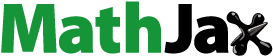
ABSTRACT
Human mobility is subject to collective dynamics that are the outcome of numerous individual choices. Smart card data which originated as a means of facilitating automated fare collection has emerged as an invaluable source for analysing mobility patterns. A variety of clustering and segmentation techniques has been adopted and adapted for applications ranging from market segmentation to the analysis of urban activity locations. In this paper we provide a systematic review of the state-of-the-art on clustering public transport users based on their temporal or spatial-temporal characteristics as well as studies that use the latter to characterise individual stations, lines or urban areas. Furthermore, a critical review of the literature reveals an important distinction between studies focusing on the intra-personal variability of travel patterns versus those concerned with the inter-personal variability of travel patterns. We synthesise the key analysis approaches as well as substantive findings and subsequently identify common trends and shortcomings and outline related directions for further research.
1. Introduction
1.1. Smart card data and the identification of mobility patterns
Human mobility is subject to collective dynamics that are the outcome of numerous individual choices. Even though individual needs and travel choices exhibit large variability and the urban and regional areas in which those they are embedded are subject to great diversity, there is evidence to suggest that human mobility manifests some recurring features throughout history and across geographies (Ahmed & Stopher, Citation2014). The growing availability of disaggregate mobility data enables the analysis of temporal and spatial patterns and the link between microscopic behaviour and resulting aggregate flows (Schläpfer et al., Citation2021). Geo-location traces are increasingly available from mobile phone and GPS data, social media posts and travel-related records such as automated fare collection (AFC) records (see Barbosa et al., Citation2018), giving rise to a plethora of studies analysing human mobility patterns and the decomposition thereof in the past decade. In this paper we provide a systematic review of the state-of-the-art on identifying and clustering human mobility patterns from smart card data and propose an outlook for addressing persisting challenges.
Smart card transactions offer a unique and rich source of passively collected data that enable the analysis of individual travel patterns. In the last decade, an extensive research attention has been devoted to the identification and classification of mobility patterns from smart card data. A number of review papers were devoted since the early 2010′s to smart card data analysis. Pelletier et al. (Citation2011) reviewed the technological advancements making the analysis of smart card data possible, followed by a review of strategic, tactical and operational applications of smart card data in public transport. Another relevant work is the one by Yue et al. (Citation2014) who reviewed trajectory-based travel behaviour studies, where smart card data is covered as one of the emerging sources enabling the analysis of human mobility patterns, replacing travel diaries and stated preferences data. Their main focus is on the properties of different trajectory data categories. In a more recent review, Hussain et al. (Citation2021) examined recent developments in using smartcard data for the purpose of origin-destination matrix estimation. The latter – as well as related inference algorithms that are performed in order to estimate travel destinations, vehicles boarded, transfer locations and home-zones – is a pre-requisite for conducting some of the research devoted to identifying human patterns based on smart card data. In this review we seek to answer the following three questions:
What are the key approaches and findings in relation to the analysis of individual spatial-temporal travel patterns based on smart card data transactions and in performing a market segmentation analysis?
What are the key approaches and findings in relation to the analysis of urban and network characteristics based on individual’s spatial-temporal travel patterns as revealed by smart card data transactions?
What are the key on-going trends, common shortcomings, knowledge gaps and related research directions?
1.2. Research strategy
Our search strategy was based on applying combinations of “Smart card data” and one or more of the following keywords when performing the bibliometric search: “Clustering”, “Travel patterns”, “Mobility patterns”, “User segmentation”, “Spatial”, “Temporal” and “Spatiotemporal”. The term “user” is used interchangeably with the terms “traveller” and “passenger” in the public transport demand analysis literature and we indeed find that these terms are used interchangeably in referring to individuals.
The databases of Scopus and Google Scholar were used to identify all relevant literature. The search, last performed on February 17, 2023, resulted in 724 papers of which 57 papers were identified as directly relevant for the scope of this analysis. Several additional studies were identified by means of a snowball search. Some of those excluded performed aggregate descriptive or statistical analysis of the spatial-temporal properties of public transport demand rather than identifying distinctive ones. Other papers excluded from this review focused on one of more of the following topics: inference algorithms, modes other than public transport, aggregate analysis of statistics such as OD matrix estimation, ridership, service evaluation and travel time distributions, and the statistical distributions thereof. Moreover, unsupervised learning methods other than clustering that could also be used to conduct pattern knowledge discovery from smart card mobility data are also considered out-of-scope in this survey.
All of the studies included in our analysis were published in the last decade, the vast majority (84%) of which in the past five years. The selected papers were then categorised based on whether they analyse individual mobility patterns or network and urban analytics. Based on the papers reviewed, an additional distinction was made between studies that characterise individual mobility patterns based exclusively on their temporal patterns and studies that also consider spatial features as part of the pattern identification.
In order to address the research questions posed in the previous subsection, we first defined and carried out the search strategy described above. For each individual article included in this review we critically examined and noted the following: study aim, analysis approach (including variables used in performing the clustering), the clustering technique(s) used, any additional variables that were used in the analysis or interpretation that we have identified, the characteristics of the application in terms of geographical context, transport modes included and whether the fare collection system consists of check-in only or also check-out (since it has consequences for whether destinations are observed or inferred) and summarising the key substantive findings. We then synthesised the review results in relation to objectives, methods, applications and results and identify relevant categories of studies and related trends. The following section, Section 2, provides a synthesis of the literature concerned with identifying and characterising travellers’ mobility patterns whereas Section 3 reviews studies that use the latter to characterise individual stations (stop), lines or urban areas. Next, we reflect on the state-of-the-art and point related shortcomings (Section 4) and remaining knowledge gaps, based on which we make suggestions for future research and offer a research agenda (Section 5).
2. Characterising individual mobility patterns
Mobility patterns are characterised by their temporal and spatial features. A careful inspection of the review results reveals that majority of the past work devoted to analysing individual mobility patterns using smart card data has focused on their temporal characteristics, and is reviewed in the following sub-section 2.1. Thereafter, we turn in sub-section 2.2 into reviewing works that have explicitly considered both spatial and temporal aspects of individual’s travel patterns as input to the market segmentation analysis.
2.1. Temporal patterns of individual’s travel
provides a summary of the 22 studies analysing temporal travel patterns, including their main aim, the variable(s) which are subject to analysis, the clustering technique employed, additional information that was used in the analysis and in the interpretation of the results, and the key features of the case study application.
Table 1. Summary of studies clustering individuals’ temporal travel patterns.
A critical review of the literature reveals an important distinction – yet only seldom made explicit by the studies themselves – between studies focusing on the intra-personal variability of travel patterns versus those concerned with the inter-personal variability of travel patterns. The former aims at classifying individual in terms of the stability of the temporal features of their travel patterns whereas the latter aims at segmenting individuals based on the characteristics of their regular temporal patterns. In other words, intra-personal variability is concerned with how regular one’s travel patterns are. In the context of temporal patterns this can be measured in terms of the extent to which a given traveller boards within the same time periods on given days of the week over a long period of time. In contrast, inter-personal variability pertains to the extent that different travellers exhibit the same travel patterns. In the context of temporal patterns, this may refer to whether different travellers are likely to board in the same time instances over the course of the analysis period. Note that while those categories of studies are not mutually exclusive – one can cluster travellers in terms of their individual travel stability – the distinction pertains to the research focus adopted by the two abovementioned categories.
