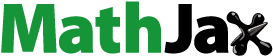
Abstract
The worked example effect has been well documented within the framework of Cognitive Load Theory (CLT), which suggests that teaching with examples would be superior to engaging in unguided problem solving, particularly for novices, as using worked examples would reduce their cognitive load, compared to solving problems, thus facilitating knowledge retention. This paper, using multiple-step mathematics problems, reports an experiment investigating the micro level of the worked example effect on learning solution steps, from the perspective of cognitive load and challenge (as a relevant affective, motivational factor), testing the worked example effect with a transfer test. The results favoured worked examples on both the retention and transfer tests after learning and showed that using worked examples would reduce cognitive load and impose less challenge on each step during learning.
Introduction
Within the framework of Cognitive Load Theory (CLT), the worked example effect has suggested that teaching with worked examples would be superior to engaging in problem solving due to reduced cognitive load. This effect has been well investigated and repeatedly found with novices across a range of domains.
Normally, solving a problem requires multiple steps, but almost all reported cognitive load during learning using worked examples is at a macro level, namely a total load after learning all steps without investigating cognitive load at the micro level, namely on each step. Chen et al. (Citation2019) investigated the worked example effect at the micro level (i.e. on each step) for the retention test only, without investigating the worked example effect at the micro level during learning and on a transfer test. This study, borrowing and extending the findings of Chen et al. (Citation2019), aims to investigate the worked example effect on cognitive load and challenge during learning at a micro level (i.e. on each step), as well as effects on retention and transfer at a macro level.
Human cognitive architecture
Human cognitive architecture, discussing the relations between working memory and long-term memory, serves as the base of CLT (Paas & van Merriënboer, Citation2020; Sweller et al., Citation2019). This architecture informs us how information is processed, stored, and retrieved in human memory systems. 5 principles underlying this architecture show us some pedagogical implications. Humans have many ways to acquire new knowledge, however, the most efficient way is to borrow the knowledge from others and reorganise it for storage in the long-term memory, suggesting the borrowing and reorganising principle. If new knowledge does not exist to be borrowed, humans generate new knowledge by mostly randomly combining elements of new information using relevant elements of prior knowledge, suggesting the randomness as the genesis principle. However, as working memory has very limited capacity (Cowan, Citation2001; Miller, Citation1994) and duration time (Peterson & Peterson, Citation1959), there are only very limited elements of new knowledge that could be generated and processed simultaneously in the working memory, suggesting the narrow limits of change principle. Only new information tested to be effective for solving problems is transferred and stored in the long-term memory (the information store principle), with the ineffective new information being discarded. Compared to working memory, long-term memory could store information for a very long period with unknown capacity. Reflecting the environmental organising and linking principle, stored information could be retrieved from long-term memory back to the working memory to solve externally presented problems. One of the original cognitive load effects generated based on this model of human cognitive architecture is the worked example effect.
The worked example effect
The worked example effect has been repeatedly found by using randomised controlled trials (i.e. Chen et al., Citation2015, Citation2016; Cooper & Sweller, Citation1987; Sweller & Cooper, Citation1985). This effect suggests that learning with examples is superior to engaging in problem solving at the initial learning stage, particularly for novices.
According to the described model of human cognitive architecture, the worked example effect is explained by the borrowing and reorganising principle and the randomness as the genesis principle. Learning with examples draws on the borrowing and reorganising principle, as students borrow the new knowledge from teachers or from peers, whereas, engaging in novel problem solving applies the randomness as genesis principle, with students randomly generating and combining solutions to solve new problems, which can easily overload working memory capacity (the narrow limit of change principle).
In CLT, worked example – problem solving pairs are used to investigate the worked example effect. In a randomised controlled trial, the performance of worked example – problem solving group is compared with the performance of the problem solving – problem solving group. The superiority of learning with a worked example – problem solving pairs is explained by students’ motivation to apply the knowledge learnt from worked examples in solving the following problems, and by the greater variety of activities allowed by solving a problem immediately after a worked example (Van Gog et al., Citation2011).
The worked example effect has been investigated in different domains with well-structured as well as ill-structured problems, such as mathematics (Retnowati et al., Citation2010), arts (Rourke & Sweller, Citation2009), and physics (Van Gog et al., Citation2011). The results consistently demonstrated the effectiveness of using worked examples with novices at the initial learning stages. However, some factors may moderate the worked example effect, such as the level of element interactivity of learning materials and learner levels of expertise.
