Abstract
This study aimed to understand the role of load reduction instruction (LRI), a framework of instructional strategies designed to reduce cognitive load, and its association with motivation, engagement, and learning outcomes in mathematics. We examined data from 221 students from 39 classrooms and tested a hypothesised longitudinal multilevel model. The results indicated positive associations of LRI with gains in motivation, engagement, and achievement. These effects were evident at both student- and classroom-levels, after accounting for prior variance, and after accounting for age, gender, and socioeconomic status at the student level. The findings are discussed in light of the importance of considering motivation and engagement as vital educational outcomes alongside achievement, as well as the role of cognitive load reduction strategies—including those associated with explicit instruction and independent enquiry—in optimising these outcomes.
Load reduction instruction (LRI) is a framework of teaching strategies aimed at easing the burden on novice learners’ working memory in the early stages of the learning process, and then allowing more self-direction and independence as learners acquire knowledge and skills required to progress to more advanced levels (Martin, Citation2016; Martin & Evans, Citation2018; Evans & Martin, Citation2023). The rationale for integrating these strategies into a single framework was that there are appropriate points in the learning process where learners will benefit from structured and closely scaffolded instruction (when they are novices) and then other points in the learning process when guided independence is important (as expertise develops; Kalyuga, Citation2007). LRI therefore challenges the false dichotomy that can exist between more structured (positivist and post-positivist) approaches to instruction and more independent (social-constructivist) approaches (see also Liem & Martin, Citation2013). The LRI framework comprises five elements of instruction based on unifying a range of cognitive load theory effects: (1) reducing difficulty during initial stages of learning, (2) providing instructional support and scaffolding, (3) providing ample time for structured practice, (4) providing instructional feedback-feedforward, and (5) independent practice and guided autonomy (Martin, Citation2016; Martin & Evans, Citation2018, Citation2019).
It is well-established that teaching strategies such as those incorporated into the LRI framework are effective at reducing extraneous cognitive load and facilitating achievement (Evans & Martin, Citation2023). However, less is known about whether students find these strategies motivating and engaging. The purpose of the present investigation is therefore to examine the association between LRI and motivation and engagement. This research extends a previous measurement study into LRI by conducting a longitudinal assessment of students’ motivation, engagement, and achievement in mathematics (Martin & Evans, Citation2018). That measurement work showed significant cross-sectional correlations between LRI and various academic outcomes. By controlling for prior motivation, engagement, and achievement in the present study we were able to extend understanding of LRI associations to that reflecting gains (or declines) across time. Moreover, because the present study adopted multilevel analysis (students at level 1 and classrooms at level 2), we could ascertain the extent to which there is a significant role for LRI at the whole-class level beyond the role of LRI in individual students’ academic lives. Previous multilevel findings have indicated LRI may operate in this way at both student and classroom levels with relation to engagement (Martin et al., Citation2021a, Citation2021b; Citation2023), but further work is needed to understand this with a longitudinal design. Additionally, previous findings (Martin et al., Citation2021a) indicated positive associations between LRI and positive motivational dimensions (e.g., self-efficacy; growth goals), but mixed findings in relation to other constructs that may affect engagement (e.g., anxiety, failure avoidance).
Cognitive load theory
According to cognitive load theory (Sweller, Citation2011; Sweller et al., Citation2011), two key components of human cognitive architecture have implications for instruction, particularly in the initial stages of learning (when students are novices): working memory and long-term memory. Working memory is a memory component that retains a small amount of information in conscious attention (Cowan, Citation2014). Models of the structure and limitations of working memory have evolved in recent decades, and debate is ongoing about whether working memory comprises multiple components, such as a for phonological and visual-spatial information (Baddeley, Citation2012); whether working memory might better be explained as the activation of long-term and sensory memory (Cowan, Citation2014); the role of timing and pace of new, incoming information (Puma et al., Citation2018); and the need to consider this range of perspectives and the role of attention (Fenesi et al., Citation2015). Across these perspectives, however, there is a clear implication for learning and instruction, which is that working memory has a very limited capacity with respect to novel information. That is, it can hold only a few units of information at a time, which are subject to interference and decay. In instructional settings, therefore, working memory resources must be carefully managed, as overwhelming them compromises learning (Sweller, Citation2011; Sweller et al., Citation2011) because there are no remaining cognitive resources available for processing the relevant information and encoding it into long-term memory.
Long-term memory, in contrast, has a theoretically unlimited capacity (Sweller, Citation2011). In addition to storing vast amounts of information, long-term memory can store complex relationships and processes. When information is familiar—when it is stored effectively in long-term memory—it can be recalled into working memory in ‘chunks’ without consuming a large amount of working memory resources (Cowan, Citation2010; Sweller, Citation2011; Sweller et al., Citation2011). This relationship with working memory can explain why people are capable of complex intellectual tasks (or, when people can be considered “experts”).
For the purposes of learning, cognitive load—the amount of information in working memory at any one time—is classified as one of two kinds (Sweller, Citation2011). Intrinsic cognitive load is the inherent difficulty of a learning task—the load imposed on working memory by the material to be learned. Information that is low in element interactivity (e.g., memorising a vocabulary list in a foreign language or the order of operations in mathematics) consumes less intrinsic load than information that is high in element interactivity (e.g., learning the grammar of a foreign language, applying the order of operations to solve simultaneous equations). It is possible to reduce intrinsic cognitive load by, for example, selecting easier material to learn (e.g., Ayres, Citation2006), but of course this is can ultimately impede learners in progressing through a prescribed curriculum. For this reason, intrinsic cognitive load is generally considered a fixed variable that cannot be manipulated by the teacher.