Studies focusing on intra-personal stability have used various temporal features to analyse travel stability. The latter was investigated in terms of departure times – i.e. a traveller is considered stable if the start times of the trips are consistent over time (Kieu et al., Citation2015a; Manley et al., Citation2018) – by the number of trips made on each day of the week – i.e. a traveller is considered stable if the number of trips per day of the week is consistent throughout the analysis period (Deschaintres et al., Citation2019) – or a combination thereof (Moradi & Trepanier, Citation2020). Note that the former sets a stricter requirement for being considered a stable traveller than the latter does. A couple of studies have specifically looked into identifying so-called “extreme” travellers defined by the percentage and frequency of trips in certain time periods or of certain duration (Long et al., Citation2016) or in terms of the number of days travelled per week, travel frequency per day of the week and temporal differences between daily trips (Cui & Long, Citation2019).
Segmenting individual travellers based on their inter-personal variability can reveal similarities and differences in their temporal travel habits. Several studies have investigated aggregate indicators of travel frequency such as overall frequency of use and frequency of travel characteristics such as travelling by train and performing transfers (Kieu et al., Citation2018) or number of travel days (Egu & Bonnel, Citation2020). Since weeks are considered to be the fundamental unit of recurrent travel schedules, the number of trips per day of the week has been often considered an important feature for market segmentation (Viallard et al., Citation2019), especially in combination with the starting hour (Briand et al., Citation2017; El Mahrsi et al., Citation2017; Liu & Cheng, Citation2020; Cats & Ferranti, Citation2022a), resulting in an hour-by-hour weekly travel profile per user which is then subject to clustering. User segmentation can then also be used for predicting the travel frequency per user per time-of-day as a conditional probability (Yang et al., Citation2018). Individual travel diaries allow to specifically focus on the timing of (certain) activities such as boarding times when travelling to and back from work (Ji et al., Citation2019), the start times as well as duration of activities (Medina, Citation2018) and the activity sequence structure (Goulet-Langlois et al., Citation2016; Lei et al., Citation2020).
Smart card data facilitates the construction of individual-level travel diaries, which in turn enable the investigation of travel patterns amongst specific user groups. Many of the studies reviewed have analysed how the temporal patterns identified vary amongst users with different fare product types using the latter as a contextual post-analysis variable, some of which contain information on different user groups such as school pupils, higher education students, and retired or older users (Kieu et al., Citation2015a; Briand et al., Citation2017; El Mahrsi et al., Citation2017; Deschaintres et al., Citation2019; Egu & Bonnel, Citation2020). A single study has specifically focused on a selected user group, namely on the variability of trip frequency per hour by older travellers (Liu et al., Citation2021). In several studies, personal information available from smart card registrations has also been used, for at least a sample of users included in the analysis (Goulet-Langlois et al., Citation2016; Liu et al., Citation2021) or from a matching household survey (Long et al., Citation2016). However, in most cases no socio-demographic data is available for individual card holders. Notwithstanding, by inferring card-holders place of residence from their travel patterns, one can link users to zonal socio-demographic characteristics available from census data such as ethnicity, employment and income (Liu & Cheng, Citation2020; Cats & Ferranti, Citation2022a).
Clustering or segmentation techniques aim at identifying mutually exclusive and collectively exhaustive subsets of the population so as to maximise the intra-group similarities and minimise the inter-group similarities. The definition of the (dis)similarity metric is therefore crucial. In the context of market segmentation, the two most common clustering techniques are -means (see Likas et al., Citation2003) and agglomerative hierarchical (see Rokach & Maimon, Citation2005) clustering. The former finds the best partitioning of the dataset given a pre-defined number of clusters,
, with the distance metric pertaining to the centre of the cluster. A variant of which,
-medoids, selects an actual data point as the centre of each cluster. Agglomerative hierarchical techniques follow a bottom-up approach with iterative merging of groups of observations based on their similarity. While hierarchical approaches are more computationally expensive, they allow for greater variety of distance specifications and their output contains more information on relations between potential clusters and does not require the a-priori specification of the number of clusters. An additional technique used in several studies for clustering users based on the similarity between subsequent trips is DBSCAN (Khan et al., Citation2014) which relies on user-specified parameter values. A model-based approach such as Gaussian mixture model offer a latent profile assignment with probabilistic assignment of members to clusters which is especially attractive in the case of continuous variables (e.g. Briand et al., Citation2017).
A variety of clustering techniques have been employed in the analysis of temporal travel patterns. The most commonly used methods are: (i) K-means (Kieu et al., Citation2018; Yang et al., Citation2018; Deschaintres et al., Citation2019; Viallard et al., Citation2019; Liu & Cheng, Citation2020; Liu et al., Citation2021; Eltved et al., Citation2021), (ii) DBSCAN (Medina, Citation2018; Manley et al., Citation2018; Cui & Long, Citation2019), (iii) hierarchical clustering (Goulet-Langlois et al., Citation2016; Ghaemi et al., Citation2017; He et al., Citation2020; Kieu et al., Citation2018; Deschaintres et al., Citation2019; Egu & Bonnel, Citation2020; Moradi & Trepanier, Citation2020; Cats & Ferranti, Citation2022a) and (iv) multinomial or Gaussian mixture models (Briand et al., Citation2017; El Mahrsi et al., Citation2017; Ji et al., Citation2019; Cats & Ferranti, Citation2022a). A single study applied a spatial affinity propagation technique (Kieu et al., Citation2018). Several studies have specifically focused on improving the projection of temporal distances to facilitate its hierarchical clustering (Ghaemi et al., Citation2017), on comparing the performance of alternative distance metrics when clustering users based on time-series data (He et al., Citation2020) or comparing alternative clustering techniques (Kieu et al., Citation2018).
Case study applications analysed smart card data from cities in Australia (Brisbane, Sydney), Canada (Gatineau, Montreal), China (Beijing, Nanjing, Shenzhen), Denmark (Copenhagen), France (Lyon, Rennes), Japan (Shizuoka), Singapore, Spain (Tarragona), Sweden (Stockholm) and United Kingdom (London). Most applications have considered metro systems, bus systems or a combination thereof, with few studies extending to other urban and suburban modes of public transport. Smart card data from most metro systems worldwide and bus systems from systems in East Asia analysed by studies included in this review contain both tap-in and tap-out data. Note that for most approaches taken in the analysis of temporal travel patterns no information regarding travel destination is per-se needed since boarding time (available from tap-in transactions) is the prime variable of interest used for constructing travel profiles that are then subject to clustering.
Few of the studies examining temporal travel patterns have also considered spatial elements such as the share of regular OD trips (Kieu et al., Citation2015a) or information on travel modes and specific services (Manley et al., Citation2018). In addition, a number of studies have considered spatial attributes such as relating those to stations (Manley et al., Citation2018), inferred home location (Kieu et al., Citation2018; Liu & Cheng, Citation2020; Cats & Ferranti, Citation2022a), inferred home and job locations (Long et al., Citation2016), land use information (Lei et al., Citation2020), diversity of travel destinations as measured by means of spatial entropy (Briand et al., Citation2017) as additional variables used for enriching the analysis and interpretation of the results. Notably, these variables range from incorporating information from other datasets such as land use registration or census survey to analysing the share of each identified user segment for different spatial resolutions (e.g. stations, zones).
A synthesis of the key findings reveals that patterns are characterised in relation to their travel frequency, days-of-the-week and times-of-the-day travel with results consistently distinguishing between regular/commuting travellers and irregular/occasional travellers categories with several clusters therein, especially in relation to a range of irregular and relatively uncommon patterns (e.g. Goulet-Langlois et al., Citation2016; El Mahrsi et al., Citation2017; Yang et al., Citation2018; Liu & Cheng, Citation2020; Cats & Ferranti, Citation2022a). The regular/commuting travellers typically constitute a large and more homogenous minority of card-holders yet they perform the vast majority of trips.