Element interactivity and the worked example effect
Element interactivity is a concept reflecting the nature of learning materials (Sweller, Citation2010). Based on the number of interactive elements processed simultaneously in working memory, learning materials could range from low to high in element interactivity. The level of element interactivity is not an absolute but a relative measurement of the complexity of learning materials. For example, learning different pronumerals, x and y, is considered as low in element interactivity, as when learning x, students do not need to refer to the y, therefore, the two pronumerals could be processed in working memory separately and individually, indicating only one element being processed in working memory (the degree of element interactivity for this material is 1). However, compared to learning pronumerals, solving an equation, such as 3x + 5 = 8, could be high in element interactivity, as students must at least process all the elements (3, x, +, 5, =, 8) simultaneously in working memory in order to understand and solve the equation, rendering at least 6 interactive elements being processed in working memory (the degree of element interactivity for this material is at least 6).
Experiments have consistently found the worked example effect with materials high in element interactivity rather than materials low in element interactivity (Chen et al., Citation2016, Citation2020; Kyun et al., Citation2013; Renkl, Citation2002; Rourke & Sweller, Citation2009). In mathematics learning, Chen et al. (Citation2020) taught novices to calculate the area of compound shapes (high in element interactivity) and some basic geometric formulas (low in element interactivity, compared to the other task). The worked example effect was found when teaching novices calculating the area of compound shapes, but it disappeared when teaching basic formulas. In the domain of English literature, Kyun et al. (Citation2013) recruited students who were not native speakers and found a worked example effect with students who had less knowledge in the domain (materials high in element interactivity), but the effect became weaker with more knowledgeable students (the same materials became low in element interactivity). The results of the experiments suggested that learners’ experience interacting with element interactivity in the domain plays a critical role influencing the effectiveness of using worked examples for teaching (Chen et al., Citation2017).
Expertise and the worked example effect
If for a given topic, using worked examples is effective for novices as they do not have prior knowledge of the subject, whereas, learning with worked examples leads to worse performance for knowledgeable learners (compared to learning from soling problems), it indicates an expertise reversal effect (Kalyuga, Citation2007). The change of expertise in the given domain also changes the levels of element interactivity of learning materials. For novices, solving an equation, such as 3x + 5 = 8, would cause at least 6 interactive elements being processed in working memory, but for knowledgeable learners, the equation could be treated as a single entity due to relevant schemas acquired, which reduces the number of interactive elements to 1. The materials high in element interactivity for novices, become low in element interactivity for more knowledgeable learners. Therefore, the effectiveness of using worked examples also depends on learners’ expertise in the domain (i.e. Nückles et al., Citation2010; Oksa et al., Citation2010; Spanjers et al., Citation2011).
Worked example effect on transfer of knowledge
Transfer of knowledge is an important learning outcome of students’ learning. Using worked examples for learning have been shown to be effective to promote transfer of knowledge in some studies. In the domain of mathematics, Retnowati et al. (Citation2010) randomly assigned participants into four groups, either individual or collaborative learning and either learning with worked examples or leaning by problem solving. The numeric and reasoning skills were measured on both similar and transfer (conceptually different) tests. Transfer of knowledge of reasoning was found in both settings when worked examples are given. Schwonke et al. (Citation2009) investigated the worked examples with geometry materials. The worked example group outperformed the problem-solving group on both the conceptual and procedural transfer performances. Kyun et al.’s study (2015) designed worked examples for teaching conceptual and procedural knowledge of algebraic equations in secondary school. Students in the worked example group outperformed their peers on transfer tests. In science, Glogger-Frey et al. (Citation2015) found that both university and school students learning with worked examples performed better than their peers who were not given worked examples on both the near and far transfer tests (Glogger-Frey et al., Citation2015).
Motivational perspective of example-based learning
Van Gog et al. (Citation2011) argued that teaching with worked example-problem solving pairs would make students more motivated to apply the knowledge acquired from worked examples when solving immediately following problems. However, not much evidence has been obtained on the motivational perspective of the effect of worked examples on learning. Likourezos and Kalyuga (Citation2017) adapted a motivational questionnaire to measure the levels of interest and probability of success, challenge and anxiety in worked example-based learning. They found that the worked example group showed higher levels of interest, probability of success but a lower level of challenge, compared to the problem-solving group.
Teaching cognitively demanding and challenging problems has been an important part of reforming mathematics education (Russo & Minas, Citation2021). Therefore, levels of challenge are closely relevant affective, motivational factor, compared to other factors, when learning mathematics. In this study, a part of the motivational questionnaire (i.e. the level of challenge), applied by Likourezos and Kalyuga (Citation2017), is used to provide more evidence about the motivational perspective of example-based mathematics learning, which could serve as supplementary evidence of reduced cognitive load in example-based learning.
