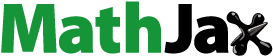
Abstract
Background
Gestational diabetes mellitus (GDM) is a prevalent pregnancy complication during pregnancy. We aimed to evaluate a risk prediction model of GDM based on traditional and genetic factors.
Methods
A total of 2744 eligible pregnant women were included. Face-to-face questionnaire surveys were conducted to gather general data. Serum test results were collected from the laboratory information system. Independent risk factors for GDM were identified using univariate and multivariate logistic regression analyses. A GDM risk prediction model was constructed and evaluated with the Hosmer–Lemeshow goodness-of-fit test, goodness-of-fit calibration plot, receiver operating characteristic curve and area under the curve.
Results
Among traditional factors, age ≥30 years, family history, GDM history, impaired glucose tolerance history, systolic blood pressure ≥116.22 mmHg, diastolic blood pressure ≥74.52 mmHg, fasting plasma glucose ≥5.0 mmol/L, 1-hour postprandial blood glucose ≥8.8 mmol/L, 2-h postprandial blood glucose ≥7.9 mmol/L, total cholesterol ≥4.50 mmol/L, low-density lipoprotein ≥2.09 mmol/L and insulin ≥11.5 mIU/L were independent risk factors for GDM. Among genetic factors, 11 single nucleotide polymorphisms (SNPs) (rs2779116, rs5215, rs11605924, rs7072268, rs7172432, rs10811661, rs2191349, rs10830963, rs174550, rs13266634 and rs11071657) were identified as potential predictors of the risk of postpartum DM among women with GDM history, collectively accounting for 3.6% of the genetic risk.
Conclusions
Both genetic and traditional factors contribute to the risk of GDM in women, operating through diverse mechanisms. Strengthening the risk prediction of SNPs for postpartum DM among women with GDM history is crucial for maternal and child health protection.
Plain Language Summary
We aimed to evaluate a risk prediction model of gestational diabetes mellitus (GDM) based on traditional and genetic factors. A total of 2744 eligible pregnant women were included. Face-to-face questionnaire surveys were conducted to collect general data. Among traditional factors, age ≥30 years old, family history, GDM history, impaired glucose tolerance history, systolic blood pressure ≥116.22 mmHg, diastolic blood pressure ≥74.52 mmHg, fasting plasma glucose ≥5.0 mmol/L, 1-hour postprandial blood glucose ≥8.8 mmol/L, 2-h postprandial blood glucose ≥7.9 mmol/L, total cholesterol ≥4.50 mmol/L, low-density lipoprotein ≥2.09 mmol/L and insulin ≥11.5 mIU/L were independent risk factors for GDM. Among genetic factors, 11 single nucleotide polymorphisms were identified as potential predictors of the risk of postpartum DM among women with GDM history, collectively accounting for 3.6% of the genetic risk. Both genetic and traditional factors increase the risk of GDM in women.
Introduction
Gestational diabetes mellitus (GDM), one of the common pregnancy complications, refers to hyperglycaemia resulting from abnormal glucose metabolism. It is first identified during pregnancy in women with previously underdiagnosed DM and in pregnant women with impaired glucose tolerance (IGT) or DM during pregnancy. The incidence rate of GDM has been increasing annually, posing a serious threat to maternal health (Gao et al. Citation2019). GDM is often difficult to detect in clinical practice, and an abnormal fasting plasma glucose (FPG) level can damage organ function in pregnant women and foetuses, potentially leading to tissue and organ failure in severe cases (Brown and Smith Citation2020). At present, GDM is mainly attributed to insulin resistance and an insufficient compensatory increase in insulin secretion. As a result, the risk of type 2 DM (T2DM) is elevated in both pregnant women and neonates, and complications such as metabolic syndrome may occur. Given the common family history of GDM and T2DM and the increased possibility of GDM complication with T2DM, it is suggested that GDM and T2DM may share a common genetic background (Bellamy et al. Citation2009, Buchanan et al. Citation2012, Popova et al. Citation2021).
Hence, a risk prediction model of GDM based on traditional and genetic factors was constructed in this study. The aim was to protect maternal and child health by exploring the causative factors of GDM, to help pregnant women recover normal physiological functions, to improve physical health, and to relieve clinical symptoms.
Methods
General data
A cohort was established in November 2021 by recruiting pregnant women who were managed with documentation and subjected to regular prenatal examinations in our hospital from 2010 to 2021. Following the questionnaire survey, an electronic health record database was created to predict the risk of GDM. The pregnancy outcomes of women and the postnatal outcomes of their foetuses were regularly followed up. This study was approved by the hospital ethics committee (approval no. PHXUAR20210053). Upon entering the cohort, all women were informed of the purpose of this survey and signed the informed consent.