Extraneous cognitive load is the load imposed on working memory by the presentation of information. It is directly influenced by the method of instruction and is thus something that can be kept low without impeding learning. One example of extraneous cognitive load is the problem-solving effect—the finding that in lessons based on problem-solving activities, learners tend to identify potential solutions, work backwards from them, and trial a range of potential solutions at random. This process does not contribute to learning the relevant information, so it is considered extraneous cognitive load. In contrast, worked examples guide students through their learning by focusing on individual elements of problems and encoding them into long-term memory (Sweller, Citation2011). The primary method of testing cognitive load theory is in laboratory and classroom-based experiments using randomised, controlled designs to generate effects based on different instructional procedures (Sweller et al., Citation2011). The key conclusion drawn from this line of research is that optimising working memory by minimising extraneous cognitive load results in more effective learning.
Load reduction instruction (LRI)
Drawing on this body of research, LRI has been developed as an instructional framework and a set of teaching principles designed to manage cognitive load (Martin, Citation2016). Measurement work using the Load Reduction Instruction Scale (LRIS) has identified significant negative associations with extraneous cognitive load (Martin & Evans, Citation2018). Like cognitive load theory, LRI assumes that learners are at first novices with respect to the information that is to be learned. This means that they do not have schemas in long-term memory that can be recalled to working memory in relation to the tasks at hand. Instead, the information in working memory is novel. As noted above, the number of units of novel information that can be processed in working memory is limited, so too much novel information can quickly exceed working memory resources. Moreover, those units of information are likely to have some level of element interactivity. Difficulty is therefore experienced by the learner when there are simply too many novel elements to consider in working memory, or the elements are high in element interactivity—i.e., they need to be understood in terms of their relations to each other (Sweller et al., Citation2011). Therefore, when presenting novel information, instruction needs to consider the number of elements and the nature of element interactivity. LRI principle #1—reduce difficulty during initial stages of instruction as appropriate to the learner’s prior knowledge—is aimed at helping learners to deal with new topics or information without overwhelming their working memory. It is achieved by reducing element interactivity, using strategies such as pre-training, modelling processes, and showcasing (Martin, Citation2016).
As instructional sequences develop, the emphasis of instruction should be on activities that promote schema acquisition in long-term memory. Because learners are generally novices, they are unable to comprehend the structure of the material to be learned, and attempts to work out the structure of the material impose load and do not contribute to learning. LRI principle #2 therefore relates to providing scaffolding and support using strategies such as reducing split attention, organising information thematically, and providing worked examples (Martin, Citation2016). Rehearsal and practice are how schemas are constructed, so LRI principle #3 relates to teachers providing ample opportunities in class for students to practice, as well as explicitly showing students features of deliberate practice and techniques such as mental practice (Martin, Citation2016). The importance of teachers providing appropriate and useful feedback-feedforward is reflected in LRI principle #4, as feedback provides the requisite corrective information to students and the feedforward provides improvement-oriented guidance based on this corrective information (Hattie & Timperley, Citation2007).
The final LRI principle relates to circumstances where students have acquired a foundation of domain-specific knowledge about a particular concept or topic and are forming deeper understandings and connections. As students accumulate more domain-specific knowledge, they are more easily able to recall basic concepts to working memory with fluency and automaticity. For example, as students become more fluent at solving algebraic equations, teachers no longer need to explicitly point out rudimentary processes such as identifying like terms. Indeed, such assistance can hinder learning when it is not needed (Kalyuga, Citation2007). At this point, strategies favoured by social-constructivist approaches are incorporated (particularly a lower amount of input and guidance from the teacher, the use of real-world or ‘authentic’ problems, and an emphasis on learning arising from social interactions; see Tobias, Citation2009 for review). Thus, LRI principle #5 relates to providing opportunities for independent practice and application of knowledge to real-world situations once students have developed a solid understanding of the required knowledge.
The five LRI principles are shown in . The principles are presented loosely as a sequence bookended by Principle 1 (Difficulty Reduction) in the early stages of learning, and Principle 5 (Guided Independence) for when students have developed sufficient prior knowledge for activities of this type. Yet rather than structuring units of learning by sequencing through the five principles, the main consideration for what type of learning should happen is prior knowledge and how it interacts with novel information. For example, asking students to undertake extensive practice (Principle 3) without first checking whether they understand the relevant concepts or procedures is unlikely to be worthwhile. Similarly, even when students have substantial prior knowledge, the introduction of a novel, advanced concept might also benefit from reducing its difficulty (Principle 1).
Figure 1. Load reduction instruction (LRI) framework – adapted with permission from Martin (Citation2016).
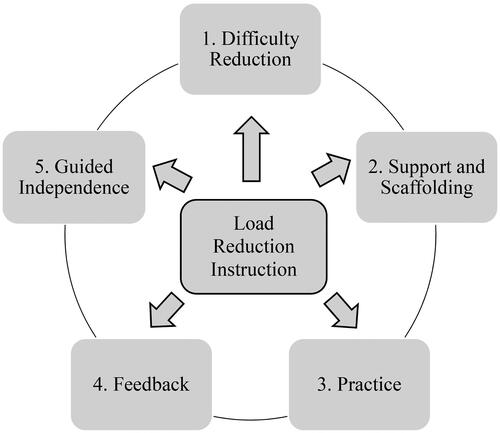
As well as providing an overall framework of strategies known to be effective for learning, LRI responds to a particular issue in educational debates about the amount of guidance and structure that should be provided during instruction (P. A. Kirschner et al., Citation2006; Martin, Citation2016; Mayer, Citation2004). Proponents of social-constructivist inspired approaches such as discovery learning, and to an extent inquiry-based learning, have argued that the role of the teacher should be reasonably minimal, and that an emphasis on generic inquiry or problem-solving skills should be made so that learners can experience the ‘discovery’ of information themselves (e.g., Hmelo-Silver, Citation2004). Proponents of cognitive information-processing approaches, in contrast, have argued that the teacher should be highly explicit with regard to the content that is to be taught, and should direct their students through internalising the structure of that content (P. A. Kirschner et al., Citation2006; Evans & Martin, Citation2023), with a view that any general problem-solving skill relies on the development of domain-specific knowledge (Tricot & Sweller, Citation2014). LRI recognises both positions and suggests that during the early stages of learning, more directed and explicit approaches are appropriate to the learner’s novice status. As learners develop knowledge within a domain, the increasing use of discovery and inquiry-oriented strategies is appropriate because learners can rely on their long-term memory and have more working memory resources available (Kalyuga, Citation2007; Martin, Citation2016; Sweller et al., Citation2003). In this way, LRI is framed on the understanding that certain features of teaching can be regarded as generally or universally effective (e.g., the provision of structure and scaffolding, effective feedback, sufficient time for practice) while at the same time acknowledging that some approaches more or less effective than others depending on the knowledge and experience of the learner.
