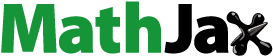
Abstract
In their pursuit of student engagement and achievement, educational institutes have increasingly been implementing student-centred approaches to learning with collaborative learning. In this study, we assessed the (mis)match effects of personal and group members’ achievement goals on student engagement and performance. Students (N = 266) from 36 different groups rated their achievement goals, their group members’ achievement goals, and their engagement for a course. Their exam performance was also included in the analysis. The results of the polynomial regression analyses indicated that both the degree of the compatibility and the direction of the incompatibility in mastery goals related to engagement, while only the degree in incompatibility in mastery goals related to performance. Conversely, neither the compatibility nor the incompatibility in performance goals related to engagement or performance. The results show the importance of examining the interplay between students’ and their group members’ achievement goals for student outcomes in a collaborative learning environment.
Collaboration is one of the four ‘21-century skills’ that educators should teach students to prepare them for a global society (National Education Association, Citation2014). As a response, educational institutes have implemented collaborative learning into their curricula. Collaborative learning constitutes ‘the grouping and pairing of learners for the purpose of achieving a learning goal’ (Laal & Ghodsi, Citation2012, p. 487). Important benefits of collaborative learning include, amongst other things, an increase in students’ engagement and performance. Student engagement refers to the tendency to be behaviourally (the focus of this study), emotionally, and cognitively involved in academic activities (Reeve, Citation2013). Indeed, studies show that students find collaborative learning motivating as it provides them with the opportunity be actively engaged and collaborative learning, furthermore, is beneficial for students’ achievement (Blasco-Arcas et al., Citation2013; Wijnia et al., Citation2011).
Although social aspects of the learning environment (e.g. collaboration) have been shown to be important (Ryan & Patrick, Citation2001), the influence of group composition (i.e. the composition of group members’ characteristics) is not often considered in the discussion on collaborative learning. Because group composition can result in homogeneous or heterogeneous groups, (dis)similarities between group members are evident in collaborative groups (Kristof-Brown & Stevens, Citation2001). This notion matters, as collaboration makes that the outcomes and the processes of the group depend on individual characteristics, the group composition, and how they interact (Kozlowski & Bell, Citation2013). For example, although group members may share a common goal, they might have very different reasons to pursue these goals (i.e. achievement goals). Discrepancies between personal achievement goals and composition achievement goals (i.e. the aggregate of group members’ achievement goals; Porter, Citation2008) affect important predictors of performance such as individual contributions to the task (Kristof-Brown & Stevens, Citation2001).
Studying student engagement and performance in a collaborative learning environment thus requires attention to how individual and group characteristics jointly affect student outcomes. Accordingly, the current study investigates to what extent students are more engaged and perform better when they resemble their group members in terms of their achievement goals in a collaborative learning environment.
Collaborative learning
As collaborative learning (CL) draws from many disciplines and theoretical perspectives (Hmelo-Silver & Bromme, Citation2007), it has been conceptualised in different ways by many researchers (e.g. Bruffee, Citation1999; Roschelle & Teasley, Citation1995; Van Boxtel et al., Citation2000). The ambiguity in the conceptualisation of CL mainly stems from disagreements about the definition of learning and the scale of interaction (i.e. the group size; Dillenbourg, Citation1999). However, researchers do seem to agree on a broad definition of CL as ‘a situation in which two or more people learn or attempt to learn something together’ (Dillenbourg, Citation1999, p. 1). As opposed to other forms of group work such as cooperative learning, collaborative learning requires students to do more than just work together on a project, it requires students to reach a consensus by engaging in a collective decision-making process (Bruffee, Citation1999). It follows then, that in CL, students share a common goal (Bruffee, Citation1999).
According to Dillenbourg (Citation1999), CL consists of the following four elements: 1) the situation, 2) interactions, 3) learning mechanisms, and 4) effects. A collaborative situation occurs if students have a similar status and abilities, have a common goal and work together. The second element, interaction, refers to the interactive and synchronous interpersonal activities that occur between students. Additionally, these activities are characterised by being negotiable, meaning that during CL, students argue, negotiate, and convince each other of their ideas. The third element concerns the cognitive and social-cognitive learning mechanisms that occur during CL. Examples include cognitive load, explanation, conflict, and internalisation. Finally, the fourth element, effects, refer to the learning outcomes of CL. Depending on the specific research interest, these four elements of CL and their complex interrelations can and have been examined through multiple theoretical lenses (Dillenbourg, Citation1999). To examine the effect of (dis)similarities between collaborative group members’ personal characteristics, we mainly utilise social-constructivism and motivational perspective lenses to focus on the CL elements of interaction and effects.
In CL the construction and development of understanding, knowledge, and skills, rely on the social interactions between students (Ertmer & Newby, Citation2013). By discussing different opinions and ideas, sharing different information, and explaining to each other, students think about and process information and thereby, further develop their understanding of the material. This is especially the case when students disagree with each other and cognitively experience conceptual conflicts (Littleton & Häkkinen, Citation1999; Schmidt et al., Citation2011). According to Schmidt et al. (Citation2011), the experience of conceptual conflict leads students to become more motivated and interested to resolve these differences so that they can understand the material better. As such, the social interaction activities that students perform in CL can lead to increased motivation, interest, and a better understanding of the material.
These effects of social interactions seem to be based on a core assumption of CL, namely that students share a common goal in CL (Bruffee, Citation1999). Although group members share a common goal, individual students might have very different achievement-related reasons to pursue these goals. These achievement goals might clash, but they might also coincide to reinforce each other. These (dis)similarities are evident in collaborative groups as a result of intense interactions between group members (Kristof-Brown & Stevens, Citation2001).
