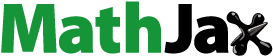
ABSTRACT
Governments worldwide are investing in innovative transport technologies to foster their development and widespread adoptions. Since accurate predictions are essential for evaluating public policies, great efforts have been devoted to forecast the potential demand and adoption times of these innovations. However, this proves to be challenging, and it often fails to deliver accurate predictions. Learning a lesson to guide future work is critical but difficult because forecast figures depend on modelling methods and assumptions, and exhibit a great variability in methodologies, data and contexts. This paper provides a critical review of the models and methods employed in the literature to forecast the demand for electric vehicles (EVs), with a focus on the methods for incorporating choice behaviour into diffusion modelling. The review complements and extends previous works in three ways: (1) it focuses specifically on the ways in which fuel type choice has been incorporated into diffusion models or vice-versa; (2) it includes a discussion on forecast accuracy, contrasting the predictions with the actual figures available and estimating an average root mean square error and (3) it compares models and methods in terms of their strengths and limitations, and their implications in forecasting accuracy. In doing that, it also contributes discussing the literature published between 2019 and 2021. The analysis shows that EV demand estimation requires solving the non-trivial issue of jointly modelling the factors that induce diffusion in a social network and the instrumental and psychological elements that might favour household adoption considering the available alternatives. Mixed models that integrate disaggregate micro-simulation tools to capture social interaction and discrete choice models for individual behaviour appear as an interesting approach, but like almost all methods analysed failed to deliver satisfactory results or accurate predictions even when using sophisticated modelling techniques. Further improvement in various components is still needed, in particular in the input data, which regardless of the method used, is key to the accuracy of any forecasting exercise.
1. Introduction
In an age of major technological advances, the transport market is rapidly evolving, with countless technological innovations enabling better use of energy, more environmentally friendly, safe and efficient behaviours, as well as significant changes in everyday mobility. The recent upraise of innovative technologies represents the most relevant change in the transport industry since the start of the transport “highway era” in the 1940s (Muller, Citation2004), and the main reason why the current time has been deemed “the cusp of a transport revolution” by researchers and stakeholders alike (National Govenors Association, Citation2021).
In general terms, an innovation can be understood as any idea, practice or object that is perceived as new by an individual or group (Rogers, Citation2003). Innovation in transport spans from Electric Vehicles (EVs), Autonomous Vehicles (AVs) and Personal Aerial Vehicles (PAVs), which are based on innovative vehicle technologies, to shared and connected transport systems such as mobility-as-a-service (MAAS), ride-sourcing and shared mobility in general, which employ smart technology for their operations, but mostly represent innovative transport concepts.
Governments, mostly in developed countries, are investing in these technologies to foster their development and boost adoptions. Regarding EV, the focus has been on implementing measures such as incentives and tax rebates, support for the deployment of charging infrastructure and fuel economy standards, to reduce the cost gap between EVs and conventional vehicles (International Energy Agency, Citation2019). For example, in 2011, the UK government introduced the “plug-in car grant” scheme to support the purchasing of ultra-low emission vehicles (Roberts, Citation2020). Originally set at £5000 for all eligible vehicles, it was reduced to £3500 for EVs and eliminated for plug-in hybrid EVs in 2018 (Suzuki, Citation2020). The scheme supported the purchase of more than 200,000 ultra-low emission vehicles at a cost of about £800 million, yet these vehicles only represent less than 2% of the vehicle stock in the UK (Roberts, Citation2020).Footnote1 A similar scheme implemented by the Chinese government in 2009 reimbursed buyers with roughly £2000 per EV purchase, although this was reduced to about £1600 in 2019 (Barrett, Citation2021), and helped China to become the largest market for EV, accounting for about 50% of global sales. The overall market share of EVs in China, however, is below 2%. Similar cases can be observed in European countries such as Denmark and the Netherlands (Suzuki, Citation2020), while in the US, the Federal Government adopted income tax credits for up to US$7500 for the first 200,000 EVs sold, for a total expenditure of about US$1500 million (Winston, Citation2021).
Given these investments, considerable effort has been devoted to forecast the potential demand for transport innovations and their adoption times. Accurate predictions are a necessity, not only to understand and predicting trends, but also for the formulation and evaluation of policy measures. However, predicting the demand for innovations proves to be particularly challenging. Forecast figures depend greatly on modelling methods and assumptions, and a great variability in methodologies, data and contexts exists. In this context, comparing results is difficult, and trying to use these results to make policy recommendation is risky.
This paper aims to provide a critical review of the methods employed in the literature to forecast the demand for transport innovation, attempting to understand the validity of their predictions and recommendations. While it is difficult to clearly identify which method is better to obtain robust forecasts in all circumstances, our focus is on analysing the strengths and weaknesses of each modelling framework, identifying the elements that might contribute to a more accurate representation of the transport diffusion innovation problem. The review focuses on the forecast of EVs for two reasons: because much more research is available for EVs than for the other “newer” innovations and, more importantly, because the EV market is the most mature and consolidated among all the innovations, allowing an interesting comparison between forecasts and actual market penetration. It is worth mentioning that even if the basic EV technology has existed for over 100 years, EVsFootnote2 – and alternative fuel vehicles in general – have experienced a resurgence in the last decade or so (International Energy Agency, Citation2019). Nevertheless, they can still be considered an “innovation”, because, with few exceptions (notably Norway with a market share of over 17% and Iceland with 6%; International Energy Agency, Citation2021), their market shares are still marginal, and the product is still under significant development. We concentrate on personal vehicles, attempting to understand the purchase behaviour from a consumer perspective, and explicitly excluding the commercial vehicle market, which operates under different criteria and must be analysed with different assumptions.
There are already excellent reviews on modelling methods for forecasting the EV market. Coffman et al. (Citation2017) identify factors that affect EV adoption, while Liao et al. (Citation2017) and Kumar and Alok (Citation2020) focus on the attributes that consumers consider relevant when choosing an EV in stated preference (SP) experiments. These three articles mainly concentrate on the substitutional aspect of the EV adoption process – i.e. the choice of EVs among other fuel alternatives. On the other hand, Al-Alawi and Bradley (Citation2013), updated later by Jochem et al. (Citation2018), provide a detailed revision of the methods used to model the diffusion of EVs adoption, focusing on methodological backgrounds and data requirements. Our review complements and extends previous works in three ways: (1) it focuses specifically on the ways in which choice has been incorporated into diffusion models or vice-versa, and hence providing a substantially different literature compared to Jochem et al. (Citation2018),Footnote3 (2) it includes a discussion on forecast accuracy, contrasting the predictions with the actual figures available and estimating an average root mean square error and (3) it compares models and methods in terms of their strengths and limitations, and their implications in forecasting accuracy. In doing that, it also contributes discussing the literature published between 2019 and 2021.
The paper is organised as follows. Section 2 provides a description of the methodology followed to review the relevant literature. Sections 3–5 review models and methods to incorporate choice behaviour into EV diffusion modelling: bottom-up models, top-down models and mixed models, respectively. Section 6 provides a discussion on forecast accuracy, model validation and some key points for further research.
2. Review criteria and article classification
In line with the objective of this review, only articles that simultaneously contain a disaggregated model of individual behaviour (choice) and a model of the aggregate effects of these choices in the network (diffusion) were considered in the analysis. We started by analysing the articles in the reviews by Al-Alawi and Bradley (Citation2013) and Jochem et al. (Citation2018), as well as their respective cited references, using a forward snowballing approach (Van Wee & Banister, Citation2016). This initial screening process yielded 88 candidate articles to review. We continued with searches in the Scopus database using the keywords “electric vehicle AND choice model”, “electric vehicle AND diffusion” and “electric vehicle AND preferences”. These generated a list of 1273 additional articles, which was later refined to include only articles published in peer-reviewed journals or conference proceedings, and then screened by titles and abstracts, reducing the list to 134. From the list of 88 + 134 candidate articles, 168 containing choice experiments without a diffusion component, or diffusion models without treatment of consumer choice, were excluded.Footnote4 54 articles were finally included in the review. We followed the scheme in Al-Alawi and Bradley (Citation2013) and Jochem et al. (Citation2018), and classified them by their main modelling method, into bottom-up, top-down and mixed models:
Bottom-up models (29 articles) adopt an individual perspective in a simulation environment, where behavioural rules are implemented at the individual level such that diffusion occurs as a product of social interaction.
Top-down models (18 articles) operate from an industry perspective, combining an aggregate framework for the diffusion component, with a disaggregate method (generally discrete choice models) for the substitutional effect.
Mixed models (5 articles) combine the bottom-up and top-down approaches in a systematic manner. They integrate aggregate diffusion models with agent-based models, taking advantage of both methods.
