ABSTRACT
Technological innovation has become critical for market survival for many companies in the era of digital transformation. However, the organisational perspective to constantly stay up-to-date with technological innovations can become strenuous on an individual level due to the need to constantly adapt to the demands of a permanently changing technological environment. Based on a large-scale online survey of employees in Germany (N = 1,115), we investigated the positive and negative effects of an organisational climate of innovation from a digital stress perspective. Our results indicate that an organisational climate of innovation creates uncertainty in individuals as it supports technological change but can also help to reduce the perception of technological unreliability as it fosters an environment of mutual support. This research, therefore, adds to the idea of a pronounced innovation climate as a double-edged sword and shows that permanently pushing technological innovation should also be viewed in the light of its potential side effects. It follows that innovation initiatives should balance organisational and individual requirements to stay, or become, more competitive, thereby explicitly considering the side effects that too much and/or too fast innovation may have on users.
1. Introduction
Being able to handle changes has become a key challenge for many companies, with information and communication technologies (ICT) being a major cause of the constant struggle to innovate (Sambamurthy, Bharadwaj, and Grover Citation2003). Not only do products or services that are provided by companies undergo constant changes, but so do the ICT that are used to support their creation. In this context, it has been argued that an information system ‘that suppresses an organization’s ability to be agile cannot be tolerated as it may lead to the demise of the organization’ (Seo and La Paz Citation2008, 139). The idea of not only keeping pace, but actively changing the organisational technology landscape to be prepared for the innovation affordances that a rapidly changing business environment demands has become critical (García-Morales, Ruiz-Moreno, and Llorens-Montes Citation2007).
Companies and their members consequently face an ever-increasing number of technological innovations (i.e. technologies or practices that they use for the first time, Klein, Conn, and Sorra Citation2001). This was, for example, illustrated by Meyer et al. (Citation2017) who investigated the technology use of a group of heavy ICT users (i.e. software developers). They found that their 20 participants collectively used 331 different applications over the study period (11 work days on average), with an average of 16 different applications per day. Such overwhelming use, among other reasons, may explain why Brynjolfsson (Citation1993), almost three decades ago, prominently discussed the general notion that the use of ICT is not a straight way to success for organisations. He presented his work on the ‘productivity paradox of IT’, which illustrated that the greater diffusion of IT (in the United States during the 1970s and 1980s) was not accompanied by a comparable increase in productivity. In the meantime, we also learned that it is not only possible that the use of ICT may not lead to the awaited benefits on the societal and organisational levels, but that it may also lead to negative effects, in particular on the individual level.
For such negative consequences of the use of digital technologies at work, Brod (Citation1982) coined the term ‘technostress’ and tellingly defined this phenomenon as (754): ‘ … a condition resulting from the inability of an individual or organization to adapt to the introduction and operation of new technology’. In line with this definition of technostress (or ‘digital stress’ as we will refer to it in the context of this study), early studies on ICT’s downsides have focused on the disruptive changes caused by technology implementations and their potential adverse consequences (e.g. Wastell and Newman Citation1993; Korunka, Weiss, and Karetta Citation1993; Smith and Carayon Citation1995). The increased diffusion of ICT has also led to increased research interest into the impact of digital stress on individual and organisational well-being (e.g. Riedl Citation2013; Fischer and Riedl Citation2017). The current wave of digitalisation (Legner et al. Citation2017) and specific technological trends (e.g. ubiquitous computing, Cascio and Montealegre Citation2016) indicate that the disruptive potential of technological change in the workplace will increase even further in the future.
Importantly, the social context in the organisation and in particular organisational culture and climate are crucial for an organisation and its members to keep up with the demands of being consistently innovative (Glisson Citation2015). Managers play a key role in this context, as they can support the creation of an organisational climate of innovation (Watts and Henderson Citation2006), which is characterised by openness to new ideas and mutual support (e.g. Ekvall Citation1996). Such an organisational climate can not only improve organisational creativity and innovation, but also positively affects individual well-being (e.g. Rasulzada and Dackert Citation2009).
Yet, the managerial-level view of technological change as being solely positive should be contested. Dwivedi et al. (Citation2015) highlighted that perceptions related to information systems can differ between groups and individuals (particularly between the manager and user views). Therefore, although it seems that technological innovation, characterised by frequent updates, upgrades and reengineering of organisational ICT can lead to substantial positive organisational effects (e.g. Melville, Kraemer, and Gurbaxani Citation2004), it is not certain that this is also true on the individual user level. Vaniea and Rashidi (Citation2016), for example, investigated how users experience the process of a software update and found that an update (i.e. a change in the technological environment) is linked to perceived user risk (e.g. lack of usefulness of new features, new bugs, unreliability of the system after the update) and may, therefore, involve substantial perceived uncertainty.
Rafferty and Griffin (Citation2006) also found that the frequency of change is an important characteristic of organisational change that leads to individual uncertainty perceptions related to the new technologies. This finding is of particular importance in the context of technological change, as the current digital transformation wave further accelerates the rate of technological innovation. In line with this development, a recent survey (N = 682 respondents, with 48% being members of the senior management or even higher in the organisational hierarchy, Harvard Business Review Analytic Services Citation2016) found that a lack of risk tolerance and the inability to experiment are amongst the five most important barriers to the successful use of digital technologies. More than 30% of the respondents in this survey also indicated that the individual ability to adapt to change is the most important skill sought in employees. The organisational pressure to innovate is, therefore, translated into a demand on the individual to constantly keep up with technological change and to cope with the resulting uncertainty.
In the study reported in the present paper, we are therefore interested in pursuing the idea that an organisational climate that encourages and supports the implementation of technological innovations (Klein and Sorra Citation1996) and therefore entails organisational disruption can also have negative side effects. In particular, we want to answer the following research question:
“Can an organizational climate of innovation lead to individual stress perceptions related to the implementation of new technology?”
2. Theoretical framework
In their discussion on the two-faced nature of a creative environment, Isaksen and Ekvall (Citation2010) supported the notion that innovation may not only be perceived as beneficial, creating positive forms of tension (e.g. constructive debates that lead to new ideas), but also as harmful when individual interests are at stake and conflict arises. One potential avenue to explain why an organisational climate of innovation can be in conflict with individual interests is through the uncertainty that can accompany organisational changes. Edwards (Citation2000), for example, argues (451): ‘Change in the repertoire (partial or total) through innovation is not guaranteed rather, it is inherently uncertain. It represents an attempt to alter the organization-specific practices previously embedded over time in its operating procedures’. This effect is also reported by Rafferty and Griffin (Citation2006) who found that perceived uncertainty mediates the relationship between organisational change and important job outcomes (i.e. reduced job satisfaction and increased turnover intention). The structure of the research model utilised by Rafferty and Griffin (Citation2006), which includes organisational change leading to the perceptions of stressors (i.e. uncertainty in their case) that then influence organisational outcomes is also common in research on digital stress. Research on digital stress does not only focus on the effects of ICT on individuals in isolation but has rather followed the recommendations by Orlikowski and Barley (Citation2001) to combine the strengths of research on information technology and research on organisations. Accordingly, organisational stress theories are the basis for most of the research into digital stress, which is particularly evident in the structure of the research models that are applied in many of these investigations. This structure includes three key components: (1) situation, (2) stressors, and (3) effects or outcomes. As prominent studies on digital stress have adopted this structure before (e.g. Ragu-Nathan et al. Citation2008; Ayyagari, Grover, and Purvis Citation2011; Adam et al. Citation2017), we also use it as the basis for this investigation.
2.1. Situation
The situational characteristic that serves as the focus of this study is an organisational climate that supports technological change. For technological innovation, a distinction can be made between the use of a novel technology and its creation (i.e. idea utilisation as opposed to idea generation, Kim and Chung Citation2017) with the focus here being on the implementation of a new technology (i.e. its continued use, Klein and Sorra Citation1996) rather than the initial decision to adopt it in the organisation. As outlined by Coccia and colleagues (e.g. Coccia Citation2017, Citation2019a, Citation2019b; Coccia and Watts Citation2020), technological innovation is an evolutionary process that requires the ability to consistently solve problems (e.g. cope with unexpected behaviours of new technology). In such a process human affect can play an important role, with behavioural consequences. This has, for example, been indicated by Brinks (Citation2020) who argues that certain situations (e.g. use of specific technologies) will be repeated if they lead to positive affective reactions in individuals or, conversely, not continued if they lead to negative effects, such as stress.
Cowan, Sanditov, and Weehuizen (Citation2011) in this context highlight that (179): ‘Ultimately, what matters is not whether an innovation in itself is stress-inducing or stress-reducing, but rather the conditions under which it is so’. This claim has been supported frequently by studies that showed that an organisational climate that encourages learning and interaction among its members supports the innovation process (e.g. Albrecht and Hall Citation1991; Andersson, Moen, and Brett Citation2020; Ramirez Heller, Berger, and Brodbeck Citation2014). Motivated by this empirical finding, we focus on the potential relationship between an organisational climate that supports the implementation of technological innovations within organisations and individual perceptions of stress related to these technologies. Innovation is therefore treated as an independent variable in our theoretical framework (Janssen Citation2004) and we assume that technological innovation will not be sustainable within an organisational climate that leads to increased stress perceptions.
To further define the concept of an organisational climate, we have to distinguish it from the related concept of an organisational culture. For this purpose, we use the distinction made by Denison (Citation1996) and differentiate culture and climate as follows:
[Culture is the] deep structure of organizations, which is rooted in the values, beliefs, and assumptions held by organizational members [… while climate is] rooted in the organization’s value system, but tends to present […] social environments in relatively static terms, describing them in terms of a fixed (and broadly applicable) set of dimensions. (624)
For organisational climate, several conceptualizations have been proposed, such as the four dimensions model of organisational climate by James and James (Citation1989) (role stress and lack of harmony; job challenge and autonomy; leadership facilitation and support; work group cooperation, friendliness, and warmth). However, generalised climate conceptualizations such as the one by James and James have also been criticised. Schneider (Citation2000), for example, argued that the dimensionality of organisational climate should depend on the specific phenomenon (topic) of an investigation. It follows that broad dimensions are typically less useful than more specific ones. Based on this rationale, we draw upon the classification of organisational climates by Patterson et al. (Citation2005). In this classification, four types of primary climates are distinguished based on whether they have a more external or internal orientation and whether they have a more flexible or controlled orientation. One of the quadrants in this two-by-two classification is referred to as the ‘open systems model’ and is essentially a climate that is open to external impulses (e.g. new technological trends) and flexible in its structure (e.g. constantly testing new ideas and having dynamic procedures).
These facets can also be found in conceptualizations of an organisational climate of innovation that focus on technology implementation. For example, Klein, Conn, and Sorra (Citation2001) highlight that an ‘implementation climate’ is characterised by (813): ‘ … employees perceiv[ing] that innovation implementation is a major organizational priority – promoted, supported, and rewarded by the organization … ’. A comparable definition has also been adopted in the context of research on digital stress by Tarafdar, Tu, and Ragu-Nathan (Citation2010) who proposed that an organisational climate that is supportive of innovation (315) ‘ … encourage[s] communication, encourage[s] new ideas, and promote[s] supportive relationships among employees … ’. Due to a comparable focus on digital stress and the fit with the ‘open systems model’ (i.e. utilisation of new ideas and organisational structures to support their implementation), we adopt the definition by Tarafdar, Tu, and Ragu-Nathan (Citation2010) for the purpose of this study.
2.2. Stressors
The Transactional Theory of Stress by Lazarus and Folkman (Citation1984) has become one of the most established theories in the context of digital stress (Fischer and Riedl Citation2015). In their review of prominent theories of organisational stress, Sonnentag and Frese (Citation2013) highlight that the Transactional Theory conceptualises stress as a constant exchange between the individual (e.g. with certain desires and available resources) and the environment (e.g. with certain situational demands such as work-related tasks). If this exchange between the individual and situational demands is out of balance (e.g. too much work than can be handled by the employee), detrimental effects for individual well-being and job-related performance can be the consequence.