LRI and implications for motivation, engagement, and achievement
Motivation
Motivation concerns the reasons for behaviour and the psychological processes involved in the initiation and persistence of goal pursuits (Ryan & Deci, Citation2017). LRI is theorised to impact motivation because by using LRI strategies, teachers explicitly communicate information that has motivating functions (Martin, Citation2016). This includes information about the activity itself (as in when the teacher explains the structure of a learning activity and what success looks like; LRI principle #2), the chances of success (as in when a teacher provides feedback-feedforward information that helps students feel efficacious, LRI principle #4), and the importance of the activity (as when students apply their knowledge to real-world problems, LRI principle #5). Motivation is important to study as an educational outcome, because of its role in students’ school and post-school wellbeing, its applicability across cultural contexts (Martin et al., Citation2015), and because it is the means by which people internalise the values of their social environments (Ryan & Deci, Citation2017). LRI has been associated with motivation in cross-sectional research in mathematics (Martin & Evans, Citation2018) and science (Martin et al., Citation2021a); in the latter, mixed results were found, with LRI generally associated with adaptive motivation, but also with anxiety and failure avoidance in some students.
Engagement
Engagement in learning concerns a range of ways learners can be present and involved in learning activities. It is generally considered a multidimensional construct: Engaged students not only show up to class, but they actively plan, monitor, and reflect on their learning (Fredricks et al., Citation2004). LRI is theorised to impact engagement by freeing cognitive resources to perform these cognitive and metacognitive activities. For example, without meaningful feedback-feedforward (LRI principle #4), students are unable to gauge their learning and plan subsequent study tasks (Hattie & Timperley, Citation2007). Teachers might explicitly teach students how to study and learn information within their domain so that time spent studying will be productive (LRI principle #3). LRI may also decrease disengagement; by helping students to navigate a path through learning a difficult topic (LRI principle #2), teachers may reduce the uncertainty with the topic which can underlie disengagement. In addition, by helping students to acquire skills through practice (LRI principle #3), they are more likely to be agentically engaged in independent study tasks. LRI has been associated with engagement cross-sectionally in mathematics (Martin & Evans, Citation2018), English (Martin et al., Citation2023), and science (Martin et al., Citation2021b); the latter explored a potential mediating relationship for engagement, but to date, no research using LRI has examined changes over time.
Achievement
LRI is theorised to impact on student learning, defined as a change in long-term memory (P. A. Kirschner et al., Citation2006), as indicated by measures of achievement (Martin & Evans, Citation2018). Achievement has long been the primary outcome of interest in research on cognitive load theory. The consistent finding from experimental research is that manipulating extraneous cognitive load in one way or another can impact learning. This gives rise to a number of cognitive load ‘effects’ that explain learning and achievement (Sweller et al., Citation2011). Examples include the split attention effect (Chandler & Sweller, Citation1992), the worked example effect (Sweller, Citation2006), and the problem-solving effect which in turn is associated with the expertise-reversal effect (the effect of providing too much scaffolding and assistance when students no longer need it, minimised by implementing LRI principle #5 at the appropriate stage of learning; Kalyuga, Citation2007). Previous research on LRI is consistent in finding a positive association with achievement (Martin et al., Citation2021b; Citation2023; Martin & Evans, Citation2018), but none has accounted for prior variance (i.e., attempted to model growth) in achievement.
Aims
The present study aimed to examine the role of LRI in motivation, engagement, and achievement in mathematics at the student- and classroom-levels. The study extended the foundational cross-sectional measurement research of Martin and Evans (Citation2018) which was the first to investigate the psychometric properties of the LRIS and its correlates, and subsequent cross-sectional LRI research in relation to motivation (Martin et al., Citation2021b) and engagement (Martin et al., Citation2021b; Citation2023). Specifically, by using longitudinal (matched) data and new variables to expand on the Martin and Evans (Citation2018) dataset, we were able to conduct further substantive and model-driven multilevel analyses to examine evidence for the role of LRI in predicting gains in student- and classroom-level mathematics outcomes. Following prior work (Martin, Citation2016; Martin & Evans, Citation2018; Sweller, Citation2011), we hypothesised that LRI would predict gains in students’ mathematics motivation, engagement, and achievement. This research extends previous measurement work by controlling for prior motivation, engagement, and achievement and therefore ascertain gains across in these factors over time. In addition, being a longitudinal multilevel investigation (students at level 1 and classrooms at level 2), the study expands understanding of LRI and academic gains at the whole-class level. Finally, prior such research into LRI has been conducted in science (Martin et al., Citation2021a, Citation2021b) and so this investigation into mathematics affords great insight into LRI in a different area of the academic curriculum.
Method
Participants and procedure
The matched longitudinal sample comprised 221 high school students in 39 mathematics classrooms. Students were surveyed twice, 6 months apart. Two schools participated; one school was a single-sex school (n = 95) and the other was co-educational (n = 126). The students were from Years 9 (n = 78), 10 (n = 92), and 11 (n = 51), their mean age was 15.52 (SD = 0.95), and 140 were females. Students were clustered in 39 classrooms, with the mean number of students per classroom of 6 (taking into account non-participation at one of the two timepoints and student absences; indeed, although a small number of clusters per class may bias model estimates in single-level modelling, this bias is not realised when using multilevel modelling as described below; Maas & Hox, Citation2005). The socioeconomic advantage of the students in the school was relatively high as indicated by the measure used by the Australian Bureau of Statistics (M = 1110, SD = 56) compared with the population (M = 1000, SD = 100). The study’s procedures were approved by the University Human Research Ethics Advisory Board (#HC14089) and by the administrations of the schools involved. Both parents/carers and students consented to participation before the survey.
