ABSTRACT
Automated vehicles (AVs) could completely change mobility in the coming years and decades. As AVs are still under development and gathering empirical data for further analysis is not yet possible, existing studies mainly applied models and simulations to assess their impact. This paper provides a comprehensive review of modelling studies investigating the impacts of AVs on travel behaviour and land use. It shows that AVs are mostly found to increase vehicle miles travelled and reduce public transport and slow modes share. This particularly applies to private AVs, which are also leading to a more dispersed urban growth pattern. Shared automated vehicle fleets, conversely, could have positive impacts, including reducing the overall number of vehicles and parking spaces. Moreover, if it is assumed that automation would make the public transport system more efficient, AVs could lead to a favouring of urbanisation processes. However, results are very sensitive to model assumptions which are still very uncertain (e.g. the perception of time in AVs) and more research to gain further insight should have priority in future research as well as the development of the models and their further adaptation to AVs.
1. Introduction
Urban and settlement development has always been closely linked to transport and the development of technological mobility innovations. Whereas until the middle of the nineteenth century journeys were almost entirely by foot, the implementation of the rail and the car reshaped transport and settlement development. Land use and transport are interdependent and their interaction is underpinned theoretically in the so-called transport land use feedback cycle (). Patterns of land use influence people’s activities, i.e. the choices and decisions for places to live, work and spend leisure time which in turn create a need of physical movement between these places (Bertolini, Citation2017). The desire to move between these different locations, based on the decisions concerning activities, is what generates transport or travel demand (Axhausen & Gärling, Citation1992; Jones, Dix, Clarke, & Heggie, Citation1983; Kitamura, Citation1988). This is accommodated by transport networks and is a key driver of network development and thus change in transport supply. The characteristics of the transport supply (e.g. capacity, costs, etc.) determine the accessibility of locations (Hansen, Citation1959) and with that their attractiveness for land use development, thus changing land use characteristics (Bertolini, Citation2017). Some of these interactions are direct or happening in a relatively short time, whereas others are observable only over the long-term. Moreover, as the system is dynamic on the left and the right-hand side of the cycle need to be considered simultaneously (Kasraian, Maat, Stead, & van Wee, Citation2016).
Figure 1. Transport land use feedback cycle (Wegener & Fürst, Citation1999; adapted by Bertolini, Citation2012).
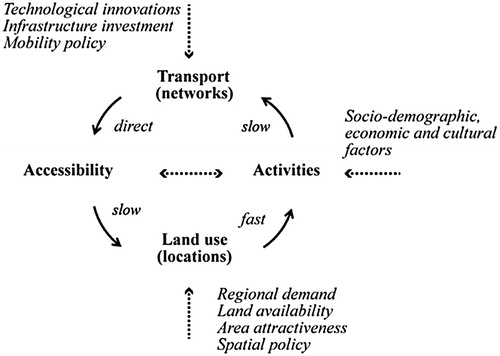
However, this interaction should be seen as open-ended and its development co-determined by other factors. Land use developments do not only depend on accessibility conditions but also on the availability of land. For the emergence of activity patterns also individual characteristics of households and characteristics of the wider socio-economic context play a role. And finally, transport supply is not only determined by transport demand but also by infrastructure investments, mobility politics (policy and regulation) or technological innovations – such as automated vehicles (AVs) (Bertolini, Citation2017).
AVs are a technological innovation which will allow organising transport supply in a radically different way. Depending on the specific use case, existing transport services could either be complemented or replaced. Changes in the transport supply will mainly impact comfort, travel time (e.g. driving time due to capacity increase (Ambühl, Ciari, & Menendez, Citation2016) or time for accessing and egressing the vehicles) and time use benefits (gain of productive time as nobody needs to steer the car), reliability, costs or operation costs and safety (Milakis, van Arem, & van Wee, Citation2017). Such changes in the transport supply will have impacts on accessibility and transport demand (Alessandrini, Campagna, Delle Site, Flilippi, & Persia, Citation2015; European Commission, Citation2016). Initially, there will be impacts on travel behaviour, such as the type of activities, number of journeys, or the choice of transport mode. However, only in the long-term, there will likely be impacts on the location choice of households and firms and hence the settlement structure. Moreover, as the interaction of land use and transport is co-determined by other factors, there is an influence of individual characteristics and needs of households and firms (e.g. household formation), area attractiveness, land availability and supply of dwellings, mobility and land use related policy as well as other accompanying technological developments. Furthermore, the potential conversion of parking spaces due to AVs will affect the availability of land and the construction activity of investors and therefore the supply (and prices) of dwellings (Moreno, Citation2017). All in all, the impacts of AVs on the interaction between land use and transport are very complex.
This topic only recently started to get attention by researchers. Until lately the ongoing discourse on AVs focused predominantly on the technology. Investigating the impacts of AVs on travel behaviour and transportation systems has been widely popular in recent years, but how these would affect land use was long neglected, with the exception of several researchers who recently addressed the subject more or less explicitly (Milakis et al., Citation2017). As AVs are not yet available to the public at large, it is impossible to gather empirical data for further analysis and studies in the literature mainly apply various models and simulations to analyse the impacts of AVs (Milakis et al., Citation2017; Zhang, Citation2017). Those modelling studies mostly focus on traffic impacts, impacts on travel behaviour and impacts on land use (Correia, Milakis, van Arem, & Hoogendoorn, Citation2015).
This paper explicitly focuses on a review of modelling studies investigating the impacts of AVs on (1) travel behaviour, i.e. trip generation rate, mode choice and the possible mobilisation of new user groups due to AVs (e.g. impaired people and elderly), and (2) land use, i.e. location choices of people and firms and reductions in parking space due to the possibility of AVs substituting conventional vehicles and related reductions in fleet size, and summarizes their results with regard to these topics.