Personal achievement goals and their impact on learning outcomes
Achievement goals refer to the reasons individuals have to pursue a task (Linnenbrink & Pintrich, Citation2001). Two broad categories of achievement goals have been distinguished in the literature; mastery-approach and performance-approach goals. Mastery-approach goals refer to the aim to develop one’s competence, while performance-approach goals refer to the aim to demonstrate one’s competence. Because these two types of AGO’s are based on different definitions of competence, they lead to different interpretations of information and feedback, different behavioural tendencies, and subsequently different levels of performance (e.g. Payne et al., Citation2007). More specifically, the way competence is defined will impact the way effort is viewed (Dweck & Leggett, Citation1988). Individuals holding mastery-approach goals believe that competence is a fluid attribute that can be improved by exerting effort. When approaching an achievement situation with the aim to improve one’s skills (i.e. a mastery-approach goal), effort is thus seen as an integral part of the development process and as an essential component of success. In sharp contrast, individuals pursuing performance-approach goals believe that competence is a fixed attribute that cannot be improved. Thus, when approaching an achievement situation with the aim to outperform others (i.e. a performance-approach goal), having to put in effort is seen as a lack of ability. Consequently, mastery-approach goals and performance-approach goals will be differentially related to behavioural patterns in the classroom, possibly resulting in differences in performance.
Studies seem to provide empirical support for these theoretical propositions. While students pursuing mastery-approach goals are better prepared, active, attentive, and persistent in class, students pursuing performance-approach goals show a variety of behavioural patterns (e.g. Handelsman et al., Citation2005; Liem et al., Citation2008; McGregor & Elliot, Citation2002). Indeed, some studies (e.g. Handelsman et al., Citation2005) seem to find negative relations between performance-approach goals and engagement, while other studies (e.g. Elliot & Harackiewicz, Citation1996) find no relation between the two. Research into the relationship between achievement goals and performance show less consistent outcome patterns. That is to say, studies show that mastery-approach goals are often positively related and sometimes unrelated to performance, while performance-approach goals have often been found to be unrelated related to performance and sometimes positively related to performance (Payne et al., Citation2007). Indeed, some authors (e.g. Harackiewicz et al., Citation2002; Senko & Miles, Citation2008) have concluded that mastery-approach goals lead students to be interested in the course material, however, this does not always translate in course achievement. This outcome pattern could be caused by these students trying to learn as much as they can about a subject, even if that means putting in effort into irrelevant but interesting materials. Conversely, subscribing to performance-approach goals does not promote course interest, but it might lead to better performance because students are more focussed on what they need to perform well on the test. Thus, the impact of different achievement goals on learning outcomes follows a selective pattern (Barron & Harackiewicz, Citation2001).
In a collaborative learning environment, personal characteristics such as personal achievement goals are probably not the sole determinant of individual learning outcomes. In this type of learning environment, characteristics of student’s group members, such as the achievement goals that are pursued by group members might matter for students’ engagement during class and subsequently for their performance on exams. Indeed, research on learning outcomes in a collaborative learning environment seems to shed a different light on the relation between performance-approach goals and behavioural engagement. When working together in groups, both students subscribing to mastery-approach and (contrary to individual learning processes) students subscribing to performance-approach goals put effort into group discussions and group work (Harris et al., Citation2008). In a CL environment, behavioural engagement (e.g. putting in effort) relates to the social interaction element. Behaviour that shows promotive interaction includes giving and receiving help and feedback, exchanging resources and information, challenging each other's reasoning, advocating increased efforts to achieve, and engaging in interpersonal skills and processing the effectiveness of the group (Johnson & Johnson, Citation1996).
Based on the achievement goal theory (Dweck & Leggett, Citation1988), one could argue that students subscribing to different goals have very different reasons to be behaviourally engaged in the social interactions in the group; being mastery-approach oriented should make students want to share their information and resources, ask critical questions to their group members, candidly share their own opinions, and discuss all possible perspectives of the task at hand. In contrast, aiming to show their competence, students subscribing to performance-approach goals should want to show how much they know by being active and giving correct answers to questions asked by their group members and the teacher. These positive effort patterns should result in higher engagement and performance (e.g. Thijs & Verkuyten, Citation2009), and indeed, mastery-approach and performance-approach goals have been shown to relate to students’ engagement (e.g. Harris et al., Citation2008) and performance (e.g. Poortvliet et al., Citation2007) in collaborative learning environments. These studies show that students subscribing to mastery-approach and performance-approach goals are generally well prepared and active in class.
Outcomes of a (mis)match between personal and composition achievement goals
While studies (e.g. Harris et al., Citation2008) have shown that group members matter in the learning process, not much is known about the exact extent to which group members matter. For example, person-group fit theory stipulates that the match or mismatch between individual member’s personal characteristics and the personal characteristics of their group members affects individual outcomes (Kristof, Citation1996). Following this, the compatibility in achievement goals between students and their group members could be an important predictor of individual learning outcomes.
According to person-group fit theory, a match between the characteristics of the individual and the group will lead to beneficial outcomes for the individual, while a mismatch is believed to lead to an enriched situation in which at least one of the entities provides what the other needs (Kristof, Citation1996). Following the outcome pattern of personal mastery-approach goals, a match between personal and composition mastery-approach goals should result in higher levels of engagement and performance. We expect this because, when their group members are very actively involved in class discussions by asking a lot of critical questions and sharing their knowledge, students subscribing to mastery goals should become even more engaged in the class discussion as their inherent interest for the topic is fuelled by these discussions. Taking into account the positive relationship between engagement and performance, we expect similar patterns to occur for the performance outcome. Finally, these positive effects of a match between personal and composition mastery-approach goals should be greater when both goals are high than when both are low.