Importantly, situational appraisal plays a key role in this exchange. More specifically, while the individual constantly processes environmental stimuli (e.g. visual stimuli and sounds), only few of these stimuli are processed consciously and constitute situational demands (e.g. job tasks that currently require attention). These situational demands (e.g. workload) do not always lead to negative consequences (e.g. work-related exhaustion), which can be explained by potential differences in individual appraisal. While one individual can consider certain situational demands as a challenge, another one can perceive them as a threat (e.g. due to different desires, such as one individual desiring skill development and new tasks while another desires a structured job with high levels of routine) (Cummings and Cooper Citation1998). Due to these differences in appraisal, we should consider that even situational characteristics that are often regarded as mainly beneficial to individuals such as an organisational climate of innovation could be a source of distress.
Sources of distress (i.e. stressors) in the context of digital technologies are often operationalised through the five ‘technostress creators’ introduced by Ragu-Nathan et al. (Citation2008). These stressors include (Tarafdar et al. Citation2011, 117): overload (‘too much’), invasion (‘always connected’), complexity (‘difficult’), insecurity (‘uncomfortable’), and uncertainty (‘too often and unfamiliar’). Amongst these stressors, we already highlighted uncertainty as a potential consequence of disruptive changes in organisations at several points before and will therefore focus on this specific stressor as a potential negative effect of an organisational climate of innovation. In previous research on digital stress, Ayyagari, Grover, and Purvis (Citation2011) linked characteristics of the technological environment such as perceived uncertainty to general work-related stressors (e.g. role ambiguity). Further, Ragu-Nathan et al. (Citation2008) present a conceptualisation of uncertainty that is specific to ICT. While they describe that uncertainty felt in relation to rapid changes of ICT in the organisation ‘ … is similar to role ambiguity in that both describe situations involving ambiguity about expectations and outcomes … ’ (430), they particularly highlight that ‘ … ICT changes and upgrades unsettle users and create uncertainty so that they must constantly learn and educate themselves about new ICTs’ (427). From a user perspective, it is not certain though that effort invested into keeping up with new technological developments will be worthwhile; moreover, education effort also takes away time from actual work tasks (Ayyagari, Grover, and Purvis Citation2011).
Although the five technostress creators are established stressors in the context of research on digital stress (e.g. Sarabadani, Carter, and Compeau Citation2018), previous research has also highlighted that there is a potential for many more stressors related to ICT to be part of future investigations (Fischer, Pehböck, and Riedl Citation2019). For example, in more recent research, this list has been extended by further stressors such as unreliability (a sense that ICT does not perform as expected, Adam et al. Citation2017; Fischer and Riedl Citation2015; Riedl et al. Citation2012). Reliability is a core characteristic of ICT as it encapsulates the need for a system to be ‘dependable’ (DeLone and McLean Citation2003, 18) in general and in particular ‘ … to perform promised service dependably and accurately … ’ (Jiang, Klein, and Carr Citation2002, 146). A lack of reliability (unreliability) can have grave consequences. For example, a downtime of Amazon’s website on ‘Prime Day’ in 2018 caused up to $ 100 million in lost sales (Wolfe Citation2018). Technological change is often accompanied by such undesirable effects (Benamati and Lederer Citation2001b), with minor hassles (e.g. slow response times or unexpected system behaviours) being a well-known problem (e.g. Riedl and Fischer Citation2018). As we conceptualised an organisational climate of innovation as having two main facets (i.e. implementation of new ideas and mutual support), we also chose to focus on two ICT-related stressors, which are expected to each have a unique relationship with these facets. Next to perceived uncertainty, we chose perceived unreliability as the second stressor as it is also among the most prevalent ICT-related stressors (e.g. Riedl Citation2013).
2.3. Outcomes
In their review, Sarabadani, Carter, and Compeau (Citation2018) summarised important outcome variables that have been frequently studied in the context of digital stress, which include user satisfaction, job satisfaction, performance, productivity, and commitment. They found that all of these outcome variables are negatively affected by the aforementioned set of technostress creators. Amongst these outcome variables, we decided to focus on user satisfaction, as it is an outcome variable specific to the context of technology use in organisations and job satisfaction as a more general outcome that is indicative of how employees feel about their work in general.
As shown by DeLone and McLean (Citation1992) in their review of measures of information systems success, user satisfaction is amongst the most important variables to determine, whether new technology has been successfully implemented. This circumstance is further highlighted in the theoretical model presented by DeLone and McLean (Citation2003) which posits that user satisfaction positively influences the actual use of technology, a relationship that has subsequently been validated in numerous studies (Petter, DeLone, and McLean Citation2008). In line with Bhattacherjee (Citation2001), we broadly define user satisfaction as (359): ‘[u]ser’s affect with (feelings about) prior [ICT] use.’ Such a wide definition is needed due to the practice to operationalise user satisfaction as a form of enjoyment and satisfaction with the use of a specific type of technology, often involving particular characteristics of the specific technology (e.g. hard- and software features, quality of information, or the provided outputs, DeLone and McLean Citation1992).
As ICT provided in the work environment are part of the resources that are required to successfully fulfil job demands, we argue that technological changes will also have consequences for job satisfaction. This has, for example, been found by Spector and Jex (Citation1998) who report that organisational constraints (e.g. interruptions and faulty equipment) negatively correlated with job satisfaction. Hence, while an organisational climate of innovation may not have a negative effect on job satisfaction in itself, its potential by-products in the form of uncertainty and unreliability could be mediators that adversely affect the ‘ … pleasurable or positive emotional state resulting from the appraisal of one’s job or job experiences’ (Locke Citation1976, 1300).
In below, we summarise the five main constructs that we focus on in this investigation and the working definitions that we base our understanding of these constructs on.
Table 1. Construct definitions for main components in the research model.
2.4. Hypothesis development
The first relationship deals with the relationship between situational characteristics, in our study an organisational climate of innovation, and the two stressors, namely perceived uncertainty and perceived unreliability.
In line with the notion supported by Orlikowski and Barley (Citation2001) that organisational influences (e.g. organisational climate) can act as an enabling force, but also as a constraining force, we argue that the two facets of an organisational climate of innovation (i.e. implementation of new ideas and mutual support) hint at its potential dual nature related to stress appraisal. In previous research on digital stress, these two aspects have mostly been investigated in isolation and the research focus has therefore also been mostly on either its beneficial or harmful nature (we highlight this circumstance in Appendix 1). In this study, focus on both sides based on the following evidence.
In a survey study by Ayyagari, Grover, and Purvis (Citation2011) it was found that among the characteristics of an organisation’s technological environment, its pace of change is most strongly related to role ambiguity perceived by individuals (role ambiguity is defined as a ‘[lack of] certainty about duties, authority, allocation of time, and relationships with others; the clarity or existence of guides, directives, policies; and the ability to predict sanctions as outcomes of behaviour’, Rizzo, House, and Lirtzman Citation1970, 156). Agility and a high pace of change are promoted in organisations with a climate of innovation (Crocitto and Youssef Citation2003); yet, such a climate may also cause individual uncertainty related to organisational ICT. The underlying rationale of this relationship is that the less constant an individual’s environment, the higher the perceived uncertainty. Evidence in brain research (e.g. Critchley, Mathias, and Dolan Citation2001) and in IS research (e.g. Pavlou, Liang, and Xue Citation2007) supports this rationale.
While a climate of innovation can foster change and therefore uncertainty (i.e. through new, disruptive ideas that are then implemented, such as new ICT), it also provides individuals with an environment in which resources are available to deal with the affordances of new ICT (i.e. through mutual support, for example, when new ICT do not function as expected). For example, King et al. (Citation2007) found that individuals showed a better work performance in an organisational climate of innovation. Specifically, they found that individuals were able to better deal with the distress caused by work demands (e.g. the feeling of being overwhelmed) in such an organisational climate. In the technology context, Day et al. (Citation2012) found that the provision of organisational support to handle ICT hassles (e.g. personal assistance to deal with technology malfunctions) reduced the likelihood of individuals showing signs of reduced well-being (e.g. reports of headache or symptoms of burnout). It therefore becomes clear that an organisational climate of innovation can both tax individual resources by causing change, and hence uncertainty, but that it also provides individuals with resources (e.g. mutual support by members of the organisation) to cope with stress. Related to our two stressors, we therefore hypothesise that while an organisational climate of innovation will increase perceived uncertainty, it also offers the resources to reduce perceived unreliability of workplace technology:
H1a: An organizational climate of innovation positively affects perceived uncertainty.
H1b: An organizational climate of innovation negatively affects perceived unreliability.
For the relationship between the two stressors and the two outcome variables (user satisfaction and job satisfaction), we partly replicate previous research findings. Hence, in line with the results of previous research on digital stress (e.g. Tarafdar, Tu, and Ragu-Nathan Citation2010; Ragu-Nathan et al. Citation2008), and in particular based on the review results by Sarabadani, Carter, and Compeau (Citation2018), we expect that both stressors will be negatively related to our outcome variables. While these hypotheses are in line with previous findings, we emphasise that unlike previous research investigating the combined impact of five ‘Technostress Creators’, we look at the individual impact of individual stressors. Moreover, replication of existing research findings is a significantly neglected topic in research in general (Camerer et al. Citation2016, Citation2018) and specifically in IS research (Dennis and Valacich Citation2014). In addition, while we expect negative effects of both stressors on our outcome variables, perceived unreliability has, to our knowledge, not been part of any previous survey studies that looked at its effects on user satisfaction or job satisfaction.
H2a: Perceived uncertainty negatively affects user satisfaction.
H2b: Perceived uncertainty negatively affects job satisfaction.
H2c: Perceived unreliability negatively affects user satisfaction.
H2d: Perceived unreliability negatively affects job satisfaction.
Next to the relationship between situational characteristics and stressors (H1) and the relationship between stressors and outcomes (H2), we also expect further relationships between the included variables that we discuss briefly, as we do not include specific hypotheses for them. While not the specific focus of the present study, these relationships are included to create a more complete research model, one that is in line with the findings of previous research and therefore more closely resembles reality. First, next to the mediating effect of an organisational climate of innovation on our outcome variables through its impact on the two included stressors, we can also assume a direct relationship. Such a relationship can also be supported by the findings of previous studies in the context of the impact of organisational climate and related practices on individual satisfaction with work and performance at work (e.g. Eisenberger et al. Citation1997; Johnson and McIntye Citation1998; Lee et al. Citation2014; Delaney and Huselid Citation1996; Gelade and Ivery Citation2003; Ramirez Heller, Berger, and Brodbeck Citation2014).
Second, next to the direct link between situation and outcomes, we can also assume relationships between our two stressors and our two outcome variables. For our included stressors this may be indicated by the previous use of higher-order constructs in the context of research on digital stress (e.g. the ‘Technostress Creators’ by Ragu-Nathan et al. Citation2008, which is a second-order construct with five reflective dimensions). Yet, we still keep these stressors separate (i.e. without using a higher-order construct) as previous research into the separate effects of ICT-related stressors has shown that there is a possibility that non-consistent effect patterns emerge (e.g. in the study by Ahmad, Amin, and Ismail Citation2014, out of the five Technostress Creators dimensions, only two where significantly related to organisational commitment).
For the directionality of this relationship, there is only sparse evidence in the context of research on digital stress, with Shu, Tu, and Wang (Citation2011) highlighting that these two stressors often accompany each other when new systems are introduced but they do not discuss whether the perception of these stressors could influence each other. We find some evidence for a potential directionality in a recent survey by Morris, Becker, and Parkin (Citation2019) though, who found that the process of changing a system causes uncertainty, particularly as users are expecting problems with their device if an update takes place.
For user satisfaction and job satisfaction, evidence for a potential connection is not as clear. While satisfaction with certain characteristics of ICT has been found to be a correlate of job satisfaction before (e.g. satisfaction with information provided by ICT or system usefulness, Ang and Soh Citation1997), this correlation has not been stable throughout previous research. For example, Joshi and Rai (Citation2000) found no direct relationship between the perceived quality of the information provided by ICT at work (a potential indicator of user satisfaction, Petter, DeLone, and McLean Citation2008; DeLone and McLean Citation2003) and job satisfaction. Rather, they found that the relationship was fully mediated by role stressors (i.e. role ambiguity and role conflict).
