Abstract
This study aimed to elucidate the differences in vulval squamous cell carcinomas (VSCC) based on the HPV infection status. The sequencing data GSE183454 which contains 23 VSCC samples based on its HPV infection status was obtained from the Gene Expression Omnibus (GEO) database. We comprehensively dissected the differences of genomic and tumour microenvironment (TME) immune cell infiltration landscapes between HPV + and HPV- VSCC. The potential molecular mechanisms of prognostic genes were explored by functional enrichment analysis. Five novel key molecules (SYCP2, SMC1B, RNF212, MAJIN and C14orf39) with significantly up-regulated expression in HPV + VSCC were identified while protein-protein interaction (PPI) networks were created upon Cytoscape software. Additionally, VSCC with up-regulated expression of these key molecules exhibited a significantly decreased TME immune cell infiltration. SYCP2 is overexpressed in HPV + VSCC and could be a candidate therapy target for further research.
What is already known on this subject? VSCC are characterised by two aetiological pathways. The former occurs in the background of lichen sclerosus, while the latter is related to HPV infection. VSCC most commonly arises from the non-HPV related pathway portends worse prognosis than VSCC derived from HPV infection.
What do the results of this study add? Five key molecules are identified and significantly up-regulated in HPV + VSCC. In which, SYCP2 is overexpressed in HPV + VSCC and exhibited a significantly decreased TME immune cell infiltration. SYCP2 constant expression could be a potential biomarker of neoplasms associated with HPV and could be a candidate therapy target in VSCC especially HPV + VSCC for further research.
What are the implications of these findings for clinical practice and/or further research? SYCP2 could be a candidate therapy target in VSCC especially with HPV + for further research.
IMPACT STATEMENT
Introduction
Vulvar cancer is an uncommon gynaecological tumour which accounts for 3% to 5% of all malignancies in female genital cancer (Weinberg and Gomez-Martinez Citation2019). Approximately 90% of vulvar cancer are vulval squamous cell carcinomas (VSCC) with an increasing incidence by an average of 0.6% per year for the past decade (Weinberg and Gomez-Martinez Citation2019). VSCC, which always develops from the skin of the labia or the vulvar mucosa, is divided into two types according to the pathogenesis with completely disparate epidemiological, clinical and molecular characteristics (Del Pino et al. Citation2013, Preti et al. Citation2020). One type arises primarily in younger patients and is associated with human papillomavirus (HPV) persistent infection while its prevalence continues to increase every year (American Cancer Society Citation2022), another form is observed mostly in elderly patients and appears to develop independently of HPV infection (Del Pino et al. Citation2013). Although, the prevalence of HPV-positive tumours is seen to be decreasing as a result of HPV vaccination (Cheng et al. Citation2020).
It has been reported that HPV-associated carcinoma accounts for 40% of all VSCC and is considered to be the cause of the recent increase worldwide. Both Low-Grade Squamous Intraepithelial Lesion (LSIL) and High-Grade Squamous Intraepithelial Lesion (HSIL) are precancerous lesions of VSCC and could be caused by high-risk HPV, which is also called oncogenic HPV based on its extreme carcinogenic capability. Among this, HPV16 is related with more than 77% cases of HSIL, whereas HPV 33 and 18 are related with 11% and 2.6% cases of cases, respectively (Leonard et al. Citation2014). As previously mentioned, the preneoplastic of another HPV independent type of VSCC is differentiated vulval intraepithelial neoplasia (VIN) which occurs frequently in elderly females with inflammatory dermatosis, mostly lichen scleroses. Furthermore, it is also reported that the aberrant of Interferon regulatory factor-6 (IRF6) promoter methylation promote the occurrence of lesions from vulva normal skin to vulvar lichen sclerosus, VIN and VSCC (Rotondo et al. Citation2016). Meanwhile, smoking, multiple sexual partners, young age of first intercourse and immunosuppression are additional risk factors for VSCC in general (Reyes and Cooper Citation2014, American Cancer Society Citation2022).