We expect a similar pattern for performance-approach goals; a match between personal and composition performance-approach goals should result in higher levels of engagement and performance. In addition, this positive effect of a match between personal and composition performance-approach goals should be greater when both goals are high than when both are low. When their group members are trying to show how much they know, students oriented towards performance goals should try even harder to show their competence by trying to outperform their group members. Again, the positive relationship between engagement and performance leads us to expect similar patterns to occur for the performance outcome.
Although some work has been done on the interaction between personal achievement goals and the learning environment (e.g. Barron & Harackiewicz, Citation2001), mismatch hypotheses concerning achievement goals are quite scarce in the educational literature. In addition, formulating hypotheses about the effects of a mismatch in achievement goals is not very straightforward. For example, the direction of the mismatch in the level of mastery-approach goals could matter in such a way that a group that is very focussed on mastery can inspire a student that is not very focussed on the development of their competence to engage more in group work. However, this group can also be annoying to the student, when he does not believe in the collaboration process and is merely there because of the attendance obligation. This might cause a student to disengage from the group process. Thus, we refrain from formulating these hypotheses a priori.
Method
Participants
The sample consisted of 454 undergraduate Psychology students enrolled at a Dutch university. These students were divided into 36 collaborative groups. However, due to logistical problems 188 of the 454 students, did not complete all measures. The final sample of participants thus consisted of 266 students. Because the students were randomly allocated to the groups and the groups were randomly allocated to the teachers, we are confident that these 188 students do not represent a separate sub-population with different characteristics than the 266 students that were included in the analysis. Participants had an average age of 21.65 (SD = 2.74) with 22.0% being male. Students were asked for their written consent to participate in the study. Participation took part on a voluntary basis and students did not receive any credits for participation.
Learning environment
The students were enrolled in a Bachelors programme, in which collaborative learning is the dominant educational approach. Each academic year is divided into eight 5-week blocks in which students follow a course. Every course the students are divided into a group consisting of approximately 10–12 students, a common group size in problem-based learning curricula (Dolmans & Schmidt, Citation2006). During each course, the groups work under the supervision of a teacher and meet two times a week. After each course, the group compositions changes and students are divided into a new group and work under the supervision of a new teacher. We decided to study the compatibility between students’ personal characteristics in one of these courses.
Learning activities
During the group meetings, following a problem-based learning approach, students were first presented with a case that they analysed and discussed together, resulting in the formulation of questions that guided their self-study. All groups discussed the same eight cases, that were comprised of topics about learning and learning disabilities. Students had to work together and engage in a collective decision-making process to collaboratively formulate the questions needed for their self-study. Although the students decided on the questions, the teachers made sure that students were on the right track and did not miss any crucial questions they should answer. During their self-study, students then selected, read, and processed their own learning resources. After their self-study, students met again to discuss, share their learning materials, and reach a consensus on the answers to their questions under the supervision of their teacher. As students found their own learning resources, they had to share different information, explain to each other, and convince each other of their ideas. The entire process from reading a case up until the sharing and discussing of the learning materials and reaching a consensus is thus directed by students themselves. As the students in the group needed each other to determine exactly what they needed to study to answer the questions and to study for the exam, their learning process was based on self-directed, student-centred learning (Schmidt, Citation2000).
Design
This study employed a cross-sectional design. The predictor variables personal achievement goals and group achievement goals were naturally occurring variables that we surveyed, while the outcome variables engagement and academic performance were automatically recorded by the office of student and educational affairs. Although the students were divided into small groups, the aim of the study was to examine intra-group differences rather than intergroup differences. The grouping of students and the allocation of the teachers to the groups was done completely at random by the office of student and educational affairs. As such, we had no control over the grouping of students. The students were therefore not matched to each other based on personal or other characteristics. This provided an opportunity for us to study the compatibility between students’ personal characteristics in a natural occurring collaborative learning setting.
Measures
Reliability measures for each scale and sub-scale, where necessary, are reported in .
Table 1. Descriptives and correlations between all variables.
Personal achievement goals
To measure student’s personal mastery-approach and performance-approach goals, we used the mastery-approach and performance-approach sub-scales of an adapted version of the achievement goal questionnaire by VandeWalle (Citation1997). To measure mastery-approach goals, five items were utilised (e.g. ‘I often look for opportunities to develop new skills and knowledge’) and four items were used to measure performance-approach goals (e.g. ‘I’m concerned with showing that I can perform better than my fellow students’). All items were answered on a 5-point Likert scale ranging from 1 (strongly disagree) to 5 (strongly agree).
Perceived composition achievement goals
The two corresponding composition achievement goals (i.e. composition mastery-approach and performance-approach goals) were assessed by asking participants to respond to a scale with the same items as the personal achievement goal measure. The only difference between these two scales was that the entity of interest differed (i.e. the individual versus the individual’s group members). Examples of these parallel items include ‘During this course, my group members often looked for opportunities to develop new skills and knowledge’ (mastery-approach goal) and ‘During this course, my group members were concerned with showing that they can perform better than each other’ (performance-approach goal). Thus, participants rated to what extent they believed that their group members (excluding themselves) were motivated by each of the two goals. As such, each group member had an individual rating of the group composition goals. Using SPSS statistical package version 25 (SPSS Inc, Chicago, IL), we analysed the inter-rater reliability measures and their 95% confident intervals based on a mean-measurement, absolute-agreement, 2-way random-effects model (McGraw & Wong, Citation1996). The results indicated that the composition mastery-approach goal subscale showed good interrater reliability, ICC2= .81, 95% CI [.77−.84]. Similarly, the group performance-approach goal measure also showed good interrater reliability, ICC2= .79, 95% CI [.75−.83].
Behavioural engagement
The behavioural engagement was assessed using the behavioural engagement sub-scale of the Engagement Versus Disaffection with Learning scale (Skinner et al., Citation2009). Students responded to five items, such as ‘In this class, I work as hard as I can’, using a 7-point Likert scale ranging from 1 (strongly disagree) to 7 (strongly agree).
