ABSTRACT
Systems designed with persuasive software features can influence users to adopt attitudes and/or behaviours that contribute to better health outcomes. Studies on Coronary Heart Disease (CHD) interventions have shown how they can be used to support lifestyle changes. Meta-analytic reviews of randomised controlled trials (RCTs) are needed to appraise, summarise, and show their effects on health. To this end, we investigated the effectiveness of digital health interventions (DHI) designed for CHD and examined the impact of persuasive features in the DHIs. We performed a systematic search using three academic databases for scientific papers on CHD and digital interventions from 2010 to 2020, yielding 1556 papers. The systematic review and meta-analysis included 12 RCTs after screening. Our findings show that digital health interventions had an impact on diastolic blood pressure, systolic blood pressure, low-density lipoprotein, high-density lipoprotein, total cholesterol, glycated haemoglobin, glucose, triglyceride, heart rate, and depression but not body mass index. We present effective interventions and insights into persuasive software features that were associated with effective DHIs. More research is needed to investigate the effectiveness of persuasive features in other contexts and other factors that can impact the effectiveness of DHIs.
1. Introduction
Websites, mobile apps, and wearables, among other information systems, can be used to persuade users in multiple ways. They may influence not only our daily routines as we use and interact with them but also our health. Such systems are designed with psychological and behavioural outcomes to form, shape, or reinforce attitudes and behaviours or simply comply without coercing or deceiving a user (Oinas-Kukkonen Citation2013).
Several studies (e.g. Kitsiou et al. Citation2017; Neubeck et al. Citation2016) have explored and demonstrated the potential use of technological interventions in healthcare particularly to support people to change risky behaviours and their negative consequences on health, manage existing health conditions, and support the delivery of healthcare.
In this research, the effectiveness of digital health interventions (DHIs) for Coronary Heart Disease (CHD), a cardiovascular disease that affects the heart and arteries (Bhatia Citation2010), will be investigated. We will also explore potential factors that may influence their effectiveness. CHD is associated with high morbidity and death and can be caused by genetic factors (e.g. family history, race, ethnicity) and lifestyle choices (e.g. unhealthy eating habits, sedentary behaviour, sleeping habits) (Poulter Citation1999). It has been found that lifestyle choices can impact clinical outcomes such as blood pressure, cholesterol, and body mass index (Mittal Citation2005).
DHIs can aid the modification of lifestyle towards better health (Spanakis et al. Citation2016). However, questions remain about their impact and effectiveness on clinical and behavioural outcomes in different problem domains. Prior research investigated the relationship between the number of persuasive features and the effectiveness of web-based mental health interventions (Wildeboer, Kelders, and van Gemert-Pijnen Citation2016) to assess the mechanisms at play in their success or failure (Stevenson et al. Citation2019). Evaluating interventions helps to understand how interventions and their features were designed to be effective (Wildeboer, Kelders, and van Gemert-Pijnen Citation2016; Karppinen et al. Citation2016). This can inform the design of new systems in the future.
1.1. Persuasive systems design
The Persuasive Systems Design (PSD) model is a three-step process for designing, developing, and evaluating persuasive systems (Oinas-Kukkonen and Harjumaa Citation2009). The first step is to comprehend the fundamental issues and assumptions underlying such systems known as postulates. The second step, analysis of the persuasion context, involves analysing and describing the intention behind a persuasive system, determining the type of change and outcomes, and analysing the problem domain, user, and technology to be used. It also entails deciding what message to present to the user and how to deliver it to the user. An examination of the context allows for a thorough understanding of what occurs in persuasion via technology. The last step involves choosing persuasive software features that can be used to support the user relating to the primary reason for the usage of the system, supporting user-to-system interaction, increasing the credibility of the persuasive system, and motivating users through social influence. The PSD model has been used for conducting systematic literature reviews to evaluate digital health interventions (Alhasani et al. Citation2020; Radomski et al. Citation2019; Portz et al. Citation2016).
1.2. Aim of study
This study aims to evaluate the effectiveness of digital health interventions designed to manage CHD as well as some factors that may lead to their effectiveness. One such factor is the use of persuasive features to support behaviour change. Currently, little is known about the relationship between the use of persuasive software features in digital health interventions and the efficacy of the intervention for this medical condition. While there are several literature reviews on digital interventions for CHD, this meta-analysis highlights the effectiveness of persuasive features in digital health interventions by examining the clinical outcomes in randomised controlled trials (RCTs). This will provide a good empirical basis for the selection of software features in similar interventions. The following questions will guide this research
How effective are digital health interventions for CHD outcomes?
What persuasive software features are associated with effective digital health interventions for CHD?
What are the most effective persuasive features?
2. Methods
A systematic literature search was conducted from 2010 to 2020. Papers were retrieved from Scopus (n = 558), Web of Science (n = 609), and Medline (n = 539) academic databases using these keywords thus (“mobile” OR “smart phone” OR “smartphone” OR “web” OR “internet)” AND (“coronary artery disease” OR “coronary heart disease” OR “ischemic heart disease)” AND “prevention.” A total of 1706 articles were retrieved. After deduplication, 1556 papers were left. The next step was screening the titles of the papers. 150 papers remained. The abstracts of these papers were screened, and irrelevant papers were excluded leaving 28 papers. A total of 12 RCTs remained after mere protocol descriptions were removed. For the data extraction and meta-analysis, 12 RCTs (out of 28) were included (Chow et al. Citation2015; Islam et al. Citation2019; Yudi et al. Citation2021; Maddison et al. Citation2019; Dale et al. Citation2015; Devi, Powell, and Singh Citation2014; Dorje et al. Citation2019; Frederix et al. Citation2015; Zheng et al. Citation2019; Huo et al. Citation2019; Snoek et al. Citation2020; Santo et al. Citation2019). See .