Inclusion and exclusion criteria
According to the following inclusion and exclusion criteria, a total of 2744 eligible pregnant women were included in this study.
The inclusion criteria were as follows: (1) pregnant women aged ≥18 years old, (2) those within 8–12 weeks of gestation and (3) those examined with the 75-g oral glucose tolerance test (OGTT) during 24–28 weeks of gestation.
The exclusion criteria involved: (1) pregnant women who had a multi-foetal pregnancy, (2) those who underwent therapeutic induction of labour due to foetal malformation or death, (3) those with malignancies, essential hypertension, T1DM or T2DM or (4) those with incomplete medical records.
All the pregnant women received 75-g OGTT during 24–28 weeks of gestation. According to the diagnostic criteria for GDM formulated by the International Association of Diabetes and Pregnancy Study Groups (Chebrolu et al. Citation2021), the pregnant women who met any of the following conditions were diagnosed with GDM: (i) FPG ≥5.1 mmol/L, (ii) 1-hour postprandial blood glucose (1-hPG) ≥10.0 mmol/L and (iii) 2-hPG ≥8.5 mmol/L.
Questionnaire contents
The following information was acquired using a face-to-face questionnaire: age at pregnancy, nationality, education level, occupation, date of last menstruation, history of adverse pregnancy outcomes, medical history, family history of DM, pregnancy and delivery history, use of folic acid, use of assisted reproduction, history of gestational hypertension, and pre-pregnancy weight. Height, weight and blood pressure were measured by professional staff. Women whose data were absent were followed up by telephone calls.
Serum test results were collected from the laboratory information system, including levels of FPG and glycosylated haemoglobin, glucose levels during 75-g OGTT during 24–28 weeks of gestation (FPG, 1-hPG and 2-hPG), blood lipid levels (total cholesterol (TC), triglyceride (TG), high-density lipoprotein (HDL), low-density lipoprotein (LDL)) and insulin level.
Prenatal examination data and pregnancy outcomes were gathered from the Chengdu Maternal and Child Health Information Platform – One-Card System, including weight gain during pregnancy, blood pressure, gestational age at delivery, mode of delivery, weight and height of neonates, and Apgar scores.
Quality control
EpiData 3.1 software was used for data entry, and edit check programming was set up in the database to reduce entry errors. The personnel responsible for data entry received specialised training, and the data were entered independently by two individuals and subsequently checked for accuracy. Multivariate and hierarchical analyses were employed to control for confounding factors during data analysis. The influence of loss to follow-up on the research results was evaluated by comparing the main variables of lost-to-follow-up and non-lost-to-follow-up populations. Confounding factors that could not be controlled by statistical methods were detailed in the limitations of this study.
Detection and selection of single nucleotide polymorphisms (SNPs)
A total of 51 SNPs were screened from the genome-wide association study (GWAS) related to T2DM, hyperglycaemia and insulin resistance. All the SNPs were genotyped using the Sequenom iPLEX assay. Subsequently, SNPs with a typing success rate of less than 90% and those that did not conform to Hardy–Weinberg equilibrium were excluded. Eventually, 40 SNP loci were included for analysis.
Statistical analysis
SPSS 22.0 software (SPSS Inc., Chicago, IL) was used for statistical analysis. The measurement data were tested for normality and homogeneity of variance. The data conforming to (approximate) normal distribution were expressed as mean ± standard deviation x ± s), and the non-normally distributed data were expressed as P50 (P25, P75). The data that complied with normal distribution and homogeneous variance were analysed by Student’s t-test for comparison between groups. If the variance was not homogeneous, the data were analysed by the corrected t-test (approximate t-test), and the non-normally distributed data were compared between groups by the Mann–Whitney U-test. The Chi-square test was used for the count data, and the rank sum test was utilised for the ranked data. p < .05 was considered statistically significant. R software (R Foundation for Statistical Computing, Vienna, Austria) was used to construct a Bayesian model with incorporating traditional and genetic factors to identify the risk factors for the occurrence of GDM.
Results
Basic characteristics of women
The sociodemographic characteristics of the 2744 cases are shown in . A total of 1323 women were diagnosed with GDM, with an incidence rate of 48.21%. Significance differences were observed between the two groups in terms of age, body mass index, number of pregnancies, family history, arterial hypertension history, GDM history, IGT history, systolic blood pressure, diastolic blood pressure and levels of FPG, 1-hPG, 2-hPG, TC, TG, LDH and insulin (p < .05). Other indicators did not show significant differences between the two groups (p > .05). The flowchart of this study is exhibited in .