2.2. Spatiotemporal patterns of individual users’ travel
provides a summary of user segmentation studies that have identified spatiotemporal travel patterns. Similarly to the main divide in the analysis of temporal travel patterns, also studies focusing on both spatial and temporal characteristics have either looked into intra-personal or inter-personal variability. The former considered in this context thus not only the extent to which users travel at the same times over the course of the analysis period (be it defined in terms of frequency per week, days of the week and/or time of the day) but also the locations between which they have travelled.
Table 2. Summary of studies clustering individual’s spatial-temporal travel patterns.
In an attempt to identify commuters, studies focusing on intra-personal variability have considered variables such as the number of active travel days and the similarity of first boarding times, stations and route sequences (Ma et al., Citation2013) or the prevalence of most frequent origin-destination and the respective boarding times and routes (Ma et al., Citation2017). A broader perspective, not limited to commuting and most commonly performed trips, considers the distribution of trips over daily time periods and the most often used ODs and stations (Kaewkluengklom et al., Citation2021), or adds greater detail in the form of the overall sequence of activity locations and their respective durations per day (Goulet-Langlois et al., Citation2018; He et al., Citation2021). A similar approach served the opposite goal in identifying irregular (undirected, potentially suspicious) users based on travel frequency per time window and station (Wang, Zhang et al., Citation2020).
Studies focusing on inter-personal variability group users based on the characteristics of their regular spatiotemporal travel patterns (rather than the extent to which those are stable). Four studies have been identified in this category, three of which focused on special user groups. Gutierrez et al. (Citation2020) examined the combination of an array of temporal (travel frequency and number of travel days) and spatial (number of locations visited and routes used, prominence and identity of most visited locations) characteristics of tourists’ travel patterns. Wang, Zhang et al. (Citation2020) investigated the activity spaces of vulnerable users’ groups and employed to this end indicators related to both temporal (frequency, time entropy) and spatial (distance, radius and shape of activity space, place entropy) travel characteristics. Pieroni et al. (Citation2021) focused on low-income workers in the context of a metropolis with large income gaps and contrasts their travel patterns with those of high-income ones. The analysis conducted by Cats and Ferranti (Citation2022b) is the only one within this category which was not limited to any specific user group. Their analysis quantifies the spatial extent of locations visited, i.e. trip destinations, by users within the course of a period. A snowball search identified one additional study which regressed the extent to which users tend to travel using the same route against user, OD and trip characteristics, albeit without seeking to identify patterns (Kim et al., Citation2017).
Two techniques are dominant when integrating spatial information into the clustering of individual users, k-means (Ma et al., Citation2013; Yang et al., Citation2018; Kaewkluengklom et al., Citation2021; Wang, Zhang et al., Citation2020; Zhang, Yang et al., Citation2021; Pieroni et al., Citation2021; Cats & Ferranti, Citation2022b) and DBSCAN (Ma et al., Citation2013; Ma et al., Citation2017; Wang, Zhang et al., Citation2020). Some exceptions exist for studies using a diverse array of travel features which use a Gaussian mixture model (Gutierrez et al., Citation2020; Cats & Ferranti, Citation2022b) or hierarchical clustering (He et al., Citation2021), as well as a study identifying irregular users based on percentile cut-off of an entropy-based metric (Goulet-Langlois et al., Citation2018).
Of the eleven studies identified which analysed the spatiotemporal characteristics of individual mobility patterns using smart card data, three of which have been conducted for the case of Beijing and the remaining, with one study each, for Brisbane, Gatineau, London, Sao Paulo, Shizuoka, Stockholm, Tarragona and Wuhu.
A special class of studies – not concerned with the identification of user segments, yet concerned with their individual travel patterns – is the one focusing on physical encounters and thereby contact networks and their potential consequences within in the context of virus spreading. All these works construct a contact network based on the inferred travel trajectories. Sun et al. (Citation2013) analysed the statistical properties of the topological indicators – including the clustering coefficient – of the resulting physical encountering network for bus users in Singapore. Liu et al. (Citation2020) searched for the co-existence of users at stations and on-board vehicles using DBSCAN. Their application considered the metro networks of Shenzhen and they analyse the frequency and duration of encounters among pairs of users. Qian et al. (Citation2021) analysed the dynamics of virus spreading in contact networks and the effectiveness of control strategies, namely vaccination and quarantine, using contract networks constructed from the metro systems of Guangzhou, Shanghai and Shenzhen in China.
A synthesis of the findings reported suggests that there are few prevailing patterns in relation to spatial-temporal travel regularity which typically pertain to a different degree of commuting vs. occasional travel (e.g. Ma et al., Citation2013; Ma et al., Citation2017; Gutierrez et al., Citation2020; Kaewkluengklom et al., Citation2021). Moreover, there is evidence to suggest that those can be reasonably well predicted (Goulet-Langlois et al., Citation2018). However, once more information on spatial features of travel characteristics is considered, a great diversity of travel patterns is observed (e.g. Faroqui et al., Citation2022; Cats & Ferranti, Citation2022b).
3. Spatiotemporal travel patterns for network and urban analytics
In the previous section we reviewed the literature on clustering public transport users based on the temporal or spatial-temporal characteristics of their individual travel patterns. Another, related, body of literature has sought to identify and characterise clusters of (public transport) network elements – most often stations but in a few cases service lines – based on the spatial-temporal characteristics of their respective users, as well as the urban area in which the networks are embedded.
presents a summary of studies that performed network and urban analytics based on spatiotemporal travel patterns derived from smart card data. The objectives of the 24 studies included in this review vary greatly. Pioneering studies in the field sought to identify key activity centres using users’ flow data (Roth et al., Citation2011; Kim et al., Citation2014; Cats et al., Citation2015). Those have been followed by a series of studies that investigated the relation between the results of the station clustering and land-use patterns and places of interest in proximity to these stations or characteristics of the respective zones (Kim et al., Citation2017; Zhang et al., Citation2018; Zhao et al., Citation2019; Gan et al., Citation2020; Kim, Citation2020; Zhuang et al., Citation2020). Recent studies have extended this to the analysis of how urban structure has evolved over time (Wang et al., Citation2021; Zhang, Marshall et al., Citation2021). Another stream of works has focused on the generation of origin-destination matrices by aggregating stations into demand zones (Kieu et al., Citation2015b; Luo et al., Citation2017), characterising the demand patterns associated with individual stations (Zhong et al., Citation2015; El Mahrsi et al., Citation2017; Wang et al., Citation2017; Li et al., Citation2020; Zhou et al., Citation2022; Park et al., Citation2022), and clustering stations (Cats & Ferranti, Citation2022c; Chan Chun et al., Citation2023) or lines (Wang, Luo et al. Citation2020; Yap et al., Citation2019) that exhibit homogenous activity patterns.
Table 3. Summary of studies clustering network elements based on related travel patterns.
With the exception of the latter group focusing on service lines which is aimed at supporting service planning, all other studies have used individual stations or set of stations in proximity (i.e. areas) as the unit of analysis subject to clustering. All of the abovementioned studies have utilised origin-destination flow information derived from smart card data in their analysis with the exception of one study relying on boarding volumes only (El Mahrsi et al., Citation2017) and two studies considering boarding and alighting flows (Cats et al., Citation2015; Gan et al., Citation2020), presumably due to data availability limitations.
Given the dynamic character of network and urban centre characteristics, it is not surprising that the vast majority of studies have considered the temporal properties of users’ flow distribution in their analysis. Most studies have integrated information on the temporal characteristics of the flows analysed as part of the clustering process (Cats et al., Citation2015; El Mahrsi et al., Citation2017; Kim et al., Citation2017; Zhao et al., Citation2019; Gan et al., Citation2020; Kim, Citation2020; Li et al., Citation2020; Zhuang et al., Citation2020; Wang et al., Citation2021; Zhang, Marshall et al., Citation2021; Cats & Ferranti, Citation2022c; Chan Chun et al., Citation2023). In addition, several studies have investigated how the results of their clustering vary for different time periods (Luo et al., Citation2017; Wang et al., Citation2017; Yap et al., Citation2019; Wang, Luo et al. Citation2020).