Figure 1. Prisma flowchart for article selection (Page et al. Citation2021).
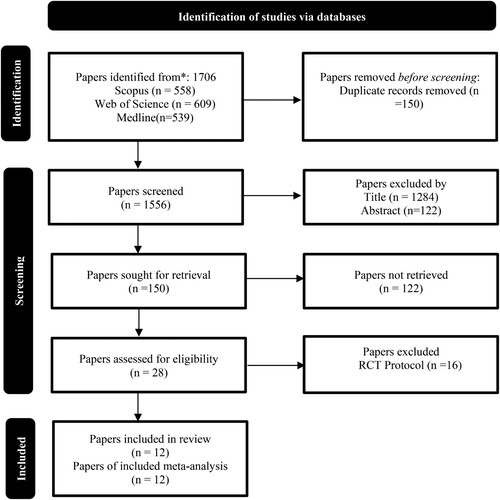
2.1. Coding of intervention features
Intervention features were identified using the PSD model (Oinas-Kukkonen and Harjumaa Citation2009). They ended up being coded under the headings “self-monitoring”, “personalization”, “tailoring”, “reduction”, “reminders”, “praise”, “reward”, “social role”, “suggestion”, “verifiability”, “authority”, and “expertise” using textual descriptions in the RCT studies. The presence or absence of these features was evaluated for each intervention.
2.2. Statistical analysis
The mean and standard deviation (SD) of changes in 11 clinical outcomes were used for the meta-analysis. The meta-analysis was conducted to pool the effect size of the intervention using the random effect model. The random effects model takes the variations between-study into account when calculating the effect size. Between-study heterogeneity was assessed using Tau2, H2, and I2. Then subgroup analysis and meta-regression were used to find out possible sources of heterogeneity. To assess the presence of publication bias, ‘Begg's funnel plot’ and Egger's test were used. Statistical analyses were conducted using SPSS 28.0.1.0 (142). Subgroup analyses were used to examine the association between persuasive systems design principles and the effect sizes pooled by the interventions. Effect sizes (measured by Cohen's d) (Cohen Citation1998) were classified as very small (0.01–0.19), small (0.20–0.49), and medium (0.50–0.79) based on the boundaries defined by (Sawilowsky Citation2009).
3. Results
3.1. Data inclusion and exclusion
A study was included if it was an RCT study published in the English language. To conduct the meta-analysis, at least three values for each clinical outcome were required as such all clinical outcomes that did not have enough data to meet this criterion were excluded.
3.2. Data extraction
Data extracted include the outcome of the studies and persuasive software features. The extraction of persuasive features from the RCT studies was done by the first author who read the articles, identified the description of the intervention, and coded them in excel spreadsheets. Rigorous interpretation of the data was done by the first and third authors who discussed and resolved all conflicts that emerged by revisiting articles when necessary. The extraction of clinical outcomes was done by the first author and two other researchers. The second author contributed to the statistical analysis and interpretation of the data.
3.3. Study quality
The risk of bias is presented in . The risk of bias was accessed using the Cochrane Handbook for Systematic Reviews (Higgins et al. Citation2011). In general, all studies had a low or high risk of bias. All studies (11 out of 12) (Chow et al. Citation2015; Islam et al. Citation2019; Yudi et al. Citation2021; Dale et al. Citation2015; Devi, Powell, and Singh Citation2014; Dorje et al. Citation2019; Frederix et al. Citation2015; Zheng et al. Citation2019; Huo et al. Citation2019; Snoek et al. Citation2020; Santo et al. Citation2019) except (Frederix et al. Citation2015) showed evidence of a low risk of bias arising from the randomisation process.
Table 1. Risk of bias in the studies analysed.
Measures were taken by all the studies to conceal allocation to prevent selection bias except for (Yudi et al. Citation2021; Frederix et al. Citation2015; Zheng et al. Citation2019; Huo et al. Citation2019) who did not mention the measures taken to prevent this risk of bias. Eight studies took adequate measures to reduce the risk that could arise from unblinding personnel in the RCT trial (Chow et al. Citation2015; Islam et al. Citation2019; Yudi et al. Citation2021; Dale et al. Citation2015; Dorje et al. Citation2019; Zheng et al. Citation2019; Huo et al. Citation2019; Snoek et al. Citation2020). Three studies (Yudi et al. Citation2021; Frederix et al. Citation2015; Santo et al. Citation2019) did not mention how research personnel was blinded. Six studies could not blind the participants possibly due to the nature of the trial and hence there is a high risk of bias from knowledge of the type of treatment received (Yudi et al. Citation2021; Maddison et al. Citation2019; Dale et al. Citation2015; Devi, Powell, and Singh Citation2014; Zheng et al. Citation2019; Huo et al. Citation2019). Two studies (Dale et al. Citation2015; Devi, Powell, and Singh Citation2014) did not blind the outcome assessors. The risk of bias associated with incomplete outcome data and selective reporting was low for all the studies.
3.4. Characteristics of participants
A total of 3998 participants (i.e. 3324 males and 680 females) were involved in the RCTs analysed with a mean age of 65.66. Out of the 3998 participants, 2127 people were exposed to digital health interventions. The mean age was 60.62.