Performance
The performance was assessed by using the grade on the exam of the course (scores ranged from 0 to 10). The exam tested students on their understanding of learning and learning disabilities (i.e. the course topics) as well as students’ ability to apply this knowledge to new cases. As the teachers made sure that the groups included all necessary questions, the exam was focussed on a general understanding of the course topics rather than specific questions about certain materials.
Analyses strategy
The data had a hierarchical structure (i.e. individual students were nested in groups). To decide whether or not to conduct multi-level analysis, we used the Hierarchical Linear Modelling (HLM; Raudenbush et al., Citation2017) programme to calculate the variance that could be accounted for by the group-level. For both the engagement and the performance outcome, the intra-class correlation for the group level did not reach significance, and respectively 0 (p > .05) and 22 (p > .05) per cent of the variance could be accounted for by the group level. A such, we decided not to conduct multi-level analyses.
Data preparation
Prior to analysis, both outcome and predictor variables were examined for missing values. The results of the Missing Values Analysis (Little, Citation1988) were not significant, χ2 (188)= 216.83, p = .07, indicating that the data were missing at random. Following recommendations by Little and Rubin (Citation2002), maximum likelihood estimation was used in the statistical analyses. In addition, following the procedures outlined by Edwards and Parry (Citation1993), we prepared the data by 1) scale-centring the independent variables (i.e. subtracting the midpoint of the scale) to avoid problems caused by multicollinearity and facilitate the interpretation of the surface plots and 2) screening for influential cases using the Bollen and Jackman (Citation1985) criteria; the results indicated that there were no outliers that influenced the analyses.
Polynomial regression
To analyse how the (mis)match between personal and composition achievement goals related to the outcome variables, we followed the recommendations put forward by Edwards and Parry (Citation1993) and made use of a combination of polynomial regression analyses and three-dimensional response surface plots. For some examples on the use of polynomial regression analysis and response surface analysis to examine (mis)match effect between individuals and their environment, we refer readers to Shanock et al. (Citation2010) and Kristof-Brown and Stevens (Citation2001). For each achievement goal and outcome, we modelled a polynomial regression line with the following regression equation:
(1)
(1)
Thus, engagement and performance levels for person i were regressed on the personal achievement goal, the composition achievement goal, the squared terms of the personal and composition achievement goal, the cross-product of the personal and composition achievement goal, and a residual term. The polynomial models were run and the response surfaces for significant models were subsequently plotted with the RSA R-package (R Development Core Team, Citation2014; Schönbrodt & Humberg, Citation2018).
Three-dimensional surface plots
Using the unstandardised regression weights obtained from EquationEquation 1(1)
(1) , we created three-dimensional surface plots to interpret how the degree a direction of both a match and a mismatch between personal and composition achievement goals related to the outcome variables. The perpendicular horizontal axes in the surface plot represent the personal achievement goal score and the composition achievement goal score and the vertical axis represents the outcome variable. To interpret how the (mis)match between personal and composition achievement goals related to the outcome variables, we examined the slope and the curvature of two lines: 1) the line x = y (i.e. personal achievement goal = composition achievement goal), the so-called line of fit and 2) the line x = −y (i.e. personal achievement goal= −composition achievement goal), the so-called line of misfit. The slope and curvature of these two lines represent the response surface pattern (see for the calculations of the slope and the curvature).
To interpret how the match between personal and composition achievement goals was related to the outcome variables (i.e. engagement and performance), we examined the slope and the curvature of the line of fit. The slope of this line indicates how a match between personal and composition achievement goals is related to the outcome variable. It shows the various levels of the outcome variable when the levels of personal and composition achievement goals are the same across the continuum from low to high scores. The curvature along this line indicates whether the relationship between the match of personal and composition achievement goals scores and the outcome variable is linear or not. Thus, a linear additive relationship along the line of fit (i.e. match effect), will be indicated by a significant slope of the line of fit and a non-significant curvature along this line.
To examine how the mismatch of personal and composition achievement goals was related to the outcome variables, we examined the slope and the curvature of the line of misfit. A significant slope of this line indicates how the direction of the mismatch affects the outcome variable, while a significant curvature of this line indicates how the degree of the mismatch affects the outcome variable.
Results
Descriptive statistics, correlations, and reliability indices for all measures are reported in . The correlations reported in indicate that personal mastery-approach goals are significantly related to engagement (r = .34) and performance (r = .12), while personal performance-approach goals are not significantly related to engagement as well as performance. In addition, composition mastery-approach and performance-approach goals are both significantly related to engagement (r = .24 and r = −.18, respectively) but not to performance. Unexpectedly, engagement and performance were not significantly related to each other. Additionally, older students reported higher levels of mastery goals (r = .16) than their younger counterparts, while female students reported lower levels of performance goals (r = −.15) and higher levels of behavioural engagement (r = 12).
The results of the four tested polynomial regressions and the corresponding values of the response surface analyses are presented in . The unstandardised regression coefficients and the explained variance are reported for each of the tested regression models.
Table 2. Results from Polynomial Regression of Engagement and Performance on Personal Achievement Goals and Composition Achievement Goals.
Engagement
(Mis)match between personal mastery-approach goals and the composition mastery-approach goals
The set of regression terms for the regression equation that explores the effects for personal and composition mastery goals explains a significant amount of variance in individuals’ engagement, R2 = .13, p < .001 (see for the outcomes of the regression models). Given its significant result, we examine the three-dimensional surface plot of this regression equation to specify the exact form of the relationship between personal and composition mastery-approach goals and engagement. As indicated by the significant (positive) values of the slope (a1) and the curvature (a2) of the line of fit, a match between personal and composition mastery-approach goals relates to engagement in a non-linear way. Thus, both the degree and the direction of the match between personal and composition mastery-approach goals relate to engagement.
