to some extent reflect their evaluation of their ability and performance before and after the EMI courses. The study also conducts model fit tests and the results show it is good fit, addressing the concern of the interdependence between the variables. Nevertheless, if we had collected data in different stages of students’ learning, the results would have been more accurate. Thus, future studies could collect longitudinal data to enrich the research area. Future research on the development of EMI could also explore the questions of how different subjects studied by the learners may interact with English learning differently along the learning process, and how different institutions or other factors may result in the heterogeneity in terms of learning outcomes of EMI learners.
Acknowledgements
We would like to acknowledge Dr Michael Evans, Dr Pascual Pérez-Paredes, Professor Michelle Ellefson, Dr Denise Chappell, Professor Yongcan Liu, the anonymous reviewers, and the editors for their helpful comments on the drafts of the paper. We also thank all the participants who devoted their time to fill in the survey and colleagues who provided support along the research process.
Disclosure statement
No potential conflict of interest was reported by the author(s).
References
- Aguilar, M., and R. Rodríguez. 2012. “Lecturer and Student Perceptions on CLIL at a Spanish University.” International Journal of Bilingual Education and Bilingualism 15 (2): 183–197.
- Aizawa, I., H. Rose, G. Thompson, and S. Curle. 2020. “Beyond the Threshold: Exploring English Language Proficiency, Linguistic Challenges, and Academic Language Skills of Japanese Students in an English Medium Instruction Programme.” Language Teaching Research 0 (0): 1362168820965510.
- Alexander, P. A., and P. K. Murphy. 1998. “Profiling the Differences in Students’ Knowledge, Interest, and Strategic Processing.” Journal of Educational Psychology 90 (3): 435.
- Athey, I. 1983. “Future Research in English Education: Vital Factors and Research Paradigms.” English Education 15 (4): 217–230.
- Ball, P., and D. Lindsay. 2013. Language demands and support for English-medium instruction in tertiary education: Learning from a specific context. In A. Doiz, D. Lasagabaster, & J. M. Sierra (Eds.), English-medium instruction at universities: Global challenges (pp. 44–61). Bristol, UK: Multilingual Matters.
- Beckett, G., and F. Li. 2012. “Content-based English Education in China: Students’ Experiences and Perspectives.” Journal of Contemporary Issues in Education 7: 1.
- Bentler, P. M., and C.-P. Chou. 1987. “Practical Issues in Structural Modeling.” Sociological Methods & Research 16 (1): 78–117.
- Breeze, R., and E. Dafouz. 2017. “Constructing Complex Cognitive Discourse Functions in Higher Education: An Exploratory Study of Exam Answers in Spanish- and English-Medium Instruction Settings.” System 70: 81–91.
- Bryman, A. 2012. Social Research Methods. Oxford: Oxford University Press.
- Byun, K., H. Chu, M. Kim, I. Park, S. Kim, and J. Jung. 2011. “English-medium Teaching in Korean Higher Education: Policy Debates and Reality.” Higher Education 62 (4): 431–449.
- Coleman, J. A. 2006. “English-medium Teaching in European Higher Education.” Language Teaching 39 (01): 1–14.
- Costa, F., and J. A. Coleman. 2013. “A Survey of English-Medium Instruction in Italian Higher Education.” International Journal of Bilingual Education and Bilingualism 16 (1): 3–19.
- Cummins, J. 2008. “Teaching for Transfer: Challenging the two Solitudes Assumption in Bilingual Education.” Encyclopedia of Language and Education 5: 65–75.
- Dafouz, E., and M. M. Camacho-Miñano. 2016. “Exploring the Impact of English-Medium Instruction on University Student Academic Achievement: The Case of Accounting.” English for Specific Purposes 44: 57–67.
- Dafouz, E., M. Camacho, and E. Urquia. 2014. “‘Surely They Can’t do as Well’: A Comparison of Business Students’ Academic Performance in English-Medium and Spanish-as-First-Language-Medium Programmes.” Language and Education 28 (3): 223–236.
- Dalton-Puffer, C. 2011. “Content-and-language Integrated Learning: From Practice to Principles?” Annual Review of Applied Linguistics 31: 182–204.