3.5. Characteristics of interventions
The interventions were offered to users for a minimum of 6 weeks to a maximum of 24 months as shown in . Technologies such as mobile/smartphone (Chow et al. Citation2015; Maddison et al. Citation2019; Dale et al. Citation2015; Dorje et al. Citation2019; Zheng et al. Citation2019; Huo et al. Citation2019; Santo et al. Citation2019), website (Dale et al. Citation2015; Snoek et al. Citation2020; Maddison et al. Citation2015), text messages (Chow et al. Citation2015; Frederix et al. Citation2015; Zheng et al. Citation2019), email (Frederix et al. Citation2015), mobile apps (Dorje et al. Citation2019; Santo et al. Citation2019), heart rate and pressure monitor (Snoek et al. Citation2020), motion sensor technologies (Dale et al. Citation2015; Frederix et al. Citation2015) and wearable chest sensors (Maddison et al. Citation2019) were used to deliver the interventions. Varying modes of interaction or frequency were supported. While some interventions sent messages to users on regular basis and thus initiated regular interaction, others provided a means for users to interact with the system via monitoring tools. For example, interventions can easily facilitate regular interaction with the user.
Table 2. Clinical outcomes and persuasive software features.
3.6. Persuasive features in the interventions
A total of 12 persuasive features were identified from the primary task support, dialogue support, and credibility support categories. Personalisation (n = 12) was the most used persuasive feature followed by self-monitoring (n = 9), praise (n = 6), reminders (n = 5),
authority (n = 5), expertise (n = 4), tailoring (n = 2), reduction (n = 1), suggestion (n = 1), reward (n = 1), social role (n = 1), and verifiability (n = 1) in the interventions analysed. See for clinical outcomes per RCT study and the persuasive features identified in the descriptions of the intervention.
Authority (n = 5), expertise (n = 4), tailoring (n = 2), reduction (n = 1), suggestion (n = 1), reward (n = 1), social role (n = 1), and verifiability (n = 1) in the interventions analysed. See for clinical outcomes per RCT study and the persuasive features identified in the descriptions of the intervention.
3.7. Types of outcomes
Different studies reported a variety of outcomes including glycosylated haemoglobin (HbA1c), systolic and diastolic blood pressure, body mass index, low, high, and total cholesterol, medication adherence, sedentary behaviour, and smoking. However, in this paper 11 clinical outcomes (i.e. namely body mass index, diastolic blood pressure, systolic blood pressure, low-density lipoprotein, high-density lipoprotein, total cholesterol, glycated haemoglobin, glucose, triglyceride, heart rate, and depression), were evaluated because there were at least three observations in each which was enough to conduct a meta-analysis.
3.8. Effectiveness of interventions on clinical outcomes
In analysing the data, we observed that clinical outcomes were measured at different time intervals in weeks. Such instances are treated as subgroups in the analysis. A total of 11 clinical outcomes were analysed. There is evidence to show that some digital health interventions affected clinical outcomes including body mass index (BMI), diastolic blood pressure (DBP), systolic blood pressure (SBP), low-density lipoprotein (LDL), high-density lipoprotein (HDL), Total Cholesterol, Glycated haemoglobin (HbA1c), glucose, triglycerides, Heart rate, and depression measured by Patient Health Questionnaire-9 (PHQ-9) as shown in . This implies that digital health interventions did improve some clinical outcomes compared with usual care.
Figure 2. (a) BMI; (b) DBP; (c) SBP; (d) LDL.
Figure 3. (a) HDL; (b) total cholesterol; (c) HBA1c; (d) glucose.
Figure 4. (a) Triglycerides; (b) heart rate; (c) PHQ-9.
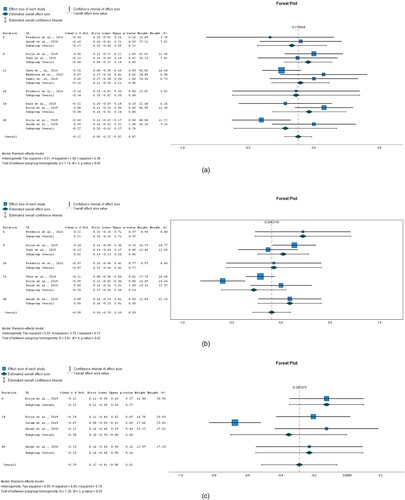
The impact of interventions on BMI was explored in 11 studies as shown in (a). The data were obtained from eight RCT papers. There were differences in BMI between the intervention and control groups. The overall effect size of 0.033 (95% CI −0.141 to 0.206) for BMI showed that the intervention group was not favoured and hence the interventions analysed did not lead to a reduction in BMI. The heterogeneity measured by I2 was 83.6%.
In terms of DBP, the overall effect of eight interventions was −0.115 (95% CI −0.217 to −0.014) and it was statistically significant (P = 0.026). See (b). This implies that the intervention favoured its users. Heterogeneity measured by I2 was 13.7%.
There was a difference in SBP between the intervention and control groups. See (c). The overall effect size pooled by 11 interventions was −0.044 (95% CI −0.197 to 0.109) but it wasn't statistically significant (P = 0.574). Heterogeneity measured by I2 was 80.2%.
Ten interventions analysed were successful in significantly (P = 0.022) reducing LDL. The overall effect size for LDL was −0.094 (95% CI −0.174 to −0.014). See (d). Heterogeneity measured by I2 was 30.5%.
Overall, there was an insignificant (P = 0.671) difference in HDL between the intervention and control groups was −0.017 (95% CI −0.095 to 0.061) for seven interventions. See (a). Heterogeneity measured by I2 was 0%.
For total cholesterol, the overall effect size pooled by eight interventions was −0.119 (95%CI −0.222 to −0.016) and it was statistically significant (P = 0.024). See (b). Heterogeneity measured by I2 was 37.6%.
The overall effect size pooled by four interventions for HBA1c was −0.144 (95%CI −0.3556 to 0.068). The observed difference between the intervention and control group is not significant (P = 0.184) See (c). Heterogeneity, as measured by I2 was 64%.