The positive slope of the line of fit indicates that the line of fit as it relates to engagement is positive and a convex surface (upward curving). Indeed, in engagement increases more sharply as the combined levels of personal and composition mastery-approach goals increase; the lowest level of engagement is at the front where personal and composition mastery-approach goals are low and increasingly higher towards the back of the graph where personal and composition mastery-approach goals are both high.
Figure 1. Response surface plot portraying the joint effects on (A) engagement of personal mastery-approach goal (MG) and the composition MG) and on (B) performance of personal MG and the composition MG.
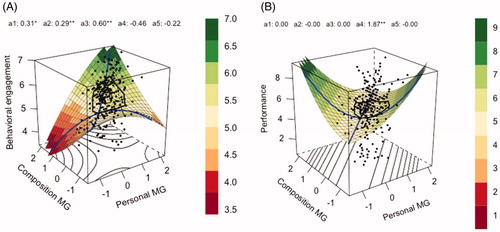
In addition, the significant positive slope on the line of misfit (a3) indicates that engagement is higher when the direction of the discrepancy is such that personal mastery-approach goals are higher than composition mastery-approach goals than vice versa. In , this pattern becomes clear when looking at the bottom left corner of the graph; engagement is relatively low where personal mastery-approach goals are low combined with high composition mastery-approach goals, while engagement is relatively higher in the middle of the graph where personal mastery-approach goals are higher than the composition mastery-approach goals.
(Mis)match between personal performance-approach goals and the composition performance-approach goals
The set of regression terms for the regression equation that explores the effects for personal and composition performance-approach goals does not explain a significant amount of variance in individuals’ engagement, indicating that neither a match or a mismatch between personal performance-approach goal and the composition performance goal relate to engagement.
Performance
(Mis)match between personal mastery-approach goals and the composition mastery-approach goals
The set of regression terms for the regression equations that explore the effects for personal and composition mastery-approach goals explains a significant amount of variance in individuals’ performance, R2 = .01, p = .02 (see for the outcomes of the regression models). Given its significant result, we examined the three-dimensional surface plot of this regression equation to specify the exact form of the relationship between personal and composition mastery-approach goals and performance. The positive curve on the line of misfit indicates a convex surface (upward curving), that is, performance increases more sharply as the degree of discrepancy between personal mastery-approach goal and the composition mastery-approach goal increases. Tracing along the line of misfit from the middle of the graph in to the far corner on the left and right, we see that his pattern becomes clear. Performance is higher at the outer ends of the graph than in the middle of the graph.
(Mis)match between personal performance-approach goals and the composition performance-approach goals
The results indicate that the set of regression terms for the regression equation that explores the effects for personal performance-approach goals and composition performance-approach goals does not explain a significant amount of variance in students’ performance, indicating that neither a match or a mismatch between personal performance-approach goals and the composition performance-approach goals relate to student’s performance.
Discussion
This study investigated to what extent students are more engaged and perform better when they resemble their group members in terms of their achievement goals (i.e. mastery-approach and performance-approach goals) in a collaborative learning environment. When students and their group members resemble each other in terms of the level of their mastery-approach goals, students are more engaged in group meetings. In addition, our results indicate that highly mastery-approach-oriented group members are not able to compensate for low levels of personal mastery-approach orientation. Conversely, when students are highly mastery-approach oriented and their group is not, this does not seem to matter that much for engagement. Thus, although the group might not be very engaging to them, their own focus on mastery-approach goals leads them to be engaged in group meetings. Interestingly, however, performance did not benefit from a mastery-approach orientation match between students and their group members. It did, however, benefit somewhat from a mismatch in mastery-approach orientation between students and their group members. It seems then, that for students that are highly mastery-approach oriented, group member’s achievement goals matter during the learning process but not really for their learning outcome. Conversely, neither the match or the mismatch between students and their group members performance-approach goals affected their engagement or performance levels. This is in contrast with the results for mastery-approach goals; the level of performance-approach goals of group members does not seem to matter for students’ engagement or performance.
Taken together, it seems that the effect of the interplay between students and their group members achievement goals on engagement and performance depends on both the type of achievement goal and the point in students’ learning process. These results are somewhat in line with the multiple goal perspective (e.g. Harackiewicz et al., Citation2002; Senko & Miles, Citation2008): subscribing to mastery-approach goals leads students to be interested in the course material, however, this does not always translate in course achievement. According to this perspective, subscribing to performance-approach goals does not promote course interest, but it does lead to better performance. However, we were not able to replicate these findings. The current study only extends part of the multiple goal perspective to the joint effect of personal and composition achievement goals. In line with the multiple goal perspective, the (in)compatibility in mastery-approach goals seems to matter for engagement, however, in contrast with this perspective, the incompatibility in mastery-approach goals also seems to matter for performance. Additionally, in line with the multiple goal perspective, students’ performance-approach goals and their (in)compatibility with group member’s performance-approach goals were not related to engagement in this study. However, in contrast with this perspective, these results also extended to student’s performance. In the literature, performance goals have been found to be positively as well as unrelated to academic performance. The unsurprising absence of a relation between the two might be traced back to the learning environment in which the exam took place. The normative standard used by individuals to define their competence is quite clear during the CL process, during the exam however, this standard might be more ambiguous. The normative standard could be one’s group members or the entire cohort. As such, the ambiguity of the normative standard might explain the lack of a relation between performance-approach goals and performance in this study.