This rough classification scheme is based on the main modelling approach employed in each article, and as such, it is not rigid. The scheme offers a tool to understand the many nuances involved in modelling this complex problem and the techniques used to tackle them.
presents a summary of the 54 articles analysed, including information about the behaviour rules and the social interaction models adopted, as well as the data source, the country where the studies were applied, and the sample data period, which usually corresponds to the reported data collection dateFootnote5 These aspects are further explored in the following sections.
Table 1. Summary of EV forecasting models.
3. Bottom-up models
Two main bottom-up frameworks have been implemented to model EV diffusion. Most articles use agent-based modelling, while some recent applications work with evolutionary game theory. Both approaches are analysed in this section.
3.1. Agent-based models
Agent-based models (ABM) are simplified virtual representations of complex systems – in this context, the social system where the innovation is diffused – constructed to study, from a bottom-up perspective, the system’s properties that emerge from social interactions. The unit of analysis is thus the agent, a software entity capable of flexible and autonomous action according to certain pre-established “rules” (Nikolic & Kasmire, Citation2013) which determine its decision-making and its interaction with other agents in the system. ABM offer an interesting methodology to account for the social component of EV diffusion, as individual decisions can be designed to depend on the interaction between several agents and the social network. The flexibility of the ABM makes them particularly suitable to test the effect of economic variables but also to analyse the effect of public attitudes and awareness, provided that a solid theoretical approach is used to ground agent behaviour. However, the models can become very complex and often simplifications are made that might diminish their benefits when used for forecasting purposed.
3.1.1. General modelling framework
The EV system is intrinsically multi-agent, as individual purchase behaviour – the demand side – depends strongly attributes of the supply side (price and other costs, driving range) and on other aspects such as charging, fuelling and maintenance infrastructure which are regulated and hence determined by government policies. However, modelling simultaneously several agents with different goals and cognitions is very complex and most of the ABM works focus on consumer adoption behaviour (i.e. single-agent models) assuming that both supply and policy measures are exogenous variables. This might be generally true for small demand increments – like those occurring during the first diffusion stages – but a greater EV demand might require relevant changes from EV suppliers, as well as from authorities and energy providers. In this case, the more complex multi-agent models could be useful to understand the interactions that drive diffusion. Only 8 articles analysed include agents representing the supply side of the EV market, i.e. vehicle manufacturers and dealers, who react to actions of other agents with their own profit maximising objective functions. In these multi-agent models, manufacturer agents can respond to government policies and demand changes by adjusting engine types, fuel economy, vehicle types and prices. These models are usually simplified representations of complex agent relationships and might suffer from lack of realism especially when data input is limited.
3.1.2. Individual decision rules
The individual behavioural rules for consumer agents represent the core of ABM and are key for forecasting policies, because they set the nature of a decision process that involves defining if the purchase of a vehicle is required – either to add a new vehicle to the household, or to replace an already existing one – and then choosing the specific vehicle to buy among a set of – possibly – many alternatives. Roughly three types of decision rules have been used: discrete choice models (DCM), ad-hoc behavioural rules and other approaches.
3.1.2.1. Discrete choice models
Discrete choice models (DCM) represent arguably the approach most used in transport (but also other fields) to reproduce the behavioural process that leads to the agent’s choice. DCM take a causal perspective, assuming that there are factors that collectively determine, or cause, the agent’s choice. Some of these factors are observed by the researcher and some are not. The treatment of the unknown factors as random variables leads to the probabilistic outcome of the DCM (Train, Citation2009).
Given the “popularity” of DCM, these would appear as an obvious method to be used to simulate the agent decision rules within an ABM. Surprisingly, DCM are only employed as behavioural rule in 9 of the 29 ABM articles analysed. As shown in , 5 of these articles adopt a simple multinomial logit (MNLFootnote6) model. Other chosen specifications include nested logit (Cui et al., Citation2010); mixed multinomial logit (Brown, Citation2013; Tran, Citation2012) and Bayesian MNL with varying coefficients (Zhang et al., Citation2011).
In their usual specifications, DCM are static, meaning that their parameters do not change over time. Different adjustments have been adopted to account for the dynamic nature of the diffusion process, i.e. the positive impact of the social network on the probability of buying EVs. While Zhang et al. (Citation2011) and Cui et al. (Citation2010) capture this effect by expressing the coefficients as a function of effects like word of mouth or previous knowledge of the innovative technology, most articles include in the DCM a willingness to consider (WtC) variable, first proposed by Struben and Sterman (Citation2008), which multiplies the utility of the EV alternative and is most commonly assumed as varying between 0 and 1 as a function of the number of EV adopters (Noori & Tatari, Citation2016; Onat et al., Citation2017; Shafiei et al., Citation2012; Sen et al., Citation2017). This approach has been designed with a practical focus, i.e. the resulting demand curve must follow the typical S-shaped diffusion curve. The methods used to achieve this are extremely diverse, and as such it is difficult to make any judgement on their theoretical soundness and robustness. In addition, as will be seen later, few of these methods have been used to produce forecasts, making a detailed accuracy-based comparison also unfeasible.
In all these applications, parameters are estimated exogenously from the ABM. By construction, this also means that the coefficients do not vary throughout the simulation process. Interestingly, only Zhang et al. (Citation2011) – relying on a SP experiment – and Brown (Citation2013) – with revealed preference information from a national transport survey – estimate their choice models as part of their ABM setup. In the remaining articles, the coefficients are imported from external sources. This might be a disadvantage, especially if parameters from the population of interest are not available and external samples are used (Shafiei et al., Citation2012, Citation2013). In addition, the modelling framework might not be entirely appropriate for the nature of the data used for estimation; for example, if MNL models are estimated with SP data, this could translate into biased standard errors for the estimated parameters (Ortúzar & Willumsen, Citation2011).
3.1.2.2. Ad-hoc behavioural rules
The remaining 20 ABM articles model EV choice with simplified heuristics that attempt to incorporate most of the behavioural elements that research has found to be relevant for EV adoption (Sovacool, Citation2017). The heuristics used can be classified in question-based rules, utility-based rules and cost minimisation rules.
Question-based rules, used in 6 articles, imply agents responding to a set of questions at each time step. These questions are used to decide first if it is necessary to buy a new vehicle and then to determine whether costs, benefits and desirability of the available alternatives reach the threshold of WtC above which adoption will occur.
Utility-based rules, used in 6 articles, entail agents evaluating a certain deterministic utility function at each time step. These cannot be considered choice models as their utilities are treated as a deterministic quantity – generally a linear combination of several attributes – to be maximised by the consumer, without including a random component. While in most cases the utility functions only consider socioeconomic variables and vehicle cost and performance attributes, the utility functions in Klein et al. (Citation2020) and Buchmann et al. (Citation2021) also include EV awareness and social network characteristics, respectively.
Cost minimisation strategies, used in 6 articles, implement a behavioural rule that establishes the monetary component as the ultimate determinant of adoption, even when considering other relevant attributes.Footnote7 The methods usually involve agents screening for available cars within their budget window, scoring them according to size, performance (Choi, Citation2016), brand preferences (Mock et al., Citation2009; Sullivan et al., Citation2009) and social influence (Ramsey et al., Citation2018; Sweda & Klabjan, Citation2011) and choosing the alternative with the highest score.
Ad-hoc behavioural rules are tailored to each specific problem. Although logic and valid, these rules lack a verified theoretical framework, that supports the behavioural assumption. Models based on a well-documented behavioural theory, with known assumptions, implications and limitations, should always be preferred over ad-hoc-methods, whose behavioural foundations are not theoretically grounded, however logical they might look. For this reason, the use of the DCM paradigm (as discussed in section 3.1.2.1) is preferable, because it is based on the random utility theory, which provides a broadly verified and tested model of individual choice behaviour (McFadden, Citation2000).
3.1.2.3. Other approaches
All articles discussed so far model individual vehicle choice mostly based on instrumental or functional EV attributes, such as purchase cost, operation cost, vehicle size, or driving range, sometimes considering socioeconomic and spatial attributes, while social influence is embedded in the ABM framework. Differently, Kangur et al. (Citation2017) include social influence using the Consumat framework devised by Jäger (Citation2000), where action depends on constant evaluations of satisfaction and certainty towards transport needs. Wolf et al. (Citation2015) uses the hot coherence model proposed by Thagard (Citation2006) where agents decide by maximising the coherence of their current beliefs and emotions, subject to interactions with other agents. The decision to adopt is mostly driven by the evolution of emotions and beliefs. However, the outcomes of the model are behavioural intentions, i.e. no choice probabilities or market shares are estimated.