There was a statistically significant (P<0.000) difference in Glucose between the intervention and control groups −0.307 (95% CI −0.461 to −0.153) for three interventions See (d). Heterogeneity, as measured by I2 was 6.6%.
The overall effect size for Triglycerides was −0.12 (95% CI −0.222 to −0.002). There was a statistically significant (P = 0.020) difference between the intervention and control groups in the five interventions analysed. See (a). Heterogeneity, as measured by I2 was 20.4%.
The overall effect size for Heart rate was −0.094 (95% CI −0.284 to 0.096) for five interventions but it was found to be insignificant (P = 0.331). See (b). Heterogeneity, as measured by I2 was 73.4%.
A small but significant (P = 0.012) effect size was pooled by three interventions for PHQ-9 −0.288 (95% CI −0.514 to −0.062). See (c). Heterogeneity measured by I2 was 78.50%.
The funnel plot (See and ) shows the potential presence of publication bias in all outcomes because the effect sizes lie asymmetrically around the pooled effect size (represented by the stripped line in the funnel). To confirm the asymmetry, Egger's test (p < 0.05) was conducted for all clinical outcomes. There was significant asymmetry for BMI (P = 0.008), DBP (P = 0.008), and SBP (P = 0.047). No significant asymmetry was detected for the remaining clinical outcomes.
3.9. The impact of persuasive software features in digital health interventions on clinical outcomes
In this section, we present insights into how persuasive software features influenced the effectiveness of the intervention.
In , we present the results of the subgroup analysis of the presence or absence of persuasive features. There is a statistically significant difference between interventions that employed persuasive features and those that did not. The presence of tailoring −0.296 (−0.430 to −0.162), expertise −0.229 (−0.340 to −0.119), reminders −0.164 (−0.239 to −0.089), and authority −0.112 (−0.214 to −0.011) is associated with a weak but significant effect size. Interestingly, the absence of self-monitoring is associated with a small but significant effect size of −0.274 (−0.399 to −0.149).
Table 3. Subgroup analysis to investigate the presence and absence of persuasive software features using 11 clinical outcomes.
There is a limitation when both the presence and absence of the variables under investigation are considered. This is because weight is given to the absence of these features and hence does reveal the true impact of the persuasive features. As such, further analyses were conducted to assess the true effect of the presence of persuasive features. See . We found that all persuasive features except reduction 0.102 (−0.205 to 0.409), and reward 0.102 (−0.205 to 0.409) were not significantly associated with the effectiveness of the interventions. The data used to conduct this level of analysis contained both effective and ineffective intervention outcomes. shows the true effect of persuasive features in the interventions. Tailoring, social role, verifiability, reminders, expertise, authority, personalisation, self-monitoring, and praise are significantly associated with effective intervention outcomes except for suggestions which were also found to be nonsignificant in ineffective interventions. As such it did not explain any variance in the effect size it pooled. We also found that similar features were utilised in both effective and ineffective interventions except tailoring (see ) was found in two effective interventions. Interestingly, the persuasive features that are associated with the effectiveness outcomes explained at least 30% of the observed variance (i.e. I2>30%) except praise and suggestions. On the other hand, persuasive features associated with ineffective outcomes did not explain any variance in the effect size pooled.
Table 4. Effect size pooled by the presence of persuasive software features extracted clinical outcomes data.
Table 5. Impact of persuasive features appearing from effective and ineffective intervention outcomes.
Table 6. Cumulative effect size of effective and ineffective interventions.
To investigate further the interventions with effective outcomes, ineffective outcomes were excluded from further analysis. shows the effective outcomes in each intervention, the effect size pooled, and the characteristics of the intervention which describes the technological medium through which persuasive features were implemented to support behaviour change, the duration of use, and the frequency of interaction. to examine possible differences among effective outcomes. Such intervention characteristics could affect the effectiveness of persuasive features. For example, how reminders were delivered via technologies such as SMS, email, or personalised (e.g. frequency and timing) may influence the effectiveness of the reminders (Chow et al. Citation2015). Also, interventions that employ self-monitoring using a sensor such as pedometers imply that the user interacts frequently with the system automatically or consciously. The intervention by (Devi, Powell, and Singh Citation2014) used only a website but was excluded from because it was ineffective. Presyasuve features were implemented in other interventions using websites with other technologies (Dale et al. Citation2015; Snoek et al. Citation2020). As such, the actual implementation of persuasive software features in the intervention can influence its effectiveness and hence matters.
3.10. Cumulative effectiveness of interventions
Further analysis identified effective interventions from ineffective interventions. This was achieved by summing up the effect sizes of all clinical outcomes identified in each of the interventions analysed. See . Cumulatively, ten interventions (Chow et al. Citation2015; Islam et al. Citation2019; Yudi et al. Citation2021; Dale et al. Citation2015; Dorje et al. Citation2019; Frederix et al. Citation2015; Zheng et al. Citation2019; Huo et al. Citation2019; Snoek et al. Citation2020; Santo et al. Citation2019) were effective while two interventions (Maddison et al. Citation2019; Devi, Powell, and Singh Citation2014) were not. These interventions led to successful or unsuccessful in all or some clinical outcomes. Three interventions (Chow et al. Citation2015; Islam et al. Citation2019; Zheng et al. Citation2019) led to successful outcomes while nine interventions (Yudi et al. Citation2021; Maddison et al. Citation2019; Dale et al. Citation2015; Dorje et al. Citation2019; Frederix et al. Citation2015; Huo et al. Citation2019; Snoek et al. Citation2020; Santo et al. Citation2019) had a mixture of successful and unsuccessful clinical outcomes. Only one intervention did not influence the clinical outcomes analysed (Devi, Powell, and Singh Citation2014).