Worked example effect on steps
The traditional worked example effect focuses more on the macro level of learning, with study participants typically being requested to rate their cognitive effort after learning a worked example – problem solving pair, reflecting perception of overall load. Although it is an effective way to investigate the worked example effect, such an approach does not provide more micro-level details of the effectiveness of using worked examples during learning, namely, whether the effectiveness of using worked examples starts when students solving the first step of the problem or only has large effect on the last few steps when students solving a problem. To answer this question and to reveal more of the process of learning, it is necessary to test the worked example effect at a micro level.
Investigating the worked example effect on steps was initiated in Chen et al.’s (Citation2019) study, in which it was hypothesised that for a multiple-step problem, the level of element interactivity would decrease when steps proceed. It may be explained as Step 1 would normally have the highest level of element interactivity, Step 2 would have a higher level of element interactivity compared to Step 3, and so on. For example, when solving an equation 3x + 5 = 8 for x, besides holding 3, =, x in the working memory, the first step involves moving the + 5 to the right (1 element) and then change it to be − 5 (1 element) and doing the subtraction (8 − 5) (1 element) to get 3x = 3, therefore, at least 6 interactive elements being processed in working memory. In terms of Step 2, except holding = and x in working memory, both sides need to be divided by 3 (1 element) to get 3x/3 = 3/3, indicating 3 interactive elements. The last step, holding = in working memory, the 3s are cancelled on both sides (1 element), suggesting 2 interactive elements. Therefore, the levels of element interactivity are decreased when steps are proceeded.
Chen et al. (Citation2019) tested the relations between the worked example effect and steps for both novices and experts on a retention test, suggesting a worked example effect for overall performance, but gradually decreased effect from Step 1 (highest in element interactivity) to Step 3 (lowest in element interactivity) for novices, and no worked example effect was found on either overall performance or step performance for experts. The two experiments clearly indicated the worked example effect at the micro level (step performance) and macro level (overall performance). However, the study did not investigate the worked example effect at the micro level during learning and its consequences for transfer test performance. Investigating the effectiveness of worked examples during learning may reveal more insights on the mechanisms behind the superiority of worked examples as instructional means.
Present study
This study is built on Chen et al.’s (Citation2019) experiments but extends their idea by measuring cognitive load, challenge at the micro level (step) during learning and at the macro level (overall performance) of both retention and near-transfer tests, to investigate micro-level and macro-level worked example effect in the domain of mathematics. It is hypothesised that:
During learning, the worked example group will report a lower level of cognitive load, compared to the problem-solving group. Since the levels of element interactivity are gradually decreased, the levels of cognitive load will be decreased from Step 1 to Step 2 and to Step 3 with accordingly reduced effect.
During learning, the worked example group will report a lower level of both challenge and proudness, compared to the problem-solving group. Since the levels of element interactivity are gradually decreased, the levels of both challenge and proudness will be decreased from Step 1 to Step 2 and to Step 3 with accordingly reduced effect.
The worked example group will be superior to the problem-solving group on both retention and near-transfer tests.
Method
An experiment comparing worked examples with problem solving was designed to investigate the effect of worked examples on cognitive load and challenge at the micro level during learning, and on both retention and near-transfer tests at the macro level after learning, in teaching opening across the brackets for Year-7 students in Indonesia. This study was approved by the University ethics committee (231/UN34.21/TU/2018).
Participants
The priori power analysis was conducted using G*Power version 3.1 (Faul et al., Citation2007) for sample size estimation. Based on Chen et al.’s (Citation2019) study with the obtained effect size of .21 (partial eta-squared), we also expected a large effect size in this study. With a significant criterion of α = 0.05 and power = 0.80, the minimum sample size suggested for a large effect size was N = 98. 114 students from the same school were recruited for this study; the mean age of the worked example and problem-solving groups were 12.52 and 12.48 respectively. Participants were 65 females (57.52%; 37 in the worked example group and 28 in the problem-solving group) and 49 males (42.48%; 19 in the worked example group and 30 in the problem-solving group), so there were 56 in the worked example group and 58 in the problem-solving group. All participants were novices in opening across brackets.
Materials
Two teaching slides were designed for the worked example group and the problem-solving group separately. For the problem solving group, the teaching slides presented two algebraic expressions as Question 1 and Question 2 for opening across the brackets sequentially by steps, such as (3x + 1)(x − 2). Each question could be solved by 3 steps: Step 1, opening across brackets using the distributive law for Step 2, calculating the multiplications in step for
Step 3: collecting like terms of
for
Participants in the problem-solving group, therefore, were required to give the answer for each step of each question. The only difference of the teaching slides for the worked example group was that the answer for each step was given for learning, therefore, there were two worked examples for them to learn how to solve the problems by steps.