3.1.3. Agent attribute and model parameter sources
Originally, ABM were largely conceptual and designed to understand complex social systems, rather than making meaningful predictions (Zhang & Vorobeychik, Citation2019). ABM were seen as “toy models” unrepresentative of real phenomena (Garcia & Jäger, Citation2011) and accused of lacking realism. However, among the articles analysed in this review, only Sullivan et al. (Citation2009), Pellon et al. (Citation2010) and Tran (Citation2012) work with simulation exercises not based on real population data. All the other articles analysed in this review are grounded on data that characterises real populations. While many works rely specifically designed surveys, Cui et al. (Citation2010), Querini and Benetto (Citation2014), McCoy and Lyons (Citation2014) and Choi (Citation2016) gather information from large scale transport surveys. In these cases, the social network is built by creating synthetic populations derived from both the disaggregate survey variables and aggregate distributions of population attributes (Jeong et al., Citation2016).
ABM offer an interesting methodology to account for the social component of EV diffusion, as individual decisions can be designed to depend on the interaction between the agent and the social network. Social influence and norms, network effects, social communication and the effects of advertisement on innovation diffusion are often included as part of the decision process. Multi-agent models should be appropriate to model all the stages in the diffusion process; however, they require disaggregate information about the vehicle supply market, the energy sector and the government, and their mutual interactions, to define realistic behavioural rules. As these relationships are extremely complex and depend on multiple factors that are difficult to analyse and predict, there is a risk of behavioural oversimplification, especially when detailed information is limited or unavailable.
It is expected that data availability and quality play a relevant role in the robustness and accuracy of the forecasts. In general terms, models with a strong focus on the demand side require a large amount of data at a disaggregate level for a proper characterisation of agents and their social networks, and thus they are more prone to bias due to low-quality input data or unrepresentative samples. Models that consider both demand and supply depend on several sources of disaggregate and aggregate data to calibrate the parameters in their sub-models. In this case, the intricate interrelation between inputs and outputs of the sub-models makes the general models more prone to error propagation due to the combination of several sources of data. These effects should be considered to ensure reproducibility and accuracy.
3.2. Evolutionary game theory models
Evolutionary game theory (EGT) (Smith, Citation1982) combines game theory with dynamic evolution process analysis. It models the interrelation between actors with multiple – and possibly diverging – interests in complex systems where individual preferences are influenced by actions performed by actors from other sectors Encarnação et al. (Citation2018). The method requires an explicit mathematical definition of both the individual strategies and their expected outcomes. The purpose is to describe how these strategies are adjusted to the current situation in a dynamic process (Li et al., Citation2019). The simulation (game) is constructed so the combination of all the agents (players) using their best response strategy leads to a certain equilibrium.
Very few EGT applications have been set up to study EV diffusion, and all refer to cases of multiple stakeholders including manufacturers. Both Li et al. (Citation2019) and Hu et al. (Citation2020) study EV diffusion in China and conclude, based on their simulations, that production subsidies for manufacturers have better effects on EV diffusion than consumer purchase subsidies.
While the EGT scheme might be useful to understand the roles of stakeholders and their strategies in the system in a controlled fashion, the requirement that the strategies and outcomes must be defined with closed mathematical expressions is only feasible in very idealised situations – uniformly connected networks, deterministic behaviour, infinite population size – which are scarcely found in the real world. This makes the method rather unrealistic in most applications. ABM are the preferred way of studying EV diffusion with a bottom-up framework, as they can reveal dynamics resulting from agent interaction in realistic environments.Footnote8
4. Top-down models
Top-down models can be understood as models built from aggregate information about the EV market to model diffusion and incorporating a choice for the substitutional effect. As summarised in , the 18 articles found belonging to this group can be classified into three groups: (1) aggregate market models, (2) system dynamics (SD) models and (3) other top-down approaches that do not belong to any of these two categories.
Top-down models are appropriate when a realistic market representation is required as, compared to the bottom-up approach, they allow testing several scenarios in a more organic manner, where economic effects appear in the system as a product of stakeholder interaction. While all the top-down models reviewed feature a choice component to consider substitutional behaviour, forecasting involves computing choice probabilities that depend on one or more exogenously estimated diffusion parameters such as WtC. This is often one of the weakest components of the diffusion model and might impact forecasting accuracy, as will be discussed below.
4.1. Aggregate market models
Aggregate market models extend the single-market diffusion process based on aggregate economic information (sales, stocks, prices) as originally proposed by Bass (Citation1969, Citation2004), by using mostly MNL to account for the substitution among products based on the vehicle characteristics. Bass-like models supplemented by MNL models are used in Mau et al. (Citation2008), Jun and Kim (Citation2011) and Higgins et al. (Citation2012). Jensen et al. (Citation2017), on the other hand, implement an advanced choice model, where the disaggregate utility parameters are estimated exogenously but the alternative specific constants and scale of the model are estimated jointly with a Bass-like diffusion model and hence adjusted to observed market shares. This method allows the improvements on EV attributes not to be overshadowed by the effect of constants that constrain the model to the low demand in the early market.
Notwithstanding their parsimony – they can be described using a few simple closed-form expressions and relatively few aggregate data are required for estimation, which can be useful for a quick assessment – aggregate market models have been criticised because they assume a fully connected social network and the shape of the diffusion curve remains constant throughout the process (Peres et al., Citation2010). These models have a very rigid structure, hence the forecasts depend more on the assumptions and the specific parameters of the models than on demand and supply response to market conditions. This can greatly undermine their realism.
4.2. System dynamics models
System dynamics (SD) is a method of studying the behaviour of complex systems over time (Forrester, Citation1962). Differently from the aggregate methods discussed in the previous section, SD is essentially a simulation tool that uses aggregate variables such as feedbacks, flows, stocks and time delays to model the behaviour of the system over time and DCM to model the substitution effect. By explicitly accounting for the dynamic nature of the process, SD considers the interactions of multiple stakeholders.
The most widely employed SD framework to model transport innovations is the theoretical model proposed by Struben and Sterman (Citation2008), which consists of three main elements: a fleet turnover model (for manufacturers), a DCM of the car purchase decision (for consumers) and a technology/social diffusion process. Social network effects are included in the choice model via a WtC indicator, which depends on feedback from consumers’ experience, word of mouth, marketing and the number of adopters in the network. This framework has been combined with DCM in Shepherd et al. (Citation2012) and Oliveira et al. (Citation2019) who estimate a MNL model using data from SP surveys. While the method is solid and behaviourally consistent, it is not clear how the parameters from the technology/social diffusion component might be estimated, and this represents an important disadvantage, especially in terms of model reproducibility and applicability to different context.
Some articles have adopted other SD frameworks to model EV diffusion, with several market-specific assumptions adopted to varying degrees of behavioural realism. Exogenously estimated choice models are integrated with simplified representations of the market in BenDor and Ford (Citation2006), Mazur et al. (Citation2018) and Kong et al. (Citation2020) and with SD models that still include a Bass-like diffusion curve in de Santa-Eulalia et al. (Citation2011), while more comprehensive market representations are supplemented with simplified choice models in Kong et al. (Citation2020) and Keith et al. (Citation2020).
Compared with aggregate market models, SD relies on more complex representations of the relationship between several system actors, including multiple interactions that are absent from the simplified approach. The detailed consideration of these effects is a welcome feature for models that deal with complex systems. This complexity, however, comes at the cost of increasing information requirements and involving a series of microeconomic and behavioural assumptions whose validity is not always verified. In addition, as with other top-down methods, the focus is on the aggregate representation of stakeholders, and the choice component of these models is usually modelled with a lower level of detail than in bottom-up approaches. The lack of historical datasets for proper model estimation is usually overcome by importing parameters from SP surveys or external studies, which might affect consistency and forecasting accuracy.
4.3. Other top-down approaches
Two additional groups of approaches cannot be included in the two categories previously defined. A first group of models (which we call simulation models) considers specifications for the aggregate diffusion model that differ from Bass-like curves or SD simulations and include a choice component. Plötz et al. (Citation2014) model EV diffusion with an aggregate model that simulates individual driving profiles for which a utility-maximising vehicle option is chosen among the available alternatives. Schmelzer and Miess (Citation2015) propose a simulated general equilibrium model that incorporates a MNL model of vehicle type choice, using a nationwide transport survey, with alternative specific constants calibrated to match aggregate figures. Finally, Liu and Lin (Citation2017) estimate choice probabilities for vehicle technologies using a nested logit model, which are then then used to calculate market shares, vehicle sales and stock. Diffusion occurs as a result of attribute evolution over time As with SD, strong assumptions might mean that behaviour models are oversimplified because the emphasis is on economic interactions at the macro level.