4. Discussion
4.1. Summary of main findings
A meta-analysis was conducted to investigate the efficacy of digital health interventions designed to manage coronary health diseases. Together, 12 RCTs were analysed. The digital health interventions analysed used different modes of technology including smartphones, websites, wearables, and email.
Glycosylated haemoglobin (HbA1c), blood pressure (i.e. systolic, and diastolic), body mass index, and cholesterol (e.g. low, high, and total cholesterol) were among the clinical outcomes evaluated. Overall, we found out that the interventions led to improvements in all clinical outcomes except body mass index. The improvements in total cholesterol and triglyceride are consistent with the findings of (Ding, Zhang, and Hu Citation2022). In their study, the interventions analysed led to a reduction in body mass index, but the observed effect was not statistically significant. Another study found improvements in diastolic blood pressure (Sua et al. Citation2020).
Cumulatively, ten of the interventions (Chow et al. Citation2015; Islam et al. Citation2019; Yudi et al. Citation2021; Dale et al. Citation2015; Dorje et al. Citation2019; Frederix et al. Citation2015; Zheng et al. Citation2019; Huo et al. Citation2019; Snoek et al. Citation2020; Santo et al. Citation2019) were found to be effective while two interventions (Maddison et al. Citation2019; Devi, Powell, and Singh Citation2014) were not. These successful interventions can be viewed as good examples to help design effective interventions. Also, we can learn the interventions that were not unsuccessful.
The most used persuasive features were personalisation, self-monitoring from the primary task support category, praise, reminders from the dialogue support category, and authority, and expertise from the credibility support category. While preliminary, this finding, suggests that software features that can support the user to engage in the desired behaviour, interact with the intervention, and demonstrate the credibility of a system are needed in digital health interventions. Future research work on the impact of persuasive features and their categories on DHIs is recommended.
Subgroup analysis of the presence or absence of these persuasive features shows that there is a difference between interventions that employed features and those that did not. The presence of tailoring, expertise, reminders, and authority is associated with a small but significant effect size.
This type of analysis did not reveal the actual impact of software features that are present because it also considers absent software features. To this end, further investigations were conducted to understand the impact of the presence of persuasive features. Surprisingly, we found that reduction and reward were not significantly associated with the effectiveness of the interventions.
Analysis of the differences between successful and unsuccessful outcomes revealed that tailoring was the only persuasive feature present in only effective interventions (two interventions applied it) but in none of the ineffective interventions. Tailoring interventions to meet the needs of a user group can increase engagement (König et al. Citation2021) and continuous use (Chan, Kow, and Cheng Citation2017) and subsequent improvement in health outcomes. Rewards and reduction were found only in one unsuccessful intervention. Even if the only intervention applied these features, it was deemed ineffective because of the unsuccessful clinical outcomes regarding systolic and diastolic blood pressure (Devi, Powell, and Singh Citation2014), the finding should not be interpreted so that reward and reduction are not useful persuasive features. While rewards can be useful in for instance serious games (Wattanasoontorn et al. Citation2013), reduction has been identified can be used to decrease the effort that users expend when performing the target behaviour in apps reviewed by (Langrial et al. Citation2012; Wozney et al. Citation2017). Also, some persuasive features (i.e. self-monitoring, personalisation, praise, reminders, suggestion, social role, authority, expertise, and verifiability) were utilised in both successful and unsuccessful interventions. The magnitude of the impact of these features on the effect size of clinical outcomes varied from very small to medium effect.
In addition to the role of persuasive features on the effectiveness of interventions, intervention characteristics such as the technological medium through which persuasive features were implemented to support behaviour change, the duration of use, and frequency of interaction do matter. Reminders, suggestions, content (both educational and metric), feedback, and other persuasive features can also be implemented in many different ways with the different technological mediums, software platforms, and environments. Also, the frequency of interaction or duration of use may influence the extent to which persuasive features are effective.
4.2. Implication for practice
We found that when compared with usual care digital health interventions and the persuasive software features implemented in them can improve clinical outcomes. Our findings agree with research by (Murphy et al. Citation2020) which shows digital health interventions provide a fluent way to support CHD patients. However, further research is required to establish the clinical significance of their use.
4.3. Implications for research
Our research revealed the impact of persuasive software features on intervention outcomes and provides insights into how persuasive features can influence the effectiveness of behaviour change interventions for CHD. Similar research can be conducted in other domains as well. Given that persuasive software features tend to influence the effectiveness of interventions, the need to identify appropriate persuasive features to use is apparent. This gap has also been highlighted by (Wildeboer, Kelders, and van Gemert-Pijnen Citation2016). More meta-analytic research is needed to validate the effectiveness of intervention features. In the same vein, study methodologies such as RCTs are encouraged because they enable researchers to compare the effectiveness of interventions and identify the factors behind them. Again, RCTs provide data for meta-analytic research to investigate intervention features in complex interventions.
4.4. Strength and limitation
In this research, we showed how intervention effectiveness on clinical outcomes is associated with software features designed into a digital intervention and hence can inform the development of successful digital health interventions. The main limitation of this study is that effect size estimates are limited to the number of studies in this research and hence likely to change if more RCT studies are added. Notwithstanding, there was enough data to generate meaningful insights to show the influence software features may have on clinical outcomes and show how such analysis can be done. Another challenge posed was the lack of sufficient descriptions of the intervention content and software features. This challenge has been identified by another study (Halldorsdottir, Thoroddsen, and Ingadottir Citation2020).