- Darling-Hammond, L. 2006. “Assessing Teacher Education.” Journal of Teacher Education 57 (2): 120–138.
- Dimova, S., and J. Kling. 2018. “Assessing English-Medium Instruction Lecturer Language Proficiency Across Disciplines.” TESOL Quarterly 52 (3): 634–656.
- Ding, L., W. F. Velicer, and L. L. Harlow. 1995. “Effects of Estimation Methods, Number of Indicators per Factor, and Improper Solutions on Structural Equation Modeling fit Indices.” Structural Equation Modeling: A Multidisciplinary Journal 2 (2): 119–143.
- Doiz, A., D. Lasagabaster, and J. Sierra. 2013. “Globalisation, Internationalisation, Multilingualism and Linguistic Strains in Higher Education.” Studies in Higher Education 38 (9): 1407–1421.
- Doumen, S., J. Broeckmans, and C. Masui. 2014. “The Role of Self-Study Time in Freshmen’s Achievement.” Educational Psychology 34 (3): 385–402.
- Duong, V. A., and C. S. Chua. 2016. “English as a Symbol of Internationalization in Higher Education: A Case Study of Vietnam.” Higher Education Research & Development 35 (4): 669–683.
- Even, R., and D. Tirosh. 1995. “Subject-matter Knowledge and Knowledge About Students as Sources of Teacher Presentations of the Subject-Matter.” Educational Studies in Mathematics 29 (1): 1–20.
- Gao, X., and W. Ren. 2019. “Controversies of Bilingual Education in China.” International Journal of Bilingual Education and Bilingualism 22 (3): 267–273.
- Garson, G. 2014. Partial Least Squares: Regression and Structural Equation Models. Asheboro, NC: Statistical Associates.
- Gerlach, R. W., B. R. Kowalski, and H. O. Wold. 1979. “Partial least-squares path modelling with latent variables.” Analytica Chimica Acta 112 (4): 417–421.
- Gilmore, J., M. Vieyra, B. Timmerman, D. Feldon, and M. Maher. 2015. “The Relationship Between Undergraduate Research Participation and Subsequent Research Performance of Early Career STEM Graduate Students.” The Journal of Higher Education 86 (6): 834–863.
- Hair Jr, J. F., G. T. M. Hult, C. Ringle, and M. Sarstedt. 2016. A primer on partial least squares structural equation modeling (PLS-SEM). Thousand Oaks: Sage publications.
- Hair, J. F., C. M. Ringle, and M. Sarstedt. 2012. “Partial Least Squares: The Better Approach to Structural Equation Modeling?” Long Range Planning 45 (5-6): 312–319.
- Hair, J. F., J. J. Risher, M. Sarstedt, and C. M. Ringle. 2019. “When to use and how to Report the Results of PLS-SEM.” European Business Review.
- Harackiewicz, J. M., J. L. Smith, and S. J. Priniski. 2016. “Interest Matters.” Policy Insights from the Behavioral and Brain Sciences 3 (2): 220–227.
- Hodara, M. 2015. “The Effects of English as a Second Language Courses on Language Minority Community College Students.” Educational Evaluation and Policy Analysis 37 (2): 243–270.
- Hou, A. Y. C., R. Morse, C.-L. Chiang, and H.-J. Chen. 2013. “Challenges to quality of English medium instruction degree programs in Taiwanese universities and the role of local accreditors: A perspective of non-English-speaking Asian country.” Asia Pacific Education Review 14 (3): 359–370.
- Hu, G. 2007. “The Juggernaut of Chinese-English Bilingual Education.” Bilingual Education and Bilingualism 64: 94.
- Hu, G. 2008. “The Misleading Academic Discourse on Chinese–English Bilingual Education in China.” Review of Educational Research 78 (2): 195–231.
- Hu, G., L. Li, and J. Lei. 2014. “English-medium Instruction at a Chinese University: Rhetoric and Reality.” Language Policy 13 (1): 21–40.
- Hu, G., and S. L. McKay. 2012. “English Language Education in East Asia: Some Recent Developments.” Journal of Multilingual and Multicultural Development 33 (4): 345–362.
- Hu, J. J., and P. Wu. 2020. “Understanding English Language Learning in Tertiary English-Medium Instruction Contexts in China.” System 93: 102305.