Methods deployed for the clustering of stations (or sets of stations) and lines include k-means or variants thereof (Kim et al., Citation2017; Luo et al., Citation2017; Zhao et al., Citation2019; Gan et al., Citation2020; Yong et al., Citation2021; Cats & Ferranti, Citation2022c; Zhou et al., Citation2022; Chan Chun et al., Citation2023), variants of hierarchical methods (Roth et al., Citation2011; Kim et al., Citation2014; Cats et al., Citation2015; Kim, Citation2020), DBSCAN (Kieu et al., Citation2015b; Yap et al., Citation2019), Poisson or Gaussian mixture models (El Mahrsi et al., Citation2017; Wang et al., Citation2021), affinity propagation (Wang et al., Citation2017; Zhuang et al., Citation2020) and a discriminative functional mixture model (Park et al., Citation2022). In addition, a number of studies adopted community detection techniques (Zhong et al., Citation2015; Yap et al., Citation2019, Zhang et al., Citation2018; Wang, Luo et al., Citation2020; Zhang, Marshall et al., Citation2021). The latter – which have not been adopted by any of the studies performing user segmentation reported in sections 2.1 and 2.2 – is a graph-based technique which partitions a network into communities by grouping together nodes (i.e. typically corresponding to stations) that are densely connected with those belonging to different communities being only sparsely connected, where the strength or distance of a connection is indicated by the selected link labelling.
Largely reflecting the geographical distribution of the research groups that have conducted related research, studies have analysed urban and system structure for cities from Australia (Brisbane), China (Beijing, Nanjing, Shanghai, Chongqing), France (Rennes), the Netherlands (The Hague), Singapore, South Korea (Seoul), Sweden (Stockholm) and the United Kingdom (London). The majority of studies have utilised (only) metro data whereas several studies included all public transport modes present in the case study area to best reflect the underlying travel demand patterns, including in areas which might be underserved by the metro. A key distinction can be made between studies that cluster stations into geographically contiguous clusters (e.g. Roth et al., Citation2011; Luo et al., Citation2017; Cats & Ferranti, Citation2022c) and those that assign each station into one of the clusters independently of its neighbouring stations (e.g. El Mahrsi et al., Citation2017; Zhuang et al., Citation2020). The former is more suitable for most travel demand planning and management purposes. In both cases the main distinction is between areas that serve commuting/business/commercial trips and residential areas with reverse morning vs. afternoon patterns (e.g. Cats et al., Citation2015; El Mahrsi et al., Citation2017; Kim et al., Citation2017; Li et al., Citation2020; Wang et al., Citation2021).
Given the scope of this review, the discussion above and are limited to studies that identify patterns in how user flows differ across the system for the purpose of either network or urban analytics. The bibliometric search yielded several adjacent studies that are either grounded in complex system theory or in urban planning. Studies within the realm of the former investigate statistical properties of travel flows across the network, such as the stability of how travel patterns vary over days for the networks of London, Singapore and Beijing (Zhong et al., Citation2016) or calculated several measures of urban diversity based on the temporal pattern of flows per spatial units (Sulis et al., Citation2018). Examples of the latter include the analysis of year-on-year change in the job to worker ratio per station in the Beijing metro network (Huang et al., Citation2019) and the identification of employment areas in Beijing and the evolution thereof based on temporal characteristics of boarding and alighting flows (Huang et al., Citation2021). Note that these studies, unlike the studies included in , use descriptive statistics to observe spatial variations.
4. Discussion
As is evident from the synthesis of the literature provided in sections 2 and 3, there is an increasing body of research that has either characterised user travel patterns or performed network and urban analytics based on the associated travel patterns. In particular, several on-going trends in this research domain can be identified:
Specific user groups. There is increasing interest in investigating travel patterns for particular target segments which exhibit distinctive travel patterns ranging from tourists (Gutierrez et al., Citation2020) and older travellers (Liu et al., Citation2021) to lower income individuals (Pieroni et al., Citation2021).
Enriching smart card data with socio-demographic data. This has been accomplished by means of including personal information available from card registrations (Goulet-Langlois et al., Citation2016; Liu et al., Citation2021) or a matching household survey (Long et al., Citation2016). Alternatively, zonal socio-demographic data was linked by inferring the home-zone location of card holders (Liu & Cheng, Citation2020; Cats & Ferranti, Citation2022a).
Evolution of travel patterns. The availability of smart card data over the course of a substantial time period allows for the analysis of changes in observed travel patterns in terms of identified market segments (Briand et al., Citation2017; Viallard et al., Citation2019), station clusters (Huang et al., Citation2021) or underlying urban characteristics (Zhang, Marshall et al., Citation2021).
When examining the literature devoted to user segmentations, it is evident that the number of studies that consider the spatial characteristics of travel patterns in the identification of user segments is comparatively small compared with the body of literature devoted to temporal aspects of user travel patterns using smart card data. Furthermore, all of the relevant studies identified in this review, except for the notable exception of Cats and Ferranti (Citation2022b), have analysed spatial features in conjunction with temporal ones, rather than analysing spatial aspects in isolation. In line with the scope of this review, studies that analysed the spatiotemporal characteristics of ridership flows or origin-destination matrices are not included in this analysis.
The limited research effort devoted to the consideration of spatial features as part of user segmentation arguably stems from its greater complexity as compared to temporal aspects. The latter can easily be discretized and are universal and therefore the transferability of the associated feature definition and clustering techniques is fairly straightforward. In contrast, spatial features are subject to local variations and are often labelled, their discretization is not trivial and the findings cannot be easily made transferable to other contexts. The main challenge associated with studies that are concerned with clustering – either users or network elements – based on spatial features is how to incorporate those in a generic way as opposed to tailored to local features. It is therefore advisable to refer to topological characteristics which can be transferable to other contexts (e.g. centrality indicators of stations or diversity of locations visited) rather than references/labels of specific locations.
In our review of the literature it also became apparent that certain important aspects related to the underlying data used are under-discussed, hampering transparency and cross-comparison, and consequently hamper the advancement of this research field. In the following we discuss three such aspects:
Time span covered by the smart card dataset. The time period for which smart card data has been analysed varies greatly among studies, measured in days or years. Time periods vary from a single day (e.g. Faroqui et al., Citation2022), one (e.g. Cui & Long, Citation2019) or several weeks (e.g. Goulet-Langlois et al., Citation2016; El Mahrsi et al., Citation2017; Ghaemi et al., Citation2017; Gan et al., Citation2020) to several months (e.g. Egu & Bonnel, Citation2020), about a year (e.g. Deschaintres et al., Citation2019; Cats & Ferranti, Citation2022a, Citation2022b, Citation2022c) or up to several years (e.g. Goulet-Langlois et al., Citation2018). Several authors commented on the importance of using a sufficiently long period in order to extract meaningful patterns. For example, Goulet-Langlois et al. (Citation2016) assert that it is important to consider several weeks in the analysis because of changes in behaviour from one week to the other. Cui and Long (Citation2019) argue that a week-long analysis is sufficient even for their comparison over two non-consecutive years, whereas Briand et al. (Citation2017) used one month data for each of five consecutive years in their pattern year-on-year changes analysis. There is thus no consensus as to how long of a period should be analysis. This calls for the development of standards and criteria to ensure that patterns reported are based on data collected over a sufficiently long period for a given analysis/application.