5. Conclusion
This meta-analysis shows that digital health interventions can be effective in supporting people to manage coronary heart disease and improve their quality of life. This study showed how intervention effectiveness is linked with persuasive software features implemented in digital interventions. We call for further research to investigate the role of intervention features and other factors in explaining the effectiveness of digital interventions. This will inform the design and development of effective and successful interventions.
Acknowledgement
We are grateful to Mr Fedja Poikonen and and Dr. Franklin Tabughang for helping with the data collection.
Disclosure statement
No potential conflict of interest was reported by the author(s).
References
- Alhasani, M., D. Mulchandani, O. Oyebode, and R. Orji. 2020. “A Systematic Review of Persuasive Strategies in Stress Management Apps.”
- Bhatia, S. K. 2010. Biomaterials for Clinical Applications. New York: Springer.
- Chan, A., R. Kow, and J. K. Cheng. 2017. “Adolescents’ Perceptions on Smartphone Applications (Apps) for Health Management.” Journal of Mobile Technology in Medicine 6 (2): 47–55. doi:10.7309/jmtm.6.2.6.
- Chow, C. K., J. Redfern, G. S. Hillis, J. Thakkar, K. Santo, M. L. Hackett, S. Jan, et al. 2015. “Effect of Lifestyle-Focused Text Messaging on Risk Factor Modification in Patients with Coronary Heart Disease: A Randomized Clinical Trial.” JAMA 314 (12): 1255–1263. doi:10.1001/jama.2015.10945.
- Chow, C. K., J. Redfern, A. Thiagalingam, S. Jan, R. Whittaker, M. Hackett, N. Graves, J. Mooney, and G. S. Hillis. 2012. “Design and Rationale of the Tobacco, Exercise and Diet Messages (TEXT ME) Trial of a Text Message-Based Intervention for Ongoing Prevention of Cardiovascular Disease in People with Coronary Disease: A Randomised Controlled Trial Protocol.” BMJ Open 2 (1): e000606. doi:10.1136/bmjopen-2011-000606.
- Cohen, J. 1998. Statistical Power Analysis for the Behavioral Sciences. New York: Routledge.
- Dale, L. P., R. Whittaker, Y. Jiang, R. Stewart, A. Rolleston, and R. Maddison. 2014. “Improving Coronary Heart Disease Self-Management Using Mobile Technologies (Text4Heart): A Randomised Controlled Trial Protocol.” Trials 15 (1): 71. doi:10.1186/1745-6215-15-71.
- Dale, L. P., R. Whittaker, Y. Jiang, R. Stewart, A. Rolleston, and R. Maddison. 2015. “Text Message and Internet Support for Coronary Heart Disease Self-Management: Results from the Text4Heart Randomized Controlled Trial.” Journal of Medical Internet Research 17 (10): e237. doi:10.2196/jmir.4944.
- Devi, R., J. Powell, and S. Singh. 2014. “A Web-Based Program Improves Physical Activity Outcomes in a Primary Care Angina Population: Randomized Controlled Trial.” Journal of Medical Internet Research 16 (9): e186. doi:10.2196/jmir.3340.
- Ding, M., F. Zhang, and J. Hu. 2022. “Effectiveness of ‘Internet+’ Based Cardiac Rehabilitation on Prognosis of Patients with Coronary Heart Disease: A Meta-Analysis.” Computational and Mathematical Methods in Medicine 2022:1574774. doi:10.1155/2022/1574774.
- Dorje, T., G. Zhao, A. Scheer, L. Tsokey, J. Wang, Y. Chen, K. Tso, B.-K. Tan, J. Ge, and A. Maiorana. 2018. “SMARTphone and Social Media-Based Cardiac Rehabilitation and Secondary Prevention (SMART-CR/SP) for Patients with Coronary Heart Disease in China: A Randomised Controlled Trial Protocol.” BMJ Open 8 (6): e021908. doi:10.1136/bmjopen-2018-021908.
- Dorje, T., G. Zhao, K. Tso, J. Wang, Y. Chen, L. Tsokey, B.-K. Tan, et al. 2019. “Smartphone and Social Media-Based Cardiac Rehabilitation and Secondary Prevention in China (SMART-CR/SP): A Parallel-Group, Single-Blind, Randomised Controlled Trial.” The Lancet Digital Health 1 (7): e363–e374. doi:10.1016/S2589-7500(19)30151-7.
- Frederix, I., N. Van Driessche, D. Hansen, J. Berger, K. Bonne, T. Alders, and P. Dendale. 2015. “Increasing the Medium-Term Clinical Benefits of Hospital-Based Cardiac Rehabilitation by Physical Activity Telemonitoring in Coronary Artery Disease Patients.” European Journal of Preventive Cardiology 22 (2): 150–158. doi:10.1177/2047487313514018.
- Halldorsdottir, H., A. Thoroddsen, and B. Ingadottir. 2020. “Impact of Technology-Based Patient Education on Modifiable Cardiovascular Risk Factors of People with Coronary Heart Disease: A Systematic Review.” Patient Education and Counseling 103 (10): 2018–2028. doi:10.1016/j.pec.2020.05.027.
- Higgins, J. P. T., D. G. Altman, P. C. Gotzsche, P. Juni, D. Moher, A. D. Oxman, J. Savovic, K. F. Schulz, L. Weeks, and J. A. C. Sterne, Cochrane Bias Methods Group, Cochrane Statistical Methods Group. 2011. “The Cochrane Collaboration’s Tool for Assessing Risk of Bias in Randomised Trials.” BMJ 343 (7829): d5928. doi:10.1136/bmj.d5928.