Based on these initial arguments and findings, we tentatively expect that (i) perceived uncertainty will be positively related to perceived unreliability and that (ii) user satisfaction will be positively related to job satisfaction. Yet, the evidence regarding these relationships is only sparse and we therefore retain the option to exclude these relationships should they impact the quality of the tested model in a negative way (e.g. in terms of model fit).
In and , we present our research model, which illustrates how the main components of our investigation are related to each other. Hypothesised relationships are indicated by solid lines while additional relationships that are tentatively explored are indicated by dashed lines (i.e. they may be removed from the model should they reduce its explanatory power or quality in terms of model fit).
Figure 1. Research model. Solid lines between constructs indicate hypothesised relationships while dashed lines indicate relationships that can be assumed based on previous research but may be removed for parsimony reasons.
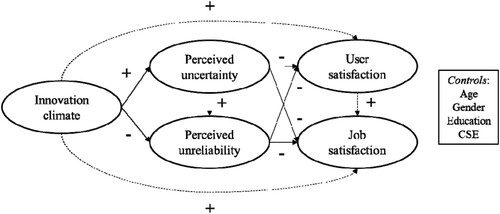
Figure 2. Overview of study results. Model fit: χ² = 572.07; d.f. = 274; χ²/d.f. = 2.09 (< 5; Wheaton et al. Citation1977); CFI = .960 (>.95; Hu and Bentler Citation1999); RMSEA = .044 (<.06 / <.08; Hu and Bentler Citation1999; Hooper, Coughlan, and Mullen Citation2008), SRMR = .048 (<.05 / <.08; Hu and Bentler Citation1999; Hooper, Coughlan, and Mullen Citation2008); GFI = .925 (>.90; Hooper, Coughlan, and Mullen Citation2008); AGFI = .904 (>.90; Hooper, Coughlan, and Mullen Citation2008); NFI = .927 (>.90 / >.95; Bentler and Bonett Citation1980; Hu and Bentler Citation1999); TLI (NNFI) = .953 (>.80 / >.95; Hu and Bentler Citation1999; Hooper, Coughlan, and Mullen Citation2008).
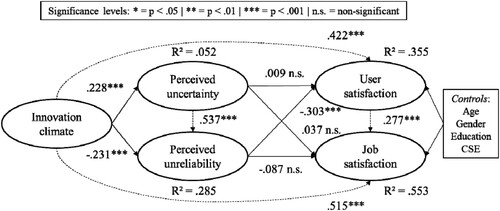
In addition to the main constructs of our study, we also included age, gender, level of education, and computer self-efficacy as control variables. Age, gender and education are variables that are routinely reported as sample characteristics in the context of research on ICT-related stress and have also been found to be related to this type of stress perception (e.g. Tams, Thatcher, and Grover Citation2018; Riedl et al. Citation2013; Ragu-Nathan et al. Citation2008). With regard to self-efficacy in general, it has been argued that people tend to avoid situations for which they may not have the capabilities to deal with. However, if they have sufficient self-efficacy, they will face the struggle and try to push through such situations until they succeed (Igbaria and Iivari Citation1995). In line with this idea, in social cognitive theory, self-efficacy is defined as ‘confidence in one’s ability to cope with a stressful situation’ (Wood and Bandura Citation1989, 588), which was extended by Compeau and Higgins (Citation1995), who define computer self-efficacy as the ‘ … judgment of one’s capability to use a computer’ (192). Because computer self-efficacy may have a positive impact on both user satisfaction (e.g. Adam Mahmood et al. Citation2000) and job satisfaction (e.g. Henry and Stone Citation1995), we included it as a control in the present study.
3. Methodology
In this section, we discuss the survey study and the data analysis procedures that were used to test our research model.
3.1. Data collection
We used a survey design to test our research model. According to the findings of a review on the data collection methods used in previous research on digital stress by Fischer and Riedl (Citation2017), self-report measures are by far the most prominent type of measurement method. This circumstance can, for example, be justified from a theoretical point of view, as a survey approach or self-reports in general are in line with the Transactional Theory of Stress by Lazarus and Folkman (Citation1984), because individual perceptions and their appraisal are pivotal for the emergence of stress. Therefore, we collected our data through an online survey that was distributed by a market research company (i.e. Bilendi; see https://www.bilendi.co.uk/). To make it clear for our respondents that we are interested in the organisational context, we provided an introductory text that explained that we focus on ICT at work. Further, we also provided a list of technologies that we consider as ICT in line with Ayyagari, Grover, and Purvis (Citation2011, A2) (e.g. communication technologies such as e-mail or generic application technologies such as word processing software).
We aimed for a sample that is representative of the German employed population (i.e. average age close to 44 years, Destatis - Statistisches Bundesamt Citation2018; with approximately equal gender distribution, Destatis - Statistisches Bundesamt Citation2019). We gathered a total of N = 1,392 completed surveys of which 265 were removed due to a significant amount of missing data (i.e. more than 10% of answers were ‘Don’t know’); a further 6 questionnaires were removed because of low engagement (i.e. standard deviation of answers below .5) (e.g. DeSimone, Harms, and DeSimone Citation2015; Meade and Craig Citation2012), and an additional 6 questionnaires were removed as they did not completely indicate their demographic information (i.e. age, gender, or highest level of education). Hence, our final sample is N = 1,115 completed questionnaires.
We used established measures for construct measurement, except for perceived unreliability. We mainly used a 7-point Likert scale for measurement (1 – strongly disagree to 7 – strongly agree) and the survey was administered in German. For innovation climate we used the 5-item scale by Tarafdar, Tu, and Ragu-Nathan (Citation2010) (sample item: ‘We have a very open communications environment.’). For user satisfaction we used the 4-item scale by Bhattacherjee (Citation2001) (e.g. ‘How do you feel about your overall experience of utilising ICT in connection with your work tasks?). For job satisfaction we used the 3-item scale by Ragu-Nathan et al. (Citation2008) (e.g. ‘I like doing the things I do at work.’). For perceived uncertainty we used the 4-item scale by Ragu-Nathan et al. (Citation2008) (e.g. ‘There are constant changes in computer software in our organization.’). For computer self-efficacy we used the 10-item scale by Compeau and Higgins (Citation1995) (e.g. ‘I could complete my tasks using new ICTs if there was no one around to tell me what to do as I go.’). The three remaining controls were measured as follows: age as metric variable in years, gender as nominal variable (male, female), and education as ordinal variable (no degree, compulsory education, vocational training, university-entrance diploma, university degree, other).
At the time of data collection, there was no existing scale for perceived unreliability available and we therefore developed a measurement instrument. The items were based on the indicators used to measure reliability in a seminal paper by Ayyagari, Grover, and Purvis (Citation2011) and centred around the idea of ICT in the workplace being ‘too unstable’ (Fischer and Riedl Citation2015; Adam et al. Citation2017) (e.g. ‘I think that I lose too much time due to technical malfunctions.’). For the development of the scale for perceived unreliability, initially formulated items were circulated at the authors’ research institution and refined based on the feedback (e.g. unclear formulations were changed). We then included the preliminary measure in a survey that took place at this institution in the beginning of fall 2017, which resulted in N = 198 complete questionnaires mostly from students and faculty. The reliability score (coefficient alpha) of this initial instrument was .756 and we decided to retain all four items for this study, as we aimed for at least three strong items during exploratory factor analysis (EFA) (Costello and Osborne Citation2005; Reio and Shuck Citation2015). In line with our introduction of the unreliability construct, these items mainly reflect the impact of constant hassles rather than singular disruptive events and since the time of data collection, comparable instruments have been used by Adam et al. (Citation2017). An overview of our measurement instruments and initial results of our reliability and validity assessments, which are presented in more detail in the next section, can be found in Appendix 2.
For our analyses, the complete sample was randomly split up into two sub-samples, one for the exploratory factor analysis (EFA) (N = 564) and one for the confirmatory factor analysis (CFA) (N = 551) and the causal modelling in accordance with comparable studies such as Ragu-Nathan et al. (Citation2008). After splitting our sample, we used Levene tests of all included indicators to test whether these two samples were statistically different. As none of these tests were statistically significant at the .05 level, the sub-samples are comparable (see for an overview of the main characteristics for both samples; further descriptive statistics for both sub-samples, including indicator means, standard deviations, skewness and kurtosis, can be found in Appendix 2). Further, we achieve an item-to-subject ratio of 19:1 (N = 564 / 30 items) for our EFA, which is above the recommended thresholds of 10:1 and close to 20:1 (Costello and Osborne Citation2005).
Table 2. Overview of samples used for EFA, CFA and SEM.
3.2. Reliability and Validity Assessment
We conducted an exploratory factor analysis (EFA) with all items in our latent constructs in SPSS 25. First, we tested the normality assumption for all our indicators using Shapiro-Wilks tests and found that univariate normality cannot be assumed. Hence, instead of maximum likelihood we used principal axis factoring as our extraction method (Costello and Osborne Citation2005). For the rotation we used promax, as oblique methods are generally recommended for the social and behavioural sciences where correlations amongst constructs should always be expected (Treiblmaier and Filzmoser Citation2010; Reio and Shuck Citation2015; Matsunaga Citation2010). As we have an a priori theory of the dimensionality of our indicators, we fixed the extraction to six factors (i.e. innovation climate, user satisfaction, job satisfaction, uncertainty, unreliability, computer self-efficacy), which is generally favoured over unconstrained extraction based on Eigenvalues alone (Costello and Osborne Citation2005; Reio and Shuck Citation2015).
Barlett’s test was significant (p < .001) and we reached a KMO (Kaiser-Meyer-Olkin criterion) of .881 (‘meritorious’ according to Kaiser and Rice Citation1974; values above .90 would be considered ‘marvelous’), which indicates good potential for dimension reduction. The six extracted factors explained 64.20% of the total variance, which is well above the recommended threshold of 40% (Reio and Shuck Citation2015). After removing items with high cross-loadings or low loadings on their main factor, we ended up with the factor structure presented in Appendix 2. Importantly, though we had to remove several items, no factor includes less than three high loading items (Costello and Osborne Citation2005; Reio and Shuck Citation2015). The pattern matrices, including all loadings and cross-loadings for the initial EFA and the final EFA can be found in Appendix 3.
Using our holdout sample, we then tested our measurement model based on a confirmatory factor analysis (CFA) (Matsunaga Citation2010) using AMOS 25. The model fit for our measurement model was acceptable with a χ² of 447.4, d.f. of 215, χ²/d.f. of 2.08 (<5; Wheaton et al. Citation1977), CFI of .969 (>.95; Hu and Bentler Citation1999), RMSEA of .044 (<.60; Hu and Bentler Citation1999) and SRMR of .041 (<.08; Hu and Bentler Citation1999). We therefore accepted the factor structure of our measurement model. In (Appendix 4), we summarise the results including the composite reliability for all constructs, which is acceptable (>.7; Nunnally and Bernstein Citation1994; see also Appendix 2 for the coefficient alpha values as another indicator of reliability, which are also acceptable).
For convergent validity, we assessed the average variance extracted (AVE) for each construct, which should be above .5 (MacKenzie, Podsakoff, and Podsakoff Citation2011). In the case of job satisfaction, this value was missed slightly, but we retain the construct nonetheless as the specific value for this threshold has been criticised before (e.g. Malhotra and Dash Citation2011). For discriminant validity, we applied the Fornell-Larcker criterion (i.e. square root of AVE for a construct should be larger than any correlations with other constructs, Fornell and Larcker Citation1981), which is fulfilled in all cases. In addition, we assessed the heterotrait-monotrait ratio of correlations (HTMT) as a second indicator of discriminant validity (Henseler, Ringle, and Sarstedt Citation2015). As none of the values reported in Table A7 (Appendix 4) are above the more lenient threshold of .900 or even the more strict threshold of .850 (Henseler, Ringle, and Sarstedt Citation2015), we can assume discriminant validity for our constructs based on this criterion as well.