Based on estimates of element interactivity, to learn Step 1, at least 8 interactive elements must be processed simultaneously in the working memory, for Step 2, at least four interacting elements, and Step 3, only two interacting elements. Therefore, the levels of element interactivity gradually decreased across steps.
The 7-point Paas scale (Paas, Citation1992) was used to measure the cognitive load for either solving (problem-solving group) or learning (worked example group) each step during learning, namely, after solving (problem-solving group) or learning (worked example group) each step, the cognitive load scale was presented to indicate the level of mental effort invested in each step of both questions during learning by circling a number between 1 and 7 (1: very little effort; 7: very much effort). The Cronbach alpha of the cognitive load measure was 0.943.
The motivational rating scale used in this study consisted of two items categorised as a challenge and had been previously applied by Likourezos and Kalyuga (Citation2017). The items were ‘I was proud of my achievement for completing this step’; ‘This is a challenging step for me’. For each item, participants needed to circle a number from 1 to 7 to show strong disagreement (1) or strong agreement (7) with the statement. The questionnaire was presented after solving (the problem-solving group) or learning (the worked example group) each step for both questions during learning, therefore, the two items would reveal how challenging was each step in answering both questions across different groups. The Cronbach alpha of the motivational questionnaire was 0.801.
A retention test was designed based on the teaching slides. Five questions that were similar to those in the teaching slides were used for testing opening across the brackets. A near-transfer test, including five questions that were structurally different from the questions used during learning, was designed with questions like (–2x + y)2 to test opening across the brackets. The Cronbach alpha of the retention test was 0.959 and the Cronbach alpha of the near-transfer test was 0.850.
Procedure
Participants were randomly assigned to be in either the worked example group or problem-solving group before the experiment. The two questions in the teaching slides were presented sequentially to each group for 15 min, including studying the solution or solving the questions by steps and rating the levels of cognitive load and challenge for each step. After the learning phase, a retention test and a near-transfer test were conducted for 30 min in total to test the participants’ skills in opening across the brackets. All the slides, ratings and tests for both groups were delivered by the same mathematics teacher in the classrooms.
Scoring
Cognitive load and motivational scales
During learning, there were two pairs of worked examples for the worked example group and problems to solve for the problem-solving group, so the cognitive load and motivational ratings for each step of both pairs were averaged for final analyses.
Post-tests
The full mark for each question, in the retention and near-transfer tests (5 questions each), was 3 points (1 point for each step), therefore, the full mark for each test was 15 points. The total score for the five questions was transformed to the percentage-correct score (ranging from 0 to 100) for both the retention and near-transfer tests. During marking, the carry-over calculation errors for each step were considered, namely, the correctness of each step depended on the immediately preceding step. Therefore, even though some values were not actually correct, they were still counted as correct (i.e. step correctness was considered not in absolute, but in relative terms – relative to the immediately preceding step).
Results
Cognitive load during learning
To unpack the effect of worked examples on steps during learning, a 2 (group: worked example vs. problem solving) × 3 (steps: 1, 2, and 3) ANOVA was used, where group was a between-subject factor and ratings of cognitive load for different steps were repeatedly measured. The means and standard deviations of averaged cognitive load ratings are presented in .
Table 1. Means and standard deviations of averaged cognitive load ratings during learning.
The main effect of the group was significant, F(1,112) = 28.29, p < .001, partial eta squared = 0.20, which indicates that overall the worked example group reported significantly lower cognitive load than the problem-solving group. The effect of the step on cognitive load was significant, F(2, 224) = 4.61, p = .011, partial eta squared = 0.04. The interaction between the group and steps was significant, F(2, 224) = 4.87, p = .009, partial eta squared = 0.04. Following the significant interaction, simple effect analyses were conducted on each step. The worked example group reported significantly lower levels of cognitive load for all steps, compared to the problem-solving group, with the effect being increased first then decreased: For Step 1: t(112) = −4.56, p < .001, d = 0.79; for Step 2: t(112) = −6.25, p < .001, d = 1.00; for Step 3: t(112) = −4.09, p < .001, d = 0.71.
Motivational scales during learning
Level of proudness
A 2 (group: worked example vs. problem solving) × 3 (steps: 1, 2, and 3) ANOVA was used to analyse the item 1 (‘I was proud of my achievement for completing this step’) during learning, where group was a between-subject factor and the averaged motivational ratings were repeatedly measured for different steps. The means and standard deviations of averaged proudness scores on each step are presented in .