- Huo, X., H. M. Krumholz, X. Bai, E. S. Spatz, Q. Ding, P. Horak, W. Zhao, et al. 2019. “Effects of Mobile Text Messaging on Glycemic Control in Patients with Coronary Heart Disease and Diabetes Mellitus: A Randomized Clinical Trial.” Circulation: Cardiovascular Quality and Outcomes 12 (9): e005805. doi:10.1161/CIRCOUTCOMES.119.005805.
- Huo, X., E. S. Spatz, Q. Ding, P. Horak, X. Zheng, C. Masters, H. Zhang, et al. 2017. “Design and Rationale of the Cardiovascular Health and Text Messaging (CHAT) Study and the CHAT-Diabetes Mellitus (CHAT-DM) Study: Two Randomised Controlled Trials of Text Messaging to Improve Secondary Prevention for Coronary Heart Disease and Diabetes.” BMJ Open 7 (12): e018302. doi:10.1136/bmjopen-2017-018302.
- Islam, S. M. S., C. K. Chow, J. Redfern, C. Kok, K. Rådholm, S. Stepien, A. Rodgers, and M. L. Hackett. 2019. “Effect of Text Messaging on Depression in Patients with Coronary Heart Disease: A Substudy Analysis from the TEXT ME Randomised Controlled Trial.” BMJ Open 9 (2): e022637. doi:10.1136/bmjopen-2018-022637.
- Karppinen, P., H. Oinas-Kukkonen, T. Alahäivälä, T. Jokelainen, A.-M. Keränen, T. Salonurmi, and M. Savolainen. 2016. “Persuasive User Experiences of a Health Behavior Change Support System: A 12-Month Study for Prevention of Metabolic Syndrome.” International Journal of Medical Informatics 96: 51–61. doi:10.1016/j.ijmedinf.2016.02.005.
- Kitsiou, S., G. Paré, M. Jaana, and B. Gerber. 2017. “Effectiveness of MHealth Interventions for Patients with Diabetes: An Overview of Systematic Reviews.” PLoS ONE 12 (3): e0173160. doi:10.1371/journal.pone.0173160.
- König, L. M., C. Attig, T. Franke, and B. Renner. 2021. “Barriers to and Facilitators for Using Nutrition Apps: Systematic Review and Conceptual Framework.” JMIR MHealth and UHealth 9 (6): e20037. doi:10.2196/20037.
- Langrial, S., T. Lehto, H. Oinas-Kukkonen, M. Harjumaa, and P. Karppinen. 2012. “Native Mobile Applications for Personal Well-Being: A Persuasive Systems Design Evaluation.” PACIS 2012 Proceedings.
- Maddison, R., L. Pfaeffli, R. Whittaker, R. Stewart, A. Kerr, Y. Jiang, G. Kira, et al. 2015. “A Mobile Phone Intervention Increases Physical Activity in People with Cardiovascular Disease: Results from the HEART Randomized Controlled Trial.” European Journal of Preventive Cardiology 22 (6): 701–709. doi:10.1177/2047487314535076.
- Maddison, R., J. C. Rawstorn, R. A. H. Stewart, J. Benatar, R. Whittaker, A. Rolleston, Y. Jiang, et al. 2019. “Effects and Costs of Real-Time Cardiac Telerehabilitation: Randomised Controlled Non-Inferiority Trial.” Heart 105 (2): 122–129. doi:10.1136/heartjnl-2018-313189.
- Mittal, S. 2005. “Coronary Artery Disease.” In Coronary Heart Disease in Clinical Practice, 107–150. London: Springer. doi:10.1007/1-84628-135-0_6.
- Murphy, A. C., G. Meehan, A. N. Koshy, P. Kunniardy, O. Farouque, and M. B. Yudi. 2020. “Efficacy of Smartphone-Based Secondary Preventive Strategies in Coronary Artery Disease.” Clinical Medicine Insights: Cardiology 14: 1–7. doi:10.1177/1179546820927402.
- Neubeck, L., G. Coorey, D. Peiris, J. Mulley, E. Heeley, F. Hersch, and J. Redfern. 2016. “Development of an Integrated e-Health Tool for People with, or at High Risk of, Cardiovascular Disease: The Consumer Navigation of Electronic Cardiovascular Tools (CONNECT) Web Application.” International Journal of Medical Informatics 96: 24–37. doi:10.1016/j.ijmedinf.2016.01.009.
- Oinas-Kukkonen, H. 2013. “A Foundation for the Study of Behavior Change Support Systems.” Personal and Ubiquitous Computing 17 (6): 1223–1235. doi:10.1007/s00779-012-0591-5.
- Oinas-Kukkonen, H., and M. Harjumaa. 2009. “Persuasive Systems Design: Key Issues, Process Model, and System Features.” Communications of the Association for Information Systems 24 (1): 485–500. doi:10.17705/1CAIS.02428.
- Page, M. J., J. E. McKenzie, P. M. Bossuyt, I. Boutron, T. C. Hoffmann, C. D. Mulrow, L. Shamseer, et al. 2021. “The PRISMA 2020 Statement: An Updated Guideline for Reporting Systematic Reviews.” BMJ 372. doi:10.1136/bmj.n71.
- Portz, J. D., A. Miller, B. Foster, and L. Laudeman. 2016. “Persuasive Features in Health Information Technology Interventions for Older Adults with Chronic Diseases: A Systematic Review.” Health and Technology 6 (2): 89–99. doi:10.1007/s12553-016-0130-x.
- Poulter, N. 1999. “Coronary Heart Disease Is a Multifactorial Disease.” American Journal of Hypertension 12 (10 SUPPL. 1): 92–95. doi:10.1016/S0895-7061(99)00163-6.