The aetiology-based classification appears to develop clinically significant with HPV-associated carcinomas benefit more from overall outcome compared to HPV independent tumours (Kokka et al. Citation2011, Nooij et al. Citation2017). This seemingly paradoxical phenomenon could be explained by the pathogenesis of HPV-associated HSIL and HPV independent VIN. Histologically, VIN which occurs in a field transform to lichen scleroses, could frequently be seen adjacent to squamous cell carcinoma, it is associated more often with synchronous or metachronous VSCC. Under this environmental factor, VSCC occurring in a VIN background tends to recur than HSIL background (Eva et al. Citation2008, Jin and Liang Citation2019). Coincidentally, increasing evidence has been found that head and neck squamous cell carcinomas (HNSCC) associated with HPV has a better prognosis, especially in oropharynx squamous cancers (OPSCC) (Mahal et al. Citation2019). Interestingly, Moshiri (Moshiri et al. Citation2017) has confirmed that Polyomavirus-negative Merkel Cell Carcinoma may be more aggressive and more likely to recur after treatment, with virus-negative Merkel Cell Carcinoma having been shown to have higher chromosomal aberrations, greater oncogenic pathways mutations and higher nucleotide mutation burden. The profile of molecules and genes may be altered after HPV infection in VSCC while prognosis could also be influenced. It is reasonable to speculate that the potential molecules or genes involved in VSCC based on HPV infection status could be analysed for further treatment exploration.
Advancements in biotechnology improved availability of high-throughput data which supports in-depth scientific research with effective early diagnosis, prognosis prediction and investigations of molecular mechanisms for numerous types of disease. Tumour Microenvironment (TME) characterisation plays a vital role in mediating advanced metastasis, immune escape and immunotherapeutic resistance. However, the overall TME immune cell infiltration characterisations mediated by multiple key molecules in VSCC remain little known. This present study used GSE183454 sequencing data downloaded from the Gene Expression Omnibus (GEO) to determine the Differentially Expressed Genes (DEGs) of VSCC based on HPV infection status. Subsequently, functional enrichment analyses and TME immune cell infiltration characterisation were performed to identify the significant biological terms associated with the DEGs. The current study could provide a new perspective for elucidating the biological significance of VSCC based on HPV infection status, and assist with the identification of potential candidate biomarkers for diagnosis, prognosis and therapy.
Materials and methods
Gene expression profiles of vulvar cancer by HPV status
The gene expression profile GSE183454 which detected by high throughput sequencing was downloaded from the Gene Expression Omnibus (GEO) database (https://www.ncbi.nlm.nih.gov/geo). GSE183454 is a dataset submitted by Wang et al (Kolitz et al. Citation2022), containing 23 samples which were retrieved from paraffin embedded invasive vulvar squamous cell carcinoma tissues. HPV status of 23 vulvar cancer samples were determined by a consensus of high-risk HPV RNA in situ hybridisation (ISH) and HPV nested PCR. Only samples that were positive in both assays were considered ‘HPV+’, otherwise were considered ‘HPV-‘.
Identification of DEGs
The DESeq2, edgeR and limma packages were applied to perform data standardisation for each gene expression profiles. DEGs were filtered by p < 0.05 and |log fold‑change| >2 in DESeq2, edgeR and limma packages. The distribution of the DEGs analysed by the three packages was presented as a Venn diagram using R (Bhattacharya et al. Citation2018).
GO and pathway enrichment analysis of hub genes
To explore potential biological processes related to the obtained DEGs, we performed Gene Ontology (GO) and Kyoto Encyclopaedia of Gene and Genomes (KEGG) enrichment analysis using the clusterProfiler R package (Yu et al. Citation2012). The GO enrichment analysis was conducted based on three aspects including Biological Process (BP), Cellular Components (CC) and Molecular Functions (MF). Both false discovery rates (FDR) were set at <0.05 to conduct the GO and KEGG analysis of the DEGs. The activated or inactivated biological pathways among patients with low risk score and high risk score by running the gene set enrichment analysis (GSEA) of the adjusted expression data for all transcripts (Subramanian et al. Citation2005).