Measures
The survey comprised measures of load reduction instruction, motivation, engagement, and achievement. For motivation, engagement, and achievement we had pre-test data from 6 months prior. For the motivation and LRI factors, we used the same measures as in Martin and Evans (Citation2018) but with the important addition of longitudinal motivation data. The longitudinal matched engagement and achievement measures were not in the Martin and Evans (Citation2018) study and so were a novel inclusion for this investigation. For the variables measured at both timepoints, the Time 1 and Time 2 measures were identical. For each of these measures, descriptive, distribution, and reliability (McDonald’s Omega [ω]) are presented in the following sections and in . All survey items were Likert-type scales with 7 points ranging from “strongly disagree” to “strongly agree.”
Table 1. Descriptive statistics for substantive variables.
Load-reduction instruction scale (LRIS)
The LRIS (Martin & Evans, Citation2018) is a 25-item measure, with 5 items each indicating difficulty reduction (e.g., “When we learn new things in this class, the teacher makes it easy at first”); support and scaffolding (e.g., “As we work on tasks or activities in this class, the teacher gives good assistance”); practice (e.g., “In this class, the teacher makes sure we get enough practice before moving on to new tasks and activities”); feedback-feedforward (e.g., “In this class, the teacher often tells us how to improve our work”); and guided independence (e.g., “Once we know what we’re doing in this class, the teacher gives us schoolwork to figure out by ourselves”). Students responded in their mathematics lesson and in relation to their mathematics instruction. The scale has demonstrated measurement invariance as a function of gender and age (Martin & Evans, Citation2018) and external validity with engagement and achievement in the science domain (Martin et al., Citation2021a, Citation2021b). The LRIS was estimated using factor scores from a confirmatory factor analysis (CFA), with a higher-order LRI factor indicated by five lower-order factors, each indicated by five items. Fit indices were acceptable and are shown in .
Table 2. Model fit for CFAs and longitudinal measurement invariance tests.
Motivation
Motivation was measured via the positive motivation dimension of the short form of the Motivation and Engagement Scale–High School (Martin, Citation2007), an instrument designed to measure motivation in the school or classroom context. Three items comprise the motivation factor (“I believe I can do a good job in my schoolwork”; “What I am doing in my schoolwork is important and useful”; “In my schoolwork, I am focused more on improving than on competing and being the best”), indicating the motivation dimensions of self-efficacy, subjective task value, and mastery orientation, respectively. The short form of the MES-HS has been validated with Australian high school students, demonstrating strong internal reliability and external validity (Liem & Martin, Citation2012). CFA models were fitted to test for measurement invariance between the two timepoints: First, a configural model was established, followed by nested models in which parameters were fixed to be equal across time: metric, scalar, residual, and full factorial invariance. Measurement invariance was rejected if the fit of the nested model was significantly worse than the previous model (based on a log-likelihood ratio test at p < .05). The final invariant model was used to estimate factor scores in the path analysis. Fit indices were acceptable and are shown in .
Engagement
Engagement was measured using a tripartite approach in which the construct spans cognitive, behavioural, and affective dimensions (Fredricks et al., Citation2004). Three items were used: “I get involved in the things we do in math” (behavioural), “I look forward to continuing with math at school and beyond” (cognitive), and “I enjoy math” (affective). This measure has been previously validated, including in mathematics (Bostwick et al., Citation2017). Measurement invariance between the two timepoints was established using the same procedure as for Motivation, above. Measurement invariance was not rejected in any stage of the CFA, and the final invariance model was used to estimate factor scores for subsequent analysis. Fit indices were acceptable and are shown in .
Achievement
Given that achievement at school generally, or even within a subject domain such as mathematics, can vary considerably between classrooms and schools, we administered a standardised numeracy achievement test in the survey. The test consisted of a 10-item multiple-choice measure, with items increasing in difficulty from the beginning to the end of the test. The test is to the same as that used in similar survey research (Burns et al., Citation2019; Green et al., Citation2012; Martin et al., Citation2011, Citation2012), developed by mathematics educators and mathematics education researchers to be similar to the Wide Range Achievement Test (Wilkinson & Robertson, Citation2016). In previous research, the test exhibits expected correlations with other factors, shows acceptable reliability, and displays the expected pattern of difficulty according to grade level (Burns et al., Citation2019). In the present study, similar differences in performance were observed (i.e., grade 9 < grade 10 < grade 11). A single measure of achievement was calculated by summing the total number of correct responses to the test. As shown in , achievement scores were approximately normally distributed (as per skewness and kurtosis values).
Covariates
The study incorporated measures of sociodemographic covariates that are potentially associated with the outcome variables of interest. Students indicated age, sex, and as a measure of SES, their home postcode, which was then matched to the index used by the Australian Bureau of Statistics. All subsequent analyses presented here included demographics as covariates at level 1 (between-student level).
Data analysis
The multilevel path analysis was the central component of this investigation and conducted to test the hypothesised model (see ). To minimise the number of estimated parameters relative to sample size (especially at level 2), the path model used factor scores generated from the CFAs described in Measures—rather than estimating a full structural equation model using individual items. Missing data were handled using Full Information Maximum Likelihood. Multivariate outliers were identified using the Mahalanobis distance and ten outliers were removed. At level 1 (between-student), motivation, engagement, and achievement were predicted by their pre-test counterparts to control for prior variance (i.e., autoregression), as well as by LRI which is the substantive predictor of primary interest to the present study. Motivation, engagement, and achievement were also predicted by age, gender, and SES. At level 2 (between-classroom), motivation, engagement, and achievement were predicted by their pre-test counterparts (autoregression) as well as by LRI.