Assumed relation between cards and individual travellers. The identification of user segments based on smart card movements assumes that cards correspond to individual users. In many transit systems worldwide smart cards may either by anonymous or personal with the latter allowing for more complicated subscriptions and concessions. Anonymous cards may be used by multiple individuals thereby violating the underlying assumptions employed for attributing the movements associated with a card to a single individual. This key assumption is however very seldom made explicit in the vast majority of the studies reviewed. A notable exception is made by Eltved et al. (Citation2021) who explicitly limits their analysis of to the impacts of long-term disruptions to the set of personal smart card data in Copenhagen to allow for the disaggregate analysis of behavioural responses for which having panel data is a pre-requisite. In addition, studies focusing on specific user groups who are eligible for concessions such as school pupils, higher education students, and retired or older users (Kieu et al., Citation2015a; Briand et al., Citation2017; El Mahrsi et al., Citation2017; Deschaintres et al., Citation2019 and Egu & Bonnel, Citation2020). have also limited their analysis to personal cards.
Smart card data as a good proxy of travel demand. For studies investigating public transport demand, i.e. individual mobility patterns, it is relevant to know what share of all public transport trips are registered in the form of smart card data. While smart card typically form the main mode of payment, it is seldom the only mean of fare collection. Transit systems worldwide offer a variety of alternative modes of payment including single-use (often paper) tickets and tickets purchased via a smart phone application. Datasets may or may not include transactions made by other means such as debit cards and the scanning of QR codes. The vast majority of studies reviewed do not comment on these aspects which are relevant for appreciating the completeness (and possibly representativeness as some groups might be over- or under-represented in some modes of payment, e.g. tourists). Few notable exceptions include El Mahrsi et al. (Citation2017) who reported that 80% of all trips are registered in the smart card dataset in the case of Rennes, France and Egu and Bonnel (Citation2020) who reported a 75% rate for the case of Lyon, France. Another related aspect which is seldom discussed by studies analysing travel patterns using smart card data pertains to the share of (public transport) trips captured. For studies focusing on urban analytics this is a crucial piece of information.
We therefore recommend that future studies include the following information in their analysis: (i) comment on why the timespan chosen is considered adequate for the relevant application; (ii) make an explicit statement about the type of cards included in the analysis (personal/anonymised) or the composition thereof, comment on potential implications for their clustering analysis, and; (iii) report the share of public transport trips included in the dataset used for analysis as well as the modal share for the analysis area in the case of an urban analytics study. Following such reporting standards will improve the soundness of the reporting and provide context when comparing findings associated with different dataset/systems.
5. Research agenda
Based on our critical review of the state-of-the-art we identify four knowledge gaps and outline four related themes for further research. The first two themes correspond the consideration of spatial features and the availability of panel data, following up on aspects mentioned in Section 4. The latter two themes refer to moving from understanding to predicting and connecting findings to (planning and operations) applications.
Enhanced geographical characterisation of users’ travel patterns. The analysis of mobility patterns and the related user clustering and market segmentation have insofar largely focused on their temporal features (section 2.1) or the stability and variability of travel destinations (section 2.2). However, there is lack of knowledge, with the notable exception of the work of Cats and Ferranti (Citation2022b), on the geographical properties of individual travellers by characterising spatial features of locations visited based on disaggregate longitudinal data. For the latter to be made available it is essential to comply with rigid privacy protocols due to the sensitivity of sequential (panel) geo-location data. Moreover, the aforementioned trend of enriching smart card data with socio-demographic data has been observed only for temporal user clustering but is yet to enter studies focusing on spatial clustering. The analysis thereof will enable identifying the extent to which different user groups visit different parts of the urban area. An aggregate analysis of the latter will allow unravelling the composition of travellers visiting various zones and the extent to which various destinations are visited by people with different backgrounds. Community detection techniques may be apply for user segmentation with the goal of identifying groups of travellers that share similar geographical features of their mobility patterns.
In the analysis of spatial characteristics it is advised, as mentioned in Section 4, to extract features that can be generalised to other contexts such as density, diversity and geographical scope of destinations visited rather than focus on labelled ones that pertain to local features (e.g. location names). Given the significant role that features of the local urban environment are likely to play in determining the spatial characteristics of users’ mobility, there is a need to gain more knowledge on how those are manifested in diverse settings. While these variables vary also within any given city, they may be confounded and the range of values might be limited. A cross-city comparison will allow concluding on relevant determinants such as urban form, density, availability and quality of alternative modes of transport, land-use and demographics.
From demand analytics towards behavioural analytics. While the identification and characterisation of human mobility patterns offers remarkable demand analytics insights it does not advance our knowledge on the underlying determinants of user’s behaviour. A separate large body of research is devoted to the estimation of travel choice determinants from smart card data, primarily limited to the estimation of route choice models and the impact of crowding therein (e.g. Hörcher et al., Citation2017; Yap & Cats, Citation2021). There is great potential in marrying these two research streams so as to support the development of behavioural models for users with distinctive mobility patterns, for example by means of estimating latent class models that are informed by the user clustering results.
Obtaining behavioural insights will also be stimulated by segmenting mobility patterns based on a combination of travel features that provide additional information on users experience. A couple of recent examples making advancements in this direction include the identification of central users for virus spreading by means of the simultaneous consideration of the distance travelled, the number of fellow travellers one has been exposed to and the degree of exploration (El Shoghri et al., Citation2020) and the neural network approach taken for the joint consideration of travellers’ departure and arrival time, travel location, travel distance and point of interest at the destination (Li et al., Citation2021).
Predictions of travel patterns. The availability of system-wide demand data opens avenues for the development of schemes aimed at the prediction of travel patterns. Such predictions can facilitate service design adjustments as well as supply- and demand-management measures to better cater or steer anticipated flows. A couple of studies included in this review already do so specifically for travel frequency per user (Yang et al., Citation2018) and the daily pattern of boarding flows at stations (Park et al., Citation2022).
The future development of prediction schemes can be structured along two axes: short-term vs. long-term and disaggregate vs. aggregate. The former distinction pertains to the time horizon for which the prediction is made, whether it concerns within-day downstream conditions measured in minutes to hours or considering a timespan of days or even months. Short-term predictions pose requirements on computational efforts and possibly also on the real-time availability of smart card data whereas long-term predictions can rely on offline applications. Several recently deployed fare collection systems enable the real-time availability of smart card data, albeit those are still far from common practice. The distinction between disaggregate vs. aggregate pertains, respectively, to whether the unit of analysis that is subject to prediction relates to trip characteristics of each user or to the travel pattern characteristics which are the outcome of collective dynamics, such as boarding, alighting and on-board flows.
All four combinations of short- and long-term, disaggregate and aggregate predictions are relevant as future research directions and can facilitate respective applications and interventions by service providers. Several pioneering studies proposed a logistic regression model for predicting the next trip to be performed by a user (Zhao et al., Citation2018) and an elasticity model for short-term aggregate predictions (Van Oort et al., Citation2015).
Decision support for service planning and policy evaluation. The analysis of travel patterns should ultimately inform planners in making decisions ranging from strategic and tactical to operational planning. This can be further facilitated by fusing long- and short-term predictions of travel patterns. While most of the studied included in this review have alluded to potential relevant applications, none of them has made an explicit link to service planning tasks, with the exception of Yap et al. (Citation2019) and its relevance for service coordination.
There is thus clearly a missing link between the development and application of demand, urban and network clustering analysis on one hand and service design and decision support on the other hand, which is key for the value proposition of the literature reviewed here. Future research should bridge this gap by making explicit how the techniques and insights developed based on smart card data analytics can translate into service improvements. The latter can range from ex-ante policy assessment (see Kholodov et al., Citation2021 for an example concerned with price elasticities) and network and capacity allocation adjustments (see Kolkowski et al., Citation2023 for an example concerned with urban activity segregation impacts of a network development) to the design of flexible services (see Qiu et al., Citation2019 for an original contribution in this direction) on one hand to real-time management such as information provision and control measures on the other hand. In particular real-time control strategies will greatly benefit from advancements in short-term passenger flow predictions. Another promising direction is the development of fare products and fare schemes (see Halvorsen et al., Citation2020 for a relevant framework) based on the market segmentation performed using smart card data. For example, changes in travel patterns induced by the COVID pandemic crisis and work-from-home habits call for the development of new subscription models which can be devised to cater for changes in spatiotemporal user clusters.