Pathway crosstalk and gene-pathway network analysis to identify hub gens and construct PPI network
To further investigate the development mechanisms of Vulvar cancer, the hub genes were mapped into a crosstalk network. The gene-pathway network was constructed and visualised in Cytoscape version 3.9.1 (https://cytoscape.org/) which original from online STRING database (https://string-db.org/) that had been designed to identify PPI pairs (Szklarczyk et al. Citation2017) and construct Protein-protein interaction (PPI) networks from large functional groups of proteins. MCODE, a plugin in the Cytoscape software, was adopted to calculate the degree of each node and select modules of hub genes from the network. PPI network was generated by R to identify the hub genes from DEGs.
Acquisition of immunotherapeutic cohorts and collection of clinical information
The IMvigor210 immunotherapeutic cohorts (Mariathasan et al. Citation2018) with transcriptome data and complete clinical information were included in this study. The IMvigor210 cohort investigated the efficacy of pembrolizumab in advanced urothelial cancer and could be applied for immunotherapy verification. The complete transcriptome data and detailed clinical information were downloaded from the website (http://research-pub.Gene.com/imvigor210corebiologies). The DEseq2 R package was used to perform normalisation. We then transformed the count value into the transcripts per kilobase million (TPM) value.
To control the bias resulted by the tumour purity, we adjusted the enrichment scores of each TME cell subtype by calculating the tumour purity using ESTIMATE algorithm (Yoshihara et al. Citation2013). The adjusted enrichment scores calculated by single sample gene set enrichment analysis (ssGSEA) were used to represent the abundance of each TME immune infiltration cell (Barbie et al. Citation2009). In total, 28 human TME immune cell subtypes were evaluated including memory B cell, Type17 T helper cell, Activated CD4 T cell, Type2 T helper cell, CD56dim natural killer cell, Plasmacytoid dendritic cell, Activated CD8 T cell, Monocyte, Activated dendritic cell, Activated B cell, Natural killer cell, Eosinophil, Natural killer T cell, Type1 T helper cell, Immature B cell, Myeloid-derived suppressor cell (MDSC), Gamma delta T cell, CD56 bright natural killer cell, Effector memory CD8 T cell, Central memory CD4 T cell, Macrophage, Immature dendritic cell, Neutrophil, Effector memory CD4 T cell, Mast cell, T follicular helper cell, Central memory CD8 T cell, and Regulatory T cell.
Statistical analysis
The difference significance test for two groups was performed using t-test while three or more groups using One-way ANOVA test. Wilcoxon test, Spearman and distance correlation analyses were used to conduct the difference analyses between two groups (Hazra and Gogtay Citation2016). All statistical p values were two-side, with p < 0.05 considered as statistically significant (*p < 0.05, **p < 0.01, ***p < 0.001). The R.4.1.1 software was applied for all of the data processing.
Results
Difference of genomic landscape between HPV negative and positive vulvar cancer tissues
Based on the HPV status, 23 vulvar cancer samples were divided into HPV negative and HPV positive groups with 11 samples and 12 samples, respectively. Gene expression profile of GSE183454 was analysed by DESeq2, edgeR and limma packages in R.
Following screening with the criteria of p < 0.05 and |fold-change| >2, as shown in Supplementary Figure 1. A total of 301 DEGs were identified by DESeq2 in which 228 up-regulated DEGs and 73 down-regulated DEGs in HPV positive vulvar cancer. A total of 316 DEGs were identified by edgeR in which 240 up-regulated DEGs and 76 down-regulated DEGs in HPV positive vulvar cancer. A total of 107 DEGs were identified by limma in which 74 up-regulated DEGs and 33 down-regulated DEGs in HPV positive vulvar cancer. As shown in , all the DEGs were presented in Venn diagram, 40, 51 and 25 up-regulated DEGs were exclusively identified by DESeq2, edgeR and limma, respectively. Eighteen, 21 and 16 down-regulated DEGs were exclusively identified by DESeq2, edgeR and limma analysis, respectively. Finally, intersection of DESeq2, edgeR and limma analysis by Venn diagram found 46 up-regulated and 17 down-regulated DEGs in HPV positive vulvar cancer. They have been shown as Principal Component Analysis (PCA) and Heatmap in .