Table 2. Means and standard deviations of averaged proudness scores.
The main effect of group on proudness was not significant, F(1, 112) = 0.88, p = .35, partial eta squared = 0.01, indicating that the problem-solving group did not feel more proud after solving problems compared to the worked example group learning with examples. The main effect of the step on proudness was not significant, F(2, 224) = 2.4, p = .09, partial eta squared = 0.02. The interaction between the group and proudness was also not significant, F(2, 224) = 0.85, p = .43, partial eta squared = 0.01.
Level of challenge
A 2 (group: worked example vs. problem solving) × 3 (steps: 1, 2, and 3) ANOVA was used to analyse the item 2 (‘This is a challenging step for me’) during learning, where group was a between-subject factor and the averaged motivational ratings were repeatedly measured for different steps. The means and standard deviations of averaged challenge scores on each step are presented in .
Table 3. Means and standard deviations of averaged challenge scores.
The main effect of group on challenge was significant, F(1, 112) = 16.23, p < .001, partial eta squared = 0.13, indicating that the problem-solving group generally faced more challenge compared to the worked example group. The effect of the step on the challenge was significant, F(2, 224) = 3.74, p = .025, partial eta squared = 0.03. The interaction between the group and challenge was also significant, F(2, 224) = 4.91, p = .008, partial eta squared = 0.04. The simple analyses indicated that on all steps, the problem solving group experienced a significantly higher level of challenge than the worked example group, with the effect being increased first then decreased: For Step 1: t(112) = −2.82, p = .006, d = 0.51; for Step 2: t(112) = −4.84, p < .001, d = 0.83; for Step 3: t(112) = −3.66, p < .001, d = 0.65.
Retention and near-transfer tests
2 (group: worked example vs. problem solving) × 2 (tests: retention and transfer) ANOVA was used with the second factor being repeatedly measured. The mean and standard deviations of retention and near-transfer test scores are presented in . The main effect of the group was significant, F(1,112) = 10.41, p = .002, partial eta squared = 0.09, which indicates that the worked example group outperformed the problem-solving group on both the retention and near-transfer tests. The interaction between the test and group was significant, F(1,112) = 9.75, p = .002, partial eta squared = 0.08. The simple analyses revealed that the worked example group outperformed the problem-solving group on both the retention test, t(112) = 3.75, p < .001, d = 0.67 and near-transfer test, t(112) = 2.03, p = .044, d = 0.37. The relatively weaker worked example effect on the near-transfer test explains the interaction effect.
Table 4. Means and standard deviations of the percentage-correct scores for retention and near-transfer.
Correlations among cognitive load, challenge and post-tests
To further investigate the relations among cognitive load, challenge and post-tests (both retention and near-transfer tests), correlational analyses were conducted. The cognitive load and challenge were positively correlated: for Step 1, the higher cognitive load was associated with higher challenge, r(114) = 0.35, p < .001, the same pattern for Step 2, r(114) = 0.38, p < .001, and for Step 3, r(114) = 0.41, p < .001. The cognitive load and post-tests (both retention and near-transfer tests) were negatively correlated: The higher cognitive load of Step 1, the lower retention score, r(114) = −0.45, p < .001, and near-transfer score, r(114) = −0.39, p < .001, the same pattern for Step 2 on the retention score, r(114) = −0.50, p < .001, and the near-transfer score, r(114) = −0.40, p < .001, again for Step 3 on the retention score, r(114) = −0.49, p < .001, and the near-transfer score, r(114) = −0.43, p < .001. The challenge and post-tests (both retention and near-transfer tests) were also negatively correlated: The higher challenge of Step 1, the lower retention score, r(114) = −0.23, p < .001, and near-transfer score, r(114) = −0.30, p < .001; for Step 2, the negative correlation was only for the retention score, r(114) = −0.23, p < .001; Step 3 showed the same pattern of Step 1 on the retention score, r(114) = −0.21, p < .001, and the near-transfer score, r(114) = −0.22, p < .001.
The correlational results were in line with cognitive load theory hypotheses. When the cognitive load is high (particularly when the learning materials are high in element interactivity), the learning performance would be interfered with. Interestingly, the positive correlation between cognitive load and challenge suggests the associations between cognition and motivation, namely, the high cognitive load would lead to high challenge, which may suggest cognitive load theory to include some motivational factors in future studies.