Results
As shown in , ICCs indicated sufficient variation between classrooms on each of the measures to support multilevel modelling. Multilevel correlations are shown in and all were in the expected direction and magnitude. These significant correlations provided preliminary support for the hypothesised associations and so we proceeded to multivariate analyses to ascertain the role of LRI in predicting student- and classroom-level gains in motivation, engagement, and achievement.
Table 3. Multilevel correlations.
The multilevel path model yielded acceptable fit, χ2 (12) = 23.791, p = .022; RMSEA = .067, CFI = .972, SRMR (within) = .042, SRMR (between) = .061. All of the hypothesised predictive parameters were empirically supported. At level 1 (student), controlling for pre-test variables (autoregression), LRI predicted gains in motivation (β = .323, 90% CI [.232, .414]), engagement (β = .289 [.199, .379]), and achievement (β = .238 [.130, .345]). None of the covariates at level 1 (age, gender, SES) reached statistical significance. At level 2 (classroom), controlling for pre-test variables, LRI predicted gains in motivation (β = .504 [.278, .729]), engagement (β = .548 [.297, .799]), and achievement (β = .681 [.522, .841]). shows results.
Discussion
The aim of this study was to test the theorised role of LRI in predicting gains in mathematics motivation, engagement, and achievement, controlling for students’ socio-demographic attributes. As hypothesised, findings demonstrated that LRI was associated with gains in mathematics motivation, engagement, and achievement at student- and classroom-levels. It thus appears that LRI is a positive direction for mathematics practitioners’ efforts to optimise student and whole-class motivation, engagement, and achievement.
Findings of note
The focal longitudinal multilevel path analysis showed that LRI predicted gains in motivation, engagement, and achievement at both student- and classroom-levels (i.e., beyond the effects of prior motivation, engagement, and achievement). Indeed, the inclusion of students’ prior motivation, engagement, and achievement is a major extension on the previous measurement work into LRI in mathematics. That measurement work showed significant cross-sectional correlations between LRI and various academic outcomes (Martin & Evans, Citation2018). In controlling for prior motivation, engagement, and achievement, the key contribution of the present study is its extension beyond cross-sectional correlations to understanding of LRI associations that reflect gains (or declines) across time. Moreover, we can also conclude that beyond the role of LRI in individual students’ motivation, engagement, and achievement, there is also a significant role for LRI at the whole-class level. This aligns with multilevel longitudinal findings in the science domain that LRI predicts gains in student- and classroom-level engagement (Martin et al., Citation2021a). Our research is the first to demonstrate this in terms of student- and classroom-level gains in motivation, engagement, and achievement in mathematics.
The present findings also suggest that teachers’ efforts to improve motivation and engagement may not need to undermine their efforts to improve learning. Indeed, the strong, positive effects of LRI suggest that motivation and engagement are substantially increased, potentially because LRI strategies are inherently agentic for the learner (Martin & Evans, Citation2018), or because the reduction of extraneous load may itself reduce the experience of frustration and encourage learners to engage further (F. Kirschner et al., Citation2011). In sum, instructional strategies aimed at appropriately managing the cognitive burden on learners is not at cross-purposes with efforts to motivate and engage them. Nonetheless, recent research indicates that some caution may be necessary in interpreting this finding. Martin et al. (Citation2021a) assessed LRI at both student and classroom levels in the domain of science using latent profile analysis. Although most of the profiles showed a typically adaptive pattern for LRI (i.e., LRI associated with motivation and engagement), some students exhibited higher than average levels of anxiety and the adoption of failure-avoidance goals in the presence of LRI. Taken together, it seems reasonable to conclude that LRI is generally associated with positive motivation and engagement, but longitudinal design, multilevel analysis, and person-centered approaches may all be necessary in future work to expand on these findings. Additionally, a key implication of this for future research is to examine motivation and engagement in a more theoretically nuanced way, which may include studying a range of constructs, and understanding more maladaptive forms of motivation and engagement (e.g., amotivation, external motivation, anxiety, disengagement).
In relation to cognitive load theory more generally, some efforts to investigate the role of motivation in previous research have produced mixed results. Some theoretical proposals have suggested motivation might influence the perception of cognitive load, though findings in relation to this hypothesis are mixed (Eitel et al., Citation2020; Likourezos & Kalyuga, Citation2017; Rey & Buchwald, Citation2011). The present findings are more aligned with a line of research focused on the motivational or affective states that result from the experience of cognitive load (Feldon et al., Citation2019), or in response to strategies that reduce cognitive load (Martin, Citation2016). Feldon (2019), for example, proposed cognitive load as a psychological stressor that would negatively impact motivation, which is supported by a range of experimental findings showing declines in motivation following the experience of extraneous cognitive load, or increases in motivation following attempts to decrease extraneous cognitive load (e.g., Feldon et al., Citation2018; Skulmowski et al., Citation2016; Wang et al., Citation2022). The present findings are generally aligned with this avenue of research and build on them by showing that over the course of a large part of the school year, a substantial proportion of the increases in motivation, engagement, and achievement may be attributable to the degree to which students report teachers using LRI principles.
In relation to major approaches to instruction in the literature, the positive outcomes associated with LRI lend support for approaches characterised by a high level of instructional guidance in the early stages of learning—and also guided independence at the appropriate point in the learning process (Evans & Martin, Citation2023). In its initial phases, LRI is aligned with explicit instruction, an approach characterised by making learning goals explicit, providing rich explanation, allowing students to ask clarifying questions, and leading students through worked examples and similarly structured materials (Liem & Martin, Citation2013). However, whereas explicit instruction is typically contrasted with ‘minimal-guidance’ instructional strategies, such as the use of problem-based learning and inquiry-based learning (Alfieri et al., Citation2011; P. A. Kirschner et al., Citation2006; Mayer, Citation2004), LRI moves beyond this dichotomy by suggesting that when students have attained enough expertise in the topic area, more independent learning strategies are not only desirable, but necessary. The present findings showed that an instructional approach comprising both elements indicated a strong global factor that significantly predicted gains in motivation, engagement, and achievement. These results suggest that in practice, a pragmatic approach to learning, using instructional strategies most suited to students’ prior knowledge, may be most appropriate. Students low in prior knowledge might benefit more from highly structured, scaffolded lessons, while students high in prior knowledge might benefit from more guided independent activities (Martin, Citation2016). At all stages, there is a clear role for the teacher and their provision of guidance and support.