Figure 1. Intersection of DESeq2, edgeR and limma were shown as PCA (A) and Heatmap (B), Venn diagram showed the distribution of the DEGs analysed by the three packages in which 46 up-regulated (C) and 17 down-regulated (D).
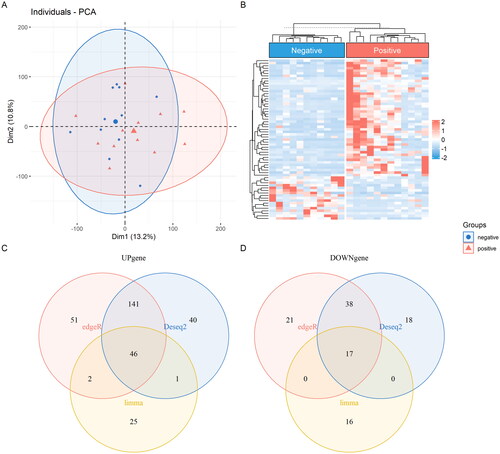
GO and pathway enrichment analysis of hub genes
A total of 63 intersection DEGs were used to perform GO enrichment analysis. As shown in , in the biological process category, DEGs were associated with ‘homologous chromosome pairing at meiosis’, ‘homologous chromosome segregation’, ‘chromosome organisation involved in meiotic cell cycle’, ‘meiotic chromosome segregation’. For cellular component terms (CC), DEGs participate in ‘synaptonemal complex’, ‘synaptonemal structure’, ‘condensed nuclear chromosome’ and ‘lateral element’. No DEGs were found related to molecular function (MF). Top5 DEGs were found in HPV positive vulvar cancer, as shown in Supplementary Figure 2 C, SYCP2 (Synaptonemal Complex Protein 2, OMIM: 604105), SMC1B (Structural Maintenance of Chromosomes 1 B, OMIM: 608685), RNF212 (Ring Finger Protein 212, OMIM: 612041) and MAJIN (Membrane-Anchored Junction Protein, OMIM: 617130) were up-regulated while C14orf39 (Chromosome 14 open reading frame 39, OMIM: 617307) was down-regulated in HPV positive vulvar cancer. Interestingly, it should be further discussed that SYCP2 was associated with all the pathways described above.
Figure 2. Sub-network module of key molecules (A), Protein correlation between key molecules using spearman analyses. Negative correlation was marked with blue and positive correlation with red (B). Top5 signalling pathways involved in the enriched biological process analysed by GSEA (C and D).
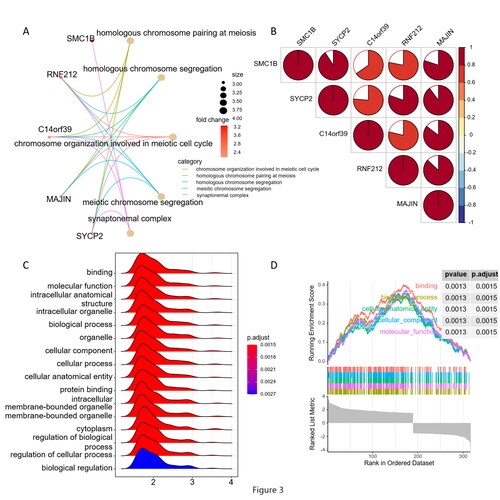
KEGG analysis revealed that these DEGs mainly participated in the signalling pathways regulating pluripotency of stem cells. As shown in Supplementary Figure 2 D, these pathways, which should be confirmed by further studies, have a definite influence on the occurrence and development of VSCC.
Sub-network of key molecules and GSEA
We determined a sub-network module with significant prognostic values, of these, with high degree of connectivity were selected as key molecules. As shown in , SYCP2, SMC1B, RNF212, MAJIN and C14orf39 were selected and significantly related with synaptonemal complex, homologous chromosome pairing at meiosis, homologous chromosome segregation, meiotic chromosome segregation and chromosome organisation involved in meiotic cell cycle, respectively. We also investigated the protein correlation between these key molecules, as shown in , SYCP2, SMC1B, RNF212, MAJIN and C14orf39 were all significantly related with each other.