Modelling studies focusing on traffic impacts of AVs, i.e. dealing with traffic flow performance, road capacity, congestion, or wider societal or environmental implications, i.e. emissions, energy consumption, etc. (Milakis et al., Citation2017; Stocker & Shaheen, Citation2017) are outside the scope of this paper. As our focus lies on impacts on travel behaviour within the overall transport system, the latter also applies to studies investigating public’s preferences only (e.g. using discrete-choice models).
In light of the growth of modelling studies investigating the impacts of AVs on travel behaviour and land use in recent years, synthesising current understandings to draw a comprehensive picture seems to be critical (Zhang, Guhathakurta, & Khalil, Citation2018). Key contributions of this paper are therefore (1) systematically collecting and reviewing such studies, (2) summarising their results, (3) derive insights on the key impacts and the interdependencies among them and (4) identify research gaps in the field and possible future avenues of research. To the best of our knowledge, it has not been done in such an exhaustive way before.
All studies reviewed were published in peer-reviewed journals between 2013 and 2018, however, also non-published studies presented at important conferences are incorporated. Included are peer-reviewed journal articles listed in Scopus, which contained any combination of the following keywords: automated vehicle(s), autonomous vehicle(s), automated car(s), autonomous car(s), self-driving vehicle(s), self-driving car(s), transport, spatial, land use, parking, accessibility, sharing, modelling, travel demand model, agent-based model, activity-based model. Moreover, the search was also expanded to Google and Google Scholar. Evidence from several countries in different continents is used and compared.
The paper is divided into three parts. Section 2 reviews the basic characteristics of the studies like (a) topic, methodology and unit of analysis, (b) assumptions/ scenarios and (c) indicators. Section 3 summarizes the findings of the studies and elaborates how model assumptions may influence results. Finally, Section 4 provides a conclusion and identifies research gaps in the field and possible future avenues of research.
2. Study characteristics
This section mentions the basic characteristics of the studies. An overview of these characteristics with regard to the individual studies is provided in .
Table 1. Summary of study characteristics and results on impacts of AVs on travel behaviour and land use.
2.1. Topic, methodology and unit of analysis
Most of the modelling studies found in the literature and reviewed in this paper focus on the impacts of AVs on travel behaviour. These studies mainly use existing travel demand modelling methods, including trip-based models as well as activity and agent-based models. Several modelling studies focus on possible impacts on land use, mainly using integrated land use and transport models or focusing on the effects of AVs on accessibility. However, several studies also investigate both topics, for example looking at the possibility of AVs substituting conventional vehicles and related reductions in fleet size, implying impacts on parking land and therefore land use, but also describing possible impacts on travel behaviour.
In the reviewed studies the unit of analysis, i.e. the area where the models are applied for, ranges from a smaller part of a city to a whole country. Most studies investigate impacts of AVs based on real world network and local travel survey data. In some cases, studies also focus on a hypothetical grid-based city context (e.g. Zhang et al., Citation2015). In the latter case, travel behaviour is mostly simulated without actual local travel survey data: travel demand is recreated based on national trip characteristics and trip generation rates are assigned to each grid cell in the hypothetical city (Zhang, Citation2017).
A total of 37 modelling studies were reviewed with studies originating from the U.S.A. (20 studies), Europe (14 studies), Asia (2 studies) and Australia (1 study).
2.2. Scenarios/variables and model assumptions
Whereas some studies investigate the impacts of private AVs, others look at shared automated vehicles (SAVs) or a mix of both. Moreover, studies partly use different model assumptions, i.e. apply simplifications to recreate travel behaviours (see before), but also regarding vehicle speeds or sharing services priorities (Zhang, Citation2017).
Transport supply variables often include the share of trips which are made by SAVs sequentially (carsharing) or simultaneously (ridesharing). This is determined by (a) assuming a specific share, e.g. studies that investigate the replacement of all (e.g. Spieser et al., Citation2014) or a small share (e.g. Fagnant & Kockelman, Citation2014) of private vehicle trips by SAVs, (b) splitting trips by modes using a rule-based mode-choice model based on the existence/non-existence of public transport (e.g. ITF, Citation2015) or (c) incorporating assumptions on SAVs such as pricing structure, etc. (see below) in the mode-choice model, looking also on possible shifts in mode choices (e.g. Hörl et al., Citation2016). Moreover, the studies on SAVs include model assumptions on how SAVs are assigned to serve calling clients, i.e. the vehicle dispatching model (e.g. first-come first-served or demand-supply balancing dispatching strategy), assumptions on the relocation of the vehicles (e.g. Fagnant & Kockelman, Citation2014), the size of SAVs, as well as assumptions on acceptable waiting times for the users. In addition, assumptions on the acceptance of longer trip durations due to the pick-up of other passengers (see e.g. Bourghout et al., Citation2014), when matching clients with similar itinerary to share rides, are made in the case of ridesharing (Zhang, Citation2017).
A recurring assumption is the reduction of the value of time or travel time (VOT) due to AVs, because of the increased comfort, reduced stress and increased productivity while travelling as a passenger instead of as a driver (e.g. Childress et al., Citation2015).
Another frequent assumption is an increase in road capacity due to AVs. This assumption is mostly used in modelling studies investigating the impact of private AVs (e.g. Gucwa, Citation2014). Studies looking at the impact of SAVs however mostly didn’t incorporate effects on road capacity. Moreover, especially earlier studies did not considered congestion (and explicit traffic assignment) and built upon rather great simplifications regarding the link travel speeds with regard to the time of the day (Levin et al., Citation2017; Zhang, Citation2017).