The present findings also align with another strand of salient research on autonomy support and structure. Autonomy support and structure are provided by teachers when they consistently and clearly communicate learning goals, provide instructional help and support, and adjust teaching strategies to suit their students (Ryan & Deci, Citation2017; Vansteenkiste et al., Citation2012). Recently, researchers have found that autonomy support and structure appear to work together to enhance learners’ educational experience and learning (Jang et al., Citation2010; Sierens et al., Citation2009; Vansteenkiste et al., Citation2012). Teaching styles characterised by autonomy support and structure are also associated with motivation, engagement, and achievement (Aelterman et al., Citation2019). Future research, therefore, may examine several possibilities in relation to this research on autonomy support and structure. First, it may be that teachers who tend to be high in autonomy support and structure also tend to use LRI strategies, and knowing this would contribute to understanding how teachers are able to best support their students’ learning. Second, it would be useful to identify the extent to which LRI explains variance in educational outcomes alongside autonomy support and structure—for example, is LRI a way to operationalise structure, or are there characteristics of LRI that provide benefits beyond what has been shown in research on structure? Third, the teacher’s autonomy-supportive style may moderate the extent to which LRI strategies are effective. For instance, a teacher who uses worked examples may not be effective if they do not communicate the structure of the lesson to students and if they administer the lesson in a controlling way.
Limitations and future research
There are limitations in this research that are important to recognise when interpreting findings and which also provide some direction for future research. First, our investigation adopted several measures to overcome some common limitations of correlational research studies, including a longitudinal design to account for prior variance, and multilevel modelling to account for the clustering of students in classrooms. However, the study comprised only two time points separated by six months. Related to this limitation, the design of the study permitted only two measures at approximately 6 months apart. This design gives some insight into the large-scale changes that happen over the course of half of an academic year, but future research may take a closer look at the dynamic nature of the teaching and learning process as it occurs over time (e.g., from week to week, or month to month), and could be complemented by qualitative or observational methods to give a richer insight into the learning process as it occurs over time. For future research, the longitudinal aspect may be expanded by examining three or more time points separated by longer time periods that would allow for latent growth modelling to meaningfully investigate trajectories in academic outcomes as a function of LRI. More broadly, similar claims may be tested by alternative methodologies such as randomised controlled trials, which may be able to make more conclusive claims to causality. This could be done on either a small scale, by varying instruction between groups (characterised by either low or high use of LRI principles); or on a large scale, by conducting professional learning programs for teachers and examining the subsequent effects on motivation, engagement, and achievement of their students over time. The present findings suggest that further work in this direction may be fruitful.
Second, our multilevel modelling was based on 39 classrooms. Although adequate for multilevel analyses, it is a modest number of units at level 2, which can risk bias in the estimates of standard errors (Maas & Hox, Citation2005). Future research may consider collecting data from more classrooms, especially if classroom effects are to be the focus of the research. Third, the study relied on surveys of student self-reports (though we did employ an objective measure of achievement). More ‘objective’ indicators, such as expert observational ratings, might be employed to triangulate the findings. Finally, we note that the study was focused on one subject—mathematics. In other subject areas, LRI strategies may differ in their influences on students’ learning (and motivation and engagement). Although similar LRI findings have been identified in the science domain (Martin et al., Citation2021b), it is important that future research focuses on other subject areas (including in the humanities) as contexts in which to investigate the role of LRI in motivation, engagement, and achievement. Relatedly, ‘achievement’ may be construed in varying ways. Insofar as it refers to students’ developing knowledge in a subject domain, the standardised measure we used in the present study overcomes the limitation of achievement being operationalised and reported in anachronistic ways from school to school, or even from classroom to classroom. Nonetheless, it is a brief measure, and depending on the focus of future research studies, researchers may wish to consider a more expanded standardised test of ability, or other indicators (such as school-reported grades) to model student achievement.
Conclusion
The present study responded to the need for research to better understand the relationships between teaching strategies and gains in educational outcomes of motivation, engagement, and learning in mathematics. Although it is well-established that minimising extraneous cognitive load results in learning that is more effective and efficient (an insight largely derived through experimental designs), comparatively little research has examined learning outcomes alongside motivation and engagement, including in longitudinal multilevel correlational designs. Using the recently articulated framework of LRI (Martin, Citation2016; Martin & Evans, Citation2018), the present study found that teachers’ use of LRI principles was associated with improved learning, motivation, and engagement in mathematics. The findings also shed further light on long-held debates concerning the academic benefits of explicit and constructivist approaches to teaching and learning.
Declaration
One of the measures (the MES) in the study is a published instrument attracting a fee, part of which is put towards its ongoing development and administration, part of which is donated to UNICEF, and part of which is received by the author as a royalty. However, for this study, there was no fee involved for its use.