The top 5 signalling pathways involved in the enriched biological process analysed by GSEA are shown in . These demonstrated that genes related with, such as binding, molecular function, intracellular anatomical structure, intracellular organelle, biological process were enriched by HPV infection in VSCC
PPI network analysis
According to the PPI pairs in the STRING database, an interaction network of the protein encoded by the DEGs was constructed. A centrality analysis of the nodes in the PPI network revealed that SYCP2, SMC1B, RNF212, MAJIN, C14orf39 and C11orf85 were crucial genes (). Among these genes, SYCP2, SMC1B, RNF212, MAJIN and C14orf39 were upregulated while C11orf85 was downregulated by HPV infection in VSCC. The dysregulation of these genes, shown in , may be closely related to HPV infection in VSCC. PCA algorithm of genes () was applied to reduce dimensionality in two groups found two completely disjoint populations, it could be easy to distinguish the significant genes between HPV negative and positive VSCC. As shown in , among the genes altered, SYCP2 exhibited significant degrees of correspondence by HPV infection in VSCC. Overexpression of SYCP2 has been reported to be a potential biomarker of neoplasms associated with HPV (Mendez-Matias et al. Citation2021).
Figure 3. Centrality analysis of the nodes in the PPI network (A). Upregulated genes shown in volcano plot (B). Principal component analysis for the key molecules revealed two completely disjoint populations, suggesting these key molecules could well distinguish in HPV negative and positive VSCC (C). Validation of the expression of key molecules in HPV negative and positive VSCC (D).

Evaluation of TME immune cell infiltration characterisation by ESTIMATE
In order to explore the roles of identified key molecules in TME immune cell infiltration, we evaluate the landscape of 28 TME immune cell infiltration in HPV + and HPV- samples. As shown in Supplementary Figure 3 A, 28 TME immune cells were presented between HPV + and HPV- samples, of these, only macrophage was significantly enriched in HPV-negative tissues, while there were no significant differences between two groups of other cell subtypes.
In order to determine whether there existed the differences of TME immune cell infiltration patterns between HPV + and HPV- samples, we used PCA algorithm to reduce dimensionality for 28 TME immune infiltrating cells (Supplementary Figure 3B). No significant difference was seen between the two TME immune cell populations, suggesting that no significant change of TME immune cells in HPV + and HPV- groups. We used ESTIMATE algorithm to evaluate the immune and stromal activity in the microenvironment. As shown in Supplementary Figure 3 C and D, it was found that there were no differences between HPV + and HPV- groups in the immune and stromal activity.
The relationship of key molecules expression and immune checkpoint molecules were detected and is shown in . No significant differences were found between key molecules expression and immune checkpoint molecules while immune checkpoint molecules were significant with correspondences, such as PD-1, LAG3, PDCD1(PD-L1), CTLA4 and PD-L2. In order to explore the relationships between key molecules and TME immune infiltrating cells, we correlated key molecules with TME immune infiltrating cells (Supplementary Figure 3 F). Spearman correlation analyses revealed negative correlation between RIBC2, SMC1B, SYCP2 and TME immune infiltrating cells, including Gamma delta T cell, Immature dendritic cell, T follicular helper cell, Central memory CD8 T cell and Regulatory T cell. However, we noted that C14orf39 exhibited a positive correlation with Memory B cell.
Discussion
It has been reported that VSCC most commonly arises from the non-HPV related pathway portends worse prognosis than VSCC derived from HPV (Allbritton Citation2017). Whereas, others suggest that HPV status does not impact survival outcomes (Zięba et al. Citation2018). It was speculated that variations in the assays utilised for HPV detection may contribute to these differences (Del Pino et al. Citation2013). Nested DNA PCR and RNA ISH were applied to determine the status of VSCC in GSE183454. Moreover, RNA-seq confirmed that HPV transcripts could be detected in all consensus HPV samples (Kolitz et al. Citation2022). Development of next generation sequencing has provided opportunities to systemically decipher key genetic changes in tumour and other diseases. Computational methods were also developed to estimate the abundance of TME immune infiltration cells based on the immunogenomic profiling included in the RNA sequencing of tumour tissue.