- Huang, F. 2006. “Internationalization of Curricula in Higher Education Institutions in Comparative Perspectives: Case Studies of China, Japan and the Netherlands.” Higher Education 51 (4): 521–539.
- Jiang, A. L., and L. J. Zhang. 2019. “Chinese Students’ Perceptions of English Learning Affordances and Their Agency in an English-Medium Instruction Classroom Context.” Language and Education 33 (4): 322–339.
- Johnson, A. 2020. “The Impact of English Learner Reclassification on High School Reading and Academic Progress.” Educational Evaluation and Policy Analysis 42 (1): 46–65.
- Kim, E. G., S.-O. Kweon, and J. Kim. 2017. “Korean Engineering Students’ Perceptions of English-Medium Instruction (EMI) and L1 use in EMI Classes.” Journal of Multilingual and Multicultural Development 38 (2): 130–145.
- Kim, S. K., and S. Y. Sohn. 2009. “Expert System to Evaluate English Medium Instruction in Korean Universities.” Expert Systems with Applications 36 (9): 11626–11632.
- Kym, I., and M. H. Kym. 2014. “Students’ Perceptions of EMI in Higher Education in Korea.” The Journal of Asia TEFL 11 (2): 35–61.
- Lee, S. K. 2002. “The Significance of Language and Cultural Education on Secondary Achievement: A Survey of Chinese-American and Korean-American Students.” Bilingual Research Journal 26 (2): 327–338.
- Lei, J., and G. Hu. 2014. “Is English-Medium Instruction Effective in Improving Chinese Undergraduate Students’ English Competence?” International Review of Applied Linguistics in Language Teaching 52 (2): 99–126.
- Lin, L., J. W.-I. Lam, and S. K. Tse. 2019. “Test Takers’ Strategy use and L2 Chinese Reading Test Performance in Mainland China: A Structural Equation Approach.” Studies in Educational Evaluation 60: 189–198.
- Liu, W. 2020. “The Chinese Definition of Internationalisation in Higher Education.” Journal of Higher Education Policy and Management 43 (2): 230–245.
- Masui, C., J. Broeckmans, S. Doumen, A. Groenen, and G. Molenberghs. 2014. “Do Diligent Students Perform Better? Complex Relations Between Student and Course Characteristics, Study Time, and Academic Performance in Higher Education.” Studies in Higher Education 39 (4): 621–643.
- Musah, M. B., H. B. M. Ali, S. H. V. Al-Hudawi, L. M. Tahir, K. B. Daud, and A. R. Hamdan. 2015. “Determinants of Students’ Outcome: A Full-Fledged Structural Equation Modelling Approach.” Asia Pacific Education Review 16 (4): 579–589.
- Nicholls, K. 2020. “‘You Have to Work from Where They Are’: Academic Leaders’ Talk About Language Development.” Journal of Higher Education Policy and Management 42 (1): 67–84.
- Nitzl, C. 2016. “The use of Partial Least Squares Structural Equation Modelling (PLS-SEM) in Management Accounting Research: Directions for Future Theory Development.” Journal of Accounting Literature 37: 19–35.
- Pan, J. 2007. “Facts and Considerations About Bilingual Education in Chinese Universities.” Bilingual Education and Bilingualism 64: 200.
- Pecorari, D., P. Shaw, A. Irvine, and H. Malmström. 2011. “English for Academic Purposes at Swedish Universities: Teachers’ Objectives and Practices.” Ibérica 22: 55–78.
- Piller, I., and J. Cho. 2013. “Neoliberalism as Language Policy.” Language in Society 42 (01): 23–44.
- Poole, B. 2013. “English-medium Instruction at Universities: Global Challenges.” System 41 (3): 880–882.
- Ramsden, P. 1991. “A Performance Indicator of Teaching Quality in Higher Education: The Course Experience Questionnaire.” Studies in Higher Education 16 (2): 129–150.
- Robinson, H., W. J. Gerace, and J. P. Mestre. 1980. “Factors Influencing the Performance of Bilingual Hispanic Students in Math and Science Related Areas.” Integrated Education 18 (5-6): 38–42.