A second group of models (which we call modified choice models) implement the dynamic nature of the diffusion problem inside a DCM without relying on an aggregate market model. Liu and Cirillo (Citation2018) estimate a joint car ownership/use model over a 9-year period. This paper is unique in considering the effect of multi-car household and second-hand vehicle purchases. Tchetchik et al. (Citation2020) instead combine concepts from Rogers’ diffusion of innovations model (Rogers, Citation2003) with the theory of multiple goal framing (Lindenberg & Steg, Citation2007), which suggests two main adoption motivators: product attributes and individual attitudes and traits. This study offers an interesting framework to study the effect of psychological traits in EV diffusion. However, as in Liu and Cirillo (Citation2018), diffusion is only simulated via scenarios and the social dimension is not considered. Archsmith et al. (Citation2021) estimate a Probit model that predicts vehicle class market shares, with a calibrated parameter called “intrinsic growth rate” driving the diffusion process. Similarly, Brand et al. (Citation2017) model EV diffusion using a MNL model where the network effect is captured by the alternative-specific constant, which the authors estimate for several consumer segments, and adjust over simulation time. Further work is required to include the social dimension in these choice modelling approaches. At their current state, its exclusion represents a major drawback.
5. Mixed models
Mixed models use aggregate (most frequently, SD) models for the interaction between vehicle supply, charging infrastructure and market shares, disaggregate ABM to capture social interaction and DCM for individual behaviour. These approaches are relatively new, and as shown in , our search found only five articles in this category.
Both Shafiei et al. (Citation2013) and Yang et al. (Citation2015) use a MNL with a WtC parameter to model vehicle choice inside their ABM. They differ in the SD component, as Shafiei et al. (Citation2013) include consumers, the government, the energy supply system, fuel/charging stations, car importers/dealers, car manufacturers, vehicles and consumers, while Yang et al. (Citation2015) consider vehicle demand, policy, electricity pricing and EV evolution. Kieckhäfer et al. (Citation2014), on the other hand, implement a hybrid simulation approach to estimate EV diffusion, with choice probabilities estimated using a nested logit model with a WtC parameter. Finally, Pasaoglu et al. (Citation2016) combine a comprehensive representation of vehicle technology transition in the European Union with the socio-technological model by Struben and Sterman (Citation2008), and a MNL model estimated to assess consumer preferences.
Mixed models are an interesting tool for integrating choice and diffusion, as they maintain the market focus of top-down models, while incorporating ABM to improve behavioural characterisation. If detailed information is available from both the demand and the supply side, they offer a more realistic representation of the system and its interactions. There is still room for further improvement in both the diffusion and the substitution components. Choice models should incorporate more complex structures and include attributes such as social influence, individual psychological traits and agent communication. In addition, a realistic representation of economic relations between market stakeholders – authorities, vehicle producers and sellers, the energy sector – requires increasing data acquisition and processing efforts, considering public and private sources available.
6. Method comparison and perspectives for model integration
This article has discussed several methods to predict EV diffusion, providing a breakdown of their advantages and shortcomings. The following sections present a comparative analysis of the methods discussed, based on validation techniques, and forecast accuracy compared to real figures. Some comments on the integration of diffusion and substitution models are provided in the final section.
6.1. Model validation methods
One of the greatest challenges with any forecasting model is the validation phase, i.e. evaluating if its assumptions and methods are appropriate for modelling the problem of interest (Nikolic & Kasmire, Citation2013). We study the validation methods used in the reviewed articles following the scheme proposed by Carley (Citation2017), which classifies validation techniques in four groups:
Grounding, or establishing the theoretical reasonableness of the model. All the 52 articles reviewed include storytelling (authors setting a claim for why the proposed model is reasonable), and 49 of them are grounded in terms of initialisation (setting initial model parameters based on real data, where possible). In 7 articles, grounding is extended by including a parameter sensitivity analysis (simple performance evaluation).
Calibration, or the process of tuning model parameters and initial conditions to fit detailed external real data, i.e. data that was not considered for model estimation or simulation. Here, the focus is on the model’s inputs, and the purpose of calibration is to ensure that the model output comes as close to reality as possible. Other than simple grounding, this is the most frequently used validation technique. 12 articles calibrate their parameters using independent historic information – for example, early years not forecast by the model –. In other four cases, calibration is based on model-to-model comparison, i.e. these models are calibrated against results from another – external – simulation model.
Verification, or determining the validity of predictions against a set of real external data, using statistical tests. This usually occurs after calibration and does not involve parameter adjustment, but an overall assessment of the model’s forecasting ability. Hence, the emphasis is in the modelling output. The type of data available determines the level of detail of the verification process (point, pattern, distribution). Four recent articles consider this technique. Model performance is statistically tested against a benchmark model (e.g. a model estimated using data for the same context with different assumptions) in Kangur et al. (Citation2017) and Zhuge et al. (Citation2019), and real-world data in Sen et al. (Citation2017) and Klein et al. (Citation2020).
Harmonisation, or the integration of calibration and verification in a multi-step process using an auxiliary benchmark model. Here, modelling predictions are compared with the predictions of a linear model, to statistically assess the adequacy of the theoretical assumptions and their predictive value over and above that achievable through a simple linear benchmark model (Carley, Citation2017). According to Carley (Citation2017), harmonisation allows measuring the marginal improvement that the non-linear components in comparison to a simple benchmark model, fitted with the same data. As each model component could theoretically be compared with a baseline model, harmonisation could also be a useful tool to locate “areas or assumptions of the model that need to be improved”. This approach is followed in Zhang et al. (Citation2011) and Noori and Tatari (Citation2016). However, some authors do not consider harmonisation worthwhile and do not include it as a relevant step of the validation process (e.g. Ngo & See, Citation2012; Nikolic & Kasmire, Citation2013), possibly because some of its benefits can also be attained by other methods with less implementation difficulties.
Perhaps unsurprisingly, 22 of the 52 articles do not report any validation other than storytelling or initialisation. Only a handful of single-agent ABM include data calibration, and most top-down and mixed models report only a calibration stage to tweak internal parameters. The most detailed validation schemes are found only in the more complex multi-agent approaches. The lack of validation in most of the articles analysed makes it impossible to identify patterns.
It has been argued that in some cases, validation might not be possible due to a lack of proper out-of-sample datasets or benchmark models (Carley, Citation2017). Nevertheless, validation is essential for reproducibility, especially if the model will be used to identify and recommend policy measures, as it is the only method to evaluate its effectiveness in representing the system of interest (e.g., Mabit et al., Citation2015; Parady et al., Citation2021). In addition, as will be discussed, model grounding greatly helps reaching better forecasting accuracy. Efforts should be made to include validation in any innovation diffusion research, as results and conclusions depend on the validity of the modelling tools used.
6.2. Estimated EV adoption versus real figures
To understand the forecasting ability of the models analysed, we studied the subset of 19 articles that include annual forecasts for a clearly defined timeline and geographic context, and for which actual market shares are publicly available.Footnote9 presents the annual market share predictions we could derive from these articles, and the corresponding actual market figures of EV market shares for the same year and population, obtained by retrieving data from the forecast tables, where available, or analysing the relevant plots using the Plot Digitizer app (PlotDigitizer, Citation2022). In all studies, the figures represent the predicted EV market shares, with just two exceptions – Eppstein et al. (Citation2015) predict plug-in hybrid vehicle stock shares only, and Sen et al. (Citation2017) forecast the EV share in terms of annual sales. The actual market share figures were sourced from the International Energy Agency (Citation2021) website and the European Alternative Fuels Observatory (Citation2020). For consistency, we only consider predictions starting one year after the formal publication date.
Table 2. Comparison between predictions and actual figures for some EV diffusion models.
Modellers often work with a range of variation for each relevant parameter and combine their values to create several forecasting scenarios. For each article and scenario, we contrast predictions with actual figures by estimating the average root mean square error , where m is the number of forecasting years, and
and
are the predicted and the actual shares in year i, respectively. We also computed a relative measure of error provide to account for the fact that small error can be more relevant when predicting small quantities. However, in most cases analysed in this paper the market shares are very low numbers, and this can create numerical problems even if the method performs well. With this in mind, our analysis will be based on the absolute errors though the results of the relative measure will also be discussed in some cases.
It is important to note that often the models aim only to test the effect of several policies and economic conditions and do not necessarily try to achieve realistic predictions. Accordingly, only contains the best performing scenario (the one with the minimum RMSE value) for each article.Footnote10
A first striking conclusion of this analysis is that, for all articles, the scenario that best reproduces the actual figures is the most pessimistic one – either the “business as usual” case, or one that assumes more unfavourable conditions for EV diffusion. Even in those cases, predictions almost invariably overestimate the actual market shares. This is also confirmed when analysing the relative measures of error, whose lowest values are also associated with pessimistic or default scenarios, as in Pasaoglu et al. (Citation2016) (mean error of 86.3% in their “petroleum persistence” scenario) and Kangur et al. (Citation2017) (87.0% error in their “default” scenario).