Discussion
The reported experiment was designed to investigate the worked example effect during learning at the micro level (i.e. the level of individual solution steps) and on both retention and near-transfer tests at the macro level. Unpacking the worked example effect during learning has the potential to reveal more refined mechanisms responsible for the effectiveness of worked examples in comparison to pure problem-solving. The majority of worked example studies have focused more on the macro level during learning, such as the overall cognitive load during learning, without investigating relations between cognitive load and instructional consequences of worked examples at micro level and motivational factors. This study further extended the approach suggested by Chen et al. (Citation2019) investigating the relations between cognitive load and the effectiveness of worked examples during learning on the level of individual solution steps (i.e. the micro level) with measuring levels of challenge (one of the most relevant motivational factors) during learning of steps and testing the worked example effect not only with a retention test but also with a structurally different transfer test at macro level.
Based on the results for cognitive load ratings during learning, Hypothesis 1 was partially supported. The worked example group reported a lower level of cognitive load on each step, compared to the problem-solving group. However, the effect size did not gradually decrease from Step 1 to Step 2, and to Step 3 in accordance with the decreased level of element interactivity. The effect size actually increased from Step 1 to Step 2, then decreased from Step 2 to Step 3. Although Step 2 had a lower level of element interactivity compared to Step 1, using worked examples demonstrated a stronger impact on learning Step 2 (mid-step) during learning. It could be suggested that during learning, the mid-step was linked to both the first and the third (final) steps, therefore, extra working memory resources might be required to understand the relations of the mid-step with the previous and following steps. In Chen et al. (Citation2019), the effect size of the worked example provision on each step of the post-test gradually decreased. It may be explained by taking into account that after the learning phase was completed, students might only need to focus on calculations during the test, as the relations of mid-steps with the previous and following steps had been acquired during the learning phase.
The challenge scores during learning also partially supported Hypothesis 2 and showed a similar pattern to cognitive load scores on each step during learning, with no effects being found for the proudness scores. The results indicated that learning with worked examples imposed less challenge compared to learning by problem solving for each step. The results consistently showed that the problem-solving group faced a higher level of challenge than the worked example group but did not feel more proud after problem solving compared to learning with examples. This extended the results of Chen et al. (Citation2019) and replicated the results of Likourezos and Kalyuga (Citation2017). Similar to cognitive load ratings, the effect size for each step increased from Step 1 to Step 2, then decreased from Step 2 to Step 3. A similar explanation to that provided for the cognitive load might be applicable here. Generally, the results for challenge scales confirmed the results for cognitive load ratings, showing that during learning, using worked examples would reduce cognitive load, and the reduced cognitive load would lead to less challenge for learning, compared to learning by problem solving.
The results for retention and near-transfer tests confirmed Hypothesis 3. The worked example group outperformed the problem-solving group on both the retention and transfer tests. The worked example effect as measured by the retention tests has been supported by the majority of studies (e.g. Chen et al., Citation2015; Cooper & Sweller, Citation1987; Renkl et al., Citation2002) with some evidence of its transfer effect on learning (e.g. Schwonke et al., Citation2009). The less widespread result was that the worked example effect was also found on a transfer test with structurally different questions, namely, the worked example group outperformed the problem-solving group on a near-transfer test. This result is in line with the results of Cooper and Sweller (Citation1987), who established that worked examples enhanced learning on structurally different questions. Although in this study, the worked example group also outperformed the problem-solving group on the near-transfer test, the effect size was smaller compared to the effect size on the retention test which could be attributed to the fact that the instruction phase of this study was rather short and did not provide enough opportunities for schema automation as a prerequisite for transfer according to Sweller and Cooper (Citation1985). Therefore, in this study, the worked example effect was more distinctive on structurally similar problems to those used during learning.
Combining the results of cognitive load and challenge during learning, the worked example effect on the near-transfer test could be readily explained. Students in the worked example group during learning had more working memory resources (lower cognitive load) to deal with the structures and principles used for solving a relatively novel problem, compared to the problem-solving group. The well-structured solutions presented in the worked examples would provide less challenge for the worked example group in learning the structure and principles for solving the given class of problems, as they could directly focus on the key parts without the need to search for such key parts in a rather random way. Therefore, the worked example group would be better prepared to solve structurally different problems in the near-transfer test.
Theoretical contributions
This study conceptually replicates Chen et al.’s (Citation2019) study but extends that study by investigating the worked example effect at a micro level during learning (i.e. individual steps) with motivational and cognitive load ratings and at a macro level on transfer test. The results of this study may provide further theoretical explanation to the faded worked example strategy (Renkl & Atkinson, Citation2003). When learning with worked examples, the levels of cognitive load and challenge are gradually reduced with progressing steps, therefore, the worked examples may be replaced by completion problems for later steps. For example, for Step 1 which has the highest level of element interactivity, guidance by worked examples is needed, but the last step with the lowest level of element interactivity (and, accordingly, the smallest effect size), the guidance by worked examples may be replaced by problem completion.