Table 1. Basic characteristics of women.
Univariate analysis results
Univariate analysis revealed that the difference in prevalence rate was statistically significant for each factor, including age, family history, GDM history, IGT history, systolic blood pressure, diastolic blood pressure and levels of FPG, 1-hPG, 2-hPG, TC, LDL and insulin (p < .05) (Table S1, supporting information).
Multivariate logistic regression analysis results
Multivariate logistic regression analysis was conducted with the presence or absence of GDM as the dependent variable and the influential factors with statistical differences in univariate analysis as the independent variables. The results indicated that age ≥30 years old, family history, GDM history, IGT history, systolic blood pressure ≥116.22 mmHg, diastolic blood pressure ≥74.52 mmHg, FPG ≥5.0 mmol/L, 1-hPG ≥8.8 mmol/L, 2-hPG ≥7.9 mmol/L, TC ≥4.50 mmol/L, LDL ≥2.09 mmol/L and insulin ≥11.5 mIU/L were independent risk factors for GDM ().
Table 2. Multivariate logistic regression analysis results.
Strength of association between GWAS SNPs and postpartum DM among women with GDM history
The genetic risk of postpartum DM among women with GDM history was found to be 12.9% of all SNPs. The SNP with the highest odds ratio (OR) was rs11558471, which indicated the strongest ability to explain the genetic risk (about 1.2% of genetically explained proportion). The A allele frequencies of rs11558471 in women with and without postpartum DM were 50.4% and 39.5%, respectively. In comparison with the G allele, the A allele of rs10906115 showed an approximately 1.275-fold risk of postpartum DM among the women with GDM history ().
Table 3. Strength of association between SNPs and postpartum diabetes mellitus among women with gestational diabetes mellitus history.
Prediction of GWAS SNPs for genetic risk of postpartum DM among women with GDM history and improvement of risk reclassification ability
Based on the SNP (rs11558471) with the strongest ability to explain the genetic risk, other SNPs were successively included in the analysis. According to the improvement of area under the curve (AUC), integrated discrimination index and net reclassification index by SNPs from GWAS, a total of 11 SNP loci (rs2779116, rs5215, rs11605924, rs7072268, rs7172432, rs10811661, rs2191349, rs10830963, rs174550, rs13266634 and rs11071657) were identified as the potential predictors of postpartum DM among women with GDM history, which accounted for 3.6% of the genetic risk (Table S2, supporting information).
Construction of combined prediction model
The variables screened from the above-mentioned traditional and genetic factors were analysed with the Bayesian model constructed by R software. The results revealed that family history, GDM history and IGT history affected the corresponding SNP loci in genetic factors (family history: rs2779116 and rs5215, GDM history: rs2779116, rs5215, rs11605924, rs7072268, rs7172432, rs10811661, rs174550 and rs11071657, and IGT history: rs10830963, rs13266634, rs2191349 and rs7072268). Other traditional factors did not affect the genetic factors ().
Figure 2. Bayesian model with incorporation of traditional and genetic factors for predicting GDM. BP: blood pressure; CHOL: cholesterol; GDM: gestational diabetes mellitus; IGT: impaired glucose tolerance; LDL: low-density lipoprotein. rs2779116, rs5215, rs11605924, rs7072268, rs7172432, rs10811661, rs2191349, rs10830963, rs174550, rs13266634 and rs11071657 are SNP loci.
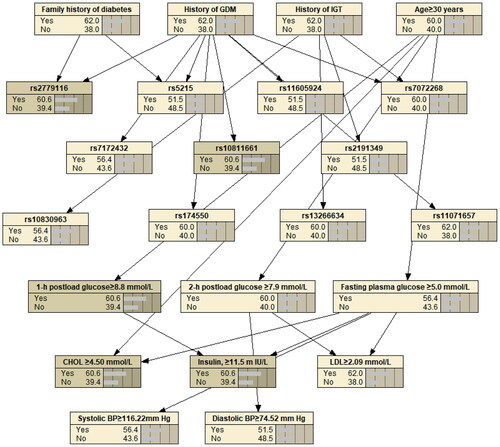
Evaluation of model with incorporation of traditional and genetic factors
The constructed Bayesian model was internally evaluated using the bootstrap method (1000 times of repeated sampling for original data), and 100 pregnant women with GDM admitted to our hospital from January 2022 to May 2022 were selected for external validation. The results revealed that the C-index values of internal evaluation and external validation were 0.820 (95% confidence interval (CI): 0.747–0.893) and 0.803 (95% CI: 0.725–0.881), respectively (). The calibration curves were well fitted to the ideal ones, and the AUC values of internal evaluation and external validation were 0.815 (95% CI: 0.738–0.892) and 0.805 (95% CI: 0.730–0.880), respectively (). A higher net benefit was observed when the threshold probability was within the range of 1–90% in the decision curve (Figure S1, supporting information). The above findings suggested that this model had high predictive accuracy.