In the present study, we comprehensively analysed the RNA sequencing data of VSCC in GSE183454 which downloaded from GEO. Difference of genomic landscape between HPV negative and positive vulvar cancer tissues were found by DESeq2, edgeR and limma analysis. Intersection DEGs of 46 up-regulated and 17 down-regulated in HPV positive vulvar cancer were identified by Venn diagram. Furthermore, key molecules with significant prognostic values were selected as sub-network module with high degree of connectivity. Consequently, based on the PPI network, we found that SYCP2, SMC1B, RNF212, MAJIN and C14orf39 were upregulated by HPV infection in VSCC.
The influence of microenvironment cell infiltration patterns by the genomic variation of VSCC after HPV infection was also analysed in ESTIMATE algorithm. Only macrophage was significantly enriched in HPV-negative VSCC. Recent studies found that cell components of microenvironment play a crucial role in tumour progression. The tumour part was composed of not only tumour cells but also stromal cells such as macrophage (Bindea et al. Citation2013). Increased evidence demonstrated single key molecule could induce immune tolerance by changing microenvironment immune cell infiltration characterisations, and avoid immune attack by reshaping the microenvironment structures (Zhou et al. Citation2019). These five key molecules mentioned above were investigated with immune checkpoint expression and the infiltration levels of TME immune cells in VSCC. Unfortunately, we noted that there were no significant relationships between five key molecules and the expression of immune checkpoint molecules. However, significant negative correlations between expression of key molecules and TME immune cell infiltrations were found. Gamma delta T cell was unique T cell subpopulation which is enriched in peripheral tissues including skin and makes key contributions to immune response (Ribot et al. Citation2021). Regulatory T cell which prevents autoimmune reactions by suppressing the activation and function of conventional T cells (Savage et al. Citation2020). Both were found negative related with SYCP2. However, central memory CD8+ T cell, which depends on its stem-cell-like capacity to expand, differentiate and self-renew (Pais Ferreira et al. Citation2020), was also found negative related with SYCP2. It is necessary to further investigate the role of key molecules in TME immune cell infiltrations.
A number of key molecules in the present study have been previously implicated in malignant tumours. As shown in , SYCP2 had the highest mutation frequency, which has been reported overexpressed in HPV + HNSCC (Masterson et al. Citation2015, Costa et al. Citation2018). SYCP2 directly interacts with heterochromatin protein 1α (HP1α) and has been associated with HPV infection in almost all of the investigations in cancer (Hosoya and Miyagawa Citation2021). Galo (Mendez-Matias et al. Citation2021) found that SYCP2 constant expression could be a potential biomarker of neoplasms associated with HPV and higher SYCP2 expression predict better survival in HNSCC. Meanwhile, survival plots of SYCP2 in HNSCC (Supplementary Figure 4) on GEPIA website (Tang et al. Citation2017) found that higher SYCP2 expression predicts better prognostic than lower SYCP2 expression in HNSCC although there were no significant differences between two groups. Based on the analysis in the present study, SYCP2 was also overexpressed in HPV + VSCC and related with infiltration of TME immune cell mentioned above. It could be a candidate therapy target in VSCC especially HPV + VSCC for further research.
Nonetheless, there were some limitations which should be considered in this study. Firstly, external datasets are still needed to verify the prognostic values of SYCP2 and other key molecules with HPV infection status in VSCC. Secondly, the role and underlying molecular mechanism of SYCP2 in HPV + VSCC needs to be further explored in following studies. Thirdly, functional validation should be confirmed and the very reduced sample size is also limited in this study. Furthermore, spearman correlation analyses revealed negative correlation between SYCP2 and TME immune infiltrating cells which should be further verified.
Conclusion
This present study distinguishes the gene expression pattern in VSCC based on HPV infection status and proposes that the function of SYCP2 could be further explored in VSCC. These findings provide promising clues to predict the clinical outcomes and potential mechanism in VSCC based on HPV infection status.