Another important theoretical contribution is unpacking the worked example effect during learning (compared to the previous studies on the worked example effect that focused mostly on post-learning test scores), which reveals more details of cognitive mechanisms explaining the effectiveness of this effect in fostering learning.
The observed positive correlation between cognitive load and challenge (motivational factor) may serve as an important argument for broadening the research in cognitive load theory by including some motivational factors in future studies.
Educational implications
Based on the unpacked mechanisms of using worked examples during learning, we have a better understanding of why they are superior to problem solving, not only from the cognitive load perspective on the macro level (i.e. retention and transfer post-tests), but also from the motivational perspective and the micro-level of analysis (i.e. level of individual solution steps). Therefore, when teaching novices, providing explicit instruction during the first few steps would reduce the imposed cognitive load and make students less challenged during learning, but with decreasing levels of complexity and challenge at the later steps of learning, explicit instruction may be omitted by using problem solving. Eventually, the benefits of teaching with worked examples would be the enhanced learning outcomes and fostered transfer of knowledge.
Limitations
The study focused only on a limited range of tasks in mathematics learning of secondary school students. It is not clear whether similar patterns would be found for other subjects and other levels of students, particularly in ill-structured domains, or for younger students. Further studies using diverse task domains and participants are needed.
Authors contributions
Ouhao Chen: conceptualisation, research design, data analysis and writing
Endah Retnowati: data collection and research design
BoBo Kai Yin Chan: data analysis
Slava Kalyuga: conceptualisation and writing
Disclosure statement
No potential conflict of interest was reported by the author(s).
Additional information
Funding
References
- Chen, O., Kalyuga, S., & Sweller, J. (2015). The worked example effect, the generation effect, and element interactivity. Journal of Educational Psychology, 107(3), 689–704. https://doi.org/10.1037/edu0000018
- Chen, O., Kalyuga, S., & Sweller, J. (2016). Relations between the worked example and generation effects on immediate and delayed tests. Learning and Instruction, 45, 20–30. https://doi.org/10.1016/j.learninstruc.2016.06.007
- Chen, O., Kalyuga, S., & Sweller, J. (2017). The expertise reversal effect is a variant of the more general element interactivity effect. Educational Psychology Review, 29(2), 393–405. https://doi.org/10.1007/s10648-016-9359-1
- Chen, O., Retnowati, E., & Kalyuga, S. (2019). Effects of worked examples on step performance in solving complex problems. Educational Psychology, 39(2), 188–202. https://doi.org/10.1080/01443410.2018.1515891
- Chen, O., Retnowati, E., & Kalyuga, S. (2020). Element interactivity as a factor influencing the effectiveness of worked example–problem solving and problem solving–worked example sequences. The British Journal of Educational Psychology, 90(S1), 210–223. https://doi.org/10.1111/bjep.12317
- Cooper, G., & Sweller, J. (1987). Effects of schema acquisition and rule automation on mathematical problem-solving transfer. Journal of Educational Psychology, 79(4), 347–362. https://doi.org/10.1037/0022-0663.79.4.347
- Cowan, N. (2001). The magical number 4 in short-term memory: A reconsideration of mental storage capacity. The Behavioral and Brain Sciences, 24(1), 87–114. https://doi.org/10.1017/S0140525X01003922
- Faul, F., Erdfelder, E., Lang, A. G., & Buchner, A. (2007). G* Power 3: A flexible statistical power analysis program for the social, behavioral, and biomedical sciences. Behavior Research Methods, 39(2), 175–191. https://doi.org/10.3758/bf03193146
- Glogger-Frey, I., Fleischer, C., Grüny, L., Kappich, J., & Renkl, A. (2015). Inventing a solution and studying a worked solution prepare differently for learning from direct instruction. Learning and Instruction, 39, 72–87. https://doi.org/10.1016/j.learninstruc.2015.05.001
- Kalyuga, S. (2007). Expertise reversal effect and its implications for learner-tailored instruction. Educational Psychology Review, 19(4), 509–539. https://doi.org/10.1007/s10648-007-9054-3