As all of our data was measured through the same approach (i.e. self-reports), we tested for the potential influence of common method bias (Podsakoff et al. Citation2003). In line with current recommendations, we used several methods to assess the influence of CMB on our results (Turel, Serenko, and Giles Citation2011; Turel Citation2015). First, we used Harman’s Single Factor test (Harman Citation1976) in SPSS (i.e. principal component analysis with no rotation), with the first and largest factor explaining 26.59% of the total variance, which is clearly below a majority of the total variance. Second, we inspected the inter-construct correlations matrix (see Table A6 in Appendix 4), looking for significant correlations above .90 (Pavlou, Liang, and Xue Citation2007). As our correlations range from |.026| to |.678|, this criterion also does not indicate common method bias. Third, we used the common latent factor approach (Podsakoff et al. Citation2003; Turel Citation2016; Podsakoff, MacKenzie, and Podsakoff Citation2012) in AMOS, comparing an unconstrained model (χ² = 447.0, d.f. = 409) to a zero-constrained model (χ² = 447.0, d.f. = 409), which showed no statistically significant difference (Δ χ² = 0, Δ d.f. = 0, p = 1.000). Hence, we can assume that there is no significant influence of common method bias on our results.
As AMOS uses Maximum Likelihood for model estimation, we further had to ensure that multivariate normality could be assumed based on our data (Kline Citation2011). For this purpose, we first tested for multicollinearity in SPSS for our endogenous variables user satisfaction and job satisfaction. As the variance inflation factors (VIF) are below 10 (Kline Citation2011) and even below 4 (O’brien Citation2007) in each case (User satisfaction: unreliability: 1.021, computer self-efficacy: 1.028, innovation climate: 1.042; Job satisfaction: unreliability: 1.021, computer self-efficacy: 1.028, innovation climate: 1.042), we can rule out multicollinearity as an influencing factor. We then also tested for influential outliers in SPSS using Cook’s Distance (Cook Citation1977). As the largest Cook’s Distance (i.e. .076 between computer self-efficacy and job satisfaction) was clearly below the threshold of 1 (Cook and Weisberg Citation1982), we can also rule out outliers as an influential factor and can therefore assume multivariate normality. As our data does not show a normal distribution on the univariate level, we used bootstrapping with 2000 samples for all model estimations.
3.3. Model estimation
For our model estimation, we first introduced our control variables (i.e. age, gender, education, computer-self-efficacy) and assessed their influence on each of our five main constructs. Together, the four control variables explained 3.0% of the variance in organisational climate of innovation, 2.8% of the variance in perceived uncertainty, 7.7% of variance in perceived unreliability, 7.1% of the variance in user satisfaction, and 9.2% of the variance in job satisfaction. For the test of our hypotheses, we then only retained the effects between the control variables and our main dependent variables (i.e. user satisfaction and job satisfaction).
The model fit for our research model (see ), was overall acceptable with a χ² of 572.07, d.f. of 274, χ²/d.f. of 2.09 (<5; Wheaton et al. Citation1977), CFI of .960 (>.95; Hu and Bentler Citation1999; not fulfilled), RMSEA of .044 (<.60 / <.80; Hu and Bentler Citation1999; Hooper, Coughlan, and Mullen Citation2008) SRMR of .048 (<.05 / <.08; Hu and Bentler Citation1999; Hooper, Coughlan, and Mullen Citation2008), GFI of .925 (>.90; Hooper, Coughlan, and Mullen Citation2008, not fulfilled), AGFI of .904 (>.90; Hooper, Coughlan, and Mullen Citation2008, not fulfilled), NFI of .927 (>.90 / >.95; Bentler and Bonett Citation1980; Hu and Bentler Citation1999, not fulfilled), and TLI of .953 (>.80 / >.95; Hooper, Coughlan, and Mullen Citation2008; Hu and Bentler Citation1999). As previously noted, this research model included a number of further relationships for which we did not formulate hypotheses. Hence, we also ran a model in which these relationships were removed (i.e. relationships with dashed lines in ). The model fit for this simplified model was overall not acceptable with a χ² of 958.63, d.f. of 278, χ²/d.f. of 3.45 (<5; Wheaton et al. Citation1977), CFI of .909 (>.95; Hu and Bentler Citation1999; not fulfilled), RMSEA of .067 (<.60 / <.80; Hu and Bentler Citation1999; Hooper, Coughlan, and Mullen Citation2008) SRMR of .104 (<.05 / <.08; Hu and Bentler Citation1999; Hooper, Coughlan, and Mullen Citation2008), GFI of .878 (>.90; Hooper, Coughlan, and Mullen Citation2008, not fulfilled), AGFI of .846 (>.90; Hooper, Coughlan, and Mullen Citation2008, not fulfilled), NFI of .877 (>.90 / >.95; Bentler and Bonett Citation1980; Hu and Bentler Citation1999, not fulfilled), and TLI of .894 (>.80 / >.95; Hooper, Coughlan, and Mullen Citation2008; Hu and Bentler Citation1999). Hence, the results for these further relationships are also discussed in the next section.
4. Results and discussion
In this section, we present the main results of our model estimation and discuss their implications for research and practice.
4.1. Results of model estimation
In , we summarise the estimates for the effects included in our research model, their effect sizes and whether the related hypotheses were supported or not.
Table 3. Model estimates and overview of study results.
In the tested model, the support for our hypothesised relationships is mixed and although H1 is fully supported, only one of the four effects hypothesised in H2 is significant. More specifically, we only find that perceived unreliability (e.g. faulty equipment and related time loss) negatively affects user satisfaction (H2c), which is in line with the assumption that reliability is a core characteristic of information systems success (e.g. Petter, DeLone, and McLean Citation2008). Importantly though, we find support for duality of an organisational climate of innovation related to stress as it increases the perception of uncertainty (H1a), while it decreases the perception of unreliability (H1b).
In addition, we find that the inclusion of direct relationships between an organisational climate of innovation and user satisfaction as well as job satisfaction has merit, as both relationships are significant and part of the model with the overall better model fit. This finding is comparable to the study results reported by Cullen et al. (Citation2014) who found that while change-related uncertainty had no effect on job satisfaction, there was a positive relationship between perceived organisational support and job satisfaction. In their study though, organisational support was included as a mediator of the relationship between stressors and outcomes rather than as a situational characteristic that is an antecedent to stress appraisal.
Regarding the relationships between our stressors and our outcome variables, while we find both relationships to be significant (i.e. perceived uncertainty positively affects perceived unreliability and user satisfaction positively affects job satisfaction), the effect size for the relationship between user satisfaction and job satisfaction is negligible and therefore only of minor importance. The positive effect of perceived uncertainty on perceived unreliability is the most substantial effect in our model (based on Cohen Citation1992 it can be considered a large effect as the f² value is >.35).
A potential avenue to explain this relationship can be found in research into the emotional effects of uncertainty. For example, in an experimental study which involved the affective evaluation of movies, Bar-Anan, Wilson, and Gilbert (Citation2009) found that introducing uncertainty (through verbal cues) led to more extreme evaluations (positive or negative). This effect can be explained by the human tendency of increasing attention and arousal in uncertain situations (Carretié et al. Citation2004; Fiorillo, Tobler, and Schultz Citation2003). It follows that uncertainty that results from technological changes may also lead to increased awareness of potential ICT unreliability (i.e. a positive effect of perceived uncertainty on perceived unreliability).
Hence, while perceived uncertainty itself does not have a negative impact on user satisfaction or job satisfaction, we can assess its indirect effect through perceived unreliability on user satisfaction. We find a standardised effect of β = −.163 (bootstrapped confidence interval: −.224 to −.114 with a standard error of .033). Hence, perceived uncertainty can have a negative impact, but mainly through other stressors (i.e. perceived unreliability in our study). It has to be noted though that this effect is significant, but not substantial due to the small effect size of the relationship between perceived unreliability and user satisfaction (f² = .064).
Overall, the effects in our model explain 5.2% of the variance in perceived uncertainty, 28.5% of the variance in perceived unreliability, 35.5% of the variance in user satisfaction, and 55.3% of the variance in job satisfaction. Based on the relationships included in our research model (), it can therefore be stated that while there is also a potential negative side to having an organisational climate of innovation (i.e. increasing perceived uncertainty), overall its positive side (i.e. reduction of perceived unreliability and direct positive effects on user satisfaction and job satisfaction) outweighs its negative effects.
4.2. Implications for research
This study investigated the potential dual nature of an organisational climate of innovation as an antecedent to the appraisal of distress related to technological change in organisations. In contrast to previous research, it was found that such a climate can lead to stress perceptions in the form of heightened uncertainty, while at the same providing organisational members with a way to deal with ICT-related distress caused by the unreliability of technology. This finding creates a more sophisticated picture of the effects that managerial attempts at fostering technological change (by implementing an organisational climate of innovation) can have on individual well-being. Importantly, it highlights that, while technological change can be a source of uncertainty, the way with which it is implemented is crucial. In a climate that allows for mutual support of organisational members, the downsides of technological change (e.g. heightened awareness of ICT unreliability due to uncertainty) can be alleviated substantially. If this facet of an organisational climate of innovation is neglected though, technological change can have detrimental effects. This is, for example, supported by results of a study by Wang, Shu, and Tu (Citation2008) who found that an organisational environment in which innovation is accompanied by competitiveness amongst organisational members will lead to an increase in ICT-related distress appraisal. Edwards (Citation2000) in this context argues that (452): ‘An organization’s ability to deal with […] uncertainty depends […] on the extent to which the innovation can be unpacked and the extent to which individuals have the necessary skills and knowledge to do so.’ As mutual support amongst individuals allows for the transfer of such skills and knowledge, an organisational climate of innovation cannot only be an environment of uncertainty, but also an environment of learning and successful technological change.
The positive side of such an organisational climate was further evidenced by the initially not hypothesised direct effects of an organisational climate of innovation on user satisfaction and job satisfaction. Further, the related effects were more substantial in size than the effects of an organisational climate of innovation on ICT-related distress appraisal (i.e. f² of .189 for user satisfaction and f² of .336 for job satisfaction as compared to an f² of .055 for perceived uncertainty and an f² of .085 for perceived unreliability, see ). Hence, while an organisational climate of innovation plays an important role in the context digital stress, it plays an even more important role for the general job-related well-being of individuals. Nonetheless, additional research on the stress-related effects of such situational characteristics should not be neglected and, in particular, additional research is needed that addresses the unique relationships of such characteristics with ICT-related stressors.
In addition, in their review of previous research that used the Technostress Creators set of digital stressors, Sarabadani, Carter, and Compeau (Citation2018) found that there is a lack of investigations into the antecedents and effects of separate ICT-related stressors. Only one paper in their review (i.e. Ahmad, Amin, and Ismail Citation2014) investigated separate effects and showed that no consistent pattern emerged (e.g. only overload and uncertainty where significantly related to organisational commitment and, contrary to expectations, both enhanced commitment). In the context of this study too, the widely used conceptualisation of digital stress as a higher-order construct (Ragu-Nathan et al. Citation2008; Sarabadani, Carter, and Compeau Citation2018) would not have revealed the dual nature of an organisational climate of innovation. Therefore, conceptualizations such as the one used by Ayyagari, Grover, and Purvis (Citation2011), which investigate situational characteristics, stressors, and outcomes in separation should be favoured. This separation led to more actionable insights, which can be the basis for a number of practical implications.