In addition, a couple of studies include and investigate possible models and assumptions on operating and parking costs of private AVs and SAVs (e.g. Chen & Kockelman, Citation2016). While in most of the studies the scenarios are defined through assumptions regarding private AVs and SAVs only, some studies (e.g. Gelauff et al., Citation2017) make assumptions also regarding public transport. Moreover, while some studies focused on the aspects mentioned above, in some cases several such assumptions were combined.
2.3. Indicators
Several indicators are used to examine the impacts of AVs on travel behaviour and land use. Regarding travel behaviour, mostly changes in vehicle miles travelled (VMT) or vehicle kilometres travelled (VKT) and changes in mode shares are used as impact indicators (e.g. Hörl et al., Citation2016). Some studies also use indicators such as travel time changes, i.e. vehicles hours travelled (VHT) (e.g. Childress et al., Citation2015).
Regarding land use impacts, studies focusing on reductions of parking land use the change in the number of parking spaces as an impact indicator, calculated by investigating the change in the number of vehicles, i.e. the AVs fleet size, to process the current transport demand and using a specific parking space per car ratio (e.g. Zhang et al., Citation2015). However, also studies not using a specific parking space per car ratio but only investigating the change in the number of vehicles to process the current transport demand (e.g. Boesch et al., Citation2016) imply possible changes in parking needs and therefore parking space needed and ultimately would make land reclaim and land use changes possible. Studies focusing on location choices of people and firms mostly investigate the population development (e.g. Gelauff et al., Citation2017) or changes in job density (e.g. Zhang, Citation2017) in different areas of the study region. Zhang (Citation2017) uses changes in the median distance of household locations to the central business district (CBD). Meyer et al. (Citation2017) investigate changes in accessibility for municipalities, implying land use impacts.
3. Results of the studies
This section first reports the main results of the studies and then elaborates on how model assumptions may influence results. The description of the results is separated in impacts on travel behaviour and on land use. gives an overview of the results of the individual studies.
3.1. Impacts on travel behaviour
3.1.1. Vehicles miles travelled (VMT)/vehicle kilometres travelled (VKT)
Studies mostly indicate an increase of VMT or VKT due to AVs. Regarding private AVs, studies (e.g. Auld et al., Citation2017; Gucwa, Citation2014; Kim et al., Citation2015a) report higher increases in VMT (about 15–59%) resulting from migration effects from other modes, when a high reduction of the value of time, a reduction in parking costs and high market shares of AVs are assumed. However, assumptions on an increase in road capacity seem to contribute less to additional VMT (about +1–4%). Kim et al. (Citation2015a) for example – who use a mode and trip-choice model and assume a change in road capacity by +50% – indicate an increase in VMT by 4% for the Atlanta region in the U.S.A. However, a higher increase in VMT of 24% is reported, when also changes of the value of time by −50% and vehicle operating (−71%) and parking costs (−100%) for private AVs compared to current private cars are assumed.
Studies on SAVs (e.g. Childress et al., Citation2015; Hörl et al., Citation2016; Liu et al., Citation2017) also mainly report that SAVs lead to an increase in VMT because of empty trips and migration effects from other modes. High additional VMT (about 35–60%) is reported in particular, when a high reduction in the value of time and low costs for SAVs are assumed. Hörl et al. (Citation2016) for instance use a mode-choice model as well as assumptions on operating costs of SAVs of $0.85 and on a reduction of the value of time by 65% compared to current private cars and report an increase of VMT by 60% for the city of Sioux Falls in the USA. Studies that assume a replacement of private vehicle trips by SAVs based on assuming a specific share or by splitting trips by modes using a rule-based mode-choice model, i.e. not modelling a competition with existing modes (e.g. Chen et al., Citation2016; Fagnant et al., Citation2015; Fagnant & Kockelman, Citation2014; Friedrich & Hartl, Citation2016; ITF, Citation2015), also indicate an increase in VMT. This increase is in the magnitude of about 8–10%, when assuming that only a small share (1.3–10%) of private vehicle trips is replaced by SAVs and results from additional empty trips. ITF (Citation2015) and Friedrich and Hartl (Citation2016) however, splitting trips by modes based on the existence/non-existence of public transport, also report higher increases in VKT (39–89%), when assuming that all private vehicles are replaced by SAVs and public transport is non-existent.
However, several studies (e.g. Bourghout et al., Citation2014; Heilig et al., Citation2016; Martinez & Viegas, Citation2017) also indicate that SAVs could decrease VMT (about 10–25%) if a large share of the travellers is willing to rideshare. For example, Bourghout et al. (Citation2014) assume the replacement of all home-based work private vehicle trips by SAVs with ridesharing and report a decrease of VKT by 11–24% dependent on the acceptable increase of travel time (+30% to +50%) due to ridesharing. Moreover, a decrease in VMT is reported when costs per mile for SAVs are high and travellers opt for short trips: Childress et al. (Citation2015) use a mode and trip-choice model and report that VMT experience a reduction by 35% for the Seattle region in the USA, when costs per mile of $1.65 for SAVs as well as no possibility of using private vehicles are assumed.
3.1.2. Mode/modal share
Studies that investigate impacts on mode share by modelling a competition of AVs with currently existing modes mostly indicate that AVs lead to a reduction in public transport and slow modes share. For private AVs, studies (e.g. Correia & van Arem, Citation2016; Kim et al., Citation2015a; Kröger et al., Citation2018) particularly report larger mode shifts, when a high reduction of the value of time and a reduction in parking or operating costs (as well as high penetration rates) are assumed, leading to an increase in private car modal split. Kröger et al. (Citation2018) for example use a combined mode and distance-choice model and assume a reduction in the value of time by 25%, different penetration rates for private AVs (based on a diffusion model) and private AV availability for teenagers, adults without driving license and mobility-impaired people. They report a decrease in public transport share from 2.6% to 2.2% and an increase in car share from 65.6% to 69.4% (penetration rate = 29.3%) for the USA as well as a decrease in public transport share from 8.6% to 7.7% and an increase in car share from 45.1% to 48.8% (penetration rate = 37.6%) for Germany.