Limitations
This study has its limitations which need to be addressed. Firstly, we chose to focus on approach goals without taking avoidance goals into account, because it has been well established that avoidance goals have a rather negative outcome pattern (Baranik et al., Citation2010; Church et al., Citation2001; Elliot & Church, Citation1997; Payne et al., Citation2007). As this was a first attempt at examining potential (mis)match effects in a naturally collaborative learning environment, we therefore, chose to solely focus on achievement goals that have been proven to lead to positive learning outcomes. Learning environments with self-directed learning at their core, such as problem-based learning, bring about certain uncertainties for students. This could be frustrating and fear-inducing for students oriented towards avoidance goals and especially master-avoidance goals, making it important to study avoidance goals in these learning environments. Thus, examining the effects of the (in)compatibility in avoidance achievement goals would be an interesting and important avenue to examine in future studies.
Secondly, we conceptualised group achievement goals as a composite of the individual scores as opposed to a collective perception variable (DeShon et al., Citation2004). However, our decision to use a composite score was based on the nature of the groups’ task (see Barrick et al., Citation1998 for a discussion). Students had to find their own learning resources which did not overlap with that of their group members, thus to fully grasp the topic they had to combine their resources. This additive nature of the task justifies the conceptualisation of group variables as a composite of the individual scores.
Additionally, we chose to focus on the stable nature of achievement goals in this study. Previous studies have treated achievement goals both as a stable, trait-like characteristic, and as a situationally specific state (Noordzij et al., Citation2013; Payne et al., Citation2007). Nonetheless, research has shown that the achievement goals that students adopt in a specific achievement domain remain quite stable over time (Lee et al., Citation2017). In addition, some researchers (Linnenbrink, Citation2005; Linnenbrink & Pintrich, Citation2001) argue that only very extreme differences in the achievement goal contexts affect which goals students adopt.
Finally, the performance outcome measure was operationalised as an individual exam rather than a collective performance measure. As mentioned before, learning has been conceptualised in different ways by CL researchers. While some seem to refer to learning in a CL environment as students studying course materials together for an exam, others refer to learning as a by-product of joint problem solving or as learning from collaborative work (Dillenbourg, Citation1999). Our operationalisation of the performance outcome measure seems to be in a line with the former conceptualisation of learning. Thus, although students were learning together and from each other, they were assessed individually. The learning process, therefore, did not match the assessment. This might explain the absence of a relation between the two outcome measures in this study.
Suggestions for future studies
This study provides the first evidence for non-linear effects of the interplay between personal achievement goals and composition achievement goals in a CL environment. However, these results need to be confirmed in different samples and settings to examine how they hold. For example, it would be interesting to see whether these same joint effects hold in other collaborative groups were students work together to create a product instead of gaining knowledge. Groups in these learning environments tend to stay together for an extended period, perhaps resulting in more homogenous groups and all the consequences that come with this. Additionally, social interaction processes in CL are bound to be influenced by the size of the group. As such, it is also worthwhile to study the (in)compatibility of achievement goals between students and their group members in different group sizes. Thirdly, it would be interesting to see if and how the interaction between personal achievement goals and composition achievement goals affects the separate components of engagement (i.e. behavioural, cognitive, and emotional) and a measure of group performance rather than individual performance. Furthermore, future studies could examine whether students are more affected by a (mis)match with their group members than a (mis)match with their teachers and whether these effects differ for certain students (e.g. first-generation versus continuing generation students).
Conclusion
The current study provided a first account of the joint effects of students’ and their group members achievement goals on engagement and performance in a collaborative learning environment. Traditionally, the joints effects of students and their learning context have been examined in traditional classrooms where students typically receive whole-class instruction. In addition, these investigations have made use of statistical models (i.e. linear interaction effects) that provide a limited picture. This study utilised the operationalisation of the interplay between individuals and their group members, as commonly done in the organisational psychology field, by applying person-group fit analyses strategies to an educational context. By doing so, we both broadened and deepened the limited knowledge we have on the interaction between personal achievement goals and the achievement goal context. To foster student outcomes in collaborative learning environments, it is important to have a better understanding of how student characteristics interact with their learning environment. To do so, we need to utilise methodologies that allow us to examine complicated, non-linear effects, such as those found in the current study.
Disclosure statement
No potential conflict of interest was reported by the author(s).
Additional information
Funding
References
- Baranik, L. E., Stanley, L. J., Bynum, B. H., & Lance, C. E. (2010). Examining the construct validity of mastery-avoidance achievement goals: A meta-analysis. Human Performance, 23(3), 265–282. https://doi.org/10.1080/08959285.2010.488463
- Barrick, M. R., Stewart, G. L., Neubert, M. J., & Mount, M. K. (1998). Relating member ability and personality to work-team processes and team effectiveness. Journal of Applied Psychology, 83(3), 377–391. https://doi.org/10.1037/0021-9010.83.3.377
- Barron, K. E., & Harackiewicz, J. M. (2001). Achievement goals and optimal motivation: Testing multiple goal models. Journal of Personality and Social Psychology, 80(5), 706–722. https://doi.org/10.1037//0022-3514.80.5.706
- Blasco-Arcas, L., Buil, I., Hernández-Ortega, B., & Sese, F. J. (2013). Using clickers in class. The role of interactivity, active collaborative learning and engagement in learning performance. Computers & Education, 62, 102–110. https://doi.org/10.1016/j.compedu.2012.10.019
- Bollen, K. A., & Jackman, R. W. (1985). Regression diagnostics: An expository treatment of outliers and influencial cases. Sociological Methods & Research, 13(4), 510–542. https://doi.org/10.1177/0049124185013004004
- Bruffee, K. A. (1999). Collaborative learning: Higher education, interdependence, and the authority of knowledge (2nd ed.). John Hopkins University Press.