Discussion
GDM has an incidence rate of about 1–5%, which is increasing annually (Joglekar et al. Citation2021). GDM may induce glucose metabolism disorders and metabolic acidosis in pregnant women, and long-term hyperglycaemia may lead to hyperinsulinaemia, thereby inhibiting normal insulin secretion, causing metabolic disorders, reducing physical energy and raising the risk of dystocia (Pace et al. Citation2020, Nicolì et al. Citation2021, Sun et al. Citation2021). The pathogenic mechanism of GDM remains unclear, but it has been associated with insulin (e.g. insulin resistance and pancreatic β-cell damage) and oxidative stress (Li et al. Citation2019, Zuccolotto et al. Citation2019, Dalfrà et al. Citation2020). Excessive glucose causes auto-oxidation and protein glycosylation, activates aldose reductase polyol metabolic pathways and promotes the production of reactive oxygen species (Benny et al. Citation2021). This results in lipid peroxidation, which occurs between excessive oxygen free radicals and unsaturated fatty acids on the cell membrane, impairing the structure and function of normal cells (Li et al. Citation2020, Ortega-Contreras et al. Citation2022). In addition, hyperglycaemia may reduce the activity of antioxidant enzymes, thus attenuating the antioxidant defence ability (Li et al. Citation2020, Wei et al. Citation2021). The imbalance between oxidation and antioxidation aggravates tissue and cell damage (Preston et al. Citation2020). Therefore, this study focused on the traditional and genetic factors affecting GDM, aiming to provide new insights for protecting maternal and infant health.
Among the traditional factors, older age, family history, GDM history, IGT history, high systolic and diastolic blood pressure, and high levels of FPG, 1-hPG, 2-hPG, TC, LDL and insulin were identified as independent risk factors for GDM. Pregnancy at an older age is generally recognised as the main risk factor, as the regulation of glucose and lipid metabolism weakens with ageing (Correa-de-Araujo and Yoon Citation2021). The risk of GDM in pregnant women aged ≥40 years is 8.2 times that of the women aged 20–30 years. Family history, GDM history and IGT history may affect the genetic factors in GDM patients. In this study, the SNP with the highest OR was rs11558471, indicating the strongest ability to explain the genetic risk (about 1.2% of genetically explained proportion). In terms of laboratory indicators, GDM-related metabolic abnormalities include increased insulin resistance and cellular defects. Insulin resistance often leads to persistent rise in blood glucose, thereby raising TC and LDL levels, causing apparent metabolic changes, disrupting insulin signalling cascades through inflammatory indicators, and elevating blood pressure (van der Kemp et al. Citation2018, Alharbi et al. Citation2019).
Regarding genetic factors, 11 SNPs were identified with the potential to predict postpartum DM among the women with GDM history, which together explained 3.6% of the genetic risk. In this study, the A allele frequencies of rs11558471 in women with and without postpartum DM were 50.4% and 39.5%, respectively, indicating that rs11558471 had a relatively high genetic influence. Probably, this SNP was associated with GDM susceptibility. In addition, the incorporation of genetic and traditional factors into the Bayesian model revealed that family history, GDM history and IGT history affected the corresponding SNP loci in genetic factors (Wei et al. Citation2020). Finally, the high predictive ability of the model was verified by combining internal evaluation with external validation.
Regardless, this study has some limitations. First, the specific mechanism of genetic factors remains unclear and needs further investigation. Second, the sample size for analysing traditional factors is small, which may introduce bias. Thus, a multicentre collaborative pattern should be utilised in future studies to enlarge the sample size and to reduce potential errors.
Conclusions
In conclusion, both genetic and traditional factors increase the risk of GDM in women, involving multiple mechanisms. Regular monitoring of relevant laboratory indicators is necessary for pregnant women with older age, family history, GDM history, IGT history, high systolic and diastolic blood pressures, and high levels of FPG, 1-hPG, 2-hPG, TC, LDL and insulin. In addition, the risk prediction pattern of SNPs for postpartum DM among women with GDM history should be improved to better protect maternal and child health.