- Prescott, E., E. P. Meindersma, A. E. van der Velde, J. R. Gonzalez-Juanatey, M. C. Iliou, D. Ardissino, G. B. Zoccai, et al. 2016. “A EUropean Study on Effectiveness and Sustainability of Current Cardiac Rehabilitation Programmes in the Elderly: Design of the EU-CaRE Randomised Controlled Trial.” European Journal of Preventive Cardiology 23 (2_suppl.): 27–40. doi:10.1177/2047487316670063.
- Radomski, A. D., L. Wozney, P. McGrath, A. Huguet, L. Hartling, M. P. Dyson, K. Bennett, and A. S. Newton. 2019. “Design and Delivery Features That May Improve the Use of Internet-Based Cognitive Behavioral Therapy for Children and Adolescents with Anxiety: A Realist Literature Synthesis with a Persuasive Systems Design Perspective.” Journal of Medical Internet Research 21 (2): e11128. doi:10.2196/11128.
- Santo, K., C. K. Chow, A. Thiagalingam, K. Rogers, J. Chalmers, and J. Redfern. 2017. “MEDication Reminder APPs to Improve Medication Adherence in Coronary Heart Disease (MedApp-CHD) Study: A Randomised Controlled Trial Protocol.” BMJ Open 7 (10): e017540. doi:10.1136/bmjopen-2017-017540.
- Santo, K., A. Singleton, K. Rogers, J. Chalmers, C. K. Chow, and J. Redfern. 2019. “Medication Reminder Applications to Improve Adherence in Coronary Heart Disease: A Randomised Clinical Trial.” Heart 105: 323–329. doi:10.1136/heartjnl-2018-313479.
- Sawilowsky, S. S. 2009. “New Effect Size Rules of Thumb.” Journal of Modern Applied Statistical Methods 8 (2): 597–599. doi:10.22237/jmasm/1257035100.
- Snoek, J. A., E. I. Prescott, A. E. van der Velde, T. M. H. Eijsvogels, N. Mikkelsen, L. F. Prins, W. Bruins, et al. 2020. “Effectiveness of Home-Based Mobile Guided Cardiac Rehabilitation as Alternative Strategy for Nonparticipation in Clinic-Based Cardiac Rehabilitation Among Elderly Patients in Europe: A Randomized Clinical Trial.” JAMA Cardiology 6: 463. doi:10.1001/jamacardio.2020.5218.
- Spanakis, E. G., S. Santana, M. Tsiknakis, K. Marias, V. Sakkalis, A. Teixeira, J. H. Janssen, H. de Jong, and C. Tziraki. 2016. “Technology-Based Innovations to Foster Personalized Healthy Lifestyles and Well-Being: A Targeted Review.” Journal of Medical Internet Research 18 (6): e128. doi:10.2196/jmir.4863.
- Stevenson, J. K., Z. C. Campbell, A. C. Webster, C. K. Chow, A. Tong, J. C. Craig, K. L. Campbell, and V. W. S. Lee. 2019. “Ehealth Interventions for People with Chronic Kidney Disease.” Cochrane Database of Systematic Reviews 2019 (8). doi:10.1002/14651858.CD012379.pub2.
- Sua, Y. S., Y. Jiang, D. R. Thompson, and W. Wang. 2020. “Effectiveness of Mobile Phone-Based Self-Management Interventions for Medication Adherence and Change in Blood Pressure in Patients with Coronary Heart Disease: A Systematic Review and Meta-Analysis.” European Journal of Cardiovascular Nursing 19 (3): 192–200. doi:10.1177/1474515119895678.
- Wattanasoontorn, V., I. Boada, R. García, and M. Sbert. 2013. “Serious Games for Health.” Entertainment Computing 4 (4): 231–247. doi:10.1016/j.entcom.2013.09.002.
- Wildeboer, G., S. M. Kelders, and J. E. W. C. van Gemert-Pijnen. 2016. “The Relationship between Persuasive Technology Principles, Adherence and Effect of Web-Based Interventions for Mental Health: A Meta-Analysis.” International Journal of Medical Informatics 96: 71–85. doi:10.1016/j.ijmedinf.2016.04.005.
- Wozney, L., A. Huguet, K. Bennett, A. D. Radomski, L. Hartling, M. Dyson, P. J. McGrath, and A. S. Newton. 2017. “How Do EHealth Programs for Adolescents with Depression Work? A Realist Review of Persuasive System Design Components in Internet-Based Psychological Therapies.” Journal of Medical Internet Research 19 (8): e266. doi:10.2196/jmir.7573.
- Yudi, M. B., D. J. Clark, D. Tsang, M. Jelinek, K. Kalten, S. Joshi, K. Phan, et al. 2016. “SMARTphone-Based, Early Cardiac REHABilitation in Patients with Acute Coronary Syndromes [SMART-REHAB Trial]: A Randomized Controlled Trial Protocol.” BMC Cardiovascular Disorders 16 (1): 170. doi:10.1186/s12872-016-0356-6.
- Yudi, M. B., D. J. Clark, D. Tsang, M. Jelinek, K. Kalten, S. B. Joshi, K. Phan, et al. 2021. “SMARTphone-Based, Early Cardiac REHABilitation in Patients with Acute Coronary Syndromes: A Randomized Controlled Trial.” Coronary Artery Disease 32 (5): 432–440. doi:10.1097/MCA.0000000000000938.
- Zheng, X., E. S. Spatz, X. Bai, X. Huo, Q. Ding, P. Horak, X. Wu, et al. 2019. “Effect of Text Messaging on Risk Factor Management in Patients with Coronary Heart Disease: The CHAT Randomized Clinical Trial.” Circulation: Cardiovascular Quality and Outcomes 12 (4). doi:10.1161/CIRCOUTCOMES.119.005616.