Smart card data which originated and has been designed as a means of facilitating automated fare collection has clearly emerged as an invaluable source for analysing human mobility patterns. Fusing smart card data with other sources of geo-location data will potentially allow analysts to consider additional aspects of human mobility beyond those performed by means of public transport. Moreover, for the research analysing mobility patterns using smart card data to become an integral part of service design and decision making and offer insights that go beyond describing current travel patterns and related user segments, future research must combine methodological advancements in data science, machine learning and clustering techniques with knowledge in travel behaviour, demand forecasting and transport planning.
Disclosure statement
No potential conflict of interest was reported by the author(s).
References
- Ahmed, A., & Stopher, P. (2014). Seventy minutes plus or minus 10 – A review of travel time budget studies. Transport Reviews, 34(5), 607–625. https://doi.org/10.1080/01441647.2014.946460
- Barbosa, H., Barthelemy, M., Ghoshal, G., James, C. R., Lenormand, M., Louail, T., Menezes, R., Ramasco, J., Simini, F., & Tomasini, M. (2018). Human mobility: Models and applications. Physics Reports, 734, 1–74. https://doi.org/10.1016/j.physrep.2018.01.001
- Briand, A.-S., Come, E., Trepanier, M., & Oukhellou, L. (2017). Analyzing year-to-year changes in public transport passenger behaviour using smart card data. Transportation Research Part C: Emerging Technologies, 79, 274–289. https://doi.org/10.1016/j.trc.2017.03.021
- Cats, O., & Ferranti, F. (2022a). Unravelling individual mobility temporal patterns using longitudinal smart card data. Research in Transportation Business & Management, 43, 100816. https://doi.org/10.1016/j.rtbm.2022.100816
- Cats, O., & Ferranti, F. (2022b). Unravelling the spatial properties of individual mobility patterns using longitudinal travel data. Journal of Urban Mobility, 2, 100035. https://doi.org/10.1016/j.urbmob.2022.100035
- Cats, O., & Ferranti, F. (2022c). Voting with one’s feet: Unraveling urban centers attraction using visiting frequency. Cities, 127, 103773. https://doi.org/10.1016/j.cities.2022.103773
- Cats, O., Wang, Q., & Zhao, Y. (2015). Identification and classification of public transport activity centres in Stockholm using passenger flows data. Journal of Transport Geography, 48, 10–22. https://doi.org/10.1016/j.jtrangeo.2015.08.005
- Chan Chun, K., Bahk, J., Kim, H., Jeong, H.-C., & Kim, G. (2023). Classification of the metropolitan subway stations and spheres of influence of main commercial areas in Seoul. Physica A: Statistical Mechanics and its Applications, 609, 128387. https://doi.org/10.1016/j.physa.2022.128387
- Cui, Z., & Long, Y. (2019). Perspectives on stability and mobility of transit passenger's travel behaviour through smart card data. IET Intelligent Transport Systems, 13(12), 1761–1769. https://doi.org/10.1049/iet-its.2019.0212
- Deschaintres, E., Morency, C., & Trepanier, M. (2019). Analyzing transit user behavior with 51 weeks of smart card data. Transportation Research Record: Journal of the Transportation Research Board, 2673(6), 33–45. https://doi.org/10.1177/0361198119834917
- Egu, O., & Bonnel, P. (2020). Investigating day-to-day variability of transit usage on a multimonth scale with smart card data. A case study in Lyon. Travel Behaviour and Society, 19, 112–123. https://doi.org/10.1016/j.tbs.2019.12.003
- El Mahrsi, M. K., Come, E., Oukhellou, L., & Verleysen, M. (2017). Clustering smart card data for urban mobility analysis. IEEE Transactions on Intelligent Transportation Systems, 18(3), 712–728. https://doi.org/10.1109/TITS.2016.2600515
- El Shoghri, A., Liebig, J., Jurdak, R., Gardner, L., & Kanhere, S. S. (2020, August 31–September 3). Identifying highly influential travellers for spreading disease on a public transport system. Proceedings - 21st IEEE international symposium on a world of wireless, mobile and multimedia networks, WoWMoM, 30–39.
- Eltved, M., Breyer, N., Blafoss Ingvardson, J., & Anker Nielsen, O. (2021). Impacts of long-term service disruptions on passenger travel behaviour: A smart card analysis from the greater Copenhagen area. Transportation Research Part C: Emerging Technologies, 131, 103198. https://doi.org/10.1016/j.trc.2021.103198
- Faroqui, H., Mesvah, M., Kim, J., & Khodaii, A. (2022). Targeted advertising in the public transit network using smart card data. Networks and Spatial Economics, 22(1), 97–124. https://doi.org/10.1007/s11067-022-09558-9
- Gan, Z., Yang, M., Feng, T., & Timmermans, H. (2020). Understanding urban mobility patterns from a spatiotemporal perspective: Daily ridership profiles of metro stations. Transportation, 47(1), 315–336. https://doi.org/10.1007/s11116-018-9885-4
- Ghaemi, M. S., Agard, B., Trepanier, M., & Partovi Nia, V. (2017). A visual segmentation method for temporal smart card data. Transportmetrica A: Transport Science, 13(5), 381–404. https://doi.org/10.1080/23249935.2016.1273273
- Goulet-Langlois, G., Koutsopoulos, H. N., & Zhao, J. (2016). Inferring patterns in the multi-week activity sequences of public transport users. Transportation Research Part C: Emerging Technologies, 64, 1–16. https://doi.org/10.1016/j.trc.2015.12.012
- Goulet-Langlois, G., Koutsopoulos, H. N., Zhao, Z., & Zhao, J. (2018). Measuring regularity of individual travel patterns. IEEE Transactions on Intelligent Transportation Systems, 19(5), 1583–1592. https://doi.org/10.1109/TITS.2017.2728704
- Gutierrez, A., Domenech, A., Zaragozi, B., & Miravet, D. (2020). Profiling tourists’ use of public transport through smart travel card data. Journal of Transport Geography, 88, 102820. https://doi.org/10.1016/j.jtrangeo.2020.102820
- Halvorsen, A., Koutsopoulos, H. N., Ma, Z., & Zhao, J. (2020). Demand management of congested public transport systems: A conceptual framework and application using smart card data. Transportation, 47(5), 2337–2365. https://doi.org/10.1007/s11116-019-10017-7
- He, L., Agard, B., & Trepanier, M. (2020). A classification of public transit users with smart card data based on time series distance metrics and a hierarchical clustering method. Transportmetrica A: Transport Science, 16(1), 56–75. https://doi.org/10.1080/23249935.2018.1479722
- He, L., Trepanier, M., & Agard, B. (2021). Space–time classification of public transit smart card users’ activity locations from smart card data. Public Transport, 13(3), 579–595. https://doi.org/10.1007/s12469-021-00274-0
- Hörcher, D., Graham, D. J., & Anderson, R. J. (2017). Crowding cost estimation with large scale smart card and vehicle location data. Transportation Research Part B: Methodological, 95, 105–125. https://doi.org/10.1016/j.trb.2016.10.015
- Huang, D., Liu, T., Kong, F., & Guang, R. (2021). Employment centers change faster than expected: An integrated identification method and application to Beijing. Cities, 115, 103224. https://doi.org/10.1016/j.cities.2021.103224
- Huang, J., Levinson, D., Wang, J., & Jin, H. (2019). Job-worker spatial dynamics in Beijing: Insights from smart card data. Cities, 86, 83–93. https://doi.org/10.1016/j.cities.2018.11.021
- Hussain, E., Bhaskar, A., & Chung, E. (2021). Transit OD matric estimation using smartcard data: Recent developments and future research challenges. Transportation Research Part C: Emerging Technologies, 125, article no. 103044. https://doi.org/10.1016/j.trc.2021.103044
- Ji, Y., Cao, Y., Liu, Y., Guo, W., & Gao, L. (2019). Research on classification and influencing factors of metro commuting patterns by combining smart card data and household travel survey data. IET Intelligent Transport Systems, 13(10), 1525–1532. https://doi.org/10.1049/iet-its.2018.5512
- Kaewkluengklom, R., Kurauchi, F., & Iwamoto, T. (2021). Investigation of changes in passenger behavior using longitudinal smart card data. International Journal of Intelligent Transportation Systems Research, 19(1), 155–166. https://doi.org/10.1007/s13177-020-00232-3
- Khan, K., Rehman, S. U., Aziz, K., Fong, S., & Sarasvady, S. (2014, February 17–19). DBSCAN: Past, present and future. IEEE proceedings of the fifth international conference on the applications of digital information and web technologies (ICADIWT 2014), 232–238.