- Church, M. A., Elliot, A. J., & Gable, S. L. (2001). Perceptions of classroom environment, achievement goals, and achievement outcomes. Journal of Educational Psychology, 93(1), 43–54. https://doi.org/10.1037/0022-0663.93.1.43
- DeShon, R. P., Kozlowski, S. W. J., Schmidt, A. M., Milner, K. R., & Wiechmann, D. (2004). A multiple-goal, multilevel model of feedback effects on the regulation of individual and team performance. The Journal of Applied Psychology, 89(6), 1035–1056. https://doi.org/10.1037/0021-9010.89.6.1035
- Dillenbourg, P. (1999). What do you mean by collaborative learning? In P. Dillenbourg (Ed.), Collaborative-learning: Cognitive and computational approaches (pp. 1–19). Elsevier.
- Dolmans, D. H. J. M., & Schmidt, H. G. (2006). What do we know about cognitive and motivational effects of small group tutorials in problem-based learning? Advances in Health Sciences Education: Theory and Practice, 11(4), 321–336. https://doi.org/10.1007/s10459-006-9012-8
- Dweck, C. S., & Leggett, E. L. (1988). A social-cognitive approach to motivation and personality. Psychological Review, 95(2), 256–273. https://doi.org/10.1037/0033-295X.95.2.256
- Edwards, J. R., & Parry, M. E. (1993). On the use of polynomial regression equations as an alternative to difference scores in organizational research. Academy of Management Journal, 36(6), 1577–1613. https://doi.org/10.2307/256822
- Elliot, A. J., & Church, M. A. (1997). A hierarchical model of approach and avoidance achievement motivation. Journal of Personality and Social Psychology, 72(1), 218–232. https://doi.org/10.1037/0022-3514.72.1.218
- Elliot, A. J., & Harackiewicz, J. M. (1996). Approach and avoidance achievement goals and intrinsic motivation: A mediational analysis. Journal of Personality and Social Psychology, 70(3), 461–475. https://doi.org/10.1037/0022-3514.70.3.461
- Ertmer, P. A., & Newby, T. J. (2013). Behaviorism, cognitivism, constructivism: Comparing critical features from an instructional design perspective. Performance Improvement Quarterly, 26(2), 43–71. https://doi.org/10.1111/j.1937-8327.1993.tb00605.x
- Handelsman, M. M., Briggs, W. L., Sulliva, N., & Towler, A. (2005). A measure of college student engagement. The Journal of Educational Research, 98(3), 184–191. https://doi.org/10.3200/JOER.98.3.184-192
- Harackiewicz, J. M., Barron, K. E., Pintrich, P. R., Elliot, A. J., & Thrash, T. M. (2002). Revision of achievement goal theory: Necessary and illuminating. Journal of Educational Psychology, 94(3), 638–645. https://doi.org/10.1037//0022-0663.94.3.638
- Harris, A., Yuill, N., & Luckin, R. (2008). The influence of context-specific and dispositional achievement goals on children's paired collaborative interaction. The British Journal of Educational Psychology, 78(Pt 3), 355–374. https://doi.org/10.1348/000709907X267067
- Hmelo-Silver, C. E., & Bromme, R. (2007). Coding discussions and discussing coding: Research on collaborative learning in computer-supported environments. Learning and Instruction, 17(4), 460–464. https://doi.org/10.1016/j.learninstruc.2007.04.004
- Johnson, D. W., & Johnson, R. T. (1996). Cooperation and the use of technology. In D. H. Jonassen (Ed.), Handbook of research for educational communications and technology (pp. 1017–1044). Simon and Schuster Macmillan.
- Kozlowski, S. W. J., & Bell, B. S. (2013). Work groups and teams in organizations: Review update. In N. Schmitt & S. Highhouse (Eds.), Handbook of psychology: Vol. 12. Industrial and organizational psychology (2nd ed., pp. 412–469). Wiley. https://doi.org/10.1002/0471264385.wei1214
- Kristof, A. L. (1996). Person-organization fit: An integrative review of its conceptualizations, measurement, and implications. Personnel Psychology, 49(1), 1–49. https://doi.org/10.1111/j.1744-6570.1996.tb01790.x
- Kristof-Brown, A. L., & Stevens, C. K. (2001). Goal congruence in project teams: Does the fit between members' personal mastery and performance goals matter? The Journal of Applied Psychology, 86(6), 1083–1095. https://doi.org/10.1037/0021-9010.86.6.1083
- Laal, M., & Ghodsi, S. M. (2012). Benefits of collaborative learning. Procedia - Social and Behavioral Sciences, 31(2011), 486–490. https://doi.org/10.1016/j.sbspro.2011.12.091
- Lee, Y., Wormington, S. V., Linnenbrink-Garcia, L., & Roseth, C. J. (2017). A short-term longitudinal study of stability and change in achievement goal profiles. Learning and Individual Differences, 55, 49–60. https://doi.org/10.1016/j.lindif.2017.02.002
- Liem, A. D., Lau, S., & Nie, Y. (2008). The role of self-efficacy, task value, and achievement goals in predicting learning strategies, task disengagement, peer relationship, and achievement outcome. Contemporary Educational Psychology, 33(4), 486–512. https://doi.org/10.1016/j.cedpsych.2007.08.001
- Linnenbrink, E. A. (2005). The dilemma of performance-approach goals: The use of multiple goal contexts to promote students’ motivation and learning. Journal of Educational Psychology, 97(2), 197–213. https://doi.org/10.1037/0022-0663.97.2.197
- Linnenbrink, E. A., & Pintrich, P. R. (2001). Multiple goals, multiple contexts: The dynamic interplay between personal goals and contextual goal stresses. In S. Volet & S. Jarvela (Eds.), Motivation in context: Theoretical advances and methodological implications (pp. 251–269). Pergamon Press.