4.3. Implications for practice
We found that technological change in organisations can have important positive effects (i.e. increased user satisfaction, increased job satisfaction, and a means to deal with the unreliability of ICT). However, we also found negative side effects of an organisational climate of innovation that should not be neglected. It is critical to consider these effects, as change is a constant companion for many organisations, particularly in the current era of digital transformation (Hess et al. Citation2016). The need for technological change, however, has already been highlighted in the early 2000s. For example, Benamati and Lederer (Citation2001b) argued that ‘[d]ue to today’s highly competitive environment, the failure to capitalize on new IT can lead to lost opportunities and can be especially costly’ (184). In line with this ‘quasi-obligation’ for companies to embrace change, they found that in order to cope with rapid technological change, most companies enforce an organisational climate of innovation that supports learning and procedures to implement technological changes (Benamati and Lederer Citation2001a).
What our study suggests is the fact that these attempts at handling change are translating environmental uncertainty (e.g. new technologies and resulting competitive challenges) into internal uncertainty (e.g. ambiguity related to employed technologies) which employees have to deal with in their daily business. Further, our study also revealed that this perceived uncertainty may translate into elevated perceptions of unreliability of technology. Because perceived unreliability of technology may negatively affect intention and actual use of computer systems (e.g. Liang and Xue Citation2009), which, in turn, are necessary preconditions for the positive effects of ICT use (e.g. increased access to information, better decision quality, higher productivity; e.g. Brynjolfsson and Hitt Citation2000), we argue that organisations should not blindly introduce and establish a climate of innovation. Rather, responsible managers should always consider the potential negative effects of their actions and the potential remedies. As shown in this study, technological change being accompanied by mutual support (instead of fostering competitiveness, Wang, Shu, and Tu Citation2008) can be beneficial to individuals, though it is not the sole option to deal with uncertainty (e.g. quality of change communication, participation in decision-making, or increased job autonomy are also viable options, Bordia et al. Citation2003).
5. Conclusion
In this study, we showed that an organisational climate of innovation, which embraces technological change, has benefits, but also downsides. In particular, we found that such an organisational climate has facets that can increase the perception of ICT-related stressors (i.e. perceived uncertainty), but also offers the means to reduce the perception of other ICT-related stressors (i.e. perceived unreliability). From a practical perspective, this finding shows that technological change, if implemented in the right environment (i.e. an environment of mutual support as found in this study) can be coped with effectively by individuals. Our findings also highlight the need for more in-depth investigations into the specific relationships between ICT-related stressors and situational characteristics, as each of the included stressors (i.e. perceived uncertainty and perceived unreliability) was affected differently by an organisational climate of innovation and, in turn, also affected important ICT-related and job-related outcome variables (i.e. user satisfaction and job satisfaction) in different ways.
This study also has some limitations, which can be opportunities for future research. First, as we strived for parsimony in our research model, there are a variety of ways to extend the nomological network that was the basis for this investigation of which we want to highlight a few related to (i) situation, (ii) stressors, and (iii) outcomes.
A first way to extend this line of research on the effects of technological change is to look at different aspects of change, as presented in the study by Rafferty and Griffin (Citation2006) (e.g. frequency of change). In line with their findings, we can, for example, expect that environments with more frequent changes are also accompanied by higher individual levels of perceived uncertainty. Also, further aspects of the organisational environment that help to establish a climate of innovation, such as progressive HRM practices (e.g. Gelade and Ivery Citation2003), or that enhance its positive effects, such as distributional fairness (e.g. Shih and Susanto Citation2011), could be added.
In addition, environmental characteristics that are outside of the organisation could have an influence on this relationship such as the country or industry that an organisation operates in and the interest in and support structures for innovation of this country or industry as suggested by Coccia and colleagues (e.g. Coccia Citation2018, Citation2019c; Coccia and Watts Citation2020). In line with the findings of Nybakk, Crespell, and Hansen (Citation2011) it would also be worthwhile to look at more specific aspects aside from the country of operation alone, such as national culture. For example, Shane (Citation1995) found that in cultures characterised by high levels of uncertainty avoidance (Hofstede, Hofstede, and Minkov Citation2010), individuals were less likely to champion innovation efforts.
Next to environmental characteristics, further individual characteristics aside from the control variables included in this study (i.e. age, gender, education, computer self-efficacy) could help us to understand in which situations technological change can lead to distress and in turn to the successful implementation of technological innovations (Kim and Chung Citation2017). For example, Cullen et al. (Citation2014) found that individual adaptability is negatively related to perceived uncertainty, while we can expect that individual characteristics such as a dispositional resistance to change (Oreg Citation2003) may increase the likelihood that individuals feel uncertainty that results from technological change. In this context, it is also important to look at different combinations of personality traits and their influence on stressor perception, as shown recently by Khedhaouria and Cucchi (Citation2019).
Related to stress appraisal, it could be worthwhile to not only focus on distress, but also consider the option of technological change leading to eustress (i.e. situations being perceived as challenge rather than as a hindrance, see, for example, Tarafdar, Cooper, and Stich Citation2019 or Zhao, Xia, and Huang Citation2020). In addition, further ICT-related stressors should be included (e.g. complexity or insecurity, Ragu-Nathan et al. Citation2008) to further investigate the separate effects that an organisational climate of innovation has on stress appraisal. Related to potential outcomes, next to the dependent variables highlighted by Sarabadani, Carter, and Compeau (Citation2018) (e.g. performance, productivity, commitment), it could prove interesting to not only focus on psychological well-being, but also on physiological well-being. For example, Pollard (Citation2001) found that disruptive changes in the workplace (i.e. workplace reorganisation) can lead to substantial reduction of physiological well-being (e.g. elevated levels of blood pressure and heightened levels of total cholesterol), which was particularly evident during phases of highest uncertainty (i.e. closest to the actual implementation of changes).
Second, we used a general definition of ICT based on the classification of technologies presented by Ayyagari, Grover, and Purvis (Citation2011). It would be worthwhile to test whether the relationships we identified can also be found for more specific technologies, and the resulting focus on more specific stressors leads to differences in effect sizes. Existing research streams to which our study could be linked are stress resulting from ICT-related security measures (e.g. D'Arcy, Herath, and Shoss Citation2014) or stress resulting from the use of social media (e.g. Maier et al. Citation2015).
Third, analysing the same relationships in the context of more specific populations could lead to different results and we argue that it could particularly influence the effect sizes. Earlier research into the stress of IS professionals specifically hints at that possibility. For example, Sethi, King, and Quick (Citation2004) found that the high demands of continuous training for IS professionals can have detrimental effects such as lower levels of job satisfaction. Based on the recent insights into the work life of software developers by Meyer et al. (Citation2017), who found that software developers, on average, use around 16 different applications per day and more than 40 per month, we can assume that unreliability of provided technologies might have a more substantial impact on overall job satisfaction in such a population. It would therefore be interesting to see additional, context-specific investigations into the effects of techno-unreliability, particularly in job environments that are characterised by a high dependence on technology (such as software engineering or service providers in social media marketing; first evidence of the physiological effects of techno-unreliability in such a sample population is provided in a pilot study by Kalischko, Fischer, and Riedl Citation2019).
Fourth, we collected our data in a cross-sectional online survey. Though we controlled for common method bias, our data collection approach nonetheless has weaknesses that need to be addressed in future research. For example, from a strict methodological viewpoint, causal inference is only possible based on longitudinal data or experimental designs that imply deliberate manipulation of the independent variable. Such study designs would be needed to investigate the proposed evolutionary process of innovations as outlined by Coccia and colleagues (e.g. Coccia Citation2017, Citation2019a, Citation2019b) or the influence of actual innovative work behaviours on organisational performance (e.g. Shanker et al. Citation2017). It would also be worthwhile to investigate the potential of other types of data collection in addition to self-reports to shed more light on the effects and coping mechanisms related to perceived uncertainty and unreliability. As shown in a systematic review (Riedl Citation2013), ICT-related stressors such as unreliability of systems may negatively affect a number of physiological parameters, including elevations of stress hormones (e.g. adrenaline, noradrenaline, or cortisol) or changed patterns of autonomic nervous system activity (e.g. increased heart rate, reduced heart rate variability, or muscle tension).
Disclosure statement
No potential conflict of interest was reported by the authors.
Additional information
Funding
References
- Adam, M. T. P., H. Gimpel, A. Maedche, and R. Riedl. 2017. “Design Blueprint for Stress-Sensitive Adaptive Enterprise Systems.” Business & Information Systems Engineering 59 (4): 277–291. doi:https://doi.org/10.1007/s12599-016-0451-3.
- Adam Mahmood, M., J. M. Burn, L. A. Gemoets, and C. Jacquez. 2000. “Variables Affecting Information Technology end-User Satisfaction: A Meta-Analysis of the Empirical Literature.” International Journal of Human-Computer Studies 52 (4): 751–771. doi:https://doi.org/10.1006/ijhc.1999.0353.
- Ahmad, U. N. U., S. M. Amin, and W. K. W. Ismail. 2014. “Moderating Effect of Technostress Inhibitors on the Relationship Between Technostress Creators and Organisational Commitment.” Jurnal Teknologi 67 (1): 51–62.
- Albrecht, T. L., and B‘J’ Hall. 1991. “Facilitating Talk About new Ideas: The Role of Personal Relationships in Organizational Innovation.” Communication Monographs 58 (3): 273–288. doi:https://doi.org/10.1080/03637759109376230.
- Andersson, M., O. Moen, and P. O. Brett. 2020. “The Organizational Climate for Psychological Safety: Associations with SMEs’ Innovation Capabilities and Innovation Performance.” Journal of Engineering and Technology Management 55: 101554. doi:https://doi.org/10.1016/j.jengtecman.2020.101554.
- Ang, J., and P. H. Soh. 1997. “User Information Satisfaction, job Satisfaction and Computer Background: An Exploratory Study.” Information & Management 32 (5): 255–266. doi:https://doi.org/10.1016/S0378-7206(97)00030-X.
- Ayyagari, R., V. Grover, and R. Purvis. 2011. “Technostress: Technological Antecedents and Implications.” MIS Quarterly 35 (4): 831–858.
- Bar-Anan, Y., T. D. Wilson, and D. T. Gilbert. 2009. “The Feeling of Uncertainty Intensifies Affective Reactions.” Emotion 9 (1): 123–127. doi:https://doi.org/10.1037/a0014607.
- Benamati, J., and A. L. Lederer. 2001a. “How IT Organizations Handle Rapid IT Change: Five Coping Mechanisms.” Information Technology and Management 2 (1): 95–112. doi:https://doi.org/10.1023/A:1009986821907.
- Benamati, J., and A. L. Lederer. 2001b. “Rapid Information Technology Change, Coping Mechanisms, and the Emerging Technologies Group.” Journal of Management Information Systems 17 (4): 183–202. doi:https://doi.org/10.1080/07421222.2001.11045663.
- Bentler, P. M., and D. G. Bonett. 1980. “Significance Tests and Goodness of fit in the Analysis of Covariance Structures.” Psychological Bulletin 88 (3): 588–606. doi:https://doi.org/10.1037/0033-2909.88.3.588.
- Bhattacherjee, A. 2001. “Understanding Information Systems Continuance: An Expectation-Confirmation Model.” MIS Quarterly 25 (3): 351–370. doi:https://doi.org/10.2307/3250921.
- Bordia, P., E. Hobman, E. Jones, C. Gallois, and V. J. Callan. 2003. “Uncertainty During Organizational Change: Types, Consequences, and Management Strategies.” Journal of Business and Psychology 18 (4): 507–532. doi:https://doi.org/10.1023/B:JOBU.0000028449.99127.f7.
- Brinks, V. 2020. “Fun or Frustration?: How Emotions Shape User Innovation Processes. Emotion.” Space and Society 34: 100651. doi:https://doi.org/10.1016/j.emospa.2019.100651.
- Brod, C. 1982. “Managing Technostress: Optimizing the Use of Computer Technology.” Personnel Journal 61 (10): 753–757.
- Brynjolfsson, E. 1993. “The Productivity Paradox of Information Technology.” Communications of the ACM 36 (12): 66–77. doi:https://doi.org/10.1145/163298.163309.
- Brynjolfsson, E., and L. M. Hitt. 2000. “Beyond Computation: Information Technology.” Organizational Transformation and Business Performance. Journal of Economic Perspectives 14 (4): 23–48. doi:https://doi.org/10.1257/jep.14.4.23.