Several factors might play a role in this behaviour. First, while many articles concentrate in evaluating scenarios with increased policies to stimulate EV uptake, their base case scenario usually assumes that these policies will remain “the same” as in the initial forecasting year. The evidence seems to show that, in general terms, this is true for the most relevant EV markets, and in some cases such as China or UK, the incentives have been decreasing over time (Kohn et al., Citation2022). Second, the most optimistic policy scenarios assumed highly increased petrol costs, and/or important reductions in EV purchase prices, neither of which have been observed. While it has been predicted that some EV models might be close to cost parity with their internal combustion counterparts (Santos & Rembalski, Citation2021), EV purchase prices have been, on average, increasing over time, despite the subsidies and rebates available (AutoTrader, Citation2022). As energy and vehicle purchase prices are crucial parameters for scenario building – and they are usually sourced from external sources – these uncertainties will distort forecasts regardless of the modelling method.
A different difficulty is observed when scenarios depend on theoretical parameters that represent modelling assumptions. For example, the best prediction in Brown (Citation2013) is obtained when the agents are assumed to be “reluctant” to the EV alternative (e.g. with a low WtC), and the largest diffusion barrier on Eppstein et al. (Citation2015) is their “technology comfort threshold” – a parameter reflecting “consumer uneasiness” with the PHEV alternative. These parameters are difficult to measure and impossible to predict, and these two studies are notable in testing their influence in the forecasts using scenarios. Carrying out a sensitivity analysis is recommended when working with theoretical parameters. As seen on , ignoring this aspect can greatly affect predictive accuracy (e.g. Choi, Citation2016).
Scenario building is an important tool to process uncertainty and generate a range of predictions where the effect of policy measures and/or economic parameters can be more easily understood. Displaying the uncertainty of each forecast should also be encouraged.Footnote11 As can be seen in the last column of , prediction errors tend to increase with time.Footnote12 As most models work with an S-shape diffusion curve, the forecasts are mostly unable to reproduce results that greatly deviate from this pattern, such as the important spike in EV stock share observed during 2020 in countries like Germany, Iceland and UK (International Energy Agency, Citation2021).
In addition, as shows, the best performing models (mean RMSE less than 1.0%) use a great variety of methods – 3 mixed models, 2 ABM, 1 aggregate market model, 1 modified choice model, 1 SD model. In contrast, the use of unrealistic parameters has a potentially great effect on predictive accuracy (Choi, Citation2016; Higgins et al., Citation2012; Mock et al., Citation2009). Regardless of the approach, the best results seem to be obtained by models well calibrated using real data. Articles that use DCM to forecast EV diffusion obtain a good predictive accuracy, though only when the alternative-specific constants are calibrated using actual market shares, either with an aggregate market model (Brand et al., Citation2017; Jensen et al., Citation2017), or the Struben and Sterman (Citation2008) framework (Oliveira et al., Citation2019; Shepherd et al., Citation2012). Similarly, in their ABM simulation, Querini and Benetto (Citation2015) carry out a detailed calibration approach and ground their model in detailed national statistics, obtaining acceptable simulation results in their most pessimistic scenario, especially during the first years of the simulation. Mixed models seem to offer the best accuracy when combining a realistic market representation in a SD component with an ABM where decision rules are derived from DCM. This is the case for Shafiei et al. (Citation2013) and Pasaoglu et al. (Citation2016), who report an extensive calibration process using real aggregate and disaggregate information. In addition, models that exclusively focus on the demand side tend to assume full availability of models and fuel types, which is not necessarily the case, especially for the newer alternatives. Only models that account also for the supply side can consider this effect and thus offer a more reliable forecast.
While these results might be difficult to generalise, as they greatly depend on the scope, assumptions, scenarios and data available, a better understanding of forecast accuracy can be obtained with a meta-analysis of the predictions. Following similar exercises by Wardman (Citation2012, Citation2014, Citation2022) with transport elasticities, we quantify how the absolute difference between predicted and actual shares varies across the estimates as a function of some relevant attributes of each study. Our dataset is relatively small (299 estimates coming from 19 articles), as it only includes the studies reviewed in above – albeit considering every scenario –. Considering this limitation, shows the results of a simple linear regression modelFootnote13 of forecast errors as a function of study attributes.
Table 3. Meta-analysis of forecasting errors.
This specification controls by scenario type (pessimistic scenarios tend to produce the minimum differences with actual figures), modelling contexts (models estimated for countries with higher population and lower number of cars tend to be more accurate, especially when considering UK and EU data) and forecasting horizon (predictions are more accurate for years closer to article publication). The results show that predictions of overall stock shares are more accurate than sales shares. More importantly, keeping everything else constant, the best approximation is obtained by using mixed models. While bottom-up models show higher average forecasting errors, top-down models seem to deviate further from actual figures, all of which confirms the relevance of accurate disaggregate information for a proper market characterisation from the demand side. It is important to stress in fact that, regardless of the method used, relying on good quality input data is key to the accuracy of any forecasting exercise,
6.3. Diffusion and substitution: towards an integrated behaviour model
A reliable estimation of EV demand requires solving the non-trivial issue of jointly modelling the factors that induce diffusion in a social network and the instrumental and psycho-social elements that favour household adoption considering the available alternatives. We have reviewed several articles that attempt to solve this problem using a large array of methods.
Forecasting variability cannot be easily explained by the information available on each article. Our results provide some insight onto the elements that can contribute to better forecasting indicators, namely:
A theoretically sound representation of individual behaviour is crucial. DCM estimated with context specific disaggregate data seem to deliver better results than top-down approaches that simplify or ignore the individual behaviour dimension.
Mixed approaches perform better than their top-down and bottom-up alternatives, as they include both dimensions of the problem in an integrated framework.
Regardless of the method, calibration is essential to properly ground the model to a specific context. While this might require important efforts in terms of data collection and analysis, the model will be more likely to provide meaningful and reproducible results.
Some authors have proposed general guidelines for a behavioural framework that might explain diffusion and substitution of innovative transport alternatives (Sovacool, Citation2017), including elements from microeconomic, psychological and sociological theories along with transport and energy demand forecasting. Several studies focusing on the substitutional side of the problem have assessed the role of psychological traits such as pro-environmental attitudes, innovative character, car performance anxiety and the symbolic value of the car, in EV choice probabilities (Liao et al., Citation2017). However, these dimensions have seldom been added to the diffusion-substitution models and only a few articles (e.g. Kangur et al., Citation2017; Wolf et al., Citation2015) carry out significant efforts towards their integration. Similarly, while every diffusion model includes a social diffusion component which roughly complies with Rogers’ (Citation2003) innovation diffusion theory, this is usually implemented in a simplified fashion, without a deep analysis of agent interaction and communication. Notwithstanding the important contributions of Struben and Sterman (Citation2008), the communication analysis in Wolf et al. (Citation2015), and the theoretical integration by Sovacool (Citation2017), the communication component of diffusion models remains in need of further research efforts.
It is difficult to think of one unique modelling framework for studying the problem in every context. Cultural, economic and political differences might play a key role in the results of a policy, and these effects must be considered on the modelling stage. In addition, the limited availability of historic data imposes a burdensome restriction on any modelling framework, with data-based approaches lacking a strong behavioural background the most affected. Understanding this problem and obtaining more accurate forecasts requires a multi-targeted approach in an era of fast technologic development, which often causes market uncertainties, modelling frameworks that attempt to integrate all phenomena (psychological, social and economic) should be encouraged, especially if they study the problem using multi-disciplinary approaches. However, considering all phenomena in a single framework is hard. Discovering new influencing factors and integrating new type of data into modelling is also interesting and promising for future research. Qualitative research – scarcely present in the reviewed articles – can be key in providing methods and tools to obtain a more detailed insight into individual and social experiences with new technology adoption, which might then be used for theory building with a wider scope.
Ultimately, robust predictions depend on the proper use of modelling techniques – understanding their strengths and limitations – the use of real-world data for validation and calibration and, notably, a robust and validated theoretical framework. As previously discussed, this is relevant for model reproducibility and for gaining a better understanding of transport diffusion motivators and their interactions. Crucially, theoretical robustness (i.e. the stability of results to variation across context or other baseline assumptions) also plays a key role in providing more accurate forecasts.
Acknowledgments
We would like to thank the editors and reviewers for their valuable comments and suggestions, which helped us to improve the paper. All remaining errors are our responsibility.
Disclosure statement
No potential conflict of interest was reported by the author(s).
Additional information
Funding
Notes
1 This article uses market shares (i.e. the proportion of EVs in the total fleet) as indicators of market penetration, except where explicitly indicated. It must be noted that, the term market share is also interchangeably referred to as penetration rate or adoption rate in the literature.
2 In this article, the EV abbreviation generically refers to electric vehicles, and includes battery electric vehicles, hybrid vehicles and plug-in hybrids. No specific distinction is made between sub-typologies unless required.
3 Jochem et al. (Citation2018)'s review consider 44 articles from 1995 to 2016. Our review includes 11 of these 44 articles (the ones that include both diffusion and substitution) plus 43 additional articles from between 2008 and 2021.