- Kholodov, Y., Jenelius, E., Cats, O., van Oort, N., Mouter, N., Cebecauer, M., & Vermeulen, A. (2021). Public transport fare elasticities from smartcard data: Evidence from a natural experiment. Transport Policy, 105, 35–43. https://doi.org/10.1016/j.tranpol.2021.03.001
- Kieu, L.-M., Bhaskar, A., & Chung, E. (2015a). Passenger segmentation using smart card data. IEEE Transactions on Intelligent Transportation Systems, 16(3), 1537–1548. https://doi.org/10.1109/TITS.2014.2368998
- Kieu, L.-M., Bhaskar, A., & Chung, E. (2015b). A modified density-based scanning algorithm with noise for spatial travel pattern analysis from smart card AFC data. Transportation Research Part C: Emerging Technologies, 58B, 193–207. https://doi.org/10.1016/j.trc.2015.03.033
- Kieu, L.-M., Ou, Y., & Cai, C. (2018). Large-scale transit market segmentation with spatial-behavioural features. Transportation Research Part C: Emerging Technologies, 90, 97–113. https://doi.org/10.1016/j.trc.2018.03.003
- Kim, K. (2020). Identifying the structure of cities by clustering using a new similarity measure based on smart card data. IEEE Transactions on Intelligent Transportation Systems, 21(5), 2002–2011. https://doi.org/10.1109/TITS.2019.2910548
- Kim, K., Oh, K., Lee, Y. K., Kim, S. H., & Jung, J.-Y. (2014). An analysis on movement patterns between zones using smart card data in subway networks. International Journal of Geographical Information Science, 28(8), 1781–1801. https://doi.org/10.1080/13658816.2014.898768
- Kim, M.-K., Kim, S.-P., Heo, J., & Sohn, H.-G. (2017). Ridership patterns at subway stations of Seoul capital area and characteristics of station influence area. KSCE Journal of Civil Engineering, 21(3), 964–975. https://doi.org/10.1007/s12205-016-1099-8
- Kolkowski, L., Cats, O., Dixit, M., Verma, T., Jenelius, E., Cebecauer, M., & Jarlebring Rubensson, I. (2023). Measuring activity-based social segregation using public transport smart card data. Journal of Transport Geography, 110, 103642. https://doi.org/10.1016/j.jtrangeo.2023.103642
- Lei, D., Chen, X., Cheng, L., Zhang, L., Ukkusuri, S. V., & Witlox, F. (2020). Inferring temporal motifs for travel pattern analysis using large scale smart card data. Transportation Research Part C: Emerging Technologies, 120, 102810. https://doi.org/10.1016/j.trc.2020.102810
- Li, C., Bai, L., Liu, W., Yao, L., & Travis Waller, S. (2021). Urban mobility analytics: A deep spatial–temporal product neural network for traveler attributes inference. Transportation Research Part C: Emerging Technologies, 124, 102921. https://doi.org/10.1016/j.trc.2020.102921
- Li, W., Zhou, M., & Dong, H. (2020). Classifications of stations in urban rail transit based on the two-step cluster. Intelligent Automation and Soft Computing, 26(3), 531–538. https://doi.org/10.32604/iasc.2020.013930
- Likas, A., Vlassis, N., & Verbeek, J. J. (2003). The global k-means clustering algorithm. Pattern Recognition, 36(2), 451–461. https://doi.org/10.1016/S0031-3203(02)00060-2
- Liu, K., Yin, L., Ma, Z., Zhang, F., & Zhao, J. (2020). Investigating physical encounters of individuals in urban metro systems with large-scale smart card data. Physica A: Statistical Mechanics and its Applications, 545, 123398. https://doi.org/10.1016/j.physa.2019.123398
- Liu, S., Yamamoto, T., Yao, E., & Nakamura, T. (2021). Examining public transport usage by older adults with smart card data: A longitudinal study in Japan. Journal of Transport Geography, 93, 103046. https://doi.org/10.1016/j.jtrangeo.2021.103046
- Liu, Y., & Cheng, T. (2020). Understanding public transit patterns with open geodemographics to facilitate public transport planning. Transportmetrica A: Transport Science, 16(1), 76–103. https://doi.org/10.1080/23249935.2018.1493549
- Long, Y., Liu, X., Zhou, J., & Chai, Y. (2016). Early birds, night owls, and tireless/recurring itinerants: An exploratory analysis of extreme transit behaviors in Beijing, China. Habitat International, 57, 223–232. https://doi.org/10.1016/j.habitatint.2016.08.004
- Luo, D., Cats, O., & van Lint, H. (2017). Constructing transit origin-destination matrices with spatial clustering. Transportation Research Record: Journal of the Transportation Research Board, 2652(1), 39–49. https://doi.org/10.3141/2652-05
- Ma, X., Liu, C., Wen, H., Wang, Y., & Wu, Y.-J. (2017). Understanding commuting patterns using transit smart card data. Journal of Transport Geography, 58, 135–145. https://doi.org/10.1016/j.jtrangeo.2016.12.001
- Ma, X., Wu, Y., Wang, Y., Chen, F., & Liu, J. (2013). Mining smart card data for transit riders’ travel patterns. Transportation Research Part C: Emerging Technologies, 36, 1–12. https://doi.org/10.1016/j.trc.2013.07.010
- Manley, E., Zhong, C., & Batty, M. (2018). Spatiotemporal variation in travel regularity through transit user profiling. Transportation, 45(3), 703–732. https://doi.org/10.1007/s11116-016-9747-x
- Medina, S. A. O. (2018). Inferring weekly primary activity patterns using public transport smart card data and a household travel survey. Travel Behaviour and Society, 12, 93–101. https://doi.org/10.1016/j.tbs.2016.11.005
- Moradi, M., & Trepanier, M. (2020). Assessing longitudinal stability of public transport users with smart card data. Transportation Research Procedia, 48, 1364–1375. https://doi.org/10.1016/j.trpro.2020.08.166
- Park, Y., Choi, Y., Kim, K., & Keun Yoo, J. (2022). Machine learning approach for study on subway passenger flow. Scientific Reports, 12(1), 2754. https://doi.org/10.1038/s41598-022-06767-7
- Pelletier, M. P., Trepanier, M., & Morency, C. (2011). Smart card data use in public transit: A literature review. Transportation Research Part C: Emerging Technologies, 19(4), 557–568. https://doi.org/10.1016/j.trc.2010.12.003
- Pieroni, C., Giannotti, M., Alves, B. B., & Renato, A. (2021). Big data for bus issues: Revealing travel patterns of low-income population based on smart card data mining in a global south unequal city. Journal of Transport Geography, 96, 103203. https://doi.org/10.1016/j.jtrangeo.2021.103203
- Qian, X., Sun, L., & Ukkusuri, S. V. (2021). Scaling of contact networks for epidemic spreading in urban transit systems. Scientific Reports, 11(1), 4408. https://doi.org/10.1038/s41598-021-83878-7
- Qiu, G., Song, R., He, S., Xu, W., & Jiang, M. (2019). Clustering passenger trip data for the potential passenger investigation and line design of customized commuter bus. IEEE Transactions on Intelligent Transportation Systems, 20(9), 3351–3360. https://doi.org/10.1109/TITS.2018.2875466
- Rokach, L., & Maimon, O. (2005). Clustering methods. In O. Maimon, & L. Rokach (Eds.), Data mining and knowledge discovery handbook (pp. 321–352). Springer.