Ethical approval
Patients involved in the GEO which belongs to public databases have obtained ethical approval. Users can download relevant data for free for research and publishing relevant articles. Our study is based on opensource data and was approved by the Ethics Committee of Shaanxi Provincial Tumour Hospital.
Author contributions
Ruxing Xi and Yan Wang conceived the study, analysed the data and reviewed drafts of the paper. Donghong Li and Guoqing Wang designed the experiments and authored drafts of the paper. Shuanque Yang, Hui Zhang, Lijuan Hu and Xiaowei Wang analysed the data and prepared figures. The final draft was approved by all the authors in this study.
Supplemental Material
Download Zip (2.5 MB)Disclosure statement
No potential conflict of interest was reported by the author(s).
Additional information
Funding
References
- Allbritton, J.I., 2017. Vulvar neoplasms, benign and malignant. Obstetrics and Gynecology Clinics of North America, 44 (3), 339–352.
- American Cancer Society 2022., Key statictics for vulvar cancer [online]. https://www.cancer.org/cancer/vulvar-cancer/about/key-statistics.html#:%7E:text=In%20the%20United%20States%2C%20women,will%20die%20of%20this%20cancer
- Barbie, D.A., et al., 2009. Systematic RNA interference reveals that oncogenic KRAS-driven cancers require TBK1. Nature, 462 (7269), 108–112.
- Bhattacharya, S., et al., 2018. ImmPort, toward repurposing of open access immunological assay data for translational and clinical research. Scientific Data, 5, 180015.
- Bindea, G., et al., 2013. Spatiotemporal dynamics of intratumoral immune cells reveal the immune landscape in human cancer. Immunity, 39 (4), 782–795.
- Cheng, L., Wang, Y. and Du, J., 2020. Human Papillomavirus Vaccines: An Updated Review. Vaccines ( Vaccines), 8 (3), 391.
- Costa, R.L., Boroni, M. and Soares, M.A., 2018. Distinct co-expression networks using multi-omic data reveal novel interventional targets in HPV-positive and negative head-and-neck squamous cell cancer. Scientific Reports, 8 (1), 15254.
- Del Pino, M., Rodriguez-Carunchio, L. and Ordi, J., 2013. Pathways of vulvar intraepithelial neoplasia and squamous cell carcinoma. Histopathology, 62 (1), 161–175.
- Eva, L.J., et al., 2008. Vulval squamous cell carcinoma occurring on a background of differentiated vulval intraepithelial neoplasia is more likely to recur: a review of 154 cases. The Journal of Reproductive Medicine, 53, 397–401.
- Hazra, A. and Gogtay, N., 2016. Biostatistics Series Module 3: Comparing Groups: Numerical Variables. Indian Journal of Dermatology, 61 (3), 251–260.
- Hosoya, N. and Miyagawa, K., 2021. Synaptonemal complex proteins modulate the level of genome integrity in cancers. Cancer Science, 112 (3), 989–996.
- Jin, C. and Liang, S., 2019. Differentiated Vulvar Intraepithelial Neoplasia: A Brief Review of Clinicopathologic Features. Archives of Pathology & Laboratory Medicine, 143 (6), 768–771.
- Kokka, F., et al., 2011. Is differentiated vulval intraepithelial neoplasia the precursor lesion of human papillomavirus-negative vulval squamous cell carcinoma? International Journal of Gynecological Cancer, 21 (7), 1297–1305.
- Kolitz, E., et al., 2022. Human PapillomavirusPositive and Negative Vulvar Squamous Cell Carcinoma Are Biologically but Not Clinically Distinct. The Journal of Investigative Dermatology, 142 (5), 1280–1290, e7.
- Leonard, B., et al., 2014. A clinical and pathological overview of vulvar condyloma acuminatum, intraepithelial neoplasia, and squamous cell carcinoma. BioMed Research International, 2014, 480573.