- Califf, C., S. Sarker, S. Sarker, and C. Fitzgerald. 2015. “The Bright and Dark Sides of Technostress: An Empirical Study of Healthcare Workers.” In: AIS (ed) Proceedings of ICIS 2015.
- Camerer, C. F., A. Dreber, E. Forsell, T.-H. Ho, J. Huber, M. Johannesson, M. Kirchler, et al. 2016. “Evaluating Replicability of Laboratory Experiments in Economics.” Science 351 (6280): 1433–1436. doi:https://doi.org/10.1126/science.aaf0918.
- Camerer, C. F., A. Dreber, F. Holzmeister, T.-H. Ho, J. Huber, M. Johannesson, M. Kirchler, et al. 2018. “Evaluating the Replicability of Social Science Experiments in Nature and Science between 2010 and 2015.” Nature Human Behaviour 2 (9): 637–644. doi:https://doi.org/10.1038/s41562-018-0399-z.
- Carretié, L., J. A. Hinojosa, M. Martín-Loeches, F. Mercado, and M. Tapia. 2004. “Automatic Attention to Emotional Stimuli: Neural Correlates.” Human Brain Mapping 22 (4): 290–299. doi:https://doi.org/10.1002/hbm.20037.
- Cascio, W. F., and R. Montealegre. 2016. “How Technology Is Changing Work and Organizations.” Annual Review of Organizational Psychology and Organizational Behavior 3 (1): 349–375. doi:https://doi.org/10.1146/annurev-orgpsych-041015-062352.
- Coccia, M. 2017. “Sources of Technological Innovation: Radical and Incremental Innovation Problem-Driven to Support Competitive Advantage of Firms.” Technology Analysis & Strategic Management 29 (9): 1048–1061. doi:https://doi.org/10.1080/09537325.2016.1268682.
- Coccia, M. 2018. “Optimization in R&D Intensity and tax on Corporate Profits for Supporting Labor Productivity of Nations.” The Journal of Technology Transfer 43 (3): 792–814. doi:https://doi.org/10.1007/s10961-017-9572-1.
- Coccia, M. 2019a. “A Theory of Classification and Evolution of Technologies within a Generalised Darwinism.” Technology Analysis & Strategic Management 31 (5): 517–531. doi:https://doi.org/10.1080/09537325.2018.1523385.
- Coccia, M. 2019b. “The Theory of Technological Parasitism for the Measurement of the Evolution of Technology and Technological Forecasting.” Technological Forecasting and Social Change 141: 289–304. doi:https://doi.org/10.1016/j.techfore.2018.12.012.
- Coccia, M. 2019c. “Why do Nations Produce Science Advances and New Technology?” Technology in Society 59: 101124. doi:https://doi.org/10.1016/j.techsoc.2019.03.007.
- Coccia, M., and J. Watts. 2020. “A Theory of the Evolution of Technology: Technological Parasitism and the Implications for Innovation Magement.” Journal of Engineering and Technology Management 55: 101552. doi:https://doi.org/10.1016/j.jengtecman.2019.11.003.
- Cohen, J. 1992. “A Power Primer.” Psychological Bulletin 112 (1): 155–159.
- Compeau, D. R., and C. A. Higgins. 1995. “Computer Self-Efficacy: Development of a Measure and Initial Test.” MIS Quarterly 19 (2): 189–211. doi:https://doi.org/10.2307/249688.
- Cook, R. D. 1977. “Detection of Influential Observation in Linear Regression.” Technometrics 19 (1): 15. doi:https://doi.org/10.2307/1268249.
- Cook, R. D., and S. Weisberg. 1982. Residuals and Influence in Regression. New York: Chapman and Hall.
- Costello, A. B., and J. W. Osborne. 2005. “Best Practices in Exploratory Factor Analysis: Four Recommendations for Getting the Most From Your Analysis. Practical Assessment.” Research and Evaluation 10 (7): 1–9.
- Cowan, R., B. Sanditov, and R. Weehuizen. 2011. “Productivity Effects of Innovation, Stress and Social Relations.” Journal of Economic Behavior & Organization 79 (3): 165–182. doi:https://doi.org/10.1016/j.jebo.2011.01.028.
- Critchley, H. D., C. J. Mathias, and R. J. Dolan. 2001. “Neural Activity in the Human Brain Relating to Uncertainty and Arousal During Anticipation.” Neuron 29 (2): 537–545. doi:https://doi.org/10.1016/S0896-6273(01)00225-2.
- Crocitto, M., and M. Youssef. 2003. “The Human Side of Organizational Agility.” Industrial Management & Data Systems 103 (6): 388–397. doi:https://doi.org/10.1108/02635570310479963.
- Cronbach, L. J., and P. E. Meehl. 1955. “Construct Validity in Psychological Tests.” Psychological Bulletin 52 (4): 281–302. doi:https://doi.org/10.1037/h0040957.
- Cullen, K. L., B. D. Edwards, W. C. Casper, and K. R. Gue. 2014. “Employees’ Adaptability and Perceptions of Change-Related Uncertainty: Implications for Perceived Organizational Support, Job Satisfaction, and Performance.” Journal of Business and Psychology 29 (2): 269–280. doi:https://doi.org/10.1007/s10869-013-9312-y.
- Cummings, T. G., and C. L. Cooper. 1998. “A Cybernetic Theory of Organizational Stress.” In Theories of Organizational Stress, edited by C. L. Cooper, 101–121. Oxford, NY: Oxford University Press.
- D'Arcy, J., T. Herath, and M. K. Shoss. 2014. “Understanding Employee Responses to Stressful Information Security Requirements: A Coping Perspective.” Journal of Management Information Systems 31 (2): 285–318. doi:https://doi.org/10.2753/MIS0742-1222310210.
- Day, A., S. Paquet, N. Scott, and L. Hambley. 2012. “Perceived Information and Communication Technology (ICT) Demands on Employee Outcomes: the Moderating Effect of Organizational ICT Support.” Journal of Occupational Health Psychology 17 (4): 473–491. doi:https://doi.org/10.1037/a0029837.
- Delaney, J. T., and M. A. Huselid. 1996. “The Impact of Human Resource Management Practices on Perceptions of Organizational Performance.” Academy of Management Journal 39 (4): 949–969. doi:https://doi.org/10.5465/256718.
- DeLone, W. H., and E. R. McLean. 1992. “Information Systems Success: The Quest for the Dependent Variable.” Information Systems Research 3 (1): 60–95. doi:https://doi.org/10.1287/isre.3.1.60.
- DeLone, W. H., and E. R. McLean. 2003. “The DeLone and McLean Model of Information System Success: A Ten-Year Update.” Journal of Management Information Systems 19 (4): 9–30.
- Denison, D. R. 1996. “What is the Difference Between Organizational Culture and Organizational Climate? A Native’s Point of View on a Decade of Paradigm Wars.” The Academy of Management Review 21 (3): 619–654.
- Dennis, A., and J. Valacich. 2014. “A Replication Manifesto.” AIS Transactions on Replication Research 1: 1–4. doi:https://doi.org/10.17705/1atrr.00001.
- DeSimone, J. A., P. D. Harms, and A. J. DeSimone. 2015. “Best Practice Recommendations for Data Screening.” Journal of Organizational Behavior 36 (2): 171–181. doi:https://doi.org/10.1002/job.1962.
- Destatis - Statistisches Bundesamt. 2018. Persons in employment are 44 years on average.
- Destatis - Statistisches Bundesamt. 2019. Employment - Labor force participation. https://www.destatis.de/EN/Themes/Labour/Labour-Market/Employment/Tables/et-etq-2008-2018.html.
- Dwivedi, Y. K., D. Wastell, S. Laumer, H. Z. Henriksen, M. D. Myers, D. Bunker, A. Elbanna, M. N. Ravishankar, and S. C. Srivastava. 2015. “Research on Information Systems Failures and Successes: Status Update and Future Directions.” Information Systems Frontiers 17 (1): 143–157. doi:https://doi.org/10.1007/s10796-014-9500-y.
- Edwards, T. 2000. “Innovation and Organizational Change: Developments Towards an Interactive Process Perspective.” Technology Analysis & Strategic Management 12 (4): 445–464. doi:https://doi.org/10.1080/713698496.
- Eisenberger, R., J. Cummings, S. Armeli, and P. Lynch. 1997. “Perceived Organizational Support, Discretionary Treatment, and job Satisfaction.” Journal of Applied Psychology 82 (5): 812–820.
- Ekvall, G. 1996. “Organizational Climate for Creativity and Innovation.” European Journal of Work and Organizational Psychology 5 (1): 105–123. doi:https://doi.org/10.1080/13594329608414845.
- Fiorillo, C. D., P. N. Tobler, and W. Schultz. 2003. “Discrete Coding of Reward Probability and Uncertainty by Dopamine Neurons.” Science 299 (5614): 1898–1902. doi:https://doi.org/10.1126/science.1077349.
- Fischer, T., A. Pehböck, and R. Riedl. 2019. “Is the Technostress Creators Inventory Still an Up-To-Date Measurement Instrument? Results of a Large-Scale Interview Study.” In Proceedings of the 14th International Conference on Wirtschaftsinformatik, Siegen, Germany, edited by T. Ludwig and V. Pipek, 1834–1845. Osnabrück: AIS (Association for Information Systems).
- Fischer, T., and R. Riedl. 2015. “Theorizing Technostress in Organizations: A Cybernetic Approach.” In Proceedings of the 12th International Conference on Wirtschaftsinformatik, edited by O. Thomas and F. Teuteberg, 1453–1467. Osnabrück: AIS (Association for Information Systems).
- Fischer, T., and R. Riedl. 2017. “Technostress Research: A Nurturing Ground for Measurement Pluralism?” Communications of the Association for Information Systems 40 (1): 375–401.
- Fornell, C., and D. F. Larcker. 1981. “Evaluating Structural Equation Models with Unobservable Variables and Measurement Error.” Journal of Marketing Research 18 (1): 39. doi:https://doi.org/10.2307/3151312.
- Fuglseth, A. M., and Ø Sørebø. 2014. “The Effects of Technostress within the Context of Employee Use of ICT.” Computers in Human Behavior 40: 161–170.
- García-Morales, V. J., A. Ruiz-Moreno, and F. J. Llorens-Montes. 2007. “Effects of Technology Absorptive Capacity and Technology Proactivity on Organizational Learning.” Innovation and Performance: An Empirical Examination. Technology Analysis & Strategic Management 19 (4): 527–558. doi:https://doi.org/10.1080/09537320701403540.
- Gelade, G. A., and M. Ivery. 2003. “The Impact of Human Resource Management and Work Climate on Organizational Performance.” Personnel Psychology 56 (2): 383–404. doi:https://doi.org/10.1111/j.1744-6570.2003.tb00155.x.
- Glisson, C. 2015. “The Role of Organizational Culture and Climate in Innovation and Effectiveness.” Human Service Organizations, Management, Leadership & Governance 39 (4): 245–250. doi:https://doi.org/10.1080/23303131.2015.1087770.
- Harman, H. H. 1976. Modern Factor Analysis. Chicago, London: University of Chicago Press.
- Harvard Business Review Analytic Services. 2016. Accelerating the pace and impact of digital transformation.
- Henry, J. W., and R. W. Stone. 1995. “Computer Self-Efficacy and Outcome Expectancy.” ACM SIGCPR Computer Personnel 16 (4): 15–34. doi:https://doi.org/10.1145/219716.219722.
- Henseler, J., C. M. Ringle, and M. Sarstedt. 2015. “A new Criterion for Assessing Discriminant Validity in Variance-Based Structural Equation Modeling.” Journal of the Academy of Marketing Science 43 (1): 115–135. doi:https://doi.org/10.1007/s11747-014-0403-8.
- Hess, T., C. Matt, A. Benlian, and F. Wiesböck. 2016. “Options for Formulating a Digital Transformation Strategy.” MIS Quarterly Executive 15 (2): 123–139.