- Roth, C., Kang, S. M., Batty, M., & Barthelemy, M. (2011). Structure of urban movements: Polycentric activity and entangled hierarchical flows. PloS one, 6(1), e15923. https://doi.org/10.1371/journal.pone.0015923
- Schläpfer, M., Dong, L., O’Keeffe, K., Santi, P., Szell, M., Salat, H., Anklesaria, S., Vazifeh, M., Ratti, C., & West, G. B. (2021). The universal visitation law of human mobility. Nature, 593(7860), 522–527. https://doi.org/10.1038/s41586-021-03480-9
- Sulis, P., Manley, E., Zhong, C., & Batty, M. (2018). Using mobility data as proxy for measuring urban vitality. Journal of Spatial Information Science, 16(2018), 137–162. https://doi.org/10.5311/JOSIS.2018.16.384
- Sun, L., Axhausen, K. W., Lee, D.-H., & Huang, X. (2013). Understanding metropolitan patterns of daily encounters. Proceedings of the National Academy of Sciences (PNAS), 110(34), 13774–13779. https://doi.org/10.1073/pnas.1306440110
- Van Oort, N., Brands, T., & de Romph, E. (2015). Short-term prediction of ridership on public transport with smart card data. Transportation Research Record: Journal of the Transportation Research Board, 2535(1), 105–111. https://doi.org/10.3141/2535-12
- Viallard, A., Trepanier, M., & Morency, C. (2019). Assessing the evolution of transit user behaviour from smart card data. Transportation Research Record: Journal of the Transportation Research Board, 2673(4), 184–194. https://doi.org/10.1177/0361198119834561
- Wang, J., Kong, X., Rahim, A., Xia, F., Tolba, A., & Al-Makhadmeh, Z. (2017). IS2Fun: identification of subway station functions using massive urban data. IEEE Access, 5, 27103–27113. https://doi.org/10.1109/ACCESS.2017.2766237
- Wang, L., Zhang, Y., Zhao, X., Liu, H., & hang, K. (2020). Irregular travel groups detection based on cascade clustering in urban subways. IEEE Transactions on Intelligent Transportation Systems, 21(5), 2216–2225. https://doi.org/10.1109/TITS.2019.2933497
- Wang, Z., Liu, H., Zhu, Y., & Zhang, Y. (2021). Identifying urban functional areas and their dynamic changes in Beijing: Using multiyear transit smart card data. Journal of Urban Planning and Development, 147(2), 04021002. https://doi.org/10.1061/(ASCE)UP.1943-5444.0000662
- Wang, Z., Luo, D., Cats, O., & Verma, T. (2020, September 20–23). Unravelling the hierarchy of public transport networks. Proceedings of the IEEE 23rd international conference on intelligent transportation systems (ITSC), 1–6.
- Yang, C., Yan, F., & Ukkusuri, S. V. (2018). Unraveling traveller mobility patterns and predicting user behavior in the Shenzhen metro system. Transportmetrica A: Transport Science, 14(7), 576–597. https://doi.org/10.1080/23249935.2017.1412370
- Yap, M., & Cats, O. (2021). Taking the path less travelled: Valuation of denied boarding in crowded public transport systems. Transportation Research Part A, 147, 1–13. https://doi.org/10.1016/j.trb.2021.02.010
- Yap, M., Luo, D., Cats, O., van Oort, N., & Hoogendoorn, S. (2019). Where shall we sync? Clustering passenger flows to identify urban public transport hubs and their key synchronization priorities. Transportation Research Part C: Emerging Technologies, 98, 433–448. https://doi.org/10.1016/j.trc.2018.12.013
- Yong, J., Zheng, L., Mao, X., Gao, A., & Weining, L. (2021). Mining metro commuting mobility patterns using massive smart card data. Physica A: Statistical Mechanics and its Applications, 584, 126351. https://doi.org/10.1016/j.physa.2021.126351
- Yue, Y., Lan, T., Yeh, A. G. O., & Li, Q.-Q. (2014). Zooming into individuals to understand the collective: A review of trajectory-based travel behaviour studies. Travel Behaviour and Society, 1(2), 69–78. https://doi.org/10.1016/j.tbs.2013.12.002
- Zhang, S., Yang, Y., Zhen, F., Losbang, T., & Li, Z. (2021). Understanding the travel behaviors and activity patterns of the vulnerable population using smart card data: An activity space-based approach. Journal of Transport Geography, 90, 102938. https://doi.org/10.1016/j.jtrangeo.2020.102938
- Zhang, X., Xu, Y., Tu, W., & Ratti, C. (2018). Do different datasets tell the same story about urban mobility – A comparative study of public transit and taxi usage. Journal of Transport Geography, 70, 78–90. https://doi.org/10.1016/j.jtrangeo.2018.05.002
- Zhang, Y., Marshall, S., Cao, M., Manley, E., & Chen, H. (2021). Discovering the evolution of urban structure using smart card data: The case of London. Cities, 112, 103157. https://doi.org/10.1016/j.cities.2021.103157
- Zhao, X., Wu, Y.-P., Ren, G., Ji, K., & Qian, W.-W. (2019). Clustering analysis of ridership patterns at subway stations: A case in Nanjing, China. Journal of Urban Planning and Development, 145(2), 04019005. https://doi.org/10.1061/(ASCE)UP.1943-5444.0000501
- Zhao, Z., Koutsopoulos, H. N., & Zhao, J. (2018). Individual mobility prediction using transit smart card data. Transportation Research Part C: Emerging Technologies, 89, 19–34. https://doi.org/10.1016/j.trc.2018.01.022
- Zhong, C., Batty, M., Manley, E., Wang, J., Wang, Z., Chen, F., & Schmitt, G. (2016). Variability in regularity: Mining temporal mobility patterns in London, Singapore and Beijing using smart-card data. PloS One, 11(2), e0149222. https://doi.org/10.1371/journal.pone.0149222
- Zhong, C., Manley, E., Muller Arisona, S., Batty, M., & Schmitt, G. (2015). Measuring variability of mobility patterns from multiday smart-card data. Journal of Computational Science, 9, 125–130. https://doi.org/10.1016/j.jocs.2015.04.021
- Zhou, Y., Zheng, S., Hu, Z., & Chen, Y. (2022). Metro station risk classification based on smart card data: A case study in Beijing. Physica A: Statistical Mechanics and its Applications, 594, 127019. https://doi.org/10.1016/j.physa.2022.127019
- Zhuang, D., Hao, S., Lee, D.-H., & Jin, J. G. (2020). From compound word to metropolitan station: Semantic similarity analysis using smart card data. Transportation Research Part C: Emerging Technologies, 114, 322–337. https://doi.org/10.1016/j.trc.2020.02.017