4 Diffusion/substitution studies outside the EV market (e.g. hydrogen or fuel-cell vehicles) and recent works involving electric share vehicles or electric/autonomous vehicles were also excluded from the review.
5 Note that, in 45% of the articles, this information is not provided. Interestingly, in some articles, data were collection concluded several years prior to article publication, which might be a slight concern in terms of parameter validity.
6 “In a deep sense, the ultimate goal of the researcher is to represent utility well enough that a logit model is appropriate (i.e. that the only remaining aspects constitute simply white noise) Seen in this way, the logit model is the ideal rather than a restriction” (Train, Citation2009, pp. 35–36). However, arguably noDCM is specified well enough for this to be true.
7 The cost minimisation technique is analogous to the utility maximisation expressed in monetary terms.
8 An interesting comparison of ABM and EGT can be found on Adami et al. (Citation2016).
9 We excluded theoretical modelling exercises, forecasts without a clearly defined timeframe and/or geographic context, predictions without a reliable source for comparison, articles without detailed yearly forecasts, and recent articles for which benchmark figures are not yet available.
10 Note that four articles present detailed results for one scenario only.
11 We could not consider uncertainty in predictions of expected market shares, as suggested by one reviewer, because the necessary information to perform these analyses is generally unavailable, with only Querini and Benetto (Citation2015) and Kangur et al. (Citation2017) providing confidence intervals for their predictions.
12 We studied the possibility of defining an “acceptable accuracy”, as suggested by one reviewer. However, we believe this should be evaluated in a case-by-case scenario. For example, the relative error in Brown (Citation2013) is 50%, but the author obtains an average RMSE of 0.4%, which means that he correctly predicted a small increase in adoption rates 8 years after the forecast.
13 More complex specifications were tested but the basic linear model was deemed to have the better level-of-fit and significance.
References
- Adami, C., Schossau, J., & Hintze, A. (2016). Evolutionary game theory using agent-based models. Physics of Life Reviews, 19, 1–26. https://doi.org/10.1016/j.plrev.2016.08.015
- Adepetu, A., Keshav, S., & Arya, V. (2016). An agent-based electric vehicle ecosystem model: San Francisco case study. Transport Policy, 46, 109–122. https://doi.org/10.1016/j.tranpol.2015.11.012
- Al-Alawi, B. M., & Bradley, T. H. (2013). Review of hybrid, plug-in hybrid, and electric vehicle market modeling studies. Renewable and Sustainable Energy Reviews, 21, 190–203. https://doi.org/10.1016/j.rser.2012.12.048
- Archsmith, J. E., Muehlegger, E., & Rapson, D. S. (2021). Future paths of electric vehicle adoption in the United States: Predictable determinants, obstacles and opportunities [Working Paper 28933]. National Bureau of Economic Research. http://www.nber.org/papers/w28933
- AutoTrader. (2022). Auto Trader Retail Price Index. Retrieved February 23, 2022, from https://plc.autotrader.co.uk/press-centre/auto-trader-retail-price-index/
- Barrett, E. (2021). China is rolling back the subsidies that fueled its electric-vehicle boom. Fortune, January 5. https://fortune.com/2021/01/05/china-electric-vehicle-subsidies-sales-tesla/
- Bass, F. M. (1969). A new product growth for model consumer durables. Management Science, 15(5), 215–227. https://doi.org/10.1287/mnsc.15.5.215
- Bass, F. M. (2004). Comments on "A new product growth for model consumer durables - The Bass model". Management Science, 50(12), 1833–1840. https://doi.org/10.1287/mnsc.1040.0300
- BenDor, T., & Ford, A. (2006). Simulating a combination of feebates and scrappage incentives to reduce automobile emissions. Energy, 31(8-9), 1197–1214. https://doi.org/10.1016/j.energy.2005.05.024
- Brand, C., Cluzel, C., & Anable, J. (2017). Modeling the uptake of plug-in vehicles in a heterogeneous car market using a consumer segmentation approach. Transportation Research Part A: Policy and Practice, 97, 121–136. https://doi.org/10.1016/j.tra.2017.01.017
- Brown, M. (2013). Catching the PHEVer: Simulating electric vehicle diffusion with an agent-based mixed logit model of vehicle choice. Journal of Artificial Societies and Social Simulation, 16(2), 5. https://doi.org/10.18564/jasss.2127
- Buchmann, T., Wolf, P., & Fidaschek, S. (2021). Stimulating e-mobility diffusion in Germany (EMOSIM): An agent-based simulation approach. Energies, 14(3), 656. https://doi.org/10.3390/en14030656
- Carley, K. M. (2017). Validating computational models (No. CMU-ISR-17-105). S. o. C. S. Institute for Software Research, Carnegie Mellon University. http://reports-archive.adm.cs.cmu.edu/anon/anon/home/ftp/usr0/ftp/isr2017/CMU-ISR-17-105.pdf
- Choi, J. (2016). Agent based model for estimating HEVs market: Focusing on the case of Korea. Science, Technology and Society, 21(2), 227–249. https://doi.org/10.1177/0971721816640625
- Coffman, M., Bernstein, P., & Wee, S. (2017). Electric vehicles revisited: A review of factors that affect adoption. Transport Reviews, 37(1), 79–93. https://doi.org/10.1080/01441647.2016.1217282
- Cui, X., Liu, C., Kyoung, H., Kao, S.-C., Mark, A., & Bhaduri, T., & L, B. (2010, January 10–14). An agent-based decision support system for electric vehicle charging infrastructure deployment. TRB Committee on Transportation Energy (ADC70), Washington DC, USA.
- de Santa-Eulalia, L. A., Neumann, D., & Klasen, J. (2011, October 23–29). A simulation-based innovation forecasting approach combining the Bass diffusion model, the discrete choice model and system dynamics. In SIMUL 2011: The third international conference on advances in system simulation, Madrid, Spain.
- Encarnação, S., Santos, F. P., Santos, F. C., Blass, V., Pacheco, J. M., & Portugali, J. (2018). Paths to the adoption of electric vehicles: An evolutionary game theoretical approach. Transportation Research Part B: Methodological, 113, 24–33. https://doi.org/10.1016/j.trb.2018.05.002
- Eppstein, M. J., Grover, D. K., Marshall, J. S., & Rizzo, D. M. (2011). An agent-based model to study market penetration of plug-in hybrid electric vehicles. Energy Policy, 39(6), 3789–3802. https://doi.org/10.1016/j.enpol.2011.04.007
- Eppstein, M. J., Rizzo, D., Lee, B., Krupa, J., & Manukyan, N. (2015). Using National Survey respondents as consumers in an agent-based model of plug-in hybrid vehicle adoption. IEEE Access, 3, 457–468. https://doi.org/10.1109/access.2015.2427252
- European Alternative Fuels Observatory. (2020). European Alternative Fuels Observatory (EAFO). Retrieved March 11, 2021, from http://www.eafo.eu
- Forrester, J. W. (1962). Industrial dynamics. MIT Press.
- Garcia, R., & Jäger, W. (2011). From the Special Issue editors: Agent-based modeling of innovation diffusion. Journal of Production Innovation Management, 28(2), 148–151. https://doi.org/10.1111/j.1540-5885.2011.00788.x
- Higgins, A., Paevere, P., Gardner, J., & Quezada, G. (2012). Combining choice modelling and multi-criteria analysis for technology diffusion: An application to the uptake of electric vehicles. Technological Forecasting and Social Change, 79(8), 1399–1412. https://doi.org/10.1016/j.techfore.2012.04.008
- Hoekstra, A., & Hogeveen, P. (2017, October 9–11). Agent-based model for the adoption and impact of electric vehicles in real neighbourhoods. EVS30 International Battery, Hybrid and Fuel Cell Electric Vehicle Symposium, Stuttgart, Germany.