- Kyun, S., Kalyuga, S., & Sweller, J. (2013). The effect of worked examples when learning to write essays in English literature. The Journal of Experimental Education, 81(3), 385–408. https://doi.org/10.1080/00220973.2012.727884
- Kyun, S., Lee, J. K., & Lee, H. (2015). The worked example effect using ill-defined problems in on-line learning: Focus on the components of a worked example. Journal of the Korea Society of IT Services, 14(1), 129–143. https://doi.org/10.9716/KITS.2015.14.1.129
- Likourezos, V., & Kalyuga, S. (2017). Instruction-first and problem-solving-first approaches: Alternative pathways to learning complex tasks. Instructional Science, 45(2), 195–219. https://doi.org/10.1007/s11251-016-9399-4
- Miller, G. A. (1994). The magical number seven, plus or minus two: Some limits on our capacity for processing information. Psychological Review, 101(2), 343–352. https://doi.org/10.1037/h0043158
- Nückles, M., Hübner, S., Dümer, S., & Renkl, A. (2010). Expertise reversal effects in writing-to-learn. Instructional Science, 38(3), 237–258. https://doi.org/10.1007/s11251-009-9106-9
- Oksa, A., Kalyuga, S., & Chandler, P. (2010). Expertise reversal effect in using explanatory notes for readers of Shakespearean text. Instructional Science, 38(3), 217–236. https://doi.org/10.1007/s11251-009-9109-6
- Paas, F. G. W. C. (1992). Training strategies for attaining transfer of problem-solving skill in statistics: A cognitive-load approach. Journal of Educational Psychology, 84(4), 429–434. https://doi.org/10.1037/0022-0663.84.4.429
- Paas, F., & van Merriënboer, J. J. (2020). Cognitive-load theory: Methods to manage working memory load in the learning of complex tasks. Current Directions in Psychological Science, 29(4), 394–398. https://doi.org/10.1177/0963721420922183
- Peterson, L., & Peterson, M. J. (1959). Short-term retention of individual verbal items. Journal of Experimental Psychology, 58(3), 193–198. https://doi.org/10.1037/h0049234
- Renkl, A. (2002). Worked-out examples: Instructional explanations support learning by self-explanations. Learning and Instruction, 12(5), 529–556. https://doi.org/10.1016/S0959-4752(01)00030-5
- Renkl, A., & Atkinson, R. K. (2003). Structuring the transition from example study to problem solving in cognitive skill acquisition: A cognitive load perspective. Educational Psychologist, 38(1), 15–22. https://doi.org/10.1207/S15326985EP3801_3
- Renkl, A., Atkinson, R. K., Maier, U. H., & Staley, R. (2002). From example study to problem solving: Smooth transitions help learning. The Journal of Experimental Education, 70(4), 293–315. https://doi.org/10.1080/00220970209599510
- Retnowati, E., Ayres, P., & Sweller, J. (2010). Worked example effects in individual and group work settings. Educational Psychology, 30(3), 349–367. https://doi.org/10.1080/01443411003659960
- Rourke, A., & Sweller, J. (2009). The worked-example effect using ill-defined problems: Learning to recognise designers’ styles. Learning and Instruction, 19(2), 185–199. https://doi.org/10.1016/j.learninstruc.2008.03.006
- Russo, J., & Minas, M. (2021). Student attitudes towards learning mathematics through challenging, problem-solving tasks: “It’s so hard – in a good way”. Lnternational Electronic Journal of Elementary Education, 13(2), 215–225. https://doi.org/10.26822/iejee.2021.185
- Schwonke, R., Renkl, A., Krieg, C., Wittwer, J., Aleven, V., & Salden, R. (2009). The worked-example effect: Not an artefact of lousy control conditions. Computers in Human Behavior, 25(2), 258–266. https://doi.org/10.1016/j.chb.2008.12.011
- Spanjers, I. A., Wouters, P., Van Gog, T., & Van Merrienboer, J. J. (2011). An expertise reversal effect of segmentation in learning from animated worked-out examples. Computers in Human Behavior, 27(1), 46–52. https://doi.org/10.1016/j.chb.2010.05.011
- Sweller, J. (2010). Element interactivity and intrinsic, extraneous, and germane cognitive load. Educational Psychology Review, 22(2), 123–138. https://doi.org/10.1007/s10648-010-9128-5
- Sweller, J., & Cooper, G. A. (1985). The use of worked examples as a substitute for problem solving in learning algebra. Cognition and Instruction, 2(1), 59–89. https://doi.org/10.1207/s1532690xci0201_3
- Sweller, J., van Merriënboer, J. J., & Paas, F. (2019). Cognitive architecture and instructional design: 20 years later. Educational Psychology Review, 31(2), 261–292. https://doi.org/10.1007/s10648-019-09465-5
- Van Gog, T., Kester, L., & Paas, F. (2011). Effects of worked examples, example-problem, and problem-example pairs on novices’ learning. Contemporary Educational Psychology, 36(3), 212–218. https://doi.org/10.1016/j.cedpsych.2010.10.004