Additional information
Funding
References
- Aelterman, N., Vansteenkiste, M., Haerens, L., Soenens, B., Fontaine, J. R. J., & Reeve, J. (2019). Toward an integrative and fine-grained insight in motivating and demotivating teaching styles: The merits of a circumplex approach. Journal of Educational Psychology, 111(3), 497–521. https://doi.org/10.1037/edu0000293
- Alfieri, L., Brooks, P. J., Aldrich, N. J., Alfieri, L., Brooks, P. J., & Tenenbaum, H. R. (2011). Does discovery-based instruction enhance learning? Journal of Educational Psychology, 103(1), 1–18. https://doi.org/10.1037/a0021017
- Ayres, P. (2006). Impact of reducing intrinsic cognitive load on learning in a mathematical domain. Applied Cognitive Psychology, 20(3), 287–298. https://doi.org/10.1002/acp.1245
- Baddeley, A. D. (2012). Working memory: Theories, models, and controversies. Annual Review of Psychology, 63(1), 1–29. https://doi.org/10.1146/annurev-psych-120710-100422
- Bostwick, K. C. P., Collie, R. J., Martin, A. J., & Durksen, T. L. (2017). Students’ growth mindsets, goals, and academic outcomes in mathematics. Zeitschrift Für Psychologie, 225(2), 107–116. https://doi.org/10.1027/2151-2604/a000287
- Burns, E. C., Martin, A. J., & Collie, R. J. (2019). Understanding the role of personal best (PB) goal setting in students’ declining engagement: A latent growth model. Journal of Educational Psychology, 111(4), 557–572. https://doi.org/10.1037/edu0000291
- Chandler, P., & Sweller, J. (1992). The split-attention effect as a factor in the design of instruction. British Journal of Educational Psychology, 62(2), 233–246. https://doi.org/10.1111/j.2044-8279.1992.tb01017.x
- Cowan, N. (2010). The magical mystery four: How is working memory capacity limited, and why? Current Directions in Psychological Science, 19(1), 51–57. https://doi.org/10.1177/0963721409359277
- Cowan, N. (2014). Working memory underpins cognitive development, learning, and education. Educational Psychology Review, 26(2), 197–223. https://doi.org/10.1007/s10648-013-9246-y
- Eitel, A., Endres, T., & Renkl, A. (2020). Self-management as a bridge between cognitive load and self-regulated learning: The illustrative case of seductive details. Educational Psychology Review, 32(4), 1073–1087. https://doi.org/10.1007/s10648-020-09559-5
- Evans, P., & Martin, A. J. (2023). Explicit Instruction. In A. O’Donnell, N. C. Barnes, & J. Reeve (Eds.), The Oxford handbook of educational psychology. Oxford University Press. https://doi.org/10.1093/oxfordhb/9780199841332.013.53
- Feldon, D. F., Callan, G., Juth, S., & Jeong, S. (2019). Cognitive load as motivational cost. Educational Psychology Review, 31(2), 319–337. https://doi.org/10.1007/s10648-019-09464-6
- Feldon, D. F., Franco, J., Chao, J., Peugh, J., & Maahs-Fladung, C. (2018). Self-efficacy change associated with a cognitive load-based intervention in an undergraduate biology course. Learning and Instruction, 56, 64–72. https://doi.org/10.1016/j.learninstruc.2018.04.007
- Fenesi, B., Sana, F., Kim, J. A., & Shore, D. I. (2015). Reconceptualizing working memory in educational research. Educational Psychology Review, 27(2), 333–351. https://doi.org/10.1007/s10648-014-9286-y
- Fredricks, J. A., Blumenfeld, P. C., & Paris, A. H. (2004). School engagement: Potential of the concept, state of the evidence. Review of Educational Research, 74(1), 59–109. https://doi.org/10.3102/00346543074001059
- Green, J., Liem, G. A. D., Martin, A. J., Colmar, S., Marsh, H. W., & McInerney, D. (2012). Academic motivation, self‐concept, engagement, and performance in high school: Key processes from a longitudinal perspective. Journal of Adolescence, 35(5), 1111–1122. https://doi.org/10.1016/j.adolescence.2012.02.016
- Hattie, J., & Timperley, H. (2007). The power of feedback. Review of Educational Research, 77(1), 81–112. https://doi.org/10.3102/003465430298487
- Hmelo-Silver, C. E. (2004). Problem-based learning: What and how do students learn? Educational Psychology Review, 16(3), 235–266. https://doi.org/10.1023/B:EDPR.0000034022.16470.f3
- Jang, H., Reeve, J., & Deci, E. L. (2010). Engaging students in learning activities: It is not autonomy support or structure, but autonomy support and structure. Journal of Educational Psychology, 102(3), 588–600. https://doi.org/10.1037/a0019682
- Kalyuga, S. (2007). Expertise reversal effect and its implications for learner-tailored instruction. Educational Psychology Review, 19(4), 509–539. https://doi.org/10.1007/s10648-007-9054-3
- Kirschner, F., Kester, L., & Corbalan, G. (2011). Cognitive load theory and multimedia learning, task characteristics and learning engagement: The Current State of the Art. Computers in Human Behavior, 27(1), 1–4. https://doi.org/10.1016/j.chb.2010.05.003
- Kirschner, P. A., Sweller, J., & Clark, R. E. (2006). Why minimal guidance during instruction does not work: An analysis of the failure of constructivist, discovery, problem-based, experiential, and inquiry-based teaching. Educational Psychologist, 41(2), 75–86. https://doi.org/10.1207/s15326985ep4102_1
- Liem, G. A. D., & Martin, A. J. (2012). The motivation and engagement scale: Theoretical framework, psychometric properties, and applied yields. Australian Psychologist, 47(1), 3–13. https://doi.org/10.1111/j.1742-9544.2011.00049.x
- Liem, G. A. D., & Martin, A. J. (2013). Direct instruction and academic achievement. In J. Hattie & E. M. Anderman (Eds.), International guide to student achievement (pp. 366–369). Routledge.
- Likourezos, V., & Kalyuga, S. (2017). Instruction-first and problem-solving-first approaches: Alternative pathways to learning complex tasks. Instructional Science, 45(2), 195–219. https://doi.org/10.1007/s11251-016-9399-4
- Maas, C. J. M., & Hox, J. J. (2005). Sufficient sample sizes for multilevel modeling. Methodology, 1(3), 86–92. https://doi.org/10.1027/1614-2241.1.3.86
- Martin, A. J. (2007). Examining a multidimensional model of student motivation and engagement using a construct validation approach. The British Journal of Educational Psychology, 77(Pt 2), 413–440. https://doi.org/10.1348/000709906X118036
- Martin, A. J. (2016). Using Load Reduction Instruction to boost motivation and engagement. British Psychological Society.