- Hu, Y., Wang, Z., & Li, X. (2020). Impact of policies on electric vehicle diffusion: An evolutionary game of small world network analysis. Journal of Cleaner Production, 265, 121703. https://doi.org/10.1016/j.jclepro.2020.121703
- Huang, X., Lin, Y., Zhou, F., Lim, M. K., & Chen, S. (2021). Agent-based modelling for market acceptance of electric vehicles: Evidence from China. Sustainable Production and Consumption, 28, 206–217. https://doi.org/10.1016/j.spc.2021.04.007
- International Energy Agency. (2019). Global EV Outlook 2019: Scaling-up the transition to electric mobility. International Energy Agency. Retrieved April 13, 2020, from https://www.iea.org/reports/global-ev-outlook-2019
- International Energy Agency. (2021). International Energy Agency (IEA): Data and statistics. Retrieved March 13, 2021, from https://www.iea.org/data-and-statistics/charts
- Jäger, W. (2000). Modelling consumer behaviour. University of Groningen. https://pure.rug.nl/ws/portalfiles/portal/9914467/thesis.pdf
- Jensen, A. F., Cherchi, E., Mabit, S. L., & Ortúzar, J. de D. (2017). Predicting the potential market for electric vehicles. Transportation Science, 51(2), 427–440. https://doi.org/10.1287/trsc.2015.0659
- Jeong, B., Lee, W., Kim, D.-S., & Shin, H. (2016). Copula-based approach to synthetic population generation. PLoS One, 11(8), e0159496. https://doi.org/10.1371/journal.pone.0159496
- Jochem, P., Gómez Vilchez, J. J., Ensslen, A., Schäuble, J., & Fichtner, W. (2018). Methods for forecasting the market penetration of electric drivetrains in the passenger car market. Transport Reviews, 38(3), 322–348. https://doi.org/10.1080/01441647.2017.1326538
- Jun, D. B., & Kim, J. i. (2011). A choice-based multi-product diffusion model incorporating replacement demand. Technological Forecasting and Social Change, 78(4), 674–689. https://doi.org/10.1016/j.techfore.2010.10.012
- Kangur, A., Jäger, W., Verbrugge, R., & Bockarjova, M. (2017). An agent-based model for diffusion of electric vehicles. Journal of Environmental Psychology, 52, 166–182. https://doi.org/10.1016/j.jenvp.2017.01.002
- Keith, D. R., Struben, J. J. R., & Naumov, S. (2020). The diffusion of alternative fuel vehicles: A generalised model and future research agenda. Journal of Simulation, 14(4), 260–277. https://doi.org/10.1080/17477778.2019.1708219
- Kieckhäfer, K., Volling, T., & Spengler, T. S. (2014). A hybrid simulation approach for estimating the market share evolution of electric vehicles. Transportation Science, 48(4), 651–670. https://doi.org/10.1287/trsc.2014.0526
- Kieckhäfer, K., Wachter, K., & Spengler, T. S. (2017). Analyzing manufacturers' impact on green products' market diffusion – the case of electric vehicles. Journal of Cleaner Production, 162, S11–S25. https://doi.org/10.1016/j.jclepro.2016.05.021
- Kim, S., Lee, K., Cho, J. K., & Kim, C. O. (2011). Agent-based diffusion model for an automobile market with fuzzy TOPSIS-based product adoption process. Expert Systems with Applications, 38(6), 7270–7276. https://doi.org/10.1016/j.eswa.2010.12.024
- Klein, M., Lüpke, L., & Günther, M. (2020). Home charging and electric vehicle diffusion: Agent-based simulation using choice-based conjoint data. Transportation Research Part D: Transport and Environment, 88, 102475. https://doi.org/10.1016/j.trd.2020.102475
- Kohn, E., Huang, C., Kong, N., & Hardman, S. (2022). Electric vehicle incentives in 15 leading electric vehicle markets [Research report]. UC Davis. https://escholarship.org/uc/item/0tn2p4x6
- Kong, D., Xia, Q., Xue, Y., & Zhao, X. (2020). Effects of multi policies on electric vehicle diffusion under subsidy policy abolishment in China: A multi-actor perspective. Applied Energy, 266, 114887. https://doi.org/10.1016/j.apenergy.2020.114887
- Kumar, R. R., & Alok, K. (2020). Adoption of electric vehicle: A literature review and prospects for sustainability. Journal of Cleaner Production, 253, 119911. https://doi.org/10.1016/j.jclepro.2019.119911
- Li, J., Jiao, J., & Tang, Y. (2019). An evolutionary analysis on the effect of government policies on electric vehicle diffusion in complex network. Energy Policy, 129, 1–12. https://doi.org/10.1016/j.enpol.2019.01.070
- Liao, F., Molin, E., & van Wee, B. (2017). Consumer preferences for electric vehicles: A literature review. Transport Reviews, 37(3), 252–275. https://doi.org/10.1080/01441647.2016.1230794
- Lindenberg, S., & Steg, L. (2007). Normative, gain and hedonic goal frames guiding environmental behavior. Journal of Social Isuses: A Journal of the Society for the Psychological Study of Social Issues, 63(1), 117–137. https://doi.org/10.1111/j.1540-4560.2007.00499.x
- Liu, C., & Lin, Z. (2017). How uncertain is the future of electric vehicle market: Results from Monte Carlo simulations using a nested logit model. International Journal of Sustainable Transportation, 11(4), 237–247. https://doi.org/10.1080/15568318.2016.1248583
- Liu, Y., & Cirillo, C. (2018). Modeling green vehicle adoption: An integrated approach for policy evaluation. International Journal of Sustainable Transportation, 12(7), 473–483. https://doi.org/10.1080/15568318.2017.1393584
- Mabit, S. L, Cherchi, E., Jensen, A. F., & Jordal-Jørgensen, J. (2015). The effect of attitudes on reference-dependent preferences: Estimation and validation for the case of alternative-fuel vehicles. Transportation Research Part A: Policy and Practice, 82, 17–28. https://dx.doi.org/10.1016/j.tra.2015.08.006
- Mau, P., Eyzaguirre, J., Jaccard, M., Collins-Dodd, C., & Tiedemann, K. (2008). The ‘neighbor effect’: Simulating dynamics in consumer preferences for new vehicle technologies. Ecological Economics, 68(1-2), 504–516. https://doi.org/10.1016/j.ecolecon.2008.05.007
- Mazur, C., Offer, G. J., Contestabile, M., & Brandon, N. B. (2018). Comparing the effects of vehicle automation, policy-making and changed user preferences on the uptake of electric cars and emissions from transport. Sustainability, 2018(10), 676. https://doi.org/10.3390/su10030676
- McCoy, D., & Lyons, S. (2014). Consumer preferences and the influence of networks in electric vehicle diffusion: An agent-based microsimulation in Ireland. Energy Research & Social Science, 3, 89–101. https://doi.org/10.1016/j.erss.2014.07.008
- McFadden, D. (2000, July 2). Disaggregate behavioral travel demand’s RUM side: A 30-year perspective. In 9th International association for travel behaviour research conference, Brisbane, Australia.
- Mock, P., Hulsebusch, D., Ungethum, J., & Schmid, S. A. (2009). Electric vehicles - A model based assessment of future market prospects and environmental impacts. World Electric Vehicle Journal, 3(1), 172–185. https://doi.org/10.3390/wevj3010172
- Muller, P. O. (2004). Transportation and urban form - stages in the spatial evolution of the American metropolis. In S. Hanson, & G. Giuliano (Eds.), The geography of urban transportation (3rd ed., pp. 59–85). The Guilford Press.
- National Govenors Association. (2021). Governors staying ahead of the transportation innovation curve: A policy roadmap for states. National Govenors Association. https://www.nga.org/center/publications/ahead-of-the-transportation-curve/
- Ngo, T. A., & See, L. (2012). Calibration and validation of agent-based models of land cover change. In A. J. Heppenstall, A. T. Crooks, L. M. See, & M. Batty (Eds.), Agent-Based models of geographical systems (pp. 181–197). Springer. https://doi.org/10.1007/978-90-481-8927-4_10
- Nikolic, I., & Kasmire, J. (2013). Theory. In K. H. van Dam, I. Nikolioc, & Z. Lukszo (Eds.), Agent-Based modelling of socio-technical systems (pp. 11–71). Springer. https://doi.org/10.1007/978-94-007-4933-7_2
- Noori, M., & Tatari, O. (2016). Development of an agent-based model for regional market penetration projections of electric vehicles in the United States. Energy, 96, 215–230. https://doi.org/10.1016/j.energy.2015.12.018
- Oliveira, G. D., Roth, R., & Dias, L. C. (2019). Diffusion of alternative fuel vehicles considering dynamic preferences. Technological Forecasting and Social Change, 147, 83–99. https://doi.org/10.1016/j.techfore.2019.06.002
- Onat, N. C., Noori, M., Kucukvar, M., Zhao, Y., Tatari, O., & Chester, M. (2017). Exploring the suitability of electric vehicles in the United States. Energy, 121, 631–642. https://doi.org/10.1016/j.energy.2017.01.035
- Ortúzar, J. de D., & Willumsen, L. (2011). Modelling transport (4th ed.). Wiley and Sons.
- Parady, G., Ory, D., & Walker, J. (2021). The overreliance on statistical goodness-of-fit and under-reliance on model validation in discrete choice models: A review of validation practices in the transportation academic literature. Journal of Choice Modelling, 38, 100257. https://doi.org/10.1016/j.jocm.2020.100257
- Pasaoglu, G., Harrison, G., Jones, L., Hill, A., Beaudet, A., & Thiel, C. (2016). A system dynamics based market agent model simulating future powertrain technology transition: Scenarios in the EU light duty vehicle road transport sector. Technological Forecasting and Social Change, 104, 133–146. https://doi.org/10.1016/j.techfore.2015.11.028
- Pellon, M. B., Eppstein, M. J., Besaw, L. E., Grover, D. K., Rizo, D. M., & Marshall, J. S. (2010, January, 10–14). An agent-based model for estimating consumer adoption of PHEV technology. Transportation Research Board (TRB) Annual Meeting 2010, Washington DC, USA.