Similarly, for SAVs, studies (e.g. Boesch et al., Citation2018; Chen & Kockelman, Citation2016) particularly indicate a reduction in public transport and slow modes share when assuming a high reduction in the value of time and rather low operating costs. This is even the case if lower operation costs for public transport are also assumed. Instead of private AVs however, SAVs also lower the private car modal split. For example, Boesch et al. (Citation2018) use a mode-choice model based on assumptions on the reduction of the value of time for SAVs by 54% compared to current private cars as well as operating costs of 0.46 CHF/km (and assumptions regarding a reduction of the value of time for private AVs and operating costs for private AVs and public transport). They indicate a reduction in public transport share from 16% to 12%, a decrease in slow modes share from 26% to 20% and a decrease in private car share from 48% to 36%.
However, studies on SAVs which assume a complete ban of privately owned vehicles and specific (rather high) operating costs (e.g. Childress et al., Citation2015; Heilig et al., Citation2016) also report increases in public transport and slow mode shares because of people using the latter modes especially for short trips to avoid costs. Heilig et al. (Citation2016) for example use a combined destination and mode-choice model and assume a reduction of operating costs for SAVs with ridesharing by 45% compared to current cars as well as no possibility of using private vehicles. They report that increases in public transport share (from 13% to 17%) and in walk share (from 22% to 31%) are indicated even when operating costs for SAVs are reduced compared to current cars.
3.1.3. Vehicle hours travelled (VHT)
Regarding the impacts of AVs on VHT, studies (e.g. Childress et al., Citation2015; Kim et al., Citation2015a) mostly indicate that VHT seems to increase with private AVs if these are assumed to imply a high reduction in the value of time and a reduction in parking costs. In contrast, SAVs could reduce VHT if these are accompanied with high costs and there is no possibility to use private vehicles: Childress et al. (Citation2015) for instance use assumptions on changes in road capacity (+30%), the value of time (−35%) and parking costs (−50%) for private AVs compared to current private cars and indicate an increase in VHT of up to 17%. However, when assuming costs per mile of $1.65 for SAVs (no ridesharing) and no possibility of using private vehicles, a decrease in VHT by 41% is reported.
3.2. Impacts on land use
3.2.1. Parking spaces (including number of vehicles to process the travel demand – fleet size)
Impacts of AVs on parking spaces were found to be quite large, either explicitly calculated by applying a specific parking space to car ratio to the modelled impact on the change in the number of vehicles to process the travel demand or by implying impacts on parking land from the latter. Most studies (e.g. Bischoff & Maciejewski, Citation2016; Chen et al., Citation2016; Fagnant et al., Citation2015; Martinez & Viegas, Citation2017; Spieser et al., Citation2014) investigated a replacement of all or a specific share of current private vehicle trips by SAVs and suggest that this could substantially (up to around 90%) reduce the number of vehicles to process the current transport demand (with regard to the replaced trips) and even more so, if ridesharing is also assumed, implying reduced parking needs. Bischoff and Maciejewski (Citation2016) for example focus on the city of Berlin in Germany and indicate that replacing all private vehicle trips with SAVs reduces the number of vehicles to process the current private vehicle demand by 91%. Regarding SAVs with ridesharing, Martinez and Viegas (Citation2017) report a reduction in the number of vehicles to process the travel demand by 95% for the Lisbon region, when assuming SAVs with ridesharing replacing all motorised trips. Several studies (e.g. ITF, Citation2015; Zhang et al., Citation2015; Zhang & Guhathakurta, Citation2017) also calculated the impacts on parking spaces explicitly by using a specific parking space to car ratio and mostly report nearly equal reductions of the number of parking spaces (around 80–90%) with regard to the number of vehicles to process the current travel demand. Similarly, assuming ridesharing further reduced the number of parking spaces needed. Zhang et al. (Citation2015) for example focus on a hypothetical grid-based city and report a reduction in the number of needed parking spaces by 90% with regard to the 2% of trips they assumed being served by SAVs; when assuming half of these trips with ridesharing, the reduction was even higher (91%).
However, if private ownership is assumed, i.e. private AVs that are shared within the household, with otherwise similar assumptions, only a much smaller reduction in the number of vehicles to process the travel demand would be possible, implying also smaller impacts on parking spaces: Zhang et al. (Citation2018) assume that the replacement of private vehicles with AVs in households is determined by the minimum number of AVs to satisfy the travel demand of all household members with no trip delay and report only a reduction in the number of vehicles by 9.5% for the Atlanta region in the U.S.A.
3.2.2. Location choices of people and firms
Concerning impacts of AVs on location choices of people and with regard to private AVs, studies (e.g. Gelauff et al., Citation2017; Kim et al., Citation2015b; Meyer et al., Citation2017; Thakur et al., Citation2016) mostly show that these lead to an increase in accessibility and an increase in population in well-connected far outer suburbs and rural regions. These processes of sprawling and more dispersed development of the settlement structure are reported in particular, when a high reduction of the value of time, an increase in road capacity and (related) reductions in travel time are assumed. Thakur et al. (Citation2016) for example model travel behaviour and residential location choices (determined by the accessibility to employment) for the Melbourne region in Australia in the year 2046 and assume a reduction of the value of time for private AVs by 50% compared to current cars. They report a reduction of the population of 4% in inner parts of the city and an increase of 3% of the population in the far outer suburbs.