- Martin, A. J., Anderson, J., Bobis, J., Way, J., & Vellar, R. (2012). Switching on and switching off in mathematics: An ecological study of future intent and disengagement among middle school students. Journal of Educational Psychology, 104(1), 1–18. https://doi.org/10.1037/a0025988
- Martin, A. J., Bobis, J., Anderson, J., Way, J., & Vellar, R. (2011). Patterns of multilevel variance in psycho-educational phenomena: Comparing motivation, engagement, climate, teaching, and achievement factors. Zeitschrift Für Pädagogische Psychologie, 25(1), 49–61. https://doi.org/10.1024/1010-0652/a000029
- Martin, A. J., & Evans, P. (2018). Load reduction instruction: Exploring a framework that assesses explicit instruction through to independent learning. Teaching and Teacher Education, 73, 203–214. https://doi.org/10.1016/j.tate.2018.03.018
- Martin, A. J., & Evans, P. (2019). Load reduction instruction (LRI): Sequencing explicit instruction and guided discovery to enhance students’ motivation, engagement, learning, and achievement. In S. Tindall-Ford, S. Agostinho, & J. Sweller (Eds.), Advances in cognitive load theory (pp. 15–29). Routledge. https://doi.org/10.4324/9780429283895-2
- Martin, A. J., Ginns, P., Burns, E. C., Kennett, R., Munro-Smith, V., Collie, R. J., & Pearson, J. (2021a). Assessing instructional cognitive load in the context of students’ psychological challenge and threat orientations: A multi-level latent profile analysis of students and classrooms. Frontiers in Psychology, 12, 656994. https://doi.org/10.3389/fpsyg.2021.656994
- Martin, A. J., Ginns, P., Burns, E. C., Kennett, R., & Pearson, J. (2021b). Load reduction instruction in science and students’ science engagement and science achievement. Journal of Educational Psychology, 113(6), 1126–1142. https://doi.org/10.1037/edu0000552
- Martin, A. J., Ginns, P., Nagy, R. P., Collie, R. J., & Bostwick, K. C. P. (2023). Load reduction instruction in mathematics and English classrooms: A multilevel study of student and teacher reports. Contemporary Educational Psychology, 72, 102147. https://doi.org/10.1016/j.cedpsych.2023.102147
- Martin, A. J., Yu, K., Papworth, B., Ginns, P., & Collie, R. J. (2015). Motivation and engagement in the United States, Canada, United Kingdom, Australia, and China: Testing a multi-dimensional framework. Journal of Psychoeducational Assessment, 33(2), 103–114. https://doi.org/10.1177/0734282914546287
- Mayer, R. E. (2004). Should there be a three-strikes rule against pure discovery learning? The case for guided methods of instruction. The American Psychologist, 59(1), 14–19. https://doi.org/10.1037/0003-066X.59.1.14
- Puma, S., Matton, N., Paubel, P.-V., & Tricot, A. (2018). Cognitive load theory and time considerations: Using the time-based resource sharing model. Educational Psychology Review, 30(3), 1199–1214. https://doi.org/10.1007/s10648-018-9438-6
- Rey, G. D., & Buchwald, F. (2011). The expertise reversal effect: Cognitive load and motivational explanations. Journal of Experimental Psychology. Applied, 17(1), 33–48. https://doi.org/10.1037/a0022243
- Ryan, R. M., & Deci, E. L. (2017). Self-determination theory. Guilford Press.
- Sierens, E., Vansteenkiste, M., Goossens, L., Soenens, B., & Dochy, F. (2009). The synergistic relationship of perceived autonomy support and structure in the prediction of self-regulated learning. The British Journal of Educational Psychology, 79(Pt 1), 57–68. https://doi.org/10.1348/000709908X304398
- Skulmowski, A., Pradel, S., Kühnert, T., Brunnett, G., & Rey, G. D. (2016). Embodied learning using a tangible user interface: The effects of haptic perception and selective pointing on a spatial learning task. Computers & Education, 92-93, 64–75. https://doi.org/10.1016/j.compedu.2015.10.011
- Sweller, J. (2006). The worked example effect and human cognition. Learning and Instruction, 16(2), 165–169. https://doi.org/10.1016/j.learninstruc.2006.02.005
- Sweller, J. (2011). Cognitive load theory. In J. P. Mestre & B. H. Ross (Eds.), The psychology of learning and motivation (Vol. 55, pp. 37–76). Academic Press. https://doi.org/10.1016/B978-0-12-387691-1.00002-8
- Sweller, J., Ayres, P., & Kalyuga, S. (2011). Cognitive load theory. Springer. https://doi.org/10.1007/978-1-4419-8126-4
- Sweller, J., Ayres, P., Kalyuga, S., & Chandler, P. (2003). The expertise reversal effect. Educational Psychologist, 38(1), 23–31. https://doi.org/10.1207/S15326985EP3801_4
- Tobias, S. (2009). An eclectic appraisal of the success or failure of constructivist instruction. In S. Tobias & T. M. Duffy (Eds.), Constructivist instruction: Success or failure? (pp. 335–350). Routledge.
- Tricot, A., & Sweller, J. (2014). Domain-specific knowledge and why teaching generic skills does not work. Educational Psychology Review, 26(2), 265–283. https://doi.org/10.1007/s10648-013-9243-1
- Vansteenkiste, M., Sierens, E., Goossens, L., Soenens, B., Dochy, F., Mouratidis, A., Aelterman, N., Haerens, L., & Beyers, W. (2012). Identifying configurations of perceived teacher autonomy support and structure: Associations with self-regulated learning, motivation and problem behavior. Learning and Instruction, 22(6), 431–439. https://doi.org/10.1016/j.learninstruc.2012.04.002
- Wang, B., Ginns, P., & Mockler, N. (2022). Sequencing tracing with imagination. Educational Psychology Review, 34(1), 421–449. https://doi.org/10.1007/s10648-021-09625-6
- Wilkinson, G. S., & Robertson, G. J. (2016). Wide range achievement test 4. [Data set]. American Psychological Association. https://doi.org/10.1037/t27160-000