- Mahal, B.A., et al., 2019. Incidence and Demographic Burden of HPV-Associated Oropharyngeal Head and Neck Cancers in the United States. Cancer Epidemiology, Biomarkers & Prevention, 28 (10), 1660–1667.
- Mariathasan, S., et al., 2018. TGFbeta attenuates tumour response to PD-L1 blockade by contributing to exclusion of T cells. Nature, 554 (7693), 544–548.
- Masterson, L., et al., 2015. Deregulation of SYCP2 predicts early stage human papillomavirus-positive oropharyngeal carcinoma: A prospective whole transcriptome analysis. Cancer Science, 106 (11), 1568–1575.
- Mendez-Matias, G., et al., 2021. Prevalence of HPV in Mexican Patients with Head and Neck Squamous Carcinoma and Identification of Potential Prognostic Biomarkers. Cancers ( Cancers, 13 (22), 5602. ),
- Moshiri, A.S., et al., 2017. Polyomavirus-Negative Merkel Cell Carcinoma: A More Aggressive Subtype Based on Analysis of 282 Cases Using Multimodal Tumor Virus Detection. The Journal of Investigative Dermatology, 137 (4), 819–827.
- Nooij, L.S., et al., 2017. Genomic Characterization of Vulvar (Pre)cancers Identifies Distinct Molecular Subtypes with Prognostic Significance. Clinical Cancer Research : An Official Journal of the American Association for Cancer Research, 23 (22), 6781–6789.
- Pais Ferreira, D., et al., 2020. Central memory CD8(+) T cells derive from stem-like Tcf7(hi) effector cells in the absence of cytotoxic differentiation. Immunity, 53 (5), 985–1000, e11.
- Preti, M., et al., 2020. Role of human papillomavirus infection in the etiology of vulvar cancer in Italian women. Infectious Agents and Cancer, 15, 20.
- Reyes, M.C. and Cooper, K., 2014. An update on vulvar intraepithelial neoplasia: terminology and a practical approach to diagnosis. Journal of Clinical Pathology, 67 (4), 290–294.
- Ribot, J.C., Lopes, N. and Silva-Santos, B., 2021. gammadelta T cells in tissue physiology and surveillance. Nature Reviews. Immunology, 21 (4), 221–232.
- Rotondo, J.C., et al., 2016. Hypermethylation-Induced Inactivation of the IRF6 Gene as a Possible Early Event in Progression of Vulvar Squamous Cell Carcinoma Associated With Lichen Sclerosus. JAMA Dermatology, 152 (8), 928–933.
- Savage, P.A., Klawon, D.E.J. and Miller, C.H., 2020. Regulatory T Cell Development. Annual Review of Immunology, 38, 421–453.
- Subramanian, A., et al., 2005. Gene set enrichment analysis: a knowledge-based approach for interpreting genome-wide expression profiles. Proceedings of the National Academy of Sciences of the United States of America, 102 (43), 15545–15550.
- Szklarczyk, D., et al., 2017. The STRING database in 2017: quality-controlled protein-protein association networks, made broadly accessible. Nucleic Acids Research, 45 (D1), D362–D368.
- Tang, Z., et al., 2017. GEPIA: a web server for cancer and normal gene expression profiling and interactive analyses. Nucleic Acids Research, 45 (W1), W98–W102.
- Weinberg, D. and Gomez-Martinez, R.A., 2019. Vulvar Cancer. Obstetrics and Gynecology Clinics of North America, 46 (1), 125–135.
- Yoshihara, K., et al., 2013. Inferring tumour purity and stromal and immune cell admixture from expression data. Nature Communications, 4, 2612.
- Yu, G., et al., 2012. clusterProfiler: an R package for comparing biological themes among gene clusters. Omics, 16 (5), 284–287.
- Zhou, L., et al., 2019. Promotion of tumor-associated macrophages infiltration by elevated neddylation pathway via NF-kappaB-CCL2 signaling in lung cancer. Oncogene, 38 (29), 5792–5804.
- Zięba, S., et al., 2018. Somatic mutation profiling of vulvar cancer: Exploring therapeutic targets. Gynecologic Oncology, 150 (3), 552–561.