Supplemental Material
Download MS Word (110.4 KB)Disclosure statement
No potential conflict of interest was reported by the author(s).
Data availability statement
The data that support the findings of this study are available from the corresponding author, upon reasonable request.
Additional information
Funding
References
- Alharbi, K. K., et al., 2019. MTNR1B genetic polymorphisms as risk factors for gestational diabetes mellitus: a case-control study in a single tertiary care center. Annals of Saudi Medicine, 39 (5), 309–318.
- Bellamy, L., et al., 2009. Type 2 diabetes mellitus after gestational diabetes: a systematic review and meta-analysis. Lancet, 373 (9677), 1773–1779.
- Benny, P., et al., 2021. Genetic risk factors associated with gestational diabetes in a multi-ethnic population. PLOS One, 16 (12), e0261137.
- Brown, H.L. and Smith, G.N., 2020. Pregnancy complications. Cardiovascular risk factors, and future heart disease. Obstetrics and Gynecology Clinics of North America, 47 (3), 487–495.
- Buchanan, T.A., Xiang, A.H., and Page, K.A., 2012. Gestational diabetes mellitus: risks and management during and after pregnancy. Nature Reviews. Endocrinology, 8 (11), 639–649.
- Chebrolu, P., et al., 2021. Gestational diabetes in rural central India: low prevalence but absence of typical risk factors. Heliyon, 7 (7), e07431.
- Correa-de-Araujo, R. and Yoon, S.S., 2021. Clinical outcomes in high-risk pregnancies due to advanced maternal age. Journal of Women’s Health (2002), 30 (2), 160–167.
- Dalfrà, M.G., et al., 2020. Genetics and epigenetics: new insight on gestational diabetes mellitus. Frontiers in Endocrinology, 11, 602477.
- Gao, C., et al., 2019. Prevalence of gestational diabetes mellitus in mainland China: a systematic review and meta-analysis. Journal of Diabetes Investigation, 10 (1), 154–162.
- Joglekar, M. V., et al., 2021. Postpartum circulating microRNA enhances prediction of future type 2 diabetes in women with previous gestational diabetes. Diabetologia, 64 (7), 1516–1526.
- Li, M., et al., 2020. Genetic factors and risk of type 2 diabetes among women with a history of gestational diabetes: findings from two independent populations. BMJ Open Diabetes Research & Care, 8 (1), e000850.
- Li, S., et al., 2019. Naringenin improves insulin sensitivity in gestational diabetes mellitus mice through AMPK. Nutrition & Diabetes, 9 (1), 28.
- Li, Y.-X., et al., 2020. Gestational diabetes mellitus in women increased the risk of neonatal infection via inflammation and autophagy in the placenta. Medicine, 99 (40), e22152.
- Nicolì, F., et al., 2021. Use of non-nutritive-sweetened soft drink and risk of gestational diabetes. Diabetes Research and Clinical Practice, 178, 108943.
- Ortega-Contreras, B., et al., 2022. Pathophysiological role of genetic factors associated with gestational diabetes mellitus. Frontiers in Physiology, 13, 769924.
- Pace, R., et al., 2020. Preventing diabetes after pregnancy with gestational diabetes in a Cree community: an inductive thematic analysis. BMJ Open Diabetes Research & Care, 8 (1), e001286.
- Popova, P. V., et al., 2021. Association of common genetic risk variants with gestational diabetes mellitus and their role in GDM prediction. Frontiers in Endocrinology, 12, 628582.
- Preston, E.V., et al., 2020. Climate factors and gestational diabetes mellitus risk – a systematic review. Environmental Health, 19 (1), 112.
- Sun, Z., et al., 2021. Gestational diabetes mellitus and risks of gynecologic cancers: results from a nationwide Swedish twin study. Gynecologic Oncology, 162 (1), 142–147.
- van der Kemp, J., et al., 2018. Women-specific risk factors for heart failure: a genetic approach. Maturitas, 109, 104–111.
- Wei, Q., Chen, X., and Chen, H., 2020. Association of single nucleotide polymorphisms of the IL-6, IL-10, and TNF-α genes with susceptibility to gestational diabetes mellitus. Genetic Testing and Molecular Biomarkers, 24 (7), 390–398.
- Wei, W., et al., 2021. Gestational diabetes mellitus: the genetic susceptibility behind the disease. Hormone and Metabolic Research = Hormon- Und Stoffwechselforschung = Hormones et Metabolisme, 53 (8), 489–498.
- Zuccolotto, D.C.C., et al., 2019. Dietary patterns of pregnant women, maternal excessive body weight and gestational diabetes. Revista de Saude Publica, 53, 52.