Studies on SAVs (e.g. Meyer et al., Citation2017; Zhang, Citation2017) mainly report that SAVs could curb urban sprawl, however, effects are mixed, as some population groups might also locate further away because of increased accessibility in more rural areas. This is especially the case when a reduction in the value of time and an increase in road capacity are assumed. Zhang (Citation2017) for example investigated the impacts of SAVs on residential location choices in the Atlanta region in the USA and reports a decrease in median distance to CDB for older people (kids/ no kids) by 2–7% but an increase of younger people (kids/no kids) by 7–10%, when assuming a replacement of all private vehicle trips by SAVs, operating costs of $0.3/mile and a reduction in the value of time by 100% of SAVs compared to current private cars. However, when SAVs with ridesharing but no changes in the value of time are assumed, the concentration of population in urbanised regions, i.e. urbanisation processes, are reported: Thakur et al. (Citation2016) assume a replacement of all private vehicle trips with SAVs with ridesharing as well as operating costs of 0.49€ per km and report an increase in population by 4% in inner parts of the city, while far outer suburbs experience a reduction of the population by 3%.
Similarly, an inclination towards urbanisation processes is indicated when a more efficient public transportation system due to automatisation (e.g. AV shuttles for the last mile) and related reductions in travel time are assumed: Gelauff et al. (Citation2017) focus on the modelling of home and job location as well as commuting mode choices in the Netherlands and assume a reduction of travel time by 20% (compared to current private cars) and out-of-vehicle travel time by 100% for public transport as well as a reduction in access/ egress times of trains by 50%. They indicate an increase in population in large cities by 3% as well as a population decline in suburbs of smaller cities by 3% and in non-urban regions by 2%.
Regarding impacts of AVs on location choices of firms, it is indicated that SAVs, when assumed with a reduction in the value of time and a reduction in parking spaces, seem to contribute to further deindustrialisation trends in cities, i.e. firms of the secondary sector locating outside of cities: Zhang (Citation2017) uses the same assumptions as above but operation costs for SAVs of $0.13/mile to $0.5/mile as well as a reduction in parking spaces by 90% and reports a decrease in job density by 1.8–17.5% in inner city parts and an increase by 0.2–9.8% in suburban areas for the secondary sector, such as construction or manufacturing. For the tertiary sector, such as service or public, an increase in job density in inner parts of the city by 0.3–11.8% and a decrease in suburban areas by 2.5–7.8% is observed.
3.3. Influence of results by model assumptions
As expected, the results confirm that impacts of AVs on travel behaviour and land use are strongly dependent on the assumptions made. The most frequently used assumptions concerned the share of trips that are replaced with SAVs and the waiting time of users, the changes in the value of time, the increase in road capacity and the penetration rate of AVs. Moreover, the modelling technique used and in particular the simplifications or assumptions for modelling applied to play an important role.
Whereas especially early studies investigated impacts on travel behaviour based on the assumption that activity pattern will not change after introducing SAVs (Zhang, Citation2017), more recent studies on SAVs, private AVs or a mix of both mostly use mode-choice models (combined with trip and destination choice) and also investigate and consider shifts in modal shares due to the assumptions made. Moreover, only a few studies (e.g. Kröger et al., Citation2018) incorporated the mobilisation of new user groups, i.e. additional changes in travel behaviour because of AV availability for children/teenagers, adults without driver license or mobility-impaired people.
Concerning assumptions on the transport supply with AVs, assumptions on a reduction of the value of time seem to have a higher influence on the impacts than assumptions on an increase in road capacity or on operating costs (e.g. Boesch et al., Citation2018). Moreover, for the impacts of SAVs on travel behaviour especially assumptions regarding the level of service acceptance, in terms of waiting and trip time, by passengers of SAVs plays an important role. The studies assume different waiting times a passenger would accept for being picked up. Average waiting time varies between about 0.3 minutes (Fagnant & Kockelman, Citation2014) and 8 minutes (Llorca et al., Citation2017). These imply also different (maximum) waiting times at peak time, which were up to more than 17 minutes (e.g. Hörl et al., Citation2016). For ridesharing, also the acceptable travel time increase plays a role (e.g. Burghout et al., Citation2014). In addition, Chen et al. (Citation2016) indicate that charging processes are important, when assuming electric SAVs. Moreover, assumptions on the vehicle dispatching model (e.g. first-come first-serve principal or demand-supply balancing dispatching strategy), the relocation of the vehicles and model simplifications or assumptions such as using grid-networks instead of local transportation networks or constant travel speeds at specific day times instead of being based on a traffic assignment and traffic flow simulator to incorporate congestion play a role.
All these factors have a direct link to the impacts of SAVs on travel behaviour, i.e. VMT, and land use, i.e. the number of parking spaces needed based on the number of SAVs needed to process the travel demand. Especially earlier modelling studies that used grid-based networks and constant travel speeds – and therefore used a lot of simplifications in modelling – may over-predict the number of SAVs to process the travel demand (Levin et al., Citation2017). In addition, Boesch et al. (Citation2016) further look at the trips generated outside the study area and show that high reductions in the number of SAVs to process the travel demand are not realistic unless the level of service is reduced.
4. Conclusion and recommendations for future research
This paper systematically reviewed modelling studies investigating the impacts of AVs on travel behaviour and land use and summarised their results, which has not been done in such an exhaustive way before. It shows that several initial studies of this kind have been carried out for cities and regions worldwide, especially in the U.S.A. and in Europe.