- Peres, R., Muller, E., & Mahajan, V. (2010). Innovation diffusion and new product growth models: A critical review and research directions. International Journal of Research in Marketing, 27(2), 91–106. https://doi.org/10.1016/j.ijresmar.2009.12.012
- PlotDigitizer. (2022). PlotDigitizer App. Retrieved February 5, 2022, from https://plotdigitizer.com
- Plötz, P., Gnann, T., & Wietschel, M. (2014). Modelling market diffusion of electric vehicles with real world driving data — part I: Model structure and validation. Ecological Economics, 107, 411–421. https://doi.org/10.1016/j.ecolecon.2014.09.021
- Querini, F., & Benetto, E. (2014). Agent-based modelling for assessing hybrid and electric cars deployment policies in Luxembourg and lorraine. Transportation Research Part A: Policy and Practice, 70, 149–161. https://doi.org/10.1016/j.tra.2014.10.017
- Querini, F., & Benetto, E. (2015). Combining agent-based modeling and life cycle assessment for the evaluation of mobility policies. Environmental Science and Technology, 49(3), 1744–1751. https://doi.org/10.1021/es5060868
- Ramsey, D. M., Kowalska-Pyzalska, A., & Bienias, K. (2018). Diffusion of electric vehicles: An agent-based modelling approach. Transactions on Computational Collective Intelligence XXXI, Wroclaw, Poland.
- Redelbach, M., Sparka, M., Schmid, S., & Friedrich, H. E. (2013). Modelling customer choice and market development for future automotive powertrain technologies. World Electric Vehicle Journal, 6(4), 935–944. https://doi.org/10.3390/wevj6040935
- Roberts, G. (2020, March 12). Budget 2020: Plug-in grant for electric vehicles cut. Fleet News. https://www.fleetnews.co.uk/news/company-car-tax-and-legislation/2020/03/12/budget-2020-plug-in-grant-for-electric-vehicles-cut
- Rogers, E. M. (2003). Diffusion of innovations (5th ed.). Free Press.
- Santos, G., & Rembalski, S. (2021). Do electric vehicles need subsidies in the UK? Energy Policy, 149, 111890. https://doi.org/10.1016/j.enpol.2020.111890
- Schmelzer, S., & Miess, M. (2015). The economic costs of electric vehicles [Report] (No. 11.1). I. f. A. S. V. (IHS). https://www.researchgate.net/profile/Michael-Miess/publication/280253582_The_Economic_Costs_of_Electric_Vehicles/links/55af928d08aeb0ab4667c74d/The-Economic-Costs-of-Electric-Vehicles.pdf
- Sen, B., Noori, M., & Tatari, O. (2017). Will corporate average fuel economy (CAFE) standard help? Modeling CAFE's impact on market share of electric vehicles. Energy Policy, 109, 279–287. https://doi.org/10.1016/j.enpol.2017.07.008
- Shafiei, E., Stefansson, H., Asgeirsson, E. I., Davidsdottir, B., & Raberto, M. (2013). Integrated agent-based and system dynamics modelling for simulation of sustainable mobility. Transport Reviews, 33(1), 44–70. https://doi.org/10.1080/01441647.2012.745632
- Shafiei, E., Thorkelsson, H., Ásgeirsson, E. I., Davidsdottir, B., Raberto, M., & Stefansson, H. (2012). An agent-based modeling approach to predict the evolution of market share of electric vehicles: A case study from Iceland. Technological Forecasting and Social Change, 79(9), 1638–1653. https://doi.org/10.1016/j.techfore.2012.05.011
- Shepherd, S., Bonsall, P., & Harrison, G. (2012). Factors affecting future demand for electric vehicles: A model based study. Transport Policy, 20, 62–74. https://doi.org/10.1016/j.tranpol.2011.12.006
- Silvia, C., & Krause, R. M. (2016). Assessing the impact of policy interventions on the adoption of plug-in electric vehicles: An agent-based model. Energy Policy, 96, 105–118. https://doi.org/10.1016/j.enpol.2016.05.039
- Smith, J. M. (1982). Evolution and the theory of games (1st ed.). Cambridge University Press.
- Sovacool, B. K. (2017). Experts, theories, and electric mobility transitions: Toward an integrated conceptual framework for the adoption of electric vehicles. Energy Research & Social Science, 27, 78–95. https://doi.org/10.1016/j.erss.2017.02.014
- Struben, J., & Sterman, J. D. (2008). Transition challenges for alternative fuel vehicle and transportation systems. Environment and Planning B: Planning and Design, 35(6), 1070–1097. https://doi.org/10.1068/b33022t
- Sullivan, J. L., Salmeen, I. T., & Simon, C. P. (2009). PHEV marketplace penetration: An agent-based simulation (No. UMTRI-2009-32). University of Michigan.
- Suzuki, T. (2020). Diffusion of electric vehicles subject to fiscal incentives. JARI Research Journal, 20200302(3), 1–6.
- Sweda, T., & Klabjan, D. (2011). An agent-based decision support system for electric vehicle charging infrastructure deployment. 2011 IEEE Vehicle Power and Propulsion Conference, Chicago, IL, USA.
- Tchetchik, A., Zvi, L. I., Kaplan, S., & Blass, V. (2020). The joint effects of driving hedonism and trialability on the choice between internal combustion engine, hybrid, and electric vehicles. Technological Forecasting and Social Change, 151, 119815. https://doi.org/10.1016/j.techfore.2019.119815
- Thagard, P. (2006). Evaluating explanations in law, science, and everyday life. Current Directions in Psychological Science, 15(3), 141–145. https://doi.org/10.1111/j.0963-7214.2006.00424.x
- Train, K. E. (2009). Discrete choice models with simulation (2nd ed.). Cambridge University Press.
- Tran, M. (2012). Agent-behaviour and network influence on energy innovation diffusion. Communications in Nonlinear Science and Numerical Simulation, 17(9), 3682–3695. https://doi.org/10.1016/j.cnsns.2012.01.016
- Van Wee, B., & Banister, D. (2016). How to write a literature review paper? Transport Reviews, 36(2), 278–288. https://doi.org/10.1080/01441647.2015.1065456
- Wardman, M. (2012). Review and meta-analysis of U.K. Time elasticities of travel demand. Transportation, 39(3), 465–490. https://doi.org/10.1007/s11116-011-9369-2
- Wardman, M. (2014). Price elasticities of surface travel demand: A meta-analysis of UK evidence. Journal of Transport Economics and Policy, 48(3), 367–384. http://www.jstor.org/stable/24396293.
- Wardman, M. (2022). Meta-analysis of price elasticities of travel demand in Great Britain: Update and extension. Transportation Research Part A: Policy and Practice, 158, 1–18. https://doi.org/10.1016/j.tra.2022.01.020
- Winston, C. (2021). Biden is going big on the future of cars. So why are autonomous vehicles lagging? Barron's, March 9. https://www.barrons.com/articles/biden-is-going-big-on-the-future-of-cars-so-why-are-autonomous-vehicles-lagging-51615298876
- Wolf, I., Schröder, T., Neumann, J., & de Haan, G. (2015). Changing minds about electric cars: An empirically grounded agent-based modeling approach. Technological Forecasting and Social Change, 94, 269–285. https://doi.org/10.1016/j.techfore.2014.10.010
- Yang, W., Zhou, H., Liu, J., Dai, S., Ma, Z., & Liu, Y. (2015, September 8–11). Market evolution modeling for electric vehicles based on system dynamics and multi-agents. International Symposium on Smart Electric Distribution Systems and Technologies (EDST), Vienna, Austria.
- Zhang, H., & Vorobeychik, Y. (2019). Empirically grounded agent-based models of innovation diffusion: A critical review. Artificial Intelligence Review, 52(1), 707–741. https://doi.org/10.1007/s10462-017-9577-z
- Zhang, T., Gensler, S., & Garcia, R. (2011). A study of the diffusion of alternative fuel vehicles: An agent-based modeling approach. Journal of Production Innovation Management, 28(2), 152–168. https://doi.org/10.1111/j.1540-5885.2011.00789.x
- Zhuge, C., Wei, B., Dong, C., Shao, C., & Shan, Y. (2019). Exploring the future electric vehicle market and its impacts with an agent-based spatial integrated framework: A case study of Beijing, China. Journal of Cleaner Production, 221, 710–737. https://doi.org/10.1016/j.jclepro.2019.02.262