- Hofstede, G. H., G. J. Hofstede, and M. Minkov. 2010. Cultures and Organizations: Software of the Mind: Intercultural Cooperation and its Importance for Survival. New York, London: McGraw-Hill.
- Hooper, D., J. Coughlan, and M. Mullen. 2008. “Structural Equation Modelling: Guidelines for Determining Model Fit.” Electronic Journal of Business Research Methods 6 (1): 53–60. doi:https://doi.org/10.21427/D7CF7R.
- Hu, L-t, and P. M. Bentler. 1999. “Cutoff Criteria for fit Indexes in Covariance Structure Analysis: Conventional Criteria Versus New Alternatives.” Structural Equation Modeling: A Multidisciplinary Journal 6 (1): 1–55. doi:https://doi.org/10.1080/10705519909540118.
- Igbaria, M., and J. Iivari. 1995. “The Effects of Self-Efficacy on Computer Usage.” Omega 23 (6): 587–605. doi:https://doi.org/10.1016/0305-0483(95)00035-6.
- Isaksen, S. G., and G. Ekvall. 2010. “Managing for Innovation: The Two Faces of Tension in Creative Climates.” Creativity and Innovation Management 19 (2): 73–88. doi:https://doi.org/10.1111/j.1467-8691.2010.00558.x.
- James, L. A., and L. R. James. 1989. “Integrating Work Environment Perceptions: Explorations Into the Measurement of Meaning.” Journal of Applied Psychology 74 (5): 739–751. doi:https://doi.org/10.1037/0021-9010.74.5.739.
- Janssen, O. 2004. “How Fairness Perceptions Make Innovative Behavior More or Less Stressful.” Journal of Organizational Behavior 25 (2): 201–215. doi:https://doi.org/10.1002/job.238.
- Jiang, J. J., G. Klein, and C. L. Carr. 2002. “Measuring Information System Service Quality: SERVQUAL From the Other Side.” MIS Quarterly 26 (2): 145. doi:https://doi.org/10.2307/4132324.
- Johnson, J. J., and C. L. McIntye. 1998. “Organizational Culture and Climate Correlates of Job Satisfaction.” Psychological Reports 82 (3): 843–850. doi:https://doi.org/10.2466/pr0.1998.82.3.843.
- Joshi, K., and A. Rai. 2000. “Impact of the Quality of Information Products on Information System Users’ Job Satisfaction: An Empirical Investigation.” Information Systems Journal 10 (4): 323–345. doi:https://doi.org/10.1046/j.1365-2575.2000.00087.x.
- Kaiser, H. F., and J. Rice. 1974. “Little Jiffy, Mark Iv.” Educational and Psychological Measurement 34 (1): 111–117. doi:https://doi.org/10.1177/001316447403400115.
- Kalischko, T., T. Fischer, and R. Riedl. 2019. “Techno-Unreliability: A Pilot Study in the Field.” In Information Systems and Neuroscience: NeuroIS Retreat 2019, edited by F. D. Davis, R. Riedl, J. Vom Brocke, P.-M. Léger, and A. Randolph, 137–145. Berlin, Heidelberg: Springer.
- Khedhaouria, A., and A. Cucchi. 2019. “Technostress Creators, Personality Traits, and Job Burnout: A Fuzzy-set Configurational Analysis.” Journal of Business Research 101: 349–361. doi:https://doi.org/10.1016/j.jbusres.2019.04.029.
- Kim, J. S., and G. H. Chung. 2017. “Implementing Innovations Within Organizations: A Systematic Review and Research Agenda.” Innovation 19 (3): 372–399. doi:https://doi.org/10.1080/14479338.2017.1335943.
- King, E. B., K. Chermont, M. West, J. F. Dawson, and M. R. Hebl. 2007. “How Innovation can Alleviate Negative Consequences of Demanding Work Contexts: The Influence of Climate for Innovation on Organizational Outcomes.” Journal of Occupational and Organizational Psychology 80 (4): 631–645. doi:https://doi.org/10.1348/096317906X171145.
- Klein, K. J., A. B. Conn, and J. S. Sorra. 2001. “Implementing Computerized Technology: An Organizational Analysis.” Journal of Applied Psychology 86 (5): 811–824. doi:https://doi.org/10.1037//0021-9010.86.5.811.
- Klein, K. J., and J. S. Sorra. 1996. “The Challenge of Innovation Implementation.” Academy of Management Review 21 (4): 1055–1080. doi:https://doi.org/10.5465/AMR.1996.9704071863.
- Kline, R. B. 2011. Principles and Practice of Structural Equation Modeling. New York, London: Guilford.
- Korunka, C., A. Weiss, and B. Karetta. 1993. “Effects of New Technologies with Special Regard for the Implementation Process per se.” Journal of Organizational Behavior 14 (4): 331–348.
- Lazarus, R. S., and S. Folkman. 1984. Stress, Appraisal, and Coping. New York: Springer Pub. Co.
- Lee, C.-S., Y.-C. Chen, P.-L. Tsui, and T.-H. Yu. 2014. “Examining the Relations Between Open Innovation Climate and job Satisfaction with a PLS Path Model.” Quality & Quantity 48 (3): 1705–1722. doi:https://doi.org/10.1007/s11135-013-9869-6.
- Legner, C., T. Eymann, T. Hess, C. Matt, T. Böhmann, P. Drews, A. Mädche, N. Urbach, and F. Ahlemann. 2017. “Digitalization: Opportunity and Challenge for the Business and Information Systems Engineering Community.” Business & Information Systems Engineering 59 (4): 301–308. doi:https://doi.org/10.1007/s12599-017-0484-2.
- Liang, H., and Y. Xue. 2009. “Avoidance of Information Technology Threats: A Theoretical Perspective.” MIS Quarterly 33 (2): 71–90.
- Locke, E. A. 1976. “The Nature and Causes of Job Satisfaction.” In Handbook of Industrial and Organizational Psychology, edited by M. D. Dunnette, 1297–1343. Chicago: Rand McNally College Pub. Co.
- MacKenzie, S. B., P. M. Podsakoff, and N. P. Podsakoff. 2011. “Construct Measurement and Validation Procedures in MIS and Behavioral Research: Integrating New and Existing Techniques.” MIS Quarterly 35 (2): 293–334. doi:https://doi.org/10.2307/23044045.
- Maier, C., S. Laumer, C. Weinert, and T. Weitzel. 2015. “The Effects of Technostress and Switching Stress on Discontinued Use of Social Networking Services: A Study of Facebook Use.” Information Systems Journal 25 (3): 275–308.
- Malhotra, N. K., and S. Dash. 2011. Marketing Research: An Applied Orientation. New Delhi: Pearson.
- Matsunaga, M. 2010. “How to Factor-Analyze Your Data Right: Do’s, Don’ts, and how-To’s.” International Journal of Psychological Research 3 (1): 97–110. doi:https://doi.org/10.21500/20112084.854.
- Meade, A. W., and S. B. Craig. 2012. “Identifying Careless Responses in Survey Data.” Psychological Methods 17 (3): 437–455. doi:https://doi.org/10.1037/a0028085.
- Melville, N., K. Kraemer, and V. Gurbaxani. 2004. “Review: Information Technology and Organizational Performance: An Integrative Model of IT Business Value.” MIS Quarterly 28 (2): 283–322. doi:https://doi.org/10.2307/25148636.
- Meyer, A. N., L. E. Barton, G. C. Murphy, T. Zimmermann, and T. Fritz. 2017. “The Work Life of Developers: Activities, Switches and Perceived Productivity.” IEEE Transactions on Software Engineering 43 (12): 1178–1193. doi:https://doi.org/10.1109/TSE.2017.2656886.
- Morris, J., I. Becker, and S. Parkin. 2019. “In Control with no Control: Perceptions and Reality of Windows 10 Home Edition Update Features.” In Proceedings of Workshop on Usable Security (USEC), edited by M. F. Theofanos and Y. Acar, 1–18. Reston, VA: Internet Society.
- Nunnally, J. C., and I. H. Bernstein. 1994. Psychometric Theory. London: McGraw-Hill, New York.
- Nybakk, E., P. Crespell, and E. Hansen. 2011. “Climate for Innovation and Innovation Strategy as Drivers for Success in the Wood Industry: Moderation Effects of Firm Size, Industry Sector, and Country of Operation.” Silva Fennica 45 (3), doi:https://doi.org/10.14214/sf.110.
- O’brien, R. M. 2007. “A Caution Regarding Rules of Thumb for Variance Inflation Factors.” Quality & Quantity 41 (5): 673–690. doi:https://doi.org/10.1007/s11135-006-9018-6.
- Oreg, S. 2003. “Resistance to Change: Developing an Individual Differences Measure.” Journal of Applied Psychology 88 (4): 680–693. doi:https://doi.org/10.1037/0021-9010.88.4.680.
- Orlikowski, W. J., and S. R. Barley. 2001. “Technology and Institutions: What Can Research on Information Technology and Research on Organizations Learn from Each Other?” MIS Quarterly 25 (2): 145. doi:https://doi.org/10.2307/3250927.
- Patterson, M. G., M. A. West, V. J. Shackleton, J. F. Dawson, R. Lawthom, S. Maitlis, D. L. Robinson, and A. M. Wallace. 2005. “Validating the Organizational Climate Measure: Links to Managerial Practices, Productivity and Innovation.” Journal of Organizational Behavior 26 (4): 379–408. doi:https://doi.org/10.1002/job.312.
- Pavlou, Paul A., Huigang Liang, and Yajiong Xue. 2007. “Understanding and Mitigating Uncertainty in Online Exchange Relationships: A Principal-Agent Perspective.” MIS Quarterly 31 (1): 105–136. doi:https://doi.org/10.2307/25148783.
- Petter, S., W. DeLone, and E. McLean. 2008. “Measuring Information Systems Success: Models, Dimensions, Measures, and Interrelationships.” European Journal of Information Systems 17 (3): 236–263. doi:https://doi.org/10.1057/ejis.2008.15.
- Podsakoff, P. M., S. B. MacKenzie, J.-Y. Lee, and N. P. Podsakoff. 2003. “Common Method Biases in Behavioral Research: A Critical Review of the Literature and Recommended Remedies.” Journal of Applied Psychology 88 (5): 879–903. doi:https://doi.org/10.1037/0021-9010.88.5.879.
- Podsakoff, P. M., S. B. MacKenzie, and N. P. Podsakoff. 2012. “Sources of Method Bias in Social Science Research and Recommendations on how to Control it.” Annual Review of Psychology 63: 539–569. doi:https://doi.org/10.1146/annurev-psych-120710-100452.
- Pollard, T. M. 2001. “Changes in Mental Well-Being, Blood Pressure and Total Cholesterol Levels During Workplace Reorganization: The Impact of Uncertainty.” Work & Stress 15 (1): 14–28. doi:https://doi.org/10.1080/02678370110064609.
- Rafferty, A. E., and M. A. Griffin. 2006. “Perceptions of Organizational Change: A Stress and Coping Perspective.” The Journal of Applied Psychology 91 (5): 1154–1162. doi:https://doi.org/10.1037/0021-9010.91.5.1154.
- Ragu-Nathan, T. S., M. Tarafdar, B. S. Ragu-Nathan, and Q. Tu. 2008. “The Consequences of Technostress for End Users in Organizations: Conceptual Development and Empirical Validation.” Information Systems Research 19 (4): 417–433.
- Ramirez Heller, B., R. Berger, and F. C. Brodbeck. 2014. “Does an Adequate Team Climate for Learning Predict Team Effectiveness and Innovation Potential?: A Psychometric Validation of the Team Climate Questionnaire for Learning in an Organizational Context.” Procedia - Social and Behavioral Sciences 114: 543–550. doi:https://doi.org/10.1016/j.sbspro.2013.12.744.
- Rasulzada, F., and I. Dackert. 2009. “Organizational Creativity and Innovation in Relation to Psychological Well-Being and Organizational Factors.” Creativity Research Journal 21 (2-3): 191–198. doi:https://doi.org/10.1080/10400410902855283.