The results indicate that AVs are mostly found to increase VMT and lead to reductions of the public transport and slow modes share, especially when private AVs or SAVs without ridesharing and a high reduction in the value of time are assumed. However, (a high share of) SAVs could significantly reduce the number of vehicles necessary to process the current travel demand, especially when ridesharing is assumed, which could result in less parking spaces needed, which could allow reclaiming such space and giving it a different use. Regarding land use effects based on changes in location choices of people and firms, studies mainly illustrate that private AVs – especially when a reduction of the value of time and capacity increases are assumed – lead to increases in population in well-connected distant suburbs and rural regions, i.e. a much more dispersed and scattered urban growth pattern. However, SAVs in combination with a ridesharing scheme (and no changes in the value of time) or a more efficient public transportation system due to automatisation (e.g. AV shuttles for the last mile) could lead to an increase in population in urban areas, i.e. population clustering in urban areas. In this regard, it should be also considered that such models generally do not have high spatial detail and tend to differentiate only between urban and suburban areas and therefore such results may be also the product of an oversimplification and more complex patterns may arise with more sophisticated modelling approaches in the future.
However, the paper also found that results are strongly dependent on model assumptions: impacts assessed by the models appear to be particularly sensitive to a reduction in value of time as compared to increases of road capacity or operating costs. Moreover, different model simplifications (especially in earlier studies) lead to overestimations regarding the possible reduction of the number of vehicles or parking spaces needed due to SAVs.
In addition, different results based on nearly the same assumptions in several studies dealing with different contexts strongly suggest that the settlement structure plays an important role. Trip durations and distances differ from city to city and in less densely areas (suburban, rural) more empty rides would be carried out and thus, comparatively, more vehicles would be needed to replace the current fleet (Bischoff & Maciejewski, Citation2016). However, studies particularly focusing on rural areas have hardly been conducted so far and more research on this aspect seem to be crucial to understand such differences. Furthermore, studies mostly investigated the impacts of highly advanced AVs, i.e. AVs that could operate in all contexts of the city (region). However, in the near future only the deployment of AVs in specific operational design domains, i.e. specific conditions and contexts (SAE International, Citation2018), seems feasible (Beiker, Citation2018). This should be specifically considered in future studies.
The studies reviewed are a first step to investigate the possible impacts of AVs on travel behaviour and land use. However, more work is needed to better understand them. Future research should grant a better insight on the socio-demographic groups which will benefit from increased accessibility due to automated driving – a first step being the work of Zhang (Citation2017) in the context of modelling location choices. For instance, working in a car is not possible for all jobs and is especially dependent on future working models: Cyganski, Fraedrich, and Lenz (Citation2015) – conducting a stated preference survey on the use of AVs – indicate that only 13% of respondents reported the ability to work as an advantage of AVs, that being especially the case for persons with higher income. And this is even without taking into account that acceleration patterns of cars (especially later acceleration) might limit the ability to do so (Le Vine, Zolfaghari, & Polak, Citation2015). Therefore, more empirical research is needed on generalised travel costs and the perception of time in AVs in the future, especially when other passengers are in the vehicle (ridesharing).
These aspects have to be incorporated in future models, e.g. by integrating new and preferably socially differentiated survey results regarding such preferences that include AVs rather as a developing pathway than a fully formed mode alternative (stated adoption). Moreover, the model structures should be further adapted, e.g. by taking into account the inter-temporal nature of AV adoption and including path dependencies (Hawkins & Habib, Citation2018; Zhang et al., Citation2018).
As future remains unknown and technologies advance especially in the area of sensors, other aspects not yet mentioned could become important too. For example, ridesharing could be very different from today: matching-algorithms on top of considering start and destinations points and possible routes of users could also integrate a social-emotional matching of passengers since sharing a ride is more attractive with socially and emotionally similar people. The service by BlaBlaCar can be considered a first attempt in this direction (BlaBlaCar, Citation2018). Also, different use cases of AVs are conceivable, with vehicles (e.g. sizes, etc.) that are completely different from those today. Therefore, impacts on travel behaviour and land use could then be totally different of those presented in the reviewed studies.
In summary, the studies reviewed to provide the best existing insight on the topic as of today and the synthesis provided by this paper can be useful for both policy-makers and researchers. Although results are overall not solid enough to rely on them to base policies upon, the causalities observed are recurrent enough that they can at least give some useful insight on where the system would head to under specific circumstances. For researchers, this can give hints on which aspects of the existing studies would need to be pursued more in depth and which have been neglected so far and should be pursued in the future.
Disclosure statement
No potential conflict of interest was reported by the authors.
Additional information
Funding
References
- Alessandrini, A., Campagna, A., Delle Site, P., Flilippi, F., & Persia, L. (2015). Automated vehicles and the rethinking of mobility and cities. Transportation Research Procedia, 5, 145–160. doi: 10.1016/j.trpro.2015.01.002
- Ambühl, L., Ciari, F., & Menendez, M. (2016). What about space? A simulation based assessment of AVs impact on road space in urban areas. Paper presented at the 16th Swiss transport research conference.
- Auld, J., Sokolov, V., & Stephens, T. S. (2017). Analysis of the effects of connected-automated vehicle technologies on travel demand. Transportation Research Record: Journal of the Transportation Research Board, 2625, 1–8. doi: 10.3141/2625-01
- Auld, J., Verbas, O., Javanmardi, M., & Rousseau, A. (2018). Impact of privately-owned level 4 CAV technologies on travel demand and energy. Procedia Computer Science, 130, 914–919. doi: 10.1016/j.procs.2018.04.089
- Axhausen, K. W., & Gärling, T. (1992). Activity-based approaches to travel analysis: Conceptual frameworks, models, and research problems. Transport Reviews, 12(4), 323–341. doi: 10.1080/01441649208716826
- Bangemann, C. (2017). Simulation einer urbanen Mobilitätslösung basierend auf autonom fahrenden E-Robotaxen in München. TU München: Berylls Strategy Advisors.