- Sahan, K., and O. Sahan. 2021. “Investigating Student and Alumni Perspectives on Language Learning and Career Prospects Through English Medium Instruction.” Teaching in Higher Education 1–22.
- Sayed, Y., and R. Ahmed. 2015. “Education Quality, and Teaching and Learning in the Post-2015 Education Agenda.” International Journal of Educational Development 40: 330–338.
- Schiefele, U. 1991. “Interest, Learning, and Motivation.” Educational Psychologist 26 (3-4): 299–323.
- Schmitter-Edgecombe, M., C. Parsey, and D. J. Cook. 2011. “Cognitive Correlates of Functional Performance in Older Adults: Comparison of Self-Report, Direct Observation, and Performance-Based Measures.” Journal of the International Neuropsychological Society 17 (5): 853–864.
- Simonsmeier, B. A., M. Flaig, A. Deiglmayr, L. Schalk, and M. Schneider. 2022. “Domain-specific Prior Knowledge and Learning: A Meta-Analysis.” Educational Psychologist 57 (1): 31–54.
- Smit, U., and E. Dafouz. 2012. “Integrating Content and Language in Higher Education.” AILA Review 25 (1): 1–12.
- Steyn, R., and J. Mynhardt. 2008. “Factors That Influence the Forming of Self-Evaluation and Self-Efficacy Perceptions.” South African Journal of Psychology 38 (3): 563–573.
- Tabatchnick, B. G., and L. S. Fidell. 2001. Using Multivariate Statistics. Boston: Allyn and Bacon.
- Tai, T.-E., and C. W. Tang. 2021. “The Role of Graduate Students’ Learning Strategies in Reducing Their English Medium Instruction Avoidance: The Mediation Effect of Language Anxiety.” Asia Pacific Journal of Education 41 (2): 368–384.
- Tange, H. 2012. “Organising Language at the International University: Three Principles of Linguistic Organisation.” Journal of Multilingual and Multicultural Development 33 (3): 287–300.
- Temple, J. A., and A. J. Reynolds. 2007. “Benefits and Costs of Investments in Preschool Education: Evidence from the Child–Parent Centers and Related Programs.” Economics of Education Review 26 (1): 126–144.
- Thompson, R. A., and B. L. Zamboanga. 2003. “Prior Knowledge and Its Relevance to Student Achievement in Introduction to Psychology.” Teaching of Psychology 30 (2): 96–101.
- Tong, F., and Q. Shi. 2012. “Chinese–English Bilingual Education in China: A Case Study of College Science Majors.” International Journal of Bilingual Education and Bilingualism 15 (2): 165–182.
- van der Worp, K. 2017. “English Medium Instruction: A way Towards Linguistically Better Prepared Professionals in the Basque Autonomous Community?” International Journal of Multilingualism 14 (1): 53–68.
- Wilkinson, R. 2013. English-medium instruction at a Dutch university: Challenges and ptifalls. In A. Doiz, D. Lasagabaster, & J. M. Sierra (Eds.), English-medium instruction at universities: Global challenges (pp. 3–24). Bristol, UK: Multilingual Matters.
- Xie, W. Y., and S. Curle. 2022. “Success in English Medium Instruction in China: Significant Indicators and Implications.” International Journal of Bilingual Education and Bilingualism 25 (2): 585–597.
- Yang, W. 2015. “Content and Language Integrated Learning Next in Asia: Evidence of Learners’ Achievement in CLIL Education from a Taiwan Tertiary Degree Programme.” International Journal of Bilingual Education and Bilingualism 18 (4): 361–382.
- Zhang, M. J., and E. Pladevall-Ballester. 2021. “Discipline-specific Language Learning Outcomes in EMI Programs in the People’s Republic of China.” Language and Education 35 (4): 357–374.
- Zhou, X. Z., C. K. Li, and X. S. Gao. 2021. “Towards a Sustainable Classroom Ecology:& Nbsp; Translanguaging in English as a Medium of Instruction (EMI) in a Finance Course at an International School in Shanghai.” Sustainability 13 (19): 14.
- Zimmerman, B. J. 2002. “Becoming a Self-Regulated Learner: An Overview.” Theory Into Practice 41 (2): 64–70.