- Little, R. J. A. (1988). A test of missing completely at random for multivariate data with missing values. Journal of the American Statistical Association, 83(404), 1198–1202. https://doi.org/10.1080/01621459.1988.10478722
- Little, R. J. A., & Rubin, D. B. (2002). Statistical analysis with missing data (2nd ed.). John Wiley & Sons.
- Littleton, K., & Häkkinen, P. (1999). Learning together: Understanding the processes of computer-based collaborative learning. In P. Dillenbourg (Ed.), Collaborative learning: Cognitive and computational approaches (pp. 20–30). Elsevier.
- McGraw, K. o., & Wong, S. P. (1996). Forming inferences about some intraclass correlation coefficients. Psychological Methods, 1(1), 30–46. https://doi.org/10.1037//1082-989X.1.4.390
- McGregor, H. A., & Elliot, A. J. (2002). Achievement goals as predictors of achiement-relevant processes prior to task engagement. Journal of Educational Psychology, 94(2), 381–395. https://doi.org/10.1037//0022-0663.94.2.381
- National Education Association. (2014). Preparing 21st century students for a global society: An educator’s guide to the “Four Cs.” National Education Association. http://www.nea.org/assets/docs/A-Guide-to-Four-Cs.pdf
- Noordzij, G., Van Hooft, E. A. J., Van Mierlo, H., Van Dam, A., & Born, M. P. (2013). The effects of a learning-goal orientation training on self-regulation: A field experiment among unemployed job seekers. Personnel Psychology, 66(3), 723–755. https://doi.org/10.1111/peps.12011
- Payne, S. C., Youngcourt, S. S., & Beaubien, J. M. (2007). A meta-analytic examination of the goal orientation nomological net. The Journal of Applied Psychology, 92(1), 128–150. https://doi.org/10.1037/0021-9010.92.1.128
- Poortvliet, P. M., Janssen, O., Van Yperen, N. W., & Van De Vliert, E. (2007). Achievement goals and interpersonal behavior: How mastery and performance goals shape information exchange. Personality & Social Psychology Bulletin, 33(10), 1435–1447. https://doi.org/10.1177/0146167207305536
- Porter, C. O. L. H. (2008). A multi-level, multi-conceptualization perspective of al orientation in teams. In V. I. Sessa & M. London (Eds.), Work group learning: Understanding, improving and assessing how groups learn in organizations (xxiv, pp. 149–173). Taylor & Francis Group/Lawrence Erlbaum Associates.
- R Development Core Team. (2014). R: A language and environment for statistical computing (Version 3.0.1) [Computer Software]. (No. 3). R Foundation for Statistical Computing.
- Raudenbush, S. W., Bryk, A., & Congdon, R. (2017). HLM 7.03 for Windows [Computer software]. Scientific Software International, Inc.
- Reeve, J. (2013). How students create motivationally supportive learning environments for themselves: The concept of agentic engagement. Journal of Educational Psychology, 105(3), 579–595. https://doi.org/10.1037/a0032690
- Roschelle, J., & Teasley, S. D. (1995). The construction of shared knowledge in collaborative problem solving. In C. E. O. O’Malley (Ed.), Computer Supported Collaborative Learning (pp. 69–97). Springer-Verlag. https://doi.org/10.1007/978-3-642-85098-1_5
- Ryan, A. M., & Patrick, H. (2001). The classroom social environment and changes in adolescents’ motivation and engagement during middle school. American Educational Research Journal, 38(2), 437–460. https://doi.org/10.3102/00028312038002437
- Schmidt, H. G. (2000). Assumptions underlying self-directed learning may be false. Medical Education, 34(4), 243–245. https://doi.org/10.1046/j.1365-2923.2000.0656a.x
- Schmidt, H. G., Rotgans, J. I., & Yew, E. H. J. (2011). The process of problem-based learning: What works and why. Medical Education, 45(8), 792–806. https://doi.org/10.1111/j.1365-2923.2011.04035.x
- Schönbrodt, F. D., & Humberg, S. (2018). RSA: An R package for response surface analysis (Version 0.9.13) [Software]. https://cran.r-project.org/package=RSA
- Senko, C., & Miles, K. M. (2008). Pursuing their own learning agenda: How mastery-oriented students jeopardize their class performance. Contemporary Educational Psychology, 33(4), 561–583. https://doi.org/10.1016/j.cedpsych.2007.12.001
- Shanock, L. R., Baran, B. E., Gentry, W. A., Pattison, S. C., & Heggestad, E. D. (2010). Polynomial regression with response surface analysis: A powerful approach for examining moderation and overcoming limitations of difference scores. Journal of Business and Psychology, 25(4), 543–554. https://doi.org/10.1007/s10869-010-9183-4
- Skinner, E. A., Kindermann, T. A., & Furrer, C. J. (2009). A motivational perspective on engagement and disaffection. Educational and Psychological Measurement, 69(3), 493–525. https://doi.org/10.1177/0013164408323233
- Thijs, J., & Verkuyten, M. (2009). Students' anticipated situational engagement: the roles of teacher behavior, personal engagement, and gender. The Journal of Genetic Psychology, 170(3), 268–286. https://doi.org/10.1080/00221320903218323
- Van Boxtel, C., Van Der Linden, J., & Kanselaar, G. (2000). Collaborative learning tasks and the elaboration of conceptual knowledge. Learning and Instruction, 10(4), 311–330. https://doi.org/10.1016/S0959-4752(00)00002-5
- VandeWalle, D. (1997). Development and validation of a work domain goal orientation instrument. Educational and Psychological Measurement, 57(6), 995–1015. https://doi.org/10.1177/0013164497057006009
- Wijnia, L., Loyens, S. M. M., & Derous, E. (2011). Investigating effects of problem-based versus lecture-based learning environments on student motivation. Contemporary Educational Psychology, 36(2), 101–113. https://doi.org/10.1016/j.cedpsych.2010.11.003