- Beiker, S. (2018). Deployment of automated driving as an example for the San Francisco Bay area. In G. Meyer & S. Beiker (Eds.), Road vehicle automation 5 (pp. 117–129). Cham: Springer.
- Bertolini, L. (2012). Integrating mobility and urban development agendas: A manifesto. Disp – The Planning Review, 48(1), 16–26. doi: 10.1080/02513625.2012.702956
- Bertolini, L. (2017). Planning the mobile metropolis. Transport for people, places and the planet. London: Palgrave.
- Bischoff, J., & Maciejewski, M. (2016). Simulation of city-wide replacement of private cars with autonomous taxis in Berlin. Procedia Computer Science, 83, 237–244. doi: 10.1016/j.procs.2016.04.121
- BlaBlaCar. (2018). How chatty are you in the car? Retrieved from: https://www.blablacar.com/blog/blablalife/travel-tips/chatty
- Boesch, P., Ciari, F., & Axhausen, K. (2018). Transport policy optimization with AVs. Paper presented at the TRB annual meeting.
- Boesch, P. M., Ciari, F., & Axhausen, K. W. (2016). Autonomous vehicle fleet sizes required to serve different levels of demand. Transportation Research Record: Journal of the Transportation Research Board, 2542, 111–119. doi: 10.3141/2542-13
- Burghout, W., Rigole, P. J., & Andreasson, I. J. (2014). Impacts of shared autonomous taxis in a metropolitan area. Paper presented at the TRB annual meeting, Washington D.C.
- Burns, L. D., Jordan, W. C., & Scarborough, B. A. (2013). Transforming personal mobility. New York, NY: Earth Institute Columbia University.
- Chen, D., Hanna, J., & Kockelman, K. M. (2016). Operations of a shared, autonomous, electric 36 vehicle (SAEV) fleet: Implications of vehicle & charging infrastructure decisions. Transport Research Part A, 94, 243–254.
- Chen, D., & Kockelman, K. M. (2016). Management of a shared autonomous electric vehicle fleet. Transportation Research Record: Journal of the Transportation Research Board, 2572, 37–46. doi: 10.3141/2572-05
- Childress, S., Nichols, B., Charlton, B., & Coe, S. (2015). Using an activity-based model to explore possible impacts Of automated vehicles. Paper presented at the TRB annual meeting.
- Correia, G., Milakis, D., van Arem, B., & Hoogendoorn, R. (2015). Vehicle automation and transport system performance. In M. Bliemer, C. Mulley, & C. J. Moutou (Eds.), Handbook on transport and urban planning in the developed world (pp. 498–516). Cheltenham: Edward Elgar.
- Correia, G., & van Arem, B. (2016). Solving the user optimum privately owned automated vehicles assignment problem (UO-POAVAP): A model to explore the impacts of self-driving vehicles on urban mobility. Transportation Research Part B, 87, 64–88. doi: 10.1016/j.trb.2016.03.002
- Cyganski, R., Fraedrich, E., & Lenz, B. (2015). Travel-Time valuation for automated driving: A Use-case-driven study. Paper presented at the TRB annual meeting.
- European Commission. (2016). Gear 2030 discussion paper. Roadmap on highly automated vehicles. Directorate-general for internal market, industry, entrepreneurship and SMEs.
- Fagnant, D. J., & Kockelman, K. M. (2014). The travel and environmental implications of shared autonomous vehicles, using agent-based model scenarios. Transportation Research Part C: Emerging Technologies, 40, 1–13. doi: 10.1016/j.trc.2013.12.001
- Fagnant, D. J., & Kockelman, K. M. (2016). Dynamic ride-sharing and fleet sizing for a system of shared autonomous vehicles in Austin, Texas. Transportation. doi: 10.1007/s11116-016-9729-z
- Fagnant, D., Kockelman, K. M., & Bansal, P. (2015). Operations of shared autonomous vehicle fleet for Austin, Texas, market. Transportation Research Record: Journal of the Transportation Research Board, 2536, 98–106. doi: 10.3141/2536-12
- Friedrich, M., & Hartl, M. (2016). Schlussbericht MEGAFON – Modellergebnisse geteilter autonomer Fahrzeugflotten des öffentlichen Nahverkehrs. Stuttgart: University of Stuttgart, Institute for Road and Transport Science.
- Gelauff, G., Ossokina, I., & Teulings, C. (2017). Spatial effects of automated driving: Dispersion, concentration or both. The Hague: KIM – Netherlands Institute for Transport Policy Analysis.
- Gucwa, M. (2014). Mobility and energy impacts of automated cars. Analysis using MTC travel model One. Paper presented at the automated vehicle symposium.
- Hansen, W. G. (1959). How accessibility shapes land Use. Journal of the American Institute of Planners, 25(2), 73–76. doi: 10.1080/01944365908978307
- Hawkins, J., & Habib, K. N. (2018). Integrated models of land use and transportation for the autonomous vehicle revolution. Transport Reviews.
- Heilig, M., Hilgert, T., Mallig, N., Kagerbauer, M., & Vortisch, P. (2017). Potentials of autonomous vehicles in a changing private transportation system – a case study in the Stuttgart region. Transportation Research Procedia, 26, 13–21. doi: 10.1016/j.trpro.2017.07.004
- Hörl, S., Erath, A., & Axhausen, K. W. (2016). Simulation of autonomous taxis in a multi-modal traffic scenario with dynamic demand. Zurich: Institute for Transport Planning and Systems. ETH Zurich.
- ITF (International Transport Forum). (2015). Urban mobility system. Upgrade. How shared self-driving cars could change city traffic. Corporate Partnership Board Report. OECD.