- Reio, T. G., and B. Shuck. 2015. “Exploratory Factor Analysis.” Advances in Developing Human Resources 17 (1): 12–25. doi:https://doi.org/10.1177/1523422314559804.
- Riedl, R. 2013. “On the Biology of Technostress: Literature Review and Research Agenda.” DATA BASE for Advances in Information Systems 44 (1): 18–55.
- Riedl, R., and T. Fischer. 2018. “System Response Time as a Stressor in a Digital World: Literature Review and Theoretical Model.” In Proceedings of the 5th International Conference on HCI in Business, Government and Organizations, edited by F. F.-H. Nah, and B. S. Xiao, 175–186. New York, NY: Springer Berlin Heidelberg.
- Riedl, R., H. Kindermann, A. Auinger, and A. Javor. 2012. “Technostress From a Neurobiological Perspective - System Breakdown Increases the Stress Hormone Cortisol in Computer Users.” Business & Information Systems Engineering 4 (2): 61–69.
- Riedl, R., H. Kindermann, A. Auinger, and A. Javor. 2013. “Computer Breakdown as a Stress Factor During Task Completion Under Time Pressure: Identifying Gender Differences Based on Skin Conductance.” Advances in Human-Computer Interaction 2013 (1): 420169. doi:https://doi.org/10.1155/2013/420169.
- Rizzo, J. R., R. J. House, and S. I. Lirtzman. 1970. “Role Conflict and Ambiguity in Complex Organizations.” Administrative Science Quarterly 15 (2): 150–163.
- Sambamurthy, Vallabh, Anandhi Bharadwaj, and Varun Grover. 2003. “Shaping Agility Through Digital Options: Reconceptualizing the Role of Information Technology in Contemporary Firms.” MIS Quarterly 27 (2): 237–263. doi:https://doi.org/10.2307/30036530.
- Sarabadani, J., M. Carter, and D. R. Compeau. 2018. 10 Years of Research on Technostress Creators and Inhibitors: Synthesis and Critique. In: AIS (ed) Proceedings of AMCIS 2018.
- Schneider, B. 2000. “The Psychological Life of Organizations.” In Handbook of Organizational Culture & Climate, edited by N. M. Ashkanasy, C. Wilderom, and M. F. Peterson, xvii–xxii. Thousand Oaks, CA, London: SAGE Publications.
- Seo, D., and A. I. La Paz. 2008. “ Exploring the Dark Side of IS in Achieving Organizational Agility.” Communications of the ACM 51 (11): 136–139. doi:https://doi.org/10.1145/1400214.1400242.
- Sethi, V., R. C. King, and J. C. Quick. 2004. “What Causes Stress in Information System Professionals?” Communications of the ACM 47 (3): 99–102. doi:https://doi.org/10.1145/971617.971623.
- Shane, S. 1995. “Uncertainty Avoidance and the Preference for Innovation Championing Roles.” Journal of International Business Studies 26 (1): 47–68. doi:https://doi.org/10.1057/palgrave.jibs.8490165.
- Shanker, R., R. Bhanugopan, B. I. J. M. van der Heijden, and M. Farrell. 2017. “Organizational Climate for Innovation and Organizational Performance: The Mediating Effect of Innovative Work Behavior.” Journal of Vocational Behavior 100: 67–77. doi:https://doi.org/10.1016/j.jvb.2017.02.004.
- Shih, H-A, and E. Susanto. 2011. “Is Innovative Behavior Really Good for the Firm?” International Journal of Conflict Management 22 (2): 111–130. doi:https://doi.org/10.1108/10444061111126666.
- Shu, Q., Q. Tu, and K. Wang. 2011. “The Impact of Computer Self-Efficacy and Technology Dependence on Computer-Related Technostress: A Social Cognitive Theory Perspective.” International Journal of Human-Computer Interaction 27 (10): 923–939.
- Smith, M. J., and P. Carayon. 1995. “New Technology, Automation, and Work Organization: Stress Problems and Improved Technology Implementation Strategies.” International Journal of Human Factors in Manufacturing 5 (1): 99–116. doi:https://doi.org/10.1002/hfm.4530050107.
- Sonnentag, S., and M. Frese. 2013. “Stress in Organizations.” In Handbook of Psychology. Industrial and Organizational Psychology, edited by I. B. Weiner, and N. W. Schmitt, 560–592. Hoboken, NJ, USA: John Wiley & Sons, Inc; Wiley.
- Spector, P. E., and S. M. Jex. 1998. “Development of Four Self-Report Measures of Job Stressors and Strain: Interpersonal Conflict at Work Scale, Organizational Constraints Scale, Quantitative Workload Inventory, and Physical Symptoms Inventory.” Journal of Occupational Health Psychology 3 (4): 356–367.
- Tams, S., J. B. Thatcher, and V. Grover. 2018. “Concentration, Competence, Confidence, and Capture: An Experimental Study of Age, Interruption-Based Technostress, and Task Performance.” Journal of the Association for Information Systems 19 (9): 857–908. doi:https://doi.org/10.17705/1jais.00511.
- Tarafdar, M., C. L. Cooper, and J.-F. Stich. 2019. “The Technostress Trifecta - Techno Eustress, Techno Distress and Design: Theoretical Directions and an Agenda for Research.” Information Systems Journal 29 (1): 6–42. doi:https://doi.org/10.1111/isj.12169.
- Tarafdar, M., E. B. Pullins, and T. S. Ragu-Nathan. 2015b. “Technostress: Negative Effect on Performance and Possible Mitigations.” Information Systems Journal 25 (2): 103–132.
- Tarafdar, M., Q. Tu, and T. S. Ragu-Nathan. 2010. “Impact of Technostress on End-User Satisfaction and Performance.” Journal of Management Information Systems 27 (3): 303–334.
- Tarafdar, M., Q. Tu, T. S. Ragu-Nathan, and B. S. Ragu-Nathan. 2011. “Crossing to the Dark Side: Examining Creators, Outcomes, and Inhibitors of Technostress.” Communications of the ACM 54 (9): 113–120.
- Treiblmaier, H., and P. Filzmoser. 2010. “Exploratory Factor Analysis Revisited: How Robust Methods Support the Detection of Hidden Multivariate Data Structures in IS Research.” Information & Management 47 (4): 197–207. doi:https://doi.org/10.1016/j.im.2010.02.002.
- Turel, O. 2015. “Quitting the use of a Habituated Hedonic Information System: A Theoretical Model and Empirical Examination of Facebook Users.” European Journal of Information Systems 24 (4): 431–446. doi:https://doi.org/10.1057/ejis.2014.19.
- Turel, O. 2016. “Untangling the Complex Role of Guilt in Rational Decisions to Discontinue the use of a Hedonic Information System.” European Journal of Information Systems 25 (5): 432–447. doi:https://doi.org/10.1057/s41303-016-0002-5.
- Turel, Ofir, Alexander Serenko, and Paul Giles. 2011. “Integrating Technology Addiction and Use: An Empirical Investigation of Online Auction Users.” MIS Quarterly 35 (4): 1043–1061. doi:https://doi.org/10.2307/41409972.
- Vaniea, K., and Y. Rashidi. 2016. “Tales of Software Updates: The Process of Updating Software.” In Proceedings of the 34th Annual CHI Conference on Human Factors in Computing Systems, edited by J. Kaye, A. Druin, C. Lampe, D. Morris, and J. P. Hourcade, 3215–3226. New York, NY: The Association for Computing Machinery.
- Wang, K., and Q. Shu. 2008. “The Moderating Impact of Perceived Organizational Support on the Relationship Between Technostress and Role Stress.” In Proceedings of the 19th International Conference on Database and Expert Systems Applications (DEXA), edited by E. Ferro, E. Paolucci, and M. Cantamessa, 420–424. Turin.
- Wang, K., Q. Shu, and Q. Tu. 2008. “Technostress Under Different Organizational Environments: An Empirical Investigation.” Computers in Human Behavior 24 (6): 3002–3013.
- Wastell, D., and M. Newman. 1993. “The Behavioral Dynamics of Information System Development: A Stress Perspective.” Accounting, Management and Information Technologies 3 (2): 121–148. doi:https://doi.org/10.1016/0959-8022(93)90003-O.
- Watts, S., and J. C. Henderson. 2006. “Innovative IT Climates: CIO Perspectives.” The Journal of Strategic Information Systems 15 (2): 125–151. doi:https://doi.org/10.1016/j.jsis.2005.08.001.
- Wheaton, B., B. Muthen, D. F. Alwin, and G. F. Summers. 1977. “Assessing Reliability and Stability in Panel Models.” Sociological Methodology 8: 84–136. doi:https://doi.org/10.2307/270754.
- Wolfe, S. 2018. Amazon’s One Hour of Downtime on Prime Day May have Cost it up to $100 million in Lost Sales. https://www.businessinsider.de/amazon-prime-day-website-issues-cost-it-millions-in-lost-sales-2018-7?r=US&IR=T.
- Wood, R., and A. Bandura. 1989. “Social Cognitive Theory of Organizational Management.” Academy of Management Review 14 (3): 361–384. doi:https://doi.org/10.2307/258173.
- Zhao, X., Q. Xia, and W. Huang. 2020. “Impact of Technostress on Productivity from the Theoretical Perspective of Appraisal and Coping Processes.” Information & Management 103265. doi:https://doi.org/10.1016/j.im.2020.103265.
Appendices
Appendix 1
We drew a selection of studies from the review of previous research on digital stress by Fischer and Riedl (Citation2017) that have focused on situational characteristics related to an organisational climate of innovation (e.g. pace of change or technical support). This selection, as listed in , illustrates that situational characteristics were either included as a beneficial force of the organisational environment (i.e. reducing distress appraisal and/or its negative effects/outcomes) or as a harmful force of the organisational environment (i.e. a source of distress). Only one of these studies considered both options (i.e. Wang, Shu, and Tu Citation2008), but focused mainly on the harmful effects of a culture of innovation (e.g. by increasing competitiveness amongst members of the organisation, as argued by the authors).
Table A1. Illustrative studies on digital stress that investigated the effect of situational characteristics related to technological change and/or organisational support.
Appendix 2
In , we list the instruments that were used to operationalise the constructs in the online survey that was part of this study and the sources that these instruments were drawn from. For each construct, we list the items and their respective loadings on the construct as well as the coefficient alpha (Cronbach and Meehl Citation1955) for the construct. In addition, in , we highlight the descriptive statistics for each indicator in both sub-samples, including its mean, standard deviation, skewness and kurtosis.
Table A2. Overview of constructs and items in the online survey.
Table A3. Descriptive statistics for indicators in both sub-samples.
Appendix 3
In , we present the results of the initial EFA, which involved all items that were part of latent variables in the survey. The KMO for this initial EFA was .897 and the cumulative explained variance for the six extracted factors was 58.955%. For each factor, we also list its individual contribution to the overall explained variance and its Eigenvalue. For each item, the loadings on the six factors that were extracted are included and the highest loading is highlighted with a grey background. In this initial EFA, there are some major issues with convergent validity (i.e. items only loading weakly on their intended construct) and discriminant validity (i.e. items with substantial cross-loadings).
In , we present the results of the final EFA, which a reduced number items that were part of latent variables in the survey. The KMO for this final EFA was .881 and the cumulative explained variance for the six extracted factors was 64.201%. For each factor, we also list its individual contribution to the overall explained variance and its Eigenvalue. For each item, the loadings on the six factors that were extracted are included and the highest loading is highlighted with a grey background. In this final EFA, all items loading strongly on their intended factor and there are no substantial cross-loadings.
Table A4. Results of initial EFA.
Appendix 4
In , we present the main results of the reliability and validity assessment for the constructs in our survey, including their composite reliability, average variance extracted, and the test for the Fornell-Larcker criterion as an indicator for the discriminant validity of our constructs on the right-hand side of the table. As an additional means to demonstrate discriminant validity, we also report the HTMT for each construct pair in Tables A6 and A7.