- Jones, P. M., Dix, M. C., Clarke, M. I., & Heggie, I. G. (1983). Understanding travel behaviour. Aldershot: Gower.
- Kasraian, D., Maat, K., Stead, D., & van Wee, B. (2016). Long-term impacts of transport infrastructure networks on land-use change: An international review of empirical studies. Transport Reviews, 36(6), 772–792. doi: 10.1080/01441647.2016.1168887
- Kim, K., Rousseau, G., Freedman, J., & Nicholson, J. (2015a). The travel impact of autonomous vehicles in metro Atlanta through activity-based modeling. Paper presented at the 15th TRB national transportation planning applications conference.
- Kim, K. H., Yook, D. H., Ko, Y. S., & Kim, D. H. (2015b). An analysis of expected effects of the autonomous vehicles on transport and land use in Korea. Anyang: New York University, Marron Institute of Urban Management.
- Kitamura, R. (1988). An evaluation of activity-based travel analysis. Transportation, 15, 9–34. doi: 10.1007/BF00167973
- Kornhauser, A. L. (2013). Smart driving cars: Transit opportunity of NHTSA level 4 driverless vehicles. Paper presented at the TRB automated vehicle workshop.
- Kröger, L., Kuhnimhof, T., & Trommer, S. ( in press). Does context matter? A comparative study modelling autonomous vehicle impact on travel behaviour for Germany and the USA. Transportation Research Part A.
- Levin, M. W., Kockelman, K. M., Boyles, S. D., & Li, T. (2017). A general framework for modeling shared autonomous vehicles with dynamic network-loading and dynamic ride-sharing application. Computers, Environment and Urban Systems, 64, 373–383. doi: 10.1016/j.compenvurbsys.2017.04.006
- Le Vine, S., Zolfaghari, A., & Polak, J. (2015). Autonomous cars: The tension between occupant experience and intersection capacity. Transportation Research Part C: Emerging Technologies, 52, 1–14. doi: 10.1016/j.trc.2015.01.002
- Liu, J., Kockelman, K. M., Boesch, P. M., & Ciari, F. (2017). Tracking a system of shared autonomous vehicles across the Austin, Texas network using agent-based simulation. Transportation. doi: 10.1007/s11116-017-9811-1
- Llorca, C., Moreno, A. T., & Moeckel, R. (2017). Effects of shared autonomous vehicles on the level of service in the greater Munich metropolitan area. Paper presented at the international conference on intelligent transport systems in theory and practice, Mobil.TUM, Munich.
- Martinez, L. M., & Viegas, J. M. (2017). Assessing the impacts of deploying a shared elf-driving urban mobility system: An agent-based model applied to the city of Lisbon, Portugal. International Journal of Transportation Science and Technology, 6, 13–17. doi: 10.1016/j.ijtst.2017.05.005
- Meyer, J., Becker, H., Boesch, P. M., & Axhausen, K. W. (2017). Autonomous vehicles: The next jump in accessibilities? Research in Transportation Economics, 62, 80–91. doi: 10.1016/j.retrec.2017.03.005
- Milakis, D., van Arem, B., & van Wee, B. (2017). Policy and society related implications of automated driving: A review of literature and directions for future research. Journal of Intelligent Transportation Systems, 21(4), 324–348. doi: 10.1080/15472450.2017.1291351
- Moreno, A. T. (2017, April 10–13). Autonomous vehicles: Implications on an integrated land-use and transport modelling suite. Paper presented at the 11th AESOP young academics conference in Munich.
- SAE International. (2018). Surface vehicles recommended practice. J3016. Taxonomy and Definitions for Terms Related to Driving Automation Systems for On-Road Motor Vehicles.
- Spieser, K., Treleaven, K., Zhang, R., Frazzoli, E., Morton, D., & Pavone, M. (2014). Toward a systemic approach to the design and evaluation of automated mobility-on-demand systems: A case study in Singapore. In G. Meyer, & S. Beiker (Eds.), Road vehicle automation. Lecture notes in mobility (pp. 229–245). Cham: Springer.
- Stocker, A., & Shaheen, S. (2017). Shared automated vehicles. A review of business models. International Transport Forum Discussion Paper 09/2017.
- Thakur, P., Kinghorn, R., & Grace, R. (2016). Urban form and function in the autonomous era. Australasian transport research forum proceedings.
- Wegener, M., & Fürst, F. (1999). Land-use transport interaction: State of the art. Deliverable D2a of the project TRANSLAND (integration of transport and land use planning). Dortmund: University of Dortmund.
- Zhang, W. (2017). The interaction between land use and transportation in the era of shared autonomous vehicles: A simulation model (Dissertation). Georgia Institute of Technology
- Zhang, W., & Guhathakurta, S. (2017). Parking spaces in the age of shared autonomous vehicles Transportation Research Record: Journal of the Transportation Research Board, 2651, 80–91. doi: 10.3141/2651-09
- Zhang, W., Guhathakurta, S., & Fang, J. (2015). Exploring the impact of shared autonomous vehicles on urban parking demand: An agent-based simulation approach. Sustainable Cities and Society, 19, 34–45. doi: 10.1016/j.scs.2015.07.006
- Zhang, W., Guhathakurta, S., & Khalil, E. B. (2018). The impact of private autonomous vehicles on vehicle ownership and unoccupied VMT generation. Transportation Research Part C: Emerging Technologies, 90, 156–165. doi: 10.1016/j.trc.2018.03.005
- Zhao, Y., & Kockelman, K. M. (2017). Anticipating the regional impacts of connected and automated vehicle travel in Austin, Texas. Paper presented at